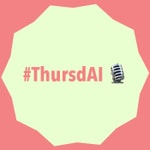
ThursdAI - The top AI news from the past week (From Weights & Biases, Join AI Evangelist Alex Volkov and a panel of experts to cover everything important that happened in the world of AI from the past week)
Explorez tous les épisodes de ThursdAI - The top AI news from the past week
Date | Titre | Durée | |
---|---|---|---|
14 Jul 2023 | ThursdAI July 13 - Show recap + Notes | 01:42:03 | |
Welcome Friends, to the first episode of ThursdAI recap. If you can’t come to the spaces, subscribing is the next best thing. Distilled, most important updates, every week, including testimony and tips and tricks from a panel of experts. Join our community 👇 Every week since the day GPT-4 released, we’ve been meeting in twitter spaces to talk about AI developments, and it slowly by surely created a community that’s thirsty to learn, connect and discuss information. Getting overwhelmed with daily newsletters about tools, folks wanted someone else to do the legwork, prioritize and condense the most important information about what is shaping the future of AI, today! Hosted by AI consultant Alex Volkov (available for hire), CEO of Targum.video, this information-packed edition covered groundbreaking new releases like GPT 4.5, Claude 2, and Stable Diffusion 1.0. We learned how Code Interpreter is pushing boundaries in computer vision, creative writing, and software development. Expert guests dove into the implications of Elon Musk's new XAI startup, the debate around Twitter's data, and pioneering techniques in prompt engineering. If you want to stay on top of the innovations shaping our AI-powered tomorrow, join Alex and the ThursdAI community. Since the audio was recorded from a twitter space, it has quite a lot of overlaps, I think it’s due to the export, so sometimes it sounds like folks talk on top of each other, most of all me (Alex) this was not the case, will have to figure out a fix. Topics we covered in July 13, ThursdAI GPT 4.5/Code Interpreter: 00:02:37 - 05:55 - General availability of Chad GPT with code interpreter announced. 8k context window, faster than GPT-4. 05:56 - 08:36 - Code interpreter use cases, uploading files, executing code, skills and techniques. 08:36 - 10:11 - Uploading large files, executing code, downloading files. Claude V2: 20:11 - 21:25 - Anthropic releases Claude V2, considered #2 after OpenAI. 21:25 - 23:31 - Claude V2 UI allows uploading files, refreshed UI. 23:31 - 24:30 - Claude V2 product experience beats GPT-3.5. 24:31 - 27:25 - Claude V2 fine-tuned on code, 100k context window, trained on longer outputs. 27:26 - 30:16 - Claude V2 good at comparing essays, creative writing. 30:17 - 32:57 - Claude V2 allows multiple file uploads to context window. 32:57 - 39:10 - Claude V2 better at languages than GPT-4. 39:10 - 40:30 - Claude V2 allows multiple file uploads to context window. X.AI: 46:22 - 49:29 - Elon Musk announces X.AI to compete with OpenAI. Has access to Twitter data. 49:30 - 51:26 - Discussion on whether Twitter data is useful for training. 51:27 - 52:45 - Twitter data can be transformed into other forms. 52:45 - 58:32 - Twitter spaces could provide useful training data. 58:33 - 59:26 - Speculation on whether XAI will open source their models. 59:26 - 61:54 - Twitter data has some advantages over other social media data. Stable Diffusion: 89:41 - 91:17 - Stable Diffusion releases SDXL 1.0 in discord, plans to open source it. 91:17 - 92:08 - Stable Diffusion releases Stable Doodle. GPT Prompt Engineering: 61:54 - 64:18 - Intro to Other Side AI and prompt engineering. 64:18 - 71:50 - GPT Prompt Engineer project explained. 71:50 - 72:54 - GPT Prompt Engineer results, potential to improve prompts. 72:54 - 73:41 - Prompts may work better on same model they were generated for. 73:41 - 77:07 - GPT Prompt Engineer is open source, looking for contributions. Related tweets shared: https://twitter.com/altryne/status/1677951313156636672 https://twitter.com/altryne/status/1677951330462371840 @Surya - Running GPT2 inside code interpreter tomviner - scraped all the internal knowledge about the env Peter got all pypi packages and their description swyx added Claude to to smol menubar (which we also discussed) SkalskiP awesome code interpreter experiments repo See the rest of the tweets shared and listen to the original space here: https://spacesdashboard.com/space/1YpKkggrRgPKj/thursdai-space-code-interpreter-claude-v2-xai-sdxl-more Full Transcript: 00:02 (Speaker A) You. First of all, welcome to Thursday. We stay up to date so you don't have to. There's a panel of experts on top here that discuss everything. 00:11 (Speaker A) If we've tried something, we'll talk about this. If we haven't, and somebody in the audience tried that specific new AI stuff, feel free to raise your hand, give us your comment. This is not the space for long debates. 00:25 (Speaker A) We actually had a great place for that yesterday. NISten and Roy from Pine, some other folks, we'll probably do a different one. This should be information dense for folks and this will be recorded and likely we posted at some point. 00:38 (Speaker A) So no debate, just let's drop an opinion and discuss the new stuff and kind of continue. And the goal is to stay up to date so you don't have to in the audience. And I think with that, I will say hi to Alan Janae and we will get started. 00:58 (Speaker B) Hi everyone, I'm NISten Tahira. I worked on, well, released one of the first Docker chat bots on the market for Dr. Gupta and scaled it, and now we're working on getting the therapist bought out once. We can also pass more testing and get Voice to work at a profitable manner because we don't really have VC. So at the scale of few hundred thousand users, the API bills matter quite a bit. 01:31 (Speaker B) So, yeah, these spaces have been pretty helpful because I have some trouble with running a Voice transformer, trying to run it on the browser on web GPU, and then the person that wrote Transformers JS comes in here and just says, oh yeah, that back end is messed up. Just try blas and synth and stuff. So these have been very interesting and technical spaces. 01:54 (Speaker A) Yeah, we need to get Zenova in here. Zenova is the guy who NISten was referring to. Al Janae, do you want to give a few words of intro and say hi and then we'll start? Just briefly, please, because I think we need to get going. 02:09 (Speaker C) Sure. Hi, I'm Janae. 02:11 (Speaker D) I'm the resident noob, I started messing around with AI at the beginning of. 02:16 (Speaker E) The year, and I also host the. 02:18 (Speaker D) Denver AI Tinkerers coming up next week. 02:20 (Speaker A) And if you're in Colorado area, greater Denver, please join us. It's going to be a blast. 02:27 (Speaker F) Hi, I'm Al Chang. I'm kind of an old school technologist. Just getting started with the AI again and just here to help. 02:36 (Speaker A) Yeah. All right, folks, so I think we've had a whole space on this. Simon Wilson and me and many, many other folks chimed in. The second this was released. 02:50 (Speaker A) Was that six? Was that Sunday? It's hard to keep track of actual days. Saturday, Saturday, last week, exactly during those spaces, by the way, as we were talking, Chad GPT, Logan and everybody else from OpenAI announced general availability of Chad GPT with code interpreter. So GPT four with code interpreter. 03:12 (Speaker A) And I think we just heard from Matt that even some folks who got access to the slept on it a little bit because it's maybe potentially because of its very horrible name that's really hard to type interpreter and get lost in the R's. But it's an extremely powerful new superpower that we've got. And we've had the whole space talking about use cases that people already had. 03:37 (Speaker A) It was like three days into it and since then I bet that many more people tried it. I think Swyx 20,000 listens to that space, plus the pod. At least people definitely want to hear new use cases, right? 03:53 (Speaker G) Yeah, not much else to add about it. I think it's the feature for Switch. 03:59 (Speaker A) Posted a whole deep dive essay and coined it GPT 4.5 between us friends. And one of the interesting things about it is that we think at least that's where we are currently after playing around with this, is that it's a fine tuned model. So they kept training this on actually running code and executing code. 04:21 (Speaker A) That's what we believe. We don't know, nobody confirmed this and then that it's fine tuned from an earlier checkpoint of GBT Four. And so we actually had some folks on spaces talking about that it's less restricted and better like previous times. 04:36 (Speaker A) So it's an interest, I think NISten right. We have some folks who tell us they're using code interpreter without the code part. They just stopped the GPT Four just because it's that model. 04:48 (Speaker A) And I think also they took down the 25 messages per hour restriction on code interpreter. I've had like four hour sessions and it stopped like I didn't saw complaints. 05:03 (Speaker G) So it's just better. 05:06 (Speaker A) It's also fast. I think it's fast because not many people maybe use this by default and this could be the reason for the speed, but it's definitely faster for sure. I think also context window, was it Yam? Somebody summarized the context window and they told us the context window for code interpreter is eight k versus the regular GPD for actually that could be also a kick. 05:29 (Speaker G) You mean Yam copied and pasted. 05:34 (Speaker A) I would encourage you and Yam need to kiss in the cup because Yama is doing a lot of legwork to take down the stuff that he posted and Yama is working on that and it's very visible and you guys need to do there you go, yam, you need to clear the air. However, Pharrell and Gabriel bring you up as well. And we're going to keep talking about code interpreter because that's what we're here to do. NISten and a few other folks and we started cooking with code interpreter. 05:59 (Speaker A) And by cooking I mean we started stretching the complete boundaries of what's possible there. And I think Simon Willison kick started this with the latent space Pod. So for folks who are not following latent space pod, feel free to follow SWIX, his main account, not this hidden one. 05:59 (Speaker A) And SWIX reposted the spaces we had simon Wilson was able to run node JS and Dino within code interpreter, even though OpenAg didn't allow for that by uploading like a binary and asking code interpreter to generate. Simon then promptly said they fine tuned the model away from that and we found ways anyway to ask it to do some stuff. I have a thread on how I was able to run a vector DB chroma inside code interpreter. 06:10 (Speaker A) I ran whisper CPP. We saw some folks running GPT-2 inside code interpreter, right? So imagine an Ll GPD Four running another and talking to it. It's like a little brother inside. 06:10 (Speaker A) I personally love that inception. I don't know if the person who ran GPD Two is in the audience as Dan I think was the nickname NISten. I don't know. 07:22 (Speaker A) Surya. 07:23 (Speaker B) Surya. He also wrote the search to PDF plugin for GP Four plugins and he wrote that in like two days and it's more used than any other enterprise thing, which is pretty hilarious. 07:36 (Speaker A) We need to get surya. 07:38 (Speaker B) Yeah, he just did that as I'm just going to do a search plugins for PDF and it's like the most used. 07:45 (Speaker A) So dope pretty amazing. Again, in that space we've talked about having like a living manual, so to speak, for code interpreter use cases because it's coding. So it covers pretty much everything that we can think of as coders, maybe just in Python, maybe restricted to an environment. And I've been trying to do that with the code interpreter can hashtag and I encourage all of you, let me pin this to the top of the space, to the jumbotron if you have an interesting code interpreter thing and I'll bring up Skalsky P to the stage as well. 08:03 (Speaker A) And Lantos, so many good friends. If you have a very interesting code interpreter technique or skill or new thing that people can do without coding skills, please tag with this hashtag so folks can find this. Otherwise I will cover the main three things the code interpreter gave us besides the new model. 08:42 (Speaker A) One of them is uploading files. And since we've talked, we've noticed that you can upload up to 250 megabyte files and those can be zips of other files. So we've uploaded like full models weights. 08:55 (Speaker A) We've uploaded bin files. It's incredible that you can now drag and drop whole directory and have JPT just know about this and read about this. We've uploaded weights in embeddings. 09:08 (Speaker A) You can then obviously execute code in a secure environment, which is again incredible, and you can download files, you can ask it to actually generate a download for you, which is also super, super cool. Maybe one last thing I'll say before I'll give it to the audience for a few more cool use cases. And folks in the stage, please feel free to raise your hand. 09:21 (Speaker A) I'll get to you in the order that you raise your hand if you have a use case. Some folks built like a built in memory built in brain within code interpreter just to save to a file. That's what I try to do with my vector DB and then they download that memory at the end of every session and then upload this to the next one and have some like a prompt that reminds the jgpd like to start from that point. 09:50 (Speaker A) So in addition to the context window, they're also having a separate offloaded file persisted memory. So code interpreter incredible. Again. 10:00 (Speaker A) Potentially GPT 4.5. And if you haven't played with this, feel free to if you don't know what to play with, follow the code interpreter can hashtag and let's get to Skowski. 10:11 (Speaker A) What's up, man? 10:14 (Speaker H) Hi, hello. Do you hear me? 10:15 (Speaker A) Yeah, we can hear you fine. 10:19 (Speaker H) Yeah, I've been playing a lot with code interpreter over the past five days, mostly with computer vision use cases because that's what I do. I haven't introduced myself. I'm pretty much doing computer vision full time for the past five years and was focusing on like when I saw that you can input image and video, that was immediately what I was thinking, we need to make it to computer vision. So I went through some low effort tasks. 10:46 (Speaker H) So I managed to run old school computer vision algorithms, face detection, tracking of objects, stuff like that. But I also managed to exploit it a little bit. So you can add yolo object detection models to the list of models that were run in code interpreter. 11:15 (Speaker H) There are some problems with memory management, so I'm not yet fully happy with the result. But yeah, I managed to run it on images and on videos and the things that are super cool and are kind of like underrated right now, false positive. So when the model detects something that shouldn't be detected, you can really use text to ask code interpreter to filter out false detections. 11:48 (Speaker H) You can just give it your feeling like why that stuff is happening or when or where. And it's very good at cleaning the detections, which was kind of like mind blowing for me. And one thing that I noticed that it sucks at is I managed to create an application that counts objects moving on the video when they cross the line. 11:55 (Speaker H) And I didn't use any off the shelf libraries, I just had detector and say, okay, now draw a line and count objects when they cross the line. It's terrible at that, writing math logic to figure out that something crossed something, we had like ten prompts or twelve prompts exchange and I basically bailed out on that, forget it. So there are some things that blow my mind, but there are something that probably not. 12:49 (Speaker A) So folks, feel free to follow Skowski. And also I just pin to the top of the Tweet his brand new awesome code interpreter use cases, git repo, and there's a list, there's a bunch of use cases there. This could also serve as a de facto manual. So feel free to go there at PRS and follow that for updates. 12:52 (Speaker A) And I want to get to Lentos because he seems to be unmuting. What's up, Lentos? 13:12 (Speaker H) I was just going to say I can't follow him because he's blocked me. 13:15 (Speaker C) Sad face. 13:16 (Speaker H) Oh, no, I noticed that, but I'm not sure why. I will undo that. 13:20 (Speaker A) All right, I'm the peacemaker in the status. Please kiss and make up. You two as well. Everybody should get along. 13:26 (Speaker A) Yay. I want to get to some other folks who came up on stage recently. And Gabriel, welcome to talk about code interpreter and your use cases. 13:35 (Speaker A) Jeanette, if you play with this, I would like to hear two more opinions before we move on to the next incredible thing. Yeah. Oh, you guys are talking about let's get together and then June sorry, I should have been explicit about the order. 13:54 (Speaker E) No worries. So I just posted a comment on this space about the message cap on a conversation. So even though in the UI, it still says 25 messages per 3 hours, if you look at the network request, you can see that. And I posted this, it's actually 100 messages per 3 hours now. 14:12 (Speaker E) And I don't know if they're scaling that up and down as demand increases and decreases, or they're just trying to trick people into conserving their messages, but it's definitely been on 100 for a little while now. Can you confirm same thing you can see in the network? 14:32 (Speaker A) Can you confirm the same for the regular mode, or do you think the regular mode is still restricted? Well. 14:41 (Speaker E) Based on just the fact that there's only one message cap, they don't have message cap per model. So I think it's just consistent across all the GP four models. And that's also my experience in the last it's been a little while now. It's probably at least a couple of weeks that it's been higher. 14:51 (Speaker E) And same thing we discussed, I think, on Saturday about the context window. And you can also see it in the API that the context window is eight K for plugins and code interpreter, and it's 4K for the base GPT four model. 15:16 (Speaker A) That's awesome. Like suicide. Better in every single way. 15:22 (Speaker D) Yeah. 15:23 (Speaker A) Awesome. Thanks. 15:24 (Speaker E) Yeah. In terms of use cases I can share, I've been digging around a lot in the code interpreter, and I was really trying to hone in on why are the packages that are installed there, the Python packages in the environment? Why are they there? Some of them seem really random, and some of them make a lot of sense. And they released it, saying it's for, basically data analysis. And a lot of them make sense for that, but some of them are just really wild, like the ML packages. 15:54 (Speaker A) And the Gabriel folks in the audience. If you look up at the jumbo tone where we pin Tweets two Tweets before there's a Tweet by Peter Zero Zero G, who actually printed all the packages and asked GPT Four to kind of summarize what they do. So if you have no idea about the potential capabilities of what it can do, feel free to pin that tweet for yourself. And then it has a bunch of descriptions of what's possible. 16:11 (Speaker A) So go ahead. Gabriel. Yeah, cool. 16:28 (Speaker E) Yeah, I've done the same kind of thing with just a short yeah, I got it to do a four word description for each one. So if you're looking for a really short description of each package, I'll post that tweet. And if you're looking for a long one, I think Peters is great. And what you can see there is that there are packages for web development, right? There's Fast API, there's Flask, there's a bunch of other packages for Web development. 16:40 (Speaker E) And besides the fact that there's no network access, which obviously other people using it might be turning it on, but it was just interesting to me. My perspective is that OpenAI has been using this internally throughout all their teams for development and testing it internally, but probably also using it pretty consistently. They probably have access to the Internet. 17:14 (Speaker A) Yeah, I'm sure they have access to. 17:15 (Speaker E) The Internet and they can install new packages. But I think they also have the ability, instead of uploading files and downloading files, they have the ability to just mount persist memory, I don't think, to persist. I think they just mount their local working directory on their computer right wherever they're working. So they have their active directory where they have their project, and they just mount that and give the code interpreter access to the whole directory with their whole repo of their project. 17:48 (Speaker C) Yeah. 17:48 (Speaker E) And then Chat Gvt is just writing code to the working directory and reading from there and it can explore their whole project. We can do that now by uploading, you can zip your whole project and upload the whole thing zipped and have it unzipped. And then it can kind of explore your whole project. But then once it makes some changes, you want to commit them, you have to ask it to zip the whole thing back, download it and upload it. 17:48 (Speaker E) And then I think what they're able to do is more of like a kind of peer programming thing where the developer makes some changes and then Chat GPT makes some changes and they're kind of working together. This is taking it one step further. I don't know if they have this or not, but it would be super. 18:29 (Speaker A) Cool in the realm of updates unless there is no speculation. But I would love to explore this more with you in the next stage because this applies to open source and how people already saw somebody tag us after the last space and said, hey, I'll build this open source. I would love to pin this to the top of the space. However, I want to move on to new space and then move on to other updates. 18:51 (Speaker A) Sorry to interrupt, but thanks. I think that the collaborative, persistent code superpower that probably maybe at some point will come to us as well. Plus the internet access is like another ten x I want to get to Skowskin and lent us and I think we'll move on to Claude. 19:08 (Speaker A) Thanks Gabriel. 19:11 (Speaker H) Yeah, I have a question. I'm not really sure guys, if you notice that I was obviously experimenting with PyTorch because I needed it for computer vision. I noticed that the PyTorch version that is installed in the environment actually pre compiled to work with CUDA. So it's a GPU version of PyTorch. 19:31 (Speaker H) Even though that in the environment you don't have access to GPU, you only have CPU. So I'm curious guys, what you think about that. Why is that? Any ideas? 19:42 (Speaker A) Ideas that just come from what Gabriel just said? Likely we're getting the same Kubernetes container. However, the open AI folks have like unlimited stuff. They probably also have CUDA that would make sense right there is probably connected to a GPU as well, but that's just an idea. Lantos, I want to get to you and then we'll move on to Claude. 20:02 (Speaker A) Folks and folks in the audience, feel free to hit the little right button on the bottom left looks like a little message and leave comments through commenting as well. Moving on to Claude V Two. Folks in the audience and folks on stage, feel free to hit up the emojis plus one. 20:19 (Speaker A) Minus one if you have tried Claude V two if you like it and you haven't liked it. I'm going to cover this anyway because I think somebody called me, I think Roy from Python called me a Cloud V Two fanboy yesterday and I first got offended and I told him that I'm just a fanboy for 24 hours. Before that I was a code interpreter fanboy and then I figured with myself whether or not I am a fanboy of Claude V Two. 20:43 (Speaker A) And yeah, I am and Sweet told me to relax and in fact I invited him here to be the red blanket on the other side of the list. Anthropic the company that we can definitely consider number two after opener. I think that's fair in terms of quality. 21:02 (Speaker A) Have long released Claude version and they made some ways when they released Claude AKS clong with 100K complex window, they have released Cloud V Two and let me paste some Claude sorry, pin some Claude thingies in the jumbotron, sorry. However, Cloud V Two released with multiple stuff and I want to focus on two stuff and I think we'll cover the UI first and then we're going to talk about the model itself, UI wise and product wise. My hot take and I'll pin this to the top. 21:38 (Speaker A) Unfortunately not debate this, but I love you, all of you. Is that as products, Cloud V Two right now beats JPD as a product. My mom can go into two websites and she'll prefer one versus the other one. 21:51 (Speaker A) Or my friends that don't know Xai as plugged in as we are, theirs is free. And I think Cloud V Two beats GPD 3.5, which is also free, and 100K context window with the model being traded, 200 unleashes, a bunch of use cases that were not possible before. 22:12 (Speaker A) It just frees you up. If you heard Skowski just say the limitations of code interpreter. A bunch of these limitations stem from the eight K context window. 22:13 (Speaker A) If you print a bunch within the code that you're doing, code interpreter sometimes forgets what you guys talked about 20 minutes ago. And the 100K context window also means a long, long conversation history with the model. And I think it's really great. 22:37 (Speaker A) Not to mention that you can drag and drop full books in there. Those books need to be in like one or two files and they still don't accept zip files. And I'm planning to release an extension soon that does this for us and unifies and single files. 22:51 (Speaker A) So hopefully by next week we'll have some updates. However, once you upload that much or you can upload like a transcript or a podcast, you can do a bunch of stuff because Cloud V Two is also better trained on code and we saw a significant jump in wait, I'm switching to the model, so let me get back to the UI. The UI allows you to upload files. 23:09 (Speaker A) The UI has a command k interface, which I personally love. I hit Command K in every website and see if they support it. You can just start a new chat real quick. 23:21 (Speaker A) It doesn't have Share, but it's definitely refreshed and free UI. It's called Cloud AI and that's the URL, and if you haven't tried it, definitely try it. Comments about just the product side and the UI side before we move to the model? Anybody play with this? Anybody like it? Anybody loves the upload files feature? I would love to hear hands and comments. 23:42 (Speaker A) Go ahead, Matt. 23:44 (Speaker D) A bit of a weird thing, but what I've noticed is it's actually quite frustrating if you want to paste text in it actually, if it's over a certain length, will paste in as a file. Little small thing. Hopefully they'll change it, but it is really annoying because then you can't edit it. Chat GP does do that much better, but I generally agree with you that overall the product experience on Claude is. 24:03 (Speaker A) Significantly the new one. The fresh coat of paint they released for us. I will say that Cloud so far was kind of a hidden gem, that only folks who got access to the API actually got access to their UI, and that UI was very restricted and folks who have access to Cloud API know what I'm talking about. I think that UI is still around. 24:22 (Speaker A) It still shows your history. It's like very restrictive. It's not as cool as this it's not as leak as this. 24:27 (Speaker A) So we like cloud AI, definitely a plus. Check it out. Now, let's talk about the model behind this UI, because that model also changed and several incredible things that changed with it. 24:38 (Speaker A) First of all, they released a new model, same price as the previous one. We love to see this. Please everybody, including opinion, continue giving the same price and cheaper and cheaper down the line. 24:41 (Speaker A) We love to see this. Second of all, they claim it's been fine tuned on several things. One of them is code. 24:54 (Speaker A) And we actually saw a bump in the evaluation called Human Eval, which is a set of questions that OpenAI released and I think the bump was from like 55% to 78%, which I think beats 3.5 and is not there compared to GPT four. Correct? 25:14 (Speaker C) Yeah, and four and four on past first on the first, not on GPT four that is allowed to refine and fix it there, but on the first trial. Yeah, by a little bit. 25:33 (Speaker A) So, news to me and thank you for joining in the past numbers is how many times it's able to reflect upon the sensors and improve them. 25:43 (Speaker C) The past time is kind of what I meant by reflection is even stronger GPT four. If GPT four sees the exception, it can come up with a solution. So this is not in the Human Eval test, but if you use GPT four this way, you get to 90 something percent, which is which I think it's more realistic if you think about it. No programmer writes the whole code in a one go. 26:10 (Speaker C) You write it intuitively, six bugs and so on. And also in code interpreter, you see it. But it is remarkable to see state. 26:19 (Speaker A) Of the art on first and it's significantly better in code. And I suggest folks who previously tried quad and haven't impressed to try as well. An additional crazy thing that they've trained on is 100K contacts window and they've actually trained, they claim on 200K contact window, so twice as much as the previous round. And we follow this one guy of your press, the guy behind Self Ask with Search and the guy behind Alibi, the ability to extend complex windows. 26:55 (Speaker A) He just defended his PhD and he talked about complex windows and he was impressed with the way they presented and the way they showed their loss curve. And so this could be we saw the paper maybe this week the folks saw the paper where the window dips in the middle. There's like less attention in the middle of the beginning at the end. 27:03 (Speaker A) And it looks like that's not the case for Claude as well. So I suggest you try the huge context window and al you have your raised hand and then we'll talk about some other model changes. 27:26 (Speaker F) Yeah, I would talk a little bit about I used Claude about a month and a half ago to win Best Solo Hacker at the Craft Ventures hackathon david Sachs won. Yeah, it had like 200 entries, but it's exceptionally good at creative writing and also like comparing and contrasting. I don't think people have really taken advantage of what the context window is capable of doing. It's more than just loading single files in. 27:53 (Speaker F) So what I did for the project was I loaded these large legislative bills, these like 50 page unreadable bills, and you turned them into relatable narratives. So one of the things that Claude can do is you can adopt a persona. So a lot of times with summaries, summaries just compress the text that you see, but you can tell it to say, write 1000 words from a social conservative point of view, or a bus driver's point of view, or a social liberal point of view. 28:21 (Speaker F) And what that does is it takes all of its knowledge about the outside world and gives you not a summary, but it gives you essentially an essay about the practical effects of something like a bill. I've actually been working with the idea of reading a book and having it tell you what I would have learned from this, because that's actually probably what you're more interested in. What it can do in terms of comparing and contrasting large essays is exceptional. 28:51 (Speaker F) So you could have it say, write 2000 words from a social conservative point of view, 2000 words from a social liberal point of view, and then have it contrast the essays, which is something that would be very difficult for a human to do. So you get to give it multiple files and have it just give you a more balanced approach so you get rid of some of the bias that comes in. 29:18 (Speaker A) My dream, go to my dream project that I never get to is to create this for Twitter as like a Chrome extension that I can select a bunch of tweets and then say, remove the bias from this and just give me the debiased version of all of this. Yeah, completely. Like the cross reference ability of Cloud between because of this context window is incredible for many, many use cases. 29:41 (Speaker F) Yeah, I would say that as far it's not as good as GPT Four for certain things. But that context window is fantastic. And I would say a lot of people that are using embeddings and retrieval, you can actually just put the whole thing in the context window and ask questions to that and then you have a baseline to compare your results from it. Most people, if they're chatting to a website or something like that, you actually can just put the whole thing in there as opposed to trying to chunk it up and do questions and you'll see that your results are much better that way. 29:51 (Speaker F) And for most people, that would be good enough. 30:17 (Speaker A) So additional thing that the additional thing that Cloud was trained on, they've talked about the output tokens, just the number. Of output tokens of how much cloud is able to generate. And they've said that previous models, I don't know if the same about GPT, I haven't seen numbers on GPT Four, but they've said that previous Claude models were focused on shorter outputs just as they were trained. And this latest model was trained to output up to 4000 tokens in output. 30:47 (Speaker A) This is added to the fact that they also fine tuned it and trained to output JSON files, complete JSON files as responses, which we as engineers, we waited for this and Open Xai gave us functions via kind of here you go, there's the function interface. And we love the function interface. The function interface kind of locks us down to the OpenAI ecosystem. 31:04 (Speaker A) And it's great to see another model that's like very close to state of the art in human evil that also is now fine tuned to respond in full intact JSONs. And those JSONs can be 4000 tokens at length. Any thoughts on these? 31:28 (Speaker F) Yeah, I can confirm on it being able to write large amounts of output. I mean, I was having it write like 2000, 3000 word like sort of essays and outputs and it was fine with that. 31:40 (Speaker A) Yes. And I think it's I'm going to. 31:45 (Speaker B) Stick with GPT Four myself. But this might be pretty useful for just dumping in an entire code base, given the 100k context window and then getting some reviews and stuff, and then maybe moving some of the stuff. 32:02 (Speaker A) Once I stop posting status and build that chrome extension that you upload the zip and it flatlines it to one file and then upload it, then we'd be able to do, like, a proper comparison, because code interpreter can take zip files and then extract them. Oh, one difference that I want to for folks in the audience, GPD Four with code interpreter allows you to upload zip files, et cetera. We talked about this. It does not load them into context window, right? So there's like eight k context window. 32:30 (Speaker A) The files that you upload are not automatically in the context window. The model doesn't it has to write Python code that actually prints the files. And it usually does like the first few lines, hint, hint. 32:30 (Speaker A) The folks in the audience who get my drift. But it doesn't usually read all the unless you specifically ask it to and Claude does. So everything you upload to, Claude goes directly to the immediate working memory of the complex window. 32:38 (Speaker A) And that's a major difference to watch out for and also take care of. Go ahead. 33:00 (Speaker C) I would like to ask everyone before I say my opinion, what do you think about it in comparison to GPT Four about the performance? What do you think? 33:10 (Speaker A) I would like comments from folks who actually use both and did the comparison. And before I get to folks, please raise your hand to answer. I want to call out SWIX's small menu bar which allows you to actually Swyx. Can you give us like a brief two minutes on the menu bar thing? 33:28 (Speaker G) Yeah, well, you don't have to choose. Just run it all the time on every single chat. So it's a little electron app that runs in the menu bar. And I've been maintaining it and I just added Cloud Two this week. 33:42 (Speaker G) Cloud Two is not super stable yet. Sometimes it will fail to submit the button. So you just have to retry manually to submit the button. 33:50 (Speaker G) But yeah, it's a great way to a B test models, but then also just amplify every question with between four to five different chat models with the answers. So I've been trying it. It's up to you if you want. 34:07 (Speaker A) To. 34:10 (Speaker C) Find it. 34:14 (Speaker A) With the announcements, if you can. Yeah, awesome. Yeah, just basically and maybe for instance, you don't have to stop using, you don't have to choose. So I think the last thing that we need to acknowledge it's, Claude, is the multilinguality. 34:28 (Speaker A) So they actually focused on showing us how much better, like, the new ones from previous ones, and they posted blue scores, Bleu scores, clock Two is significantly better at languages than the previous versions. I think, to answer your question, I think it's close to GPD Four, if not better at some things. Hebrew goes fluently, and usually Hebrew is not that great. 34:57 (Speaker A) Russian and Ukrainian that I use also go fluently. And that part is really good with a lot of context because you sometimes need to do a lot of translation, or at least I need to do a lot of translation. 35:11 (Speaker C) Yeah, multilinguality works great. I was surprised. Absolutely. What I think if you just compare the two on the same prompt, the same question, I have a feeling that GPT Four is slightly better, but I just don't have an example to tell you. 35:31 (Speaker C) Okay, here I don't know, it's a strange situation, but I really wanted to ask you, like, what did you try and work better here and there? 35:38 (Speaker A) So here's my use case that GPT Four currently cannot do. Yesterday, Lex Friedman interviewed Israel's Prime Minister Benjamin Netanyahu in one of the weirdest turns of history this podcast was, and given that I know kind of who Benjamin Netanyahu is from, before I decided to not listen to this, I decided to use the tools that we have at our disposal. So I ran this through Whisper with Diarization. So I have, like, a very nice transcript of who's talking. 36:10 (Speaker A) When I took that, I just dumped this as a text file. And I agree with Matt, it's a little bit annoying that Claude turns whatever you paste into like, a little text file uploads. That because you can't edit it. 36:21 (Speaker A) However, I uploaded that transcript directly to Cloud, and then I asked it to do sentiment analysis, entity extraction, and sentiment analysis and entity extraction. Something that if I'd asked GPT code interpreter, it would probably write some Python code to do this, and Quad just kind of did it. And I haven't seen GPT Four being able to do this for bigger files. 36:38 (Speaker A) And once I could just let me just this point. I continued by saying, hey, because of the new coding abilities of Quad, I asked it like, hey, print me a Python file that dumps whatever table of topics he mentioned and sentiment, negative, positive, dump it into a word cloud. That's something the code interpreters can actually do and show you. 37:03 (Speaker A) But I asked it from Quad because previously Claude was s**t at coding and it gave me Python files that ran from the first time. I didn't have to change anything, there was no bugs. And then showed me a word cloud of everything that was mentioned by BB in that podcast and it all took like maybe seven minutes. 37:11 (Speaker A) And I don't know if for bigger complex windows, GPT Four can currently do this. Go ahead, Al. 37:28 (Speaker F) Yeah, I've actually been putting a lot of transcripts for podcasts in there and you can actually have the because it seems so much about the speakers and it knows about the speakers, you can actually have them continue a discussion about things that they didn't actually discuss. Yeah, so it's like you can have it say, okay, well, what are some topics they disagreed on and then some things that they didn't cover? Tangentially, you can just have it give you another two minutes of interview and it does a pretty reasonable job, especially with public figures that it actually has a lot of their background on. So it's pretty interesting. 38:01 (Speaker A) And not to mention free, ngbt Four needs a $20 a month payment and quality is free. 38:08 (Speaker F) That's a good point, too. For those of you that have eval keys, you'll notice that they're actually not charging you for them, so you can actually go on as long as you want. The limitation is that you can only do one request per organization. So if it's just a single person, they only charge you basically when you start deploying for commercial purposes. 38:21 (Speaker F) So that's something that people may not have realized. 38:32 (Speaker A) So I think we've covered everything right, trained on 200K context, which they can enable tomorrow for us, and we'll get like two X. It's going to be insane. There is some stuff that they have in Cloud in a tropic called Constitution AI, so they have a mix of Rlhf access and Constitution AI. So they're working on their model to actually be more helpful, but also more safe and less jail breakable. 38:57 (Speaker A) They talked at length about this. We talked about human evil better and same price and free playground. I think we've covered most of it. 39:03 (Speaker A) So anything else about Quad that we haven't covered, feel free to raise your hand and tell us, and if not, I think we can move on. What do you guys think? 39:17 (Speaker G) I'll mention briefly, did you talk about the multiple file uploads? 39:21 (Speaker A) No, go ahead. 39:24 (Speaker G) So I think it's just an interesting way difference between co interpreter and Claude code interpreter. You can only upload one file, right? But it can be a zip file with multiple files in Zion. So it's de facto multiple files, but then you can only run code on that. Whereas what Cloud here is doing is something slightly different, which is to me is interesting, which is you can upload multiple files, it just reads the file straight into the context and it's using that 100K context to synthesize answers. 39:24 (Speaker G) So you can do, for example, PDF A and PDF B and give me a comparison between the two of them or synthesize knowledge across them. And I think that is something that code interpreter cannot do because code interpreter will only run code across files. So I think that's noteworthy. 40:15 (Speaker G) It's called genuinely coming up with one new thing that is not copying chat GBT and good for them. 40:23 (Speaker A) Yeah. And unfortunately no zip allowed. But we're going to fix this with an extension and hopefully talk about this next week. I want to say hi to Weather Report. 40:33 (Speaker A) Feel free to chime in. Sorry you raised your hand open to come up before. So if you have a comment about code interpreter, we've moved past it, but if you have a comment about Claude, feel free to tell us what's up with the report. 40:46 (Speaker A) Actually, I had only one thing about code interpreter that in the previous space I talked about that there was a hypothesis I had about code interpreter, which. 40:56 (Speaker B) Is to use it as a huddle because it's recorded. 40:59 (Speaker A) We'll move on and let's talk about code interpreter next time. I think that some folks are saying that their audio is glitching and so they're not able to and I want to see if I think Joseph has comment about code interpreter. Joseph Polak. We'll give him a second to log in and then I think we'll move on to other updates because we have many other things to talk about. 41:29 (Speaker A) What's up, Joseph? Welcome to stage. 41:31 (Speaker G) Hi there, folks. 41:33 (Speaker A) Thanks for taking my question. I didn't even know all about that code interpreter stuff with the file. 41:40 (Speaker G) So I'm really happy to have heard it. About Cloud, though. 41:46 (Speaker A) For Cloud. Well, I'm still on waitlist. First of all, it's free now. You can access it right now. 41:53 (Speaker A) Cloud AI. There's no waitlist anymore unless you live in the States and you'll have to get a VPN. Okay, I'll definitely check that out. 42:03 (Speaker A) My question was about using Cloud and actually code interpreter through API. Do you think that's ever going to exist or if it's coming so clogged API? But I think that's waitlisted. I have talked with Claude folks and they said the waitlist is now going faster. 42:24 (Speaker A) So they are ready to get more people in. I think because of the new safety updates, they're less afraid. So definitely apply for the waitlist on quads account. 42:35 (Speaker A) Code interpreter is not available via API, and we've seen some folks who hack it together with like, I think a browser plugin that proxy something. Sweets I don't know if you remember the unofficial quote unquote code interpreter API and it's how to access this, but it's not available in the official OpenAI APIs as of yet. We haven't seen them. 42:56 (Speaker G) No. For the record, there's no unofficial code interpreter API. There's the browser side thing that we are trying to but nobody's made any. 43:07 (Speaker D) Adapter for it yet. 43:08 (Speaker G) I think you can, if you want, using puppeteer. 43:12 (Speaker A) I would not recommend definitely, if anything, there was some folks that tagged us and I need to go and find this that they're working on like an open source version of code interpreter that uses laws and stuff. And that one this will likely be the way forward. If you do want something programmatic that has code interpret capabilities, go ahead. NISten. 43:35 (Speaker B) There's also Chatbot UI on GitHub. So yeah, for the other people that are hacking something together, I'll wait until there is something public before, because then. 43:45 (Speaker D) We don't know everything. 43:47 (Speaker G) Open source is going to be worse. Because you are missing the model. 43:51 (Speaker A) Yeah, because we think that it's fine tuned on actually knowing how to run code. Right. That's kind of the highlight that we get with from the less space. We think it's smarter because of that. 44:01 (Speaker A) And one of the main things again, sorry, going back to code number just real quick, it is able to then fix itself and ask itself, oh, oops, I made a mistake. Let me try again. Matt, I saw you unmute yourself. 44:13 (Speaker A) Feel free to go ahead. 44:16 (Speaker D) Well, yeah, just a quick thing. So from what I know, openi will be offering fine tuning relatively soon. So at that point, you theoretically could go and fine tune your own code interpreter like Model, even if they don't offer it, which is going to you. 44:31 (Speaker A) Can also theoretically not that we would recommend, but theoretically right now you could start distilling some stuff from code interpreter by asking it questions. Generate code and store it to a file. Ask it to download and then quote, unquote, generate the data set. But not that you should, but you can theoretically as well, so that when it's time to fine tune, you have some data set. 44:52 (Speaker D) Yeah, theoretically. I don't know if a shared GBT currently supports those types of conversations, but if it does, I'm sure that's going to happen really soon. 45:00 (Speaker G) I don't think it's maintained because chat GPT itself well, I want to speak for share GBT. I know, Steven, but I can help you move the conversation back to cloud. 45:11 (Speaker A) Yes, please. Let's move back to cloud. Thank you. 45:14 (Speaker G) So just between the how many people are listening to this chat anyway? I think it's like 60 people. Email support@anthropic.com for the Cloud API. 45:26 (Speaker A) Yes, email them, state your use case and they'll likely get you in and you can use SWIX's menu bar to actually kind of run them in parallel with the megaprom feature. Megapron super prompt, what is it called? I think SWIX dropped. There is like one prompt that you type and then it all goes to both to all the models. I want to recognize some folks in the audience. 45:50 (Speaker A) Hey, feel free to regime if you. 45:52 (Speaker D) Want to come up. 45:52 (Speaker A) Obviously, I saw some other Euro I saw in the audience. Max AI. Welcome, Dexter. There's a bunch of folks who are usually here and it's great to see, and I think we're moving on to a very spicy one. 46:06 (Speaker A) What do you guys think about Xai? So I'm pasting the summary of the people. Elon Musk and a bunch of other folks have announced X. AI they're essentially answer to OpenAI. 46:22 (Speaker A) We've all seen Elon kind of talk about safety and talk about helping open Xai and then could not be open since then. He talked about truth GPT at some point. And finally they announced Xai as we were talking. 46:37 (Speaker A) By the way, I have an application from Xai which they're going to have spaces tomorrow to go deep into deeper into Xai. But so far there's not a lot of detail. There are some details about the folks who work there. 46:50 (Speaker A) So they have folks who wrote the Adam Optimizer. There are other folks thoughts about Xai before we get to hear what they do. Obviously, there's no product yet. 46:59 (Speaker A) I don't think they've started training. The one thing that I will say is that they will have premium access to Twitter, obviously, because Twitter is now rebranded.com Xai. After closing down the APIs and closing down the scraping for Twitter, xai will now have a data set that's insane to train on Twitter. 47:21 (Speaker A) And we wish them, quote, unquote, good luck. I would love to hear from folks on stage. What do you think about the announcement, the direction, the people? And we're going to wait for tomorrow to actually hear them talk. 47:24 (Speaker A) I know. NISten, you have some ideas if you want to share to get started. 47:40 (Speaker B) Well, this is more of an old lady babushko opinion that's just talking about stuff. I found it interesting that they went from, what was it? Base GPT through street taking on GPT four and this entire competition to doing something more noble like dedicating it to be better at math and discovering new things in physics. So the way I see that, that's pretty noble. But at the same time, I feel like that's a result of having problems hiring in order to be competitive with the other ones. 48:26 (Speaker B) So, yeah, this will be interesting. But the way I see the whole set up right now is, as the kids say, it's pretty mid, in my opinion. 48:39 (Speaker A) As the kids you don't use with that. I will say that we will see tomorrow from their space. They're probably going to use Elon's Cloud to maybe try to hire and it's probably harder now to hire because everybody knows how quick they're getting fired and how much. It's not like super fun to work for X, but we're in for a nice ride because they do have access to the cross pollination from Tesla as well, right? So if they have big questions, tesla does have a few good folks still, even after Andre Capati left, and so they'd be able to ask them for assistance. 49:20 (Speaker A) There's obviously the whole Dodgy thing in play, which we can I don't think we have time to talk about Dodgy, and it's not new, but there could be something there. Gabriel, you wanted to come up? Maybe you have. Yeah, go ahead. 49:33 (Speaker A) Gabriel. 49:34 (Speaker E) Yeah, I was just going to say about Xai, I mean, you mentioned Twitter's data, and I'd be interested in hearing other people on the stage opinion on this because recently there's been a lot of work done on quality of data over quantity of data. And of course, Elon also has a ton of GPUs. Reportedly, he's bought tens of thousands of GPUs. So that's definitely important in building these big models. 49:58 (Speaker E) But I'd be interested in hearing from people on the stage if they think Twitter's data and the kind of data that Twitter has is actually going to be really powerful for training good models. 50:11 (Speaker A) Anybody wants to take this? 50:13 (Speaker F) Yeah, I'll take a little of it. One of the things that Twitter has that other people don't is that people are actually debating issues. So I think that's one of the reasons why he's really focused on the idea of Twitter being a source of truth and being sort of unrestricted so that you're not just following like, one thread, you watch the narratives being debated and he has access to all that. 50:35 (Speaker A) Data and community notes. And it's really hard to scrape. Like, I don't think it's API ball at all. It's not super simple to scrape at all. 50:42 (Speaker A) I want to get yum before I think Matt wanted to unmute and go and then yum. If Matt, you still want to chime in and then yum. 50:53 (Speaker D) Yeah, I mean, nothing too much to add here. I think the community notes are very interesting as a way to sort of like, reduce hallucinations. I think one of the things that they're going to want to do heavily is invest in sort of filtering that data set because there's a lot of great stuff on Twitter. There's a lot of crap on Twitter. 51:07 (Speaker A) A lot of yeah. 51:09 (Speaker D) And the more of that that seeps in, the worse the model is going to perform. Obviously, scale is important, but data quality is incredibly, incredibly important and the scale kind of doesn't negate bad data quality. So I think if they do one thing right, it's going to have to be getting the sort of filtering of the data set down. But they do have a ton of incredibly high quality data. 51:27 (Speaker A) Yes, I think Yam was next and then we have a few folks wanted to come in. I think Pharrell wanted to come up. So yam. And then pharrell. 51:34 (Speaker A) And then Gabriel. 51:37 (Speaker C) I just want to say, of course, if you just take Twitter data and start training your model, you can expect it to be average Twitter, which is not what you want. What you can do, which is a gold mine, is to transform this data or just rephrase it as other forms. And this just makes the data a gold mine because Twitter does have very high quality content here and there. Absolutely. 52:05 (Speaker C) If you can, and transform it and rephrase it to a different form if you want an example. So the paper textbooks are all you need. Basically, they just take data and make it into a tutorial, make it into a textbook, like perfect, clean and everything. 52:22 (Speaker C) It is very easy to do, and you don't need a powerful model to do that. You don't need chachi PT. You can use it to do it with a small model. 52:30 (Speaker C) I'm currently doing off the record, I'm currently doing it myself in a large model I'm training. It doesn't it doesn't matter matter anyway. It's a gold mine. 52:43 (Speaker C) What I'm saying, it's a gold mine. 52:45 (Speaker D) About Twitter. 52:46 (Speaker A) An additional thing before I get to Farrell and then gabriel additional thing. NISten I talked about yesterday at length in our late night line cook space. That's not going to be scheduled. If you guys are on, feel free to join that one. 53:00 (Speaker A) Twitter Spaces is also a gold mine. Transcribing Twitter spaces and seeing all the reaction emojis that they have in real time. Like the space that Elon ran with RFK Jr. For example, if you know in the audience who are actual people instead of bots, and you're able to get like emoji reactions in real time, that's a definite, definite, very high signal kind of training set that they have and almost nobody else has. 53:25 (Speaker A) And through how to get Pharrell, you are next, I think. And then gabriel yeah, I wonder what. 53:30 (Speaker D) The relation is and how useful the Twitter data will be for their goal of building a sort of math reasoning machine. Right. Also, do we know if they're open source, as in truly open source or not? 53:49 (Speaker A) No, we don't know yet. Hopefully tomorrow we'll be able to answer questions. However, we've seen Elon take Twitter's algorithm to open source, and now he's like, boasting this comparatively competitive advantage versus something like Threads. He's saying, like, hey, open source. 54:07 (Speaker A) If you go to Threads, you're under the Zucks influence algorithm. So there is definitely an attempt to open source from their side, but we don't know anything about that beyond that. Gabriel. 54:17 (Speaker A) And then Johnny. 54:20 (Speaker C) Yeah. 54:22 (Speaker E) First of all, I think it's funny that Elon's s**t posting is polluting his data set. I would say that. 54:34 (Speaker A) By the way, if there's anybody with the option to detect S**t posting, it's them, right? They're going to be able to build a model. Understand, this is s**t post. This is like somebody who made an effort to give us clean information. But sorry, go ahead. 54:49 (Speaker E) Yeah, that's exactly my point that I was going to make, that Elon was on this crusade before he bought Twitter. And this is kind of why he got forced into buying Twitter, because he was going after the bots and he made a big deal about the bots. And I think they spent a lot of resources on figuring out what's good content and what's bought content. And another thing is that we each are kind of experiencing a different Twitter, right? Because we're within whether it's an ML Twitter or Israel based Twitter, and there's many different communities and their Twitter is very good at segmenting those communities and figuring out which content belongs to what community. 54:55 (Speaker E) And they'll have the ability, I think, to segment this data and train many different models that are good at different things because they're in a literature community or in an ML community or MMA community or whatever. 55:37 (Speaker A) I actually saw a map of like 5 million, 7 million tweets all embedded in Nomic Xai Atlas. I don't know if you guys follow Nomic, they just recently announced like a 17 million round A, by the way. So kudos to Nomic good friends. Andre, the GPT for all team, and they have like an embedded map before the API was shut down that they were able to siphon, et cetera. 56:00 (Speaker A) And Gabriel, what you're saying is actually visible in the embedding map. You can actually see those tweets and then different areas of the political Twitter. There was a journalist Twitter until all of the journalists started living there's like a bunch of different pockets of Twitter that we don't get exposed to, not to mention the different languages. 56:20 (Speaker A) There's a whole Japanese Twitter that's like insane. And people go super, super hard. And translating is easy. 56:26 (Speaker A) We talked about Cloud being able to translate. So they have a bunch of very interesting data. And I think Zuck is also going after that data with Threads. 56:31 (Speaker A) And I think this is the reason why we'll see Threads getting continued work and we'll see a lot of investment from their side. But to compare to Threads, and we talked about this yesterday, is that Twitter has back history and a lot of historical data that they can train others. Threads is fairly new as well. 56:54 (Speaker A) So definitely a bunch of interesting data sets. Johnny and then Lentil. Hey. 57:00 (Speaker H) So one I think about when I think about the data from Twitter that is potentially lacking and some of the other data sets is colloquial language. Because what Twitter has that Facebook doesn't have and a lot of other things don't have, especially from what you're talking about, like historic, is the way that people actually interact with each other. You know what I mean? 57:26 (Speaker A) Not only that, how it evolved as well, right throughout exactly. 57:35 (Speaker H) To be honest, I think the data sets from earlier is probably better and stronger because it's just gotten out of hand. But I agree with what I'm not sure it was Yam or who said the filtering because all right, this is black box, it's not open source. Elon has not been shy about his kind of response to what he perceives as wokism and all of that stuff. I'll be super curious. 57:36 (Speaker H) I mean, there's a big team on this, but I will be super curious to see what that bears out in the actual model. Because, God, there's equal parts or more parts disinformation on Twitter than there is information. So if we're talking about source of truth, that rings some alarm bells for me, for me personally. 58:21 (Speaker H) So those are just my thoughts. 58:29 (Speaker A) Yeah. Thanks, johnny Lentil. Go ahead. And then Gabriel. 58:33 (Speaker A) Let's finish on the Gabriel and then we'll move on to the next topic. 58:36 (Speaker H) Cool. 58:37 (Speaker A) Yes. 58:37 (Speaker H) So I think it's going to be hugely bullish for this data. And from the perspective of relating idea space and people and the relations between those, I think that's probably going to be more of a goat information than conversation because you can build so much from that. Like dating this is just one like a dating thing. Or finding people, finding brain power compute, that's going to be huge. 58:40 (Speaker H) And to touch on the open sourceness of the data, I think not open sourcing it at some point is going to be hugely politically bad for Elon to do. 59:23 (Speaker A) That'S. 59:23 (Speaker H) My thoughts on that. 59:24 (Speaker A) Awesome. Thanks, Lance. Gabriel, let's end up and then, Matt, we're going to talk about some interesting stuff. 59:31 (Speaker E) Yeah, just on the kind of data. I think for those of us who ran, like, the early versions of Llama before they got fine tuned in all kinds of ways, and you run it, and especially the smaller models, you put in a prompt and it spits out some generic Facebook type of content. It sounds like a Facebook post of like a 15 year old or something like that. That shows what you get when you use all this kind of unfiltered data. 59:59 (Speaker E) But I think the interesting thing is that Llama was then fine tuned in many different ways and some really powerful models are built on top of it. So I think in some sense, almost any data is valuable in the sort of pretraining stages and maybe you need really high quality for the fine tuning, but I think that big volume might be really useful, maybe not the most economical. 60:21 (Speaker A) So I want to wrap up things why they potentially have like a leg up versus not a leg up. We definitely know that Twitter was used to train other models that we currently use. We know this for a fact. This was the reason why Elon and Sam Hoffman, who used to be friends, are no longer friends, sheet posting about them. 60:40 (Speaker A) And the current models we use. Do use this data set, but it's old for them. It's no longer like recent and relevant. 60:40 (Speaker A) And we know for a fact that Twitter is significantly biased and probably the best place in the world for uncovering news as they happen before the bias sets in, before the narrative sets in, before folks know how to before folks get their marching orders from MSNBC, from the Other Side, how to think about things when not. The Twitter is really good at talking about issues as they arise, the second they arise. And I think that on its own is going to teach the models a very great deal. 61:16 (Speaker A) Naval Ravican, if you guys follow Namal, he always said Twitter makes him a better writer. So we definitely know also that tweet in short form condense information better. And if their model trains on that, obviously taking all the precautions we talked about before, bots and s**t, posting, et cetera, if they're able to actually get this into the model, likely their model will be more up to date and more fine tuned like reaction. 61:20 (Speaker A) So with that, I want to close. We'll see about Xai. It's definitely exciting, right? We're potentially getting another big one, potentially open source one. 61:20 (Speaker A) So we'll see. I'm going to wrap up this update and I think the next one I want to move on. Matt, let me know if you're still around if you want to cover. 61:20 (Speaker A) So we have Matt, who introduced himself in the beginning. So I'll let you do this quickly again because maybe and then we're going to talk about the stuff that GitHub Stars is rising on, which I think is super cool. And I invite you to give us a little bit of an interview about this. 62:16 (Speaker A) Go ahead, Matt. 62:17 (Speaker D) Yeah, sure. So I'll try to summarize it a bit better than the last time. A lot of practice, but very long story short, co founder, CEO of Other Side AI, creator of Hyperwrite, and a number of other things. Basically, we've been around for a number of years now. 62:30 (Speaker D) We're one of the first companies in the space working with LLMs. The goal always has been to build a personal assistant that scales to everybody, just like a real human personal assistant, but at scale, way cheaper, digital. The tech wasn't there at the beginning. So we built other products to sort of learn and gather resources, whether that's users, revenue, bunch of other things that we can do. 62:50 (Speaker D) What we do today. Today we are actually building that personal assistant. So an AI that can operate a computer, any software to do what a human can do on pretty much anything. 62:53 (Speaker D) So it'll help you with your tasks. It's very simple. Today it's a Chrome extension that lets you sort of like control Chrome just by sort of talking to it. 62:53 (Speaker D) So you could say, go order me a pizza, or go send this person an email or go filter my email, or anything else it works okay today. The idea is that over time, it's going to get a lot better, a lot cheaper, a lot faster, to the point where six months from now, a year from now, it might actually be as good as, if not better than a human on many tasks. But that being said, while I work on this, I also like to learn about getting the most out of these technologies because they're so fast moving and you really have to stay on top of it to be effective, or you. 63:34 (Speaker A) Can every week and then stay up to date with us together. But yeah, go ahead. 63:40 (Speaker D) Exactly. I mean, a lot of what I do to learn really, is just build things that I find interesting, and I find that often, even if I'm not expecting it, a lot of those learnings do translate to stuff we're doing at other sides. So this sort of just came out of that. Happy to sort of dive into the project, or if you want to sort. 63:56 (Speaker A) Of stop me and let's pause here for a second and I'll just tell folks that I pinned Matt's Tweet from a couple of days ago with the introduction. Since then you got a few thousand stars, I think, on GitHub, and we're going to talk about the GPT Prompt Engineer project and the different reasons why Matt and folks kind of written this and what it's here to serve. So maybe give us an introduction to the GPD Prompt Engineer and what kind of made you come up with this and how it works. Yeah, go deep, man. 64:29 (Speaker A) Sure. Yeah. 64:30 (Speaker D) So forget about rambling in advance. Essentially, I find prompt engineering so fun. I've been doing it pretty much every day for everything, honestly, to the point of excess, from what I would do for work to having it decide what I'm making for dinner for years now. And as I've gone through this process, sort of like learning how to use these models, it's become very clear that especially as these models evolve, there's no best practice for anything. 64:54 (Speaker D) Prompts change ways to prompt change. Something that works for one task might not work for a very similar task. And the only way sort of get out of that is to sort of get an intuition of the model and try a lot of things, but that doesn't always work perfectly. 65:01 (Speaker D) And also you don't really know kind of what works and what doesn't. Even when you're trying things right, you have to do it sort of like in a very scientific way, but there's no real right answer to anything. It's kind of like alchemy. 65:18 (Speaker D) So starting to think I think this was right. When GPD Four came out, I was using GPD Four pretty often to just ideate prompts. I would say, here's what I'm trying to do. 65:20 (Speaker D) I would say, write a prompt me, and I would use the ideas from that to help me improve my own prompts and that actually got a lot of interest. We ended up building a sort of thing similar to that into the hyperwrite platform. At the time it was really cool, but really wasn't something that would replace what I do every day, which is really hardcore prompting. 65:43 (Speaker D) Eventually I was just sort of thinking about it, and I think this was on the 4 July, I was just sitting there kind of thinking, what if we tried it? And I started thinking about how could you design a system that actually comes up with good prompts? Not just a prompt that does the job, but something that's actually optimal, because as humans, right, we can only try so many things at once. But the magic of these LLMs is they're creative and they think faster than we do. In the time that I could write half a prompt, LLMs could write 5100. 65:48 (Speaker D) And what if you could leverage that? Because even if the average prompt isn't very good, you're going to luck into one or two that happen to be exceptional for your task. So I started by doing it actually with a classifier. I only released this notebook yesterday just because it's like a step on the road. 65:48 (Speaker D) And what we ended up using it for was actually something at other side where we needed to build a classifier for something with personal assistant. And I just wasn't getting good performance out of the prompts that I was writing. So I said f**k it, what if we have the AI try to do this? And I built this so that essentially I describe the task, I give it some test cases, so I'll give it some true false test cases. 66:11 (Speaker D) Because the classifier was classifying things as true or false. It was like classified the statement as true or false. And it was like New York is in America, it would be true. 66:54 (Speaker D) If it was new York is in Paris it would be false. And I basically created like ten or 20 of these test cases. I described the task and I had GPT generate something like, I think 20 or so prompts. 66:57 (Speaker D) And surprisingly, the quality of them just at first glance was pretty good, right? It was kind of shocking considering I spent so much time trying to do this manually. Then what I did was I just basically had each of these prompts test against each of these test cases. And I plotted sort of the success of each and turns out some of them actually outperformed what I did. 66:57 (Speaker D) I was kind of shocked, right? Like you wouldn't expect that, especially doing this for years. 67:30 (Speaker A) Just to recap real quick on this, the GPT four, I assume that's what you're using generated prompts actually performed better than Match rumors. Prompts and Matchroomr is the founder of a prompt company with a lot of prompt use cases for a long time, from GPT-3 to four, et cetera. And some of the ones that it came up with performed better than yours. 67:52 (Speaker D) Yeah, it was kind of scary. Some of them performed way worse. But the idea is that you're going to sort of luck into something that is better. Maybe two out of 20 will be better, but they're great. 68:02 (Speaker D) So I was sort of just so fascinated by this, I was like, how do you take this further? Because classification is one thing, but real prompts where you're actually having it generate text, those are harder. How do you judge that? You could use GPD four to judge them, right? If you have two prompts and you say each of them generate me something and they give you your responses and you want to know which is better, you can ask GPD four. And so I figured we could apply that. 68:29 (Speaker D) Turns out there's some issues with that and there are some papers written about this where essentially it'll be sort of like more favoring the one that's on the bottom. So just do it twice, flip the order and see if one wins. And I took that approach and I sort of combined it with sort of like an ELO style tournament where essentially you have each of them go head to head, like one on one, and each of them gets their ELO score either bumped up or down based on whether they win, lose or draw. 68:53 (Speaker A) Can you give two sentences on ELO scores as a concept? Yeah. 68:57 (Speaker D) I'm actually not super familiar with it. Funny enough, I had GPC write the code for that part, but basically think of it like a ranking system in a video game. Yeah, think of it like a ranking system in chess or a video game where you have two people competing and the one that wins gets their score increased by x. The one that loses gets their score decreased by x. 69:18 (Speaker D) And it also sort of like weighted based on the previous scores. So if somebody that has a high score beats somebody with a very low score, their score won't increase that much because they're very likely going to win. So it's sort of just like a weighting system to help figure out what's the best so instead of just sort of getting a clear cut, yes, this is right, or no, this isn't what you can do with classifiers, because there is a right and a wrong ground truth answer. 69:39 (Speaker D) I just had each prompt sort of generate for a test case and the sort of opposite prompt the competition prompt would generate for that test case. So I was a little bit complex and they would have the model judge which one was better. And it's expensive, right? It might cost like $20 in GPT calls to get to an answer, but turns out at the end, the prompts again were just kind of blowing me away. 70:04 (Speaker D) Awesome creativity in them. Like the words it used, the trigger words, it didn't do what I would do. And in a really good way. 70:10 (Speaker D) And it also opened up my eyes to sort of like new ways of prompting that I never would have thought of and just sort of like aren't standard. And that's kind of the magic of all this. I think that this sort of abstracts away the sort of atomic level of prompts, right? You talk about prompts as sort of a prompt in and of itself and then a system built around the prompts with many prompts kind of working together. 70:31 (Speaker D) This makes it so that you don't have to guess about, do I have the best prompts for this single atomic part of our system? Where the magic really comes in then, is how do you string these amazing individually crafted by AI prompts together to make something that actually works really well. 70:46 (Speaker A) And how you robustly build the evaluation system, right? Because the classifier is a simple example of evaluating, because maybe you know this, et cetera, but how do you actually scale up the evaluation system such that this could potentially run in loops and then generate the best of the best prompts for a task? 71:03 (Speaker D) Exactly. 71:03 (Speaker A) That's also like a very interesting piece. How do you think about evaluation going forward? 71:08 (Speaker D) Yeah, so I think it's sort of like that, where you could have this thing run in the loop three times and take the three winners and then have GPT read those winners right, and be like, here are prompts that worked really, really well. Here are the test cases where they failed. Now I want you to write new prompts that take what's good about these but also mitigate the failure cases and generate a whole new set of prompts. Sort of like evolution really doesn't just have to stop at one point in time after the first run. 71:37 (Speaker D) It's like, let's learn from what these amazing ones still did wrong and continue to make this better and better and better. Obviously, this relies on a relatively large test set. I'm also experimenting with ways where you can have the test set autogenerate, but that's a little bit finicky. 71:50 (Speaker D) But I do think that sort of like evolution of this could lead to some really exceptional prompts. But what I found was even on the first run I was seeing it outperform myself. For example, there was a classifier we were using GPT four with logic bias to do because it was such a hard challenge and we were getting some like 90% accuracy. 71:50 (Speaker D) I had it do these prompts with GPT four, but then I had it run them using GPT 3.5 and it got 96%. 72:19 (Speaker A) We've talked about this pattern before where you can outsource kind of the hard work to GPD four, but then once you get really good at prompting, GPD 3.5 is actually very decent in many things and it's way faster, cheaper, and has a 16K context now that you can use. And so we've seen this pattern with many folks that if you don't need the full power of the GPT four, human evil for coding, et cetera. You can go far into GPT 3. 5 and get very far along, especially as you're getting better prompts. And now, Matt, you have like a recursive crafter helper guy that's here. And my next question for you is, have you used anything else? So you mentioned GPD 3. 5 where you run the prompts. Have you tried them on different models, like Cloud maybe, or the open source llama ones? 73:07 (Speaker D) I actually haven't just because I wanted to see if this worked. It was sort of just an interesting thing for me and my time is really focused on other side and personal assistant, but it wouldn't be hard to get Claude in. I suspect Claude prompts would perform better on Claude. Open ad prompts would perform better on Open xai just because the models give the prompt them very differently. 73:18 (Speaker D) Claude is sort of like a more emotional thinker. Open xai is more of like a logical thinker. It's a very sort of simple, not perfect analogy, but I suspect you'd want to sort of like stick within the. 73:36 (Speaker A) Ecosystems, maybe, not to mention inflections pie, which is like a whole different beast. 73:41 (Speaker D) Yeah, that's an interesting one. 73:44 (Speaker A) We discussed by a couple of times and I've seen some reactions, but I don't think maybe at the end of this, if we have time, matt, one question I will have for you on this and I think we'll move on. Is that where folks can find more work of this? Is it open source? What are you looking for contributions? If you are. And yeah, just give us a wrap up of this project. 74:07 (Speaker D) Yeah, so you can find it on GitHub. It's called GPT prompt engineer Currently there are two notebooks. It's all done in Jupiter notebook format, so it's pretty easy to edit. One is for the classification system, the other is for the generation system. 74:20 (Speaker D) We're honestly sort of like at a point where it works well, so it's like, what do you build around it? One thing that's missing is the classification version only supports true and false labels, but it's not hard to use TikTok into or TikTok and whatever it is to allow it to support arbitrary labels like happy, sad, angry, whatever. That's probably like a 20 minutes ad that if somebody goes in and does that opens up a whole new set of use cases. The evolution idea that I mentioned before, right? Taking the best prompts and then saying, here's where it went wrong on these test cases, and then throwing it back to GPT and having it generate more and rerunning it, that's interesting. 74:45 (Speaker D) The ability to use Claude would be awesome if anybody wants to add that. I could even see it evaluating each prompt on each model, right? Because right now we only generate with GPD four. We only evaluate with GPT 3. 75:19 (Speaker D) 5. But imagine if you generate with GPD four half of them, you generate half of them with Claude and then you evaluate each prompt on GPT four, GPT 3.5 and Claude. 75:27 (Speaker D) And you can see sort of the latency success rates for each along with scores. I think all that would be super interesting. Also sort of like just open to ideas. 75:40 (Speaker D) I'm not really sort of supporting this at all. So if anybody wants to kind of take it and run with it, I am all for that. Also sort of just like a shameless plug right now or thing that we're looking for just because I have an audience here. We are at other side in hyperwrite, really looking for somebody to help on back end hopefully with a security set of expertise. And then also if anybody is experienced in training machine learning models, I would love some help there because we're doing a lot of LLM training. 75:55 (Speaker A) So just quick thing and also to add that now with the Prompt Engineer that's automated, the results of this would likely generate like a great data set that you can add and continue fine tuning, especially as GPT four fine tuning is coming soon. So Matt, definitely store everything you generate with the yellow score and everything and from a GPT prompt engineer that runs and doesn't know about the rest run, maybe there's going to be a path forward to actually fine tuning a prompting model, which could be exactly. Well, yeah, exactly. 76:28 (Speaker D) Imagine taking a prompt and taking one that has a slightly higher score and fine tuning a model to take the initial prompt and then sort of output the one that has a higher score and you can do that evolutionarily continue to get better prompts in theory. 76:40 (Speaker A) Awesome. So folks, if you want to work in a cool place, I can write, hit met up and also check out GPD Prompt Engineer on GitHub. Thanks for coming. Feel free to stay and kind of continue commenting and talking with us as we go through a bunch of other updates that we have. 76:57 (Speaker A) Just a quick check with NISten who promised me to follow Twitter and see if anything new comes up. Breaking news as we talk. I haven't seen anything besides the space of Xai. 77:04 (Speaker A) I will ask people's attention to the last pin tweet from Dr. Jim Fan that talks about the context length dip. Matt, you also touched on this context length dip. It's basically a paper, I think. 77:22 (Speaker A) Stanford I'm not sure that figured out. That even longer. Context windows, they have a dip in the middle, which means that at the beginning of the prompt at the end of the prompt, the model has more attention to what you actually asked it to or the details that you provide in the middle there's like a dip. 77:39 (Speaker A) And this was also released this week. However, the one thing I said previously I will repeat here claude and some folks who know about contact windows way more than me. They say the Claude is actually really good at this without the dip. 77:54 (Speaker D) Yeah, I feel like that's saying. It's an interesting paper. I feel like it's sort of saying like, hey, if you train on marketing copy, then it's going to be worse at coding, obviously. Right. 78:03 (Speaker D) We do a lot of long context stuff at other side. That's actually what I'm focused on right now, training really long context massive models. And if you train it on data where there's context in the middle that matters, it is going to be good at that. 78:16 (Speaker A) Interesting. So what you're saying, I think I've seen this kind of opinion before as well. It's just the outcome of the data that was fed in and for blog posts and other places, people want to hook your attention in the beginning and then kind of finish strong. Basically you're saying that this is potentially an outcome of that and not necessarily the tech behind it. 78:38 (Speaker D) Yeah, I believe so. I mean, who knows, maybe wrong, but from my experience, right, why I was given that analogy before is like if you train it up to do one thing and then you're asking it to do another, it's not going to do that other thing as well. And I'm guessing the data set that they sort of did this evaluation on was something that didn't have a ton of information at all. Part of the reason that so few of the language model companies have super long context length models and why it was such a big deal that Anthropic did is because a lot of the challenge in training them isn't actually in training them, it's in the data. 79:08 (Speaker D) Obviously, inference becomes a challenge. It's the cost and the overhead there. But the data to sort of do this is really sparse. 79:10 (Speaker D) It's not very available. Right. So that's I think part of it right there's not just like a sort of standard data set that has super long context link, that has information in the middle. 79:25 (Speaker D) We do actually we've been building one another side and that's sort of given me some of the ideas that I'm sort of spouting here. But my guess is that Anthropic part of the reason theirs works is because they focused on the data. The data is really important. 79:38 (Speaker A) Right. 79:39 (Speaker D) I will say model, it's just fine tuning. 79:41 (Speaker A) Yeah. I will say when I got access to Clouds Window, I did like a bunch of tests with my Twitter data. I just pasted like a bunch of JSON with Twitter numbers, twitter IDs numbers. And the smaller model, the not 100K, gave me back results that actually didn't invent those numbers. 79:57 (Speaker A) The 100K model lost in the middle and started inventing those numbers. I literally saw this difference between the longer complex one and the previous one and I thought it's because of like it loses some complex in the middle. And I need to retry this on the new ones because the new ones, they claim this doesn't happen with that. 80:01 (Speaker A) I want to go to Al and yeah, one of you I think raise your hand first to talk about the context length dip and that paper if you have read this, if you have thoughts and if you have noticed this as well. 80:29 (Speaker F) I just had a quick question for Matt about the differences that he found in prompting between say, Claude and GPT Four. I noticed like, the prompts aren't really reusable and maybe you could speak to that in the general case. 80:42 (Speaker A) Yeah, let's end with maybe this question and move on to other updates as we have. Go ahead, Matt. 80:48 (Speaker D) Yeah, sure. So it's like talking to two people with two different personalities, right? They're both people, but they respond differently to different ways. You're sort of prompting them, if you will. Claude is sort of like more emotional, I guess, where open xai is sort of more logical. 81:03 (Speaker D) And it's hard to sort of pin that down to any one thing, and it's hard to give you sort of like techniques based on that because, again, every use case is very different, but it's very clearly it's a prompt them differently. I think also talking about the idea of fine tuning a prompting model will be very interesting is fine tuning a model that takes an Open Xai prompt and converts it to the idealized version of a Claude prompt and vice versa. I mean, I think that could be very powerful because there are ways to sort of intuit your way there. 81:29 (Speaker D) It's just hard to sort of distill into a set of rules. One thing I found actually quite interestingly with Quad two is that it is insanely resistant to sort of like jailbreak attacks. So I was able to get it to do it. 81:44 (Speaker D) Turns out the stupidest method worked. It was sort of like modifying that dan prop that's been going around like reddit but the more nuanced sort of like complex methods that typically work with OpenAI they didn't. So I think the model is just qualitatively different. 81:56 (Speaker D) I think it's going to take some time to fully explore it and understand why and how still super early days. 82:06 (Speaker A) I love the fact that all of us are getting an intuition about different models and how to approach them right. And that's like Sweet was here before. This is like a specialization of what I think he talked about as an AI engineer. We're getting to start to understand the differences between those to the little fine little things that you can say. 82:11 (Speaker A) And I think it will be very interesting if you have a model that's trained to actually convert them or translate them between the models to work the same. I have an idea where not to get locked into the GPD Four ecosystem with the functions. I have an idea of wrapping the GPD Four API package with something. 82:47 (Speaker A) They will actually kind of print the functions into the context because cloud now has a huge context window. And then try to see whether or not cloud is able to kind of without additional tech, without additional changes to the API to replicate the outputs of how a GPT with functions would do. And that's going to be an idea I'll be testing, hopefully, and talk about next week. 83:08 (Speaker A) Thanks, Matt. 83:10 (Speaker C) Today, there has been a thing today, maybe yesterday, but anyway, today there have been a model that generates prompts. By the way, by giving the data, you generate the prompt. I've written about it today on Twitter. It is so powerful, it is such a cool method that you can take whatever you have, like, I don't know, scientific papers and generate instructions for them. 83:32 (Speaker C) Now you can fine tune a model that generate scientific papers. You got jokes. Now you can train a model that become funny. 83:35 (Speaker C) You can generate the instruction, convert whatever you want into instructions. Amazing it is today. One more thing about the deep in the middle thing. 83:51 (Speaker C) I don't know why it happens. I have no idea how Open Xai trained their models. But I think if you think about it, many missions, many instructions, paragraph, and before the paragraph, you tell the model, please summarize the following, or on the contrary, like a paragraph and at the end, what was that? Something. 84:10 (Speaker C) So it makes a lot of sense that a model pays a lot of attention to the beginning at the end, because of this. And on the same note, it's very easy to fix. So I wouldn't just point fingers. 84:21 (Speaker C) It's good that they pointed it, but I think it's like, I don't know, a couple of minutes of training, open AI, like, fine tune for a minute and fix it. 84:28 (Speaker A) I just want to ask yum, yum. The the pin that I just tweet sorry, the Tweet that I just pinned on top, this was the one that you talked about, the instructions generation and the problem generation. 84:38 (Speaker C) Yeah. 84:39 (Speaker A) Awesome. So folks, definitely feel free to check this out. I haven't seen this. You want to give a couple more words about that one. 84:44 (Speaker A) It looks like you wrote, like, a very deep dive. What's the model like eleven B, three B? 84:54 (Speaker C) Sure. Two models put into the models, whatever you want. Okay, let's go back. You got a data set of something, emails from your company, for example, and you want a model that will help you write emails. 85:01 (Speaker C) Okay, you can start thinking about how to train this model, or you can use this and now generate a text that basically says, help me write the following email to this following person of something something and the actual email. And all of a sudden, you have a model that is extremely you have a data set to train a model or to fuselage or whatever that is extremely tuned to this. So I think it's a very cool technique. 85:40 (Speaker C) It's very powerful, has a lot of potential. And the trick, in simple words, is training the model. What not to say? That's the missing piece here, that they added the trick. 85:51 (Speaker C) They took instructions and outputs that do not fit just a different random output from the data and train with a different laws. That the model should not say this because this input does not with that instruction, does not result in this output. That's it. 86:11 (Speaker C) That's the trick. And it works perfectly and really cool. 86:17 (Speaker A) Awesome. I have some folks who want to come up and ask questions. I think we're almost there in terms of the updates. I will just briefly run to some updates. 86:18 (Speaker A) I don't even have time to go and look for the threads, but if you're not following Rama CPP, follow gerga is one of the groups that we have in the States. I think he single handedly is in charge of so many folks trying to get a MacBook, because it's incredible how much performance they've been able to squeeze out of Llama. And it's comparatives. 86:49 (Speaker A) And many people just, like, quantize their models, basically make them smaller to run on this GGML platform that they have. The recent news that I have from over there, there's like two pieces of news. Last week, for those of us who were here last week, we talked about CFG. 86:58 (Speaker A) I forgot something. I forgot the guidance scale. And we talked about the CFG parameter moving from diffusion models that we know. 87:17 (Speaker A) Like, in stable diffusion, you can define how close to your prompt should the model generate the image. Somebody decided, I think, an illusion reaction. Somebody said, hey, can we have this control of CFG to our LLM generation? CFG is a classifying guidance scale, something like that. 87:37 (Speaker A) And they did it. The Chad GGR added this to Llama CPP. And so now you can actually kind of pass a CFG control and fine tune. 87:48 (Speaker A) It's almost like a running fine tune to an extent. You can test the model to be closer, farther away from the problem that you have. Contrasting this with the stuff that we have on a GPD, four API, which is temperature. 88:01 (Speaker A) And I think, Matt, you mentioned something to logic bias, logged bias, something like that, right? Where you can ask it not to say certain things. So contrasting CFG, it's like a different beast that we now have a different control. And so GGML just merged into their platform. 88:18 (Speaker A) Definitely worth checking out. And the second thing is, I need to find the Tweet. Yesterday, Georgia was like, oh, yeah, by the way, here's the 48% inference speed improval that somebody just merged in. 88:30 (Speaker A) Have you guys play and try this. For the 33 billion parameter model of Llama, somebody just merged in a 50% increase on inference speed just on the way. And I find this incredible because Gmail already runs many stuff on Raspberry Pi or whatever, iPhones, and now somebody's like, oh, yeah, here's a 50% increase in infinite speed. 88:41 (Speaker A) And then I think Nissan was here before he was talking about GGML runs on the iPhone, because iPhones, even from three years ago, have the same neuron chip that like the latest Max or some such, and that this performance boost on GGML also applies to iPhones as well. So, incredible stuff. And as we hear every week, we keep seeing leaps, incredible leaps in speed and performance. 89:15 (Speaker A) Definitely worth checking out GGML and the five folks that work on those stuff. GML comments, folks who use Llama, CCP, feel free to hop up and raise your hand and give us more updates from that length. I denied it. 89:28 (Speaker A) You are gay at the spaces, but sometimes as a guest as well. Other than that, I think we'll move on to some more updates and then we just have questions. No? Cool. 89:41 (Speaker A) So the next update that I have is from the diffusion side that we sometimes cover. We don't cover it often, but we do cover it from sometimes time to time. So two things from stability stable diffusion. 89:46 (Speaker A) We talked about Sdxl, the new Excel model that can generate 1024 images. We've talked about last week about the 0.9 weights dropping. 90:01 (Speaker A) Sdxl 1.0 is now available in the Stable Diffusion discord. If you've played with Me Journey before and you looked at Stable Diffusion, it's like, it's not that great. 90:05 (Speaker A) Stable diffusion sdxl one is really impressive. And besides being really impressive, they plan to release this open source. So we're going to see a bunch of folks fine tune loras and specific versions of the specific things. 90:16 (Speaker A) And I think it's like, incredible. If you want to play with those models and you haven't yet, go to Stable Diffusion discord and hit up that bot and then Netflix let us know how incredibly different that is. And we're waiting for the wait for the Sdxl 1. 90:47 (Speaker A) 0 to drop. And I will mention this every day until the year mark. It's been less than a year since table Diffusion. 90:57 (Speaker A) It's been less than a year. I remember I think it was August 22 when they actually dropped the full open source model. Less than a year. 91:12 (Speaker A) And we've seen just such incredible progress. So, like Matt said before, it's really hard to keep up, but it's also really hard to internalize how far, just how far we're coming with those incredible leaps and changes every week. And again, to just plug in this Thursday I space. 91:21 (Speaker A) This is why we're here. Every thursdai talking about everything and everything that's changed and updated. And the other thing that I want to I see art in the audience with apart. 91:28 (Speaker A) If you play the list, the Excel, feel free to raise your hand to come up. The other thing that they released, I don't know if you guys familiar with Clip Drop. So Stable Diffusion bought Clip Drop as a company and started implementing that interface compared to their Dream Studio interface. 91:49 (Speaker A) So ClipDrop is like a way simpler interface day to day release, something called Stable Doodle. Stable Doodle is I don't know if folks in the audience remember this. Meme how to draw an owl. 91:51 (Speaker A) Step one, draw a circle. Step two, draw some eyes. And step three is like, draw the rest of the f*****g owl. 92:06 (Speaker A) And then you have, like, a beautiful owl painting at the end of this. This is now the go to test on how the Doodle models work. And I pinned my attempt at this, but definitely check out ClipDrop Doodle thing. It's really fun to play with. So those are, like, the updates from the diffusion world. 92:10 (Speaker D) Hey, real quick. I was just looking at the repository for Comfy UI, and then I saw that I don't know how to say his name. Scousekip is in here. So I just wanted to come on and say, like, hey, this is incredible. 92:24 (Speaker D) This is what we've been talking about for months now, right? This node based character codex, if you will, of like there's just infinite possibilities. I just want to listen, but thanks. 92:35 (Speaker A) For bringing me up. 92:36 (Speaker D) This is really cool, man. I was just thanks for bringing up Comfy UI. 92:42 (Speaker A) I feel guilt at not being up to date on every single possible thing. I know it's impossible. I really try, and Comfy I has been on my list to try, but then Quad was released and Code Interpreter was released. Comfy I seems like the thing we want, man. 92:42 (Speaker A) I think stabilization when they tried to bring up Dream Studio, they talked about, like, a node based thing where you can pipe models to other models, you can find filters, et cetera. Comfy UI for folks who have tested it out, it looks like that's it. And I definitely want to agree with Art. 93:16 (Speaker A) It's something to watch out and maybe try because automatic one on one, even though it's, like, super advanced and has been there for a beginning since Stable Diffusion, it's just like a s**t show of a UX. Just like horrible, horrible. I'm sorry, guys. 93:30 (Speaker A) I've built a web UI before automatic. It's really hard to get Gradio to play as much as you want. It's really hard to maintain a good UX product with many, many people contributing, with many, many things are changing under your feet. 93:45 (Speaker A) So it's really not their fault, but it's a s**t show to get started with. And Comfy UI seems like a fresh, clean start. So definitely if you're playing with this, test this out and let us know. 93:55 (Speaker A) Max, you have your hand raised and you play with the Excel. Give us some of your thoughts. 94:01 (Speaker I) Yeah, I have played through the website in a studio, so I'm lately working with a company that make toys for kids. They want to start incorporating AI. And one of my concerns we're working with them is like, okay, we want to generate images for kids. Something that is going to probably freak them out is two things that diffusion models have been lacking. 94:27 (Speaker I) One is the ability of painting things like complicated shapes or intricate shapes like hands. SD. Excel is not better at it. 94:40 (Speaker I) Another one is this concept of what is named like concept bleeding, which is this diffusion model tends to mix objects that are similar in shape or form is not good at it, neither. Now, I was reading the paper from Stability or the report. They claim they are outperforming Mid Journey in five of seven categories now, mid Journey 5. 1, right? 95:12 (Speaker A) Just to make sure. Mid Journey since then released the new version also because we're in same pace, but yeah, they've compared to Mid Journey 5.1. Yeah. 95:20 (Speaker I) Well, now this is a report internal released by Stability. It's a paper, it might have some credibility, I don't know. I like the results. It's very close to me journey, but I think there is still one or two steps behind, in my opinion. 95:36 (Speaker I) What is different is what you have mentioned, Alex. Once they release the weight and we can see Lotus about this, I'm expecting to see the results that we can get because probably that is what is going to position this model like a step above Mid Journey, but not yet. This is my opinion. 95:58 (Speaker A) Yeah, definitely. And thanks for that. And I love folks coming up and sharing their opinion about these things. I will say on the top. 96:05 (Speaker A) Thanks Mike. Or I guess I know you're a new name, but I'm not sure if I can if I should. 96:10 (Speaker I) Yeah, totally, totally have it, in my view. I'm Juan Spanish, living in Mexico and I like these things. 96:17 (Speaker A) We appreciate you coming up here on the topic of UIs that we've mentioned with somebody or somebody folks released Pinocchio. They call this the AI browser. And I want to highlight this because I want to give you practical tips. Janae, I think, is coming in with some breaking news. 96:28 (Speaker A) I don't know if Janae wants to come up or can, but if you can, feel free to come up and tell us there's some news from Bard. Until we talk about Bard, the topic of UIs for those things, and you guys know we're mostly focused on the LLM side and the Engineer side. Less than there's a fusion, but we sometimes have love for both the above tool that you can download and not deal with the terminal, not deal with the bunch of stuff, unifies all of them. 97:08 (Speaker A) It's really nice. Check out the Nokio AI browser. I think it's open source. 97:12 (Speaker A) You download this once, it's cross platform, Mac, PC, et cetera, and then you're able to download Llama CPP, and then you're able to also download table diffusion. And then fairly quickly, without knowing how to code, without going through the terminal, without installing packages, folks here know that installing the packages is like a whole pain we all share and we all hate without doing all of that. That's the promise that they have, you are able to pipe Llama outputs into stable diffusion. 97:38 (Speaker A) So Yam previously mentioned kind of the model that can do, and Yam and Method are talking about a method of generating prompts for LLMs, but also we know that there's models prompts to actually generate prompts for diffusions and they're trained on different and fine tuned on different ways to generate diffusion prompts. Right, and this Pinocchio browser is actually allowing you to run like an and then pipe the output into stabilization model and then see the output of that. I think it's incredible that this exists and is downloadable. 98:07 (Speaker A) I haven't tried this yet. If you in the audience or somebody on stage have tried Pinocchio, please raise your hand. I want to bring you up and talk about Pinocchio and your experience with this. 98:19 (Speaker A) And if we haven't, I want to bring this to our attention so that next week we're able to talk about this. This is added to my list of things I like. The Comfy UI that I haven't tried it yet. 98:29 (Speaker A) Anybody use pinocchio yet? No? Cool. I wanted to get Cocktail Peanut. The guy who wrote Cocktail Peanut. 98:36 (Speaker A) If you're in the audience, feel free to raise your hand. I don't think you are, but feel free to follow the thread. He goes fairly deep. 98:44 (Speaker A) And feel free to use and try Pinocchio by next week and then come up next week and talk about the differences between this and running automatic one one. All right, folks, thanks everyone for coming to another Thursday. I space. 98:58 (Speaker A) Hope this has been helpful for a bunch of you. We tried a few new things here. We tried to give updates, but also deep dive into a conversation with Matt and looks from the reactions here that maybe this is worth putting down on paper and sending out an email for those of you who want to maybe sign up for this and not don't have the time to listen to two hour spaces, so I'll definitely try at least to do that. 99:19 (Speaker A) I want to thank a few folks on stage that have joined consistently and providing a lot of signal yum follow Yam. He has great insights into models and training and different things al in the audience. Thanks always for coming up. 99:33 (Speaker A) Junaid is running the Denver meetup, and if you're in the Denver area, feel free to join us next week. Thanks for coming. Haven't seen you in a while, buddy. 99:45 (Speaker A) Juan sorry. Yeah, I think Juan great. Maxi and Lentos has recently been joining us. 99:51 (Speaker A) It's been great. We have some more folks in the Evans who are regulars, and we invite you to also be regulars and come up and talk about Thursday. I will say this one thing, tag me in anything that's new. 100:01 (Speaker A) I would love that. And help promote the message for other folks. If you did like the space, this also really helps for more folks to get to the bottom of this for those folks. 100:01 (Speaker A) I didn't get to their questions. I apologize. I'm trying to keep this as a balance of a high signal thing versus letting everybody questions as well. 100:22 (Speaker A) Last thing I'll say is about myself, a little bit consultant. I stay up to date so you don't have to. That's my tagline. 100:29 (Speaker A) If you're in the company and needs consultancy for somebody who's up to date on everything, I try to be that guy. Feel free to tap me in the DMs. And, yeah, thursdai folks, keep tagging us everything that's new. We're going to try to cover next week with that. 100:34 (Speaker A) I thank all of you. Thanks for coming. Thanks for giving us two and a half hours of your attention. 100:34 (Speaker A) I really appreciate it. Attention is sparse and very important, and I really thank everybody who gave us, like, two and a half hours. Thank you, folks. 101:00 (Speaker A) Hey, Alex, we really appreciate you. 101:04 (Speaker B) Thanks, Alex. 101:05 (Speaker H) Thanks for doing a good space and keeping us on track, actually. 101:09 (Speaker A) Yeah, thank you. 101:10 (Speaker D) Yeah, alex definitely want to kind of. 101:13 (Speaker A) Give our thanks to you as well. 101:15 (Speaker E) For curating an awesome space. 101:17 (Speaker D) I think I'm definitely not the only one that gets a lot of good signal out of this. And I know a lot of hard work goes into keeping yourself up to. 101:27 (Speaker A) Date so that you can share it. 101:28 (Speaker E) With all of us. 101:29 (Speaker D) So just on my own behalf, thank you. And I'm sure that is echoed by. 101:34 (Speaker E) A lot of people on stage and in the audience. 101:36 (Speaker A) Humble man thank you. I appreciate you. Thank you, folks. Have a nice Thursday and bye next week. This is a public episode. If you'd like to discuss this with other subscribers or get access to bonus episodes, visit sub.thursdai.news/subscribe | |||
21 Jul 2023 | ThursdAI July 20 - LLaMa 2, Vision and multimodality for all, and is GPT-4 getting dumber? | 00:14:40 | |
ThursdAI - Recaps of the most high signal AI weekly spaces is a reader-supported publication. To receive new posts and support my work, consider becoming a free or paid subscriber. If you’d like to hear the whole 2 hour conversation, here’s the link to twitter spaces we had. And if you’d like to add us to your favorite podcatcher - here’s the RSS link while we’re pending approval from Apple/Spotify Happy LLaMa day! Meta open sourced LLaMa v2 with a fully commercial license. LLaMa 1 was considered the best open source LLM, this one can be used for commercial purposes, unless you have more than 700MM monthly active users (no 🦙 for you Google!) Meta has released the code and weights, and this time around, also a fine-tuned chat version of LLaMa v2 to all, and has put them on HuggingFace. There are already (3 days later) at least 2 models that have fine-tuned LLaMa2 that we know of: * @nousresearch have released Redmond Puffin 13B * @EnricoShippole with collaboration with Nous have released LLongMa, which extends the context window for LLaMa to 8K (and is training a 16K context window LLaMa) * I also invited and had the privilege to interview the folks from @nousresearch group (@karan4d, @teknium1 @Dogesator ) and @EnricoShippole which will be published as a separate episode. Many places already let you play with LLaMa2 for free: * https://www.llama2.ai/ * nat.dev, replicate and a bunch more! The one caveat, the new LLaMa is not that great with code (like at all!) but expect this to change soon! We all just went multi-modal! Bing just got eyes! I’ve been waiting for this moment, and it’s finally here. We all, have access to the best vision + text model, the GPT-4 vision model, via bing! (and also bard, but… we’ll talk about it) Bing chat (which runs GPT-4) has now released an option to upload (or take) a picture, and add a text prompt, and the model that responds understands both! It’s not OCR, it’s an actual vision + text model, and the results are very impressive! I’ve personally took a snap of a food-truck side, and asked Bing to tell me what they offer, it found the name of the truck, searched it online, found the menu and printed out the menu options for me! Google’s Bard also introduced their google lens integration, and many folks tried uploading a screenshot and asking it for code in react to create that UI, and well… it wasn’t amazing. I believe it’s due to the fact that Bard is using google lens API and was not trained in a multi-modal way like GPT-4 has. One caveat is, the same as text models, Bing can and will hallucinate stuff that isn’t in the picture, so YMMV but take this into account. It seems that at the beginning of an image description it will be very precise but then as the description keeps going, the LLM part kicks in and starts hallucinating. Is GPT-4 getting dumber and lazier? Researches from Standford and Berkley (and Matei Zaharia, the CTO of Databricks) have tried to evaluate the vibes and complaints that many folks have been sharing, wether GPT-4 and 3 updates from June, had degraded capabilities and performance. Here’s the link to that paper and twitter thread from Matei. They have evaluated the 0301 and the 0613 versions of both GPT-3.5 and GPT-4 and have concluded that at some tasks, there’s a degraded performance in the newer models! Some reported drops as high as 90% → 2.5% 😮 But is there truth to this? Well apparently, some of the methodologies in that paper lacked rigor and the fine folks at AI Snake Oil ( Sayash Kapoor and Arvind) have done a great deep dive into that paper and found very interesting things! They smartly separate between capabilities degradation and behavior degradation, and note that on the 2 tasks (Math, Coding) that the researches noted a capability degradation, their methodology was flawed, and there isn’t in fact any capability degradation, rather, a behavior change and a failure to take into account a few examples. The most frustrating for me was the code evaluation, the researchers scored both the previous model and the new June updated models on “code execution” with the same prompt, however, the new models defaulted to wrap the returned code with ``` which is markdown code snippets. This could have been easily fixed with some prompting, however, the researchers scored the task based on, wether or not the code snippet they get is “instantly executable”, which it obviously isn’t with the ``` in there. So, they haven’t actually seen and evaluated the code itself, just wether or not it runs! I really appreciate the AI Snake Oil deep dive on this, and recommend you all read it for yourself and make your own opinion and don’t give into the hype and scare mongering and twitter thinkfluencer takes. News from OpenAI - Custom Instructions + Longer deprecation cycles In response to the developers (and the above paper), OpenAi announced an update to the deprecation schedule of the 0301 models (the one without functions) and they will keep that model alive for a full year now! Additionally, OpenAI has released “Custom Instructions for ChatGPT” which allows a chatGPT user to store custom instructions, information and custom prompt that will be saved on OpenAI server side, and will append to every new session of yours with chatGPT. Think, personal details, preferred coding style (you love ruby and not python) and other incredible things you can achieve without copy-pasting this to every new session! Don’t forget to enable this feature (unless you’re in the UK or EU where this isn’t available) Thanks for tuning in, wether you’re a newsletter subscriber, twitter space participant, or just someone who stumbled onto this post, if you find this interesting, subscribe and tell your friends! “We stay up to date so you don’t have to” is the #ThursdAI motto! 🫡 In other news this week: LangChain has gotten some flack but they are looking ahead and releasing LangSmith, an observability framework for your agents, that does NOT required using LangChain! It looks super cool, and is very useful to track multiple prompts and tokens across agent runs! And the results are share-able so you can take a look at great runs and share yours with friends! Don’t forget to share this with your friends and come back next week 🫡 — Alex Volkov This is a public episode. If you'd like to discuss this with other subscribers or get access to bonus episodes, visit sub.thursdai.news/subscribe | |||
23 Jul 2023 | ThursdAI - Special Episode, interview with Nous Research and Enrico Shippole, fine-tuning LLaMa 2, extending it's context and more | 00:37:04 | |
Hey there, welcome to this special edition of ThursdAI. This episode is featuring an interview with Nous Research, a group of folks who fine-tune open source large language models to make them better. If you are interested to hear how finetuning an open source model works, dataset preparation, context scaling and more, tune in! You will hear from Karan, Teknium, LBJ from Nous Research and Enrico who worked along side them. To clarify, Enrico is going in depth into the method called Rope Scaling, which is a clever hack, that extends the context length of LLaMa models significantly and his project LLongMa which is an extended version of LLaMa with 8000 token context window. The first voice you will hear is Alex Volkov the host of ThursdAI who doesn’t usually have a lisp, but for some reason, during the recording, twitter spaces decided to mute all the S sounds. Links and acknowledgments: * Nous Research - https://nousresearch.com/ (@nousresearch) * Redmond Puffin 13b - First LLaMa Finetune * LLongMa - LLaMa finetune with 8K context (by Encrico, emozilla and KaioKenDev) * Nous-Hermes-Llama2-13b-GPTQ - Hermes Finetune was released after the recording 🎊 Psst, if you like this, why don’t you subscribe? Or if you are subscribed, consider a paid subscription to support #ThursdAI Show transcription with timestamps: Alex Volkov - targum.video (@altryne)[00:00:55] Yeah. That's awesome. So I guess with this, maybe, Karan, if you if you are able to, can you you talk about Nous research and how kind of how it started and what the what are you guys doing, and then we'll dive into the kind of, you know, Hermes and and Puffin and the methods and and all of it. karan (@karan4d)[00:01:16] Absolutely. Nous research. I mean, I I myself and many other of us are just, like, enthusiasts that we're fine tuning models like, you know, GPTJ or GPT 2. And, you know, we all are on Twitter. We're all on Discord, and kind of just found each other and had this same mentality of we wanna we wanna make these models. We wanna kinda take the power back from people like OpenAI and anthropic. We want stuff to be able to run easy for everyone. And a lot of like minds started to show up. karan (@karan4d)[00:01:50] I think that Technium's addition initially to Nous research, Jim, kinda showing up. And himself, I and human working on compiling the Hermes dataset was really what came to attract people when Hermes came out. I think we just have a really strong and robust, like, data curation thesis in terms of that. And I think that have just some of the most talented people who have come to join us and just volunteer and work with us on stuff. And I absolutely must say, I can see in the in the listeners is our compute provider, Redmond AI. karan (@karan4d)[00:02:30] And, you know, none of this none of these models would be possible without Redmond's generous sponsorship for us to be able to deliver these things lightning fast, you know, without making us through a bunch of hoops just a a total total pleasure to work with. So I would I have to shell and say, you know, I highly recommend everyone check out Redmond as because they really make our project possible. Alex Volkov - targum.video (@altryne)[00:02:52] Absolutely. So shout out to Redmond AI and folks give them a follow. They're the the only square avatar in the audience. Go take them out. And, Karan, thanks for that. I wanna just do a mic check for teknium. Teknium. Can you speak now? Can you? Can I hear you? Teknium (e/λ) (@Teknium1)[00:03:08] Yeah. My phone died right when you were introducing me earlier. Alex Volkov - targum.video (@altryne)[00:03:10] Yep. What's up, Eric? -- sometimes on Twitter basis. Welcome, Technium. So briefly, going back to question. I don't know if you heard it. What besides the commercial and kind of the the contact window, what kind of caught your eye in the llama, at least the base until you guys started, or have you also, like, the other guys not had a second to play with the base model and dove into fine tuning directly? Teknium (e/λ) (@Teknium1)[00:03:35] Yeah. The only thing that really caught my eye was the chat model and how horribly RLHF it was. Alex Volkov - targum.video (@altryne)[00:03:41] Yeah. I've seen some conversations about and kind of the point of Ira, RLHF as well. And okay. So so now that we've introduced Neus research, sorry, I wanna talk to you guys about what you guys are cooking. Right? The we've seen, the the Hermes model before this was, like, loved it as one of the, you know, the best fine tunes that I've seen at least and the the the most performing ones. Could you guys talk about the process to get to the Hermes model, the previous one? and then give us things about what coming soon? karan (@karan4d)[00:04:16] Teknium, you got this one. man. Teknium (e/λ) (@Teknium1)[00:04:22] Yeah. It was basically I saw Alpaca, and I wanted to make it like, remake it with GPT 4, and then from there and just pretty much exclusively included anything that was GPT 4 only, and that was the beginning of the thesis for that. Going forward, though, We still have a lot of low quality data, I think, in Hermes data set that can be cleaned out, and then there's a lot of new data sets that have come out that I wanna start merging into there. also wanna move to something like chat ML or even Vikura format so that we can do some multi turn stuff. It's not very great, long chat. Alex Volkov - targum.video (@altryne)[00:05:03] Yeah. karan (@karan4d)[00:05:03] Within within within the Hermes dataset, you know, a lot of it is public available stuff that's particularly GPT 4. Of course, Technium's massive GP teacher dataset. We also have a bunch of GPT 4 data we had generate that we didn't release necessarily just yet, as well as an instruction set that's particularly focused on tasks like Python, transformers, linguistics, very small dataset of that. That's inside Hermes that, you know, we don't really talk about much, but figure that we'll put some exposure to right now on the spaces. And yeah. Alex Volkov - targum.video (@altryne)[00:05:42] That's awesome. And so the previous Hermes was released on top of LAMA 1, and for many folks, know, obviously, they couldn't use this for different commercial points. And now that this model relates, what the models that you guys release, are you thinking about the license of them? And could you talk about, like, the availability of folks using them in commercial standing now that, you know, the the back of it is commercially available. LDJ (@Dogesator)[00:06:07] Mhmm. I think we have puffin licensed us MIT I'll have to doublecheck on our own own model. I think that's right, Curran, right, or Tech? karan (@karan4d)[00:06:18] Yeah. I think so either that or Apache 2 point Like, if if the base model is commercially usable, you know, the stuff we put out is you're good to go. It's -- Yeah. LDJ (@Dogesator)[00:06:29] So And, like, in our announcements, I put in kind of, you know, one of the main things. It's it's commercially available. the first Nous as far as I think yeah. I'm pretty sure it's the first commercially available Nous model that's released, and a big differential data from Hermes is the fact that, like tech was saying, Hermes is pretty much all single turn data. And it's surprisingly can do pretty decent at multiturn conversations when you actually use it. But then puffin is almost kind of, like, a 180 where it's a vast majority really on context multi turn data. LDJ (@Dogesator)[00:07:09] And oh, I think can you guys hear me so? I can hear. Okay. It's just something's up with that. Okay. Yeah. So puffin is a vast majority, multi turn data, GPT 4 specifically, and a lot of it is actually real human conversations with GPT for that go on for some of them 4k 6 k context, like, even all the way up to the max 8 k context length of GPT 4. And then we took those few thousand conversations of real humans interacting with GPT 4. And now after that, I'm not sure if you've A lot of people probably heard of Camel AI. LDJ (@Dogesator)[00:07:46] So they have the physics, biology, chemistry, and mathematics data set. And then within those, there's a bunch of subtopics that you can carry it through. And I just pretty much spent a good few days curating just handpicking the right subtopics, like differential geometry, logic problems, optimization problems, a bunch of different GPT, for examples, and responses from those different subtopics. And then I specifically added those in certain ways to the puffin dataset. Alex Volkov - targum.video (@altryne)[00:08:17] Awesome. So just just looking for the audience maybe. The puffin model that I think the official name is the red redmon puffin 7B or, sorry, 13B. Yes. This is this is the model that you guys fine tuned, and one of the first is maybe not the first fine tune of llama v Two. that's now publicly available, like you said, maybe with MIT license on Huggingspace, and I think you even added the GGML quantized version. Correct? Mhmm. So and so folks can can go and download that and and already start playing with this. And so first of all, thank you for contributing to the open source. That's great to see. And the speed with which you guys are fine tuned on this is also great to see. Alex Volkov - targum.video (@altryne)[00:08:55] And maybe now that we've introduced this, maybe this is like repeating a bit. So could you speak about the the difference so the difference is the in the data set, in the task that you fine tune? Like, what is the actual difference between the Hermes or the Hermes that's coming out and the Puffin model? What would people use them for differently? Is that like that? That's a question. Teknium (e/λ) (@Teknium1)[00:09:21] The profit model definitely be better at multi turn stuff. That's for sure. Yeah. nisten (@nisten)[00:09:28] So if you want to do anything like OpenAI I'll I'll paste the link above the GGML version of it because I I really I'm I'm gonna test it thoroughly, but I I really think because you guys have use GPT 4, high quality, multi turn conversations, then this can have actual, like, practical use for whoever else was to use it either as, like, something that tells you about the documentation on the site or walks a user through. In other words, this should be better than Hermes then in for, like, customer service stuff, which is just one example. nisten (@nisten)[00:10:08] Anyway, yeah, I'm gonna try. I'll I'll paste the the link above. karan (@karan4d)[00:10:14] It's it's likely better for production use alongside, like, stuff that you have with, like, a retrieval pipeline, like, with lang chain, etcetera. Like, I I would believe that without to get it, you know, or just talking, of course. But, you know, there is even though, you know, with this Lima tech unique of of small examples where we can get, like, a a really good really good model that does really well. karan (@karan4d)[00:10:41] The thing about Hermes dataset and just its size and the various types of data and topics that are in there, I think you get a totally different like, role play or storytelling experience or completion experience with Hermes. Personally, I feel that way. Alex Volkov - targum.video (@altryne)[00:11:01] Awesome. Teknium (e/λ) (@Teknium1)[00:11:01] So and that. Another thing about Puffin Dataset is that it does go up to, like, 8K and Enrico here. has been doing a ton of work on extending Llama's context. Alex Volkov - targum.video (@altryne)[00:11:13] Right. So I wanna I wanna give an introduction then introduce Enrique and and talk about this real quick. Right? LAMA version 1 was released with, again, 2,000 tokens in the contact window. And then many folks, including KaioKendev, and Emozhila. Right? And and some other folks, I think, were involved in bringing some of the quote on quote tricks about what eventually ended up being named rope, scaling, if I'm if I'm not mistaken. And we follow this, and we've talked about the previous news ThursdAI, I. And Llama V2 was released with 4000 tokens in the context window. Alex Volkov - targum.video (@altryne)[00:11:52] And, you know, we're now still used to kind of Claude and the 16k GPT 3 that four didn't seem like a lot. And then many folks were wondering, and, meanwhile, Enrico was working, whether or not the rope scaling method would apply to the next plumber and look like it did. And so I wanna introduce Enrico uh Enrico Shippole. I hope on staying this right. Welcome to the state. Hopefully, you can unmute and and this place works with you. And The second finetune that I saw rest of the was also back with Nous, the Nouse research, and this was the extended version, what's called Longma. Alex Volkov - targum.video (@altryne)[00:12:28] So Enrique will go out of the stage and feel free to introduce yourself, your affiliation with news and LlongMa with with the context window. Enrico Shippole (@EnricoShippole)[00:12:38] Hello. So I'm actually a independent researcher. I'm sponsored by Stability AI, Eleuther AI, and a few other different organizations, including NewsNow. Awesome. I work with different people like Tanishq from Medark, Aaron Komatsusaki, who also is from a Luther and Duck AI. John Ney from Nomosai. So I I have a I have a lot of affiliation with a bunch of different organizations. including together. We're starting a project right now with them. Alex Volkov - targum.video (@altryne)[00:13:13] That's that's so great to hear, and so welcome to Thursday. Welcome to this day. And can you talk to us a little bit about kind of the ROPE scaling method and and how how were you able to, like, find them like this quickly and how the results looked so far? I wasn't able to run this myself. But hopefully, yeah, talk to us about Enrico Shippole (@EnricoShippole)[00:13:34] Okay. So initially, The the thing is I actually was hoping that both Emozilla, Bowen, and KaioKenDev would have been able to make it because It was kinda like a equal parts effort on, like, all fronts from each of us. Initially, I had trained some pathways models at 8,000 context length about 4 months ago based on the exposition paper, which did rotary embedding scaling initially. They were one of the first people did it. They based their methodology off of ofer presses alibi. Enrico Shippole (@EnricoShippole)[00:14:11] I would imagine that most people are pretty familiar with Ofir Press in this work on the alibi positional bias that's been used in a wide range of models now. So Emozilla and I came into contact based off of the work that he had seen me doing with the Palm models scaling those to 8000 context length pretraining, not fine tuning. So what we had initially done is basically take a section of c 4 in different data sets that had examples that were all over 8000 context length that pretrained on them packed together. Enrico Shippole (@EnricoShippole)[00:14:50] with a beginning of string and end of string token to help with, like, the attention masking portion of that. After he had seen that, Emozilla actually became into contact with kaikode dev I believe Kaiokendev is how you pronounce it. Kaiokendev had also been following Ofir Press's research. He had started working on his own version of scaling the rotary embeddings, I believe based off of both alibi and exposition. Enrico Shippole (@EnricoShippole)[00:15:22] And what he found is that by scaling the max position all embeddings and the rotary embedding from something like 2048, which you would initially train with. He scaled it up to 8000 or 8192. And he found that by applying, like, in interpolation to the encoding by scaling basically like the the positional index in the rotary embedding, that you were able to essentially turn down the frequency window and rope by like a factor of 0.25. Enrico Shippole (@EnricoShippole)[00:16:01] The scaling depends on the length that you're trying extrapolate to and the initial context length that the model was trained with. So if you were training with LAMA 2, which had an context window of 4096, and you wanted to do the linear interpolation positional scaling to something like 8192. then you would use a scaling factor of 0.5. If you were trying to do it from 2048, which is the original LAMA was trained with, and you wanted to scale it to 8192, then you would use a scaling factor of 0 point 25. Enrico Shippole (@EnricoShippole)[00:16:39] So basically, after we had done all of this, Meta had released a paper around the same time that Kaiokendev had released his blog. They both found very similar finding. They had shown in the meta paper that you only had to fine tune for 1000 steps with the linear positional interpolation scaling to be able to get the benefit of doing a full pretrain at a context window of 8192. Enrico Shippole (@EnricoShippole)[00:17:13] So this is actually like a a big step because it shows that you no longer need to pre train right off the bat at a longer context length. Then you're able to do the fine tuning on essentially a a lower resource like, computational budget and still be able to get the, like, greater results of the longer context window. I know a lot of the major AI companies had been doing just for my work in in personal research with many of them had been doing staged scaling of the context window during training. Enrico Shippole (@EnricoShippole)[00:17:46] So they would pre train basically, when pre training, they would separate the initial examples from a dataset into multiple stages. Enrico Shippole (@EnricoShippole)[00:17:54] So anything that is under the window of 2048, you'd separate from the initial dataset then you take things between 2048 4096, then 4096, and 8192, and you would basically chunk the data sets into those different parts you'd first initially train on the 2048 chunk of the data, then you would train on the data between 2048 and 4096, and then you would do the same thing from 4096 to 8192, or if you want to scale that to 16k or 32k context length. But what we have shown now with both the meta paper and this thing, you don't even need to go through that extensive pretraining and staged process, you can just go from a context length of 2048 to 8192. Enrico Shippole (@EnricoShippole)[00:18:47] scale the rotary embeddings by whatever type of factor that you want to use. So like I was saying, if you're going from 2048 to 8192, you'd be using a scaling factor of 0.25. It only needs 2 lines of code to be able to do that. In the LLongMa post, I had provided an example of scaling the rotary embeddings. The the code was written by Emozilla or Jeff. Enrico Shippole (@EnricoShippole)[00:19:15] We also came into contact with after all these experiments we then came into contact with Bowen, who had worked a lot about the dynamic NTK scaling with Emozilla, and he had also done NTK by parts which we're we're currently training a lot of models on. So we have the Longma 1 models trained on the open llama series, like the suite of those models that use the linear interpolation scaling. Enrico Shippole (@EnricoShippole)[00:19:45] We now have the llama 2 models or the longma 2 suite, which is what we're calling it, again, trained on the linear interpolation scaling And then we have another suite of models coming out very soon that uses the the NDK by parts dynamic scaling. That was really specialized by Bowen, so I do not wanna speak on his behalf. It'd it'd probably be good to get him to talk about it in another one of these. Alex Volkov - targum.video (@altryne)[00:20:14] Absolutely. So let's get in touch after this and and and and set it up. So Thank you for the a very in-depth kind of explanation because we did cover the the the kind of the RoPE killing and how Kaioken in the image boards are ready to wherever he started this in his blog post, and then how it's gonna rotate it. So it's great to to actually hear from the folks who are doing this. I just for the audience, I've attached Enrico's tweet about LLongMA 2, which is now currently trained at AK contact length. Alex Volkov - targum.video (@altryne)[00:20:47] And and Rico, you told us that we may see even double from the So could you think about the next the next version? Enrico Shippole (@EnricoShippole)[00:20:56] Okay. So the the initial training process of doing this up to a context, like length of 8192, can be due with be done, basically, with deep speed, 02. and activation checkpointing. And you're able to fit the model on a A100 80 gigabyte node. Now, we are working on the process of scaling it both to 16 k and 32 k. This requires a different methodology during training, you either need to use deep speed 0.3 or fully sharded data parallelism. Enrico Shippole (@EnricoShippole)[00:21:35] Both of those are are very similar for people who aren't aware. Basically, you're just sharding the optimizer states. The model states across, like, different nodes. You can also use things like tensor parallelism to help with the scaling as well. And then we're going to be basically just adjusting the scaling factor again, collecting a large we've already collected large quantity of data at 16k context length, and we're going to be doing the fine tuning to 16k and be releasing those models Soon, all of this computing is sponsored by stability AI. Enrico Shippole (@EnricoShippole)[00:22:12] They've been very generous what helping with a lot of the independent research. Alex Volkov - targum.video (@altryne)[00:22:17] That so I wanna shout out Stability AI for not only given, you know, the world's stability diffusion, also participating in this kind of next wave of AI. Many folks kinda coined the stability AI moment when released the the stable diffusion of the. I wanna say 1.4 back then almost a year ago now, and many folks are saying the about the Llama 2 release now this commercially open source, and and folks can start, like, doing things for you know, for profit companies can join So we definitely wanna shout out stability for for the effort here. And, Enrico, thank you. And, folks, please follow Enrico, and and we'll stay tuned. Alex Volkov - targum.video (@altryne)[00:22:56] I wanna ask Karan and and Teknium, and other folks from Nous the efforts that that Enrico was talking about. the longer context windows. How would they kinda interplay with the stuff that you're working on with Hermes with with Pufin? Are are kind of the efforts interchangeable? We're gonna see building a top of each other? karan (@karan4d)[00:23:16] So I I think LDJ can definitely speak to this, but I'd like to happily say that once we did Longbow 1 on the 1st Llama generation of models, we already had puffin 2k, 4k, and 8 for that -- Yeah. -- already prepared and ready. So as the LLongMa models for 13B are released, we will also be doing equivalent, puff in fine tunes, and Potentially Hermes fine tunes. We can talk a little bit more about the future of Hermes at a a little bit later, though. LDJ (@Dogesator)[00:23:51] Yeah. I mean, I was pretty much going to say the same thing, but kind of elaborate on that about how before when LLongMa V1 and everything. And during the development of LLongMa, there was actually, like you know, of course, me Enrico who are usually just called concepts of mind and and and Emozilla. Like, we've all kinda, like, been butting shoulders a lot together and just kinda working closely, you know, in the same Discord and whatnot. And it's like, hey. Like, you know, working on this, like, experimental LLongMa with thing. Like, hey. You wanna try, like, fine tuning, and then the plan just kind of ended up being like, okay. Just gonna have this Puffin thing. LDJ (@Dogesator)[00:24:31] that Puffin dataset is already containing a ton of high context conversational data. from GPT 4 and, like, human high quality data. So it's like it's like the perfect fit to have something that's high context capable will be fine tuned on that. And then LLaMa 2 came out, and it's like, oh, Yeah. Let's let's get this out ASAP, and then we'll figure out what we're gonna do later. Alex Volkov - targum.video (@altryne)[00:24:58] Yeah. Great. And it's just great to see, you know, how many opportunities is like this where with open source can the stuff that we're able to now run and gonna iterate on are building on top of each other. They're just incredible. and this is maybe a watershed moment. And I I wanna thank all of you for being here. I wanna kind of let the other folks who usually hear on Thursday, I need to ask you a question or 2 for Nous visitors. Yam and Nisten, if you if you have a question for news or for Enrico, go ahead. I I will stay young. Alex Volkov - targum.video (@altryne)[00:25:29] I know you if you have to ask the super deep technical stuff, and the audience will, like it will fly over their I I won't be using the DM with LBJ and and Rico. But yeah. Of course, the stuff that we haven't covered and interesting tough news. Feel free as it pertains to LAMA 2 is gonna be very interesting, I think, for everyone. nisten (@nisten)[00:25:47] Just to quickly clarify, you guys fine tuned the plain model. Right? Not the chat 1. Teknium (e/λ) (@Teknium1)[00:25:55] Yep. Okay. Yep. The base model. We wouldn't fine that model. The chat 1 at all. Alex Volkov - targum.video (@altryne)[00:26:00] Actually, to -- Yeah. The -- -- to maybe continue this stratigram for interrupting. Just one sec. To continue this question, the there are models they were released by Meta, and you have to, like, register and get the email and everything. And then they put some stuff on Hugging Face. And then the the those models were delineated with, like, dash HF. Have you guys use the HuggingFace or the Meta 1, and do you guys know the difference? I felt somebody that, like, maybe doesn't work as well and to inform her Yeah. Teknium (e/λ) (@Teknium1)[00:26:30] The one on Hugging phase is an FP 16 and the original Llama 2 models in bf16, but we tested the difference between the two models at Carper, and there's such a negligible difference in their quality that it's irrelevant, but we trained on the Hug and Face f P Sixteen ones, but in the f Sixteen ask them. Alex Volkov - targum.video (@altryne)[00:26:52] Sorry. Yeah. Goran, for interrupting. Go ahead. karan (@karan4d)[00:26:56] No. All good. Alex Volkov - targum.video (@altryne)[00:26:58] I I totally forgot what -- That's not it. interrupted today. Yes, Randall. Okay. Nispen, if you have a question for Kiran to follow-up with feel free, and And if not, then, Yum, if you have anything that you wanna ask the the fine folks from Nous, feel feel free as well. Yam Peleg (@Yampeleg)[00:27:17] Yeah. Sure. First, thank you for what you're doing, guys. You're really making a difference for anyone. There aren't many demos online, so anyone that didn't try Hermes, I highly encourage you to try. I don't know why there aren't them. Okay. I know why there aren't demos that cost money, but just try it. Okay? And now I got a question because from my experience, if you train on the open datasets of Hermes, you get a significantly less quality of a model. No. Now I'm fine I'm fine if you don't release datasets. Don't don't get me wrong. Yam Peleg (@Yampeleg)[00:27:54] Just I wanted to ask, is there anything else besides the data that is different? What what tips can you give for, I don't know, someone else that want to train high quality model besides having high quality data. Teknium (e/λ) (@Teknium1)[00:28:08] Everyone understands this. Yeah. The hyperparameters can make key difference. LBJ knows very well because we had to do a ton of different tests. We don't have our freight owners for puffin model. But I'm not sure if those are on the model card for Hermes. If they're not, I can put them And Karen your card can probably talk about the Nous datasets that weren't made public. karan (@karan4d)[00:28:38] Yeah. We've got, like, maybe around, like, 50 k items of data, like, versus, like, total 300 k instructions there that are not released. And to be frank with you about 45 k of them is just more GPT 4, like, alpaca style instructions. The 5000 or so, the, like, 4500 them compose this dataset we have we've been working on that, you know, at this point, I'm pretty comfortable talking about a we call it the p dactyl dataset. karan (@karan4d)[00:29:14] I won't speak on everything that's in it, but, essentially, And I don't know if this is the thing that made the big difference, but it's, like, the the one place where I guess you deviate from just using the open datasets more GPT 4 instructions, but it's got some transformers instructions, some linguistics instructions, some calculus 1, instructions, etcetera. It seems to be pretty good. Teknium (e/λ) (@Teknium1)[00:29:41] Also, Yam, do you have links or anything to the models that tried it with just the makeup of the datasets that we're public from Hermes because I haven't actually seen that before. Yam Peleg (@Yampeleg)[00:29:57] And again, can you repeat that? Teknium (e/λ) (@Teknium1)[00:29:58] didn't hear. Do you have any links to the models that trained with just the open datasets from Hermes that you could share with me later? Yam Peleg (@Yampeleg)[00:30:06] No. No. It's just it's just from my experiments -- Oh, okay. -- on training. Pretty much following the same idea of let's take only GPT 4 from all the open datasets, and the the model that you get is is different. for sure. And and it might be that hyperparameters, you know. Teknium (e/λ) (@Teknium1)[00:30:25] Another thing that we did too is pretty extensive, like, cleaning. We did do deduplication. We removed things like a URL. Like, any response that had a URL in it, we removed in case it was gonna like, hallucinated URLs. Instead of, like, maybe 8 different filtering processes too that might have made our data quality higher. LDJ (@Dogesator)[00:30:48] So as an AI language model? nisten (@nisten)[00:30:51] For anybody -- What do you say? -- for anybody in the audience that hyperparameter meters are are just like the settings in the oven. So it it looks here, like, the ingredients were all okay, but yam mess something up, and before selling as a token -- Yeah. -- came out half baked at the model. LDJ (@Dogesator)[00:31:08] So we're gonna have to check that out. LDJ (@Dogesator)[00:31:10] I'm a big proponent personally of hyperparameter optimization being underrated right now, like, in -- Yeah. -- the current space. And that's something I've kind of focused on a lot specifically for things like puffin and just trying to help others around and use some stuff like trying to optimize they're doing, and even just something like like what you just said about the settings for the oven, I mean, double the amount of time you're putting something in the oven, and it's not gonna come out twice as good. It's not even gonna come out 10% as good. It's gonna come worse. You know? LDJ (@Dogesator)[00:31:45] And although it depends, like, what is your baseline for how how much time you're putting it in the oven and all these different variables that kind of are dependent on each other and affect each other. So it's definitely something you kind of have to build an intuition about to some degree. And then the other end is really I feel like there has to be more investment and more time and energy invested into actual tools that make hyperparameter optimization easier for people that are doing these things. Yam Peleg (@Yampeleg)[00:32:13] Yeah. Yeah. And the thing is that the models are are really big, so it's really expensive to run them. So you have you have a trade off of how many how much computer you're investing in searching hyperparameters rather than actually using it for training. But but I completely agree So one one last question, actually, too. Teknium (e/λ) (@Teknium1)[00:32:33] Actually, one thing before we go on. Something great about the puffin dataset is that it's just like, 3000 or so examples, I believe. And so it makes tuning a lot less expensive because you can finish the whole training in just a couple of hours. So, like, with Hermes, if we wanted to try full ablations and dozens of them, it would take weeks weeks to do. LDJ (@Dogesator)[00:32:55] Yeah. Yeah. Well, to be fair, it's not like it only takes a couple hours on one GPU. We use a a 100 80 gigabytes. So Yeah. Yeah. Teknium (e/λ) (@Teknium1)[00:33:04] Courtesy of Redman. Alex Volkov - targum.video (@altryne)[00:33:05] Thank you, Redman. Enrico Shippole (@EnricoShippole)[00:33:08] Mhmm. I should also probably clarify that when doing the context length, extrapolation, We're doing it on 1,000,000,000 tokens and 64, 80 gigabyte a 100. Yam Peleg (@Yampeleg)[00:33:20] OOf Mhmm. Alex Volkov - targum.video (@altryne)[00:33:23] Yeah. Yam is getting over excited. Alright, folks. I wanna -- Yeah. Yeah. -- maybe maybe ask her on this one less and we'll move on to the the the regular ThursdI update camera cadence. But I will say that, like, folks from Nous research and and Rick and and some other here. Thank you so much for coming up and giving us kind of the insights into how this actually happens. Lama2 just released, you know, a few days ago, and you guys are already pumping out, like, open source fine tuned models. And it's great to see. And just so you know, there's always a stage for you here to come in and and announce things. Alex Volkov - targum.video (@altryne)[00:33:53] And If you do wanna announce, like, a release or something, maybe just, you know, right now, Karan and and Teknium and some folks, I would love to hear like, when the next Hermes is coming? karan (@karan4d)[00:34:06] Before we say that, I just would like to clarify something about Hermes. So we have the original Hermes dataset on LAMA 2 as something that we will release, but also a sequel to the Hermes dataset, Hermes 2. There will be a distinction between these 2, and you'll see you'll see the the the prior come out first and the latter come out after. But as for release, etcetera, I will absolutely let Technium take the stage with those final words. Teknium (e/λ) (@Teknium1)[00:34:36] So the training is nearly done. At least it was about 2.8 epochs out of 3 a few hours ago. So it might be done already. Before I release it though, unlike puffin, I didn't we wanted it puffing out, like, same day that llama 2 came out, so we didn't run any benchmarks. And we had to put all the compute we had on Hermes immediately after we were done with that. So we don't have any compute to do any benchmarks or puffing until Hermes is done. Teknium (e/λ) (@Teknium1)[00:35:06] But before I release Hermes, I do wanna do, like, a full range of benchmarks and stuff like that to make sure everything's good and have a pretty detailed model card, but that should probably only take the rest of tonight at the most. So probably tomorrow morning would be when Hermes comes out. Alex Volkov - targum.video (@altryne)[00:35:22] That's some folks. And you you heard it here first and definitely follow Teknium, Karan, Enrico, LDJ, and the rest of, like, Nous Research folks, and stay tuned. Enrico, go ahead. Enrico Shippole (@EnricoShippole)[00:35:34] Yes. I just wanted to to piggyback off of Teknium comment a little bit. So we did do pretty sense of the valuation of the Lauma 2 AK models. We had run different things on perplexity using Gov Report in a couple different other data sets to make sure that the length extrapolation in the context was working properly. We did passkey retrieval. We also did a lot of extensive human evaluation, which took a little bit. I had wanted to get the LAMA 2 AK models out yesterday, but we decided to push it back one day. Enrico Shippole (@EnricoShippole)[00:36:08] So and what we were doing is we were feeding in research papers and seeing if it could pull out even, like, relevant pieces of information from the context length. And so far, it has been quite successful. So we're we're still running more evals, but the ones so far have shown that there's been, like, no performance degradation, no matter what context length that you're basically using with these extended models. Alex Volkov - targum.video (@altryne)[00:36:32] That sounds great. and now that this this, you know, LLongMa lies out and the next versions are gonna come out as well. I'm sure that some other folks who also contribute to this research and tell you, like, from their own experiences and vibe. So, yeah, I wanna thank folks. Again, this has been very illuminating, and very glad to have you. And, obviously, the stage is yours whenever you want to come here, and we appreciate you. And you guys are welcome to stay tuned and kinda chime in to the rest of the updates. And with that, I think, for folks in the audience, we're moving to the next thing. ThursdAI - Recaps of the most high signal AI weekly spaces is a reader-supported publication. To receive new posts and support my work, consider becoming a free or paid subscriber. This is a public episode. If you'd like to discuss this with other subscribers or get access to bonus episodes, visit sub.thursdai.news/subscribe | |||
27 Jul 2023 | 🎙️ThursdAI - Jul 27: SDXL1.0, Superconductors? StackOverflowAI and Frontier Model Forum | 00:18:54 | |
⏰ Breaking news, ThursdAI is now on Apple Podcasts and in this RSS ! So use your favorite pod-catcher to subscribe or his this button right here: Our friends at Zealous have provided an incredible platform for us to generate these awesome video podcasts from audio or from twitter spaces so if you prefer a more visual format, our deep thanks to them! P.S - You can find the full 2 hour space with speakers on our Zealous page and on Twitter Here’s a summary of the main things that happened in AI since last ThursdAI: 🧑🎨 Stability.ai releases SDXL1.0 * Generates 1024px x 1024x stunning images * High high photorealism * Supports hands and text * Different (simpler?) prompting required * Fine-tunes very well! * Supports LORAs, ControlNet in-painting and outcropping and the whole ecosystem built around SD * Refiner is a separate piece that adds high quality detail * Available on Dreamstudio, Github, ClipDrop and HuggingFace * Also, is available with incredible ComfyUI and can be used in a free Colab! Image Credit goes to Thibaud Superconductors on Hugging Face? What? Honestly, this has nothing immediate to do with AI updates, but, if it pans out, it’s so revolutionary that it will affect AI also! Here’s what we know about LK-99 so far: * 2 papers released on arXiv (and hugging face haha) in the span of several hours * First AND second paper both claim extraordinary claims of solving ambient superconductivity * Ambient pressure and room temp superconductive material called LK-99 * Straightforward process with a clear replication manual and fairly common materials * Papers lack rigor, potentially due to rushing out or due to fighting for credit for nobel prize * The science is potentially sound, and is being “baked and reproduced in multiple labs” per science mag. Potential effects of room temperature superconductivity on AI: While many places (All?) can benefit from the incredible applications of superconductors (think 1000x batteries) the field of AI will benefit as well if the result above replicates. * Production of GPU and CPU is power-constrained and could benefit * GPU/CPUs themselves are power-constrained while running inference * GPT-4 is great but consumes more power (training and inference) than previous models making it hard to scale * Local inference is also power-restricted, so running local models (and local walking robots) could explode with superconductivity * Quantum computing is going to have a field day if this is true * So will fusion reactors (which need superconductors to keep the plasma in place) As we wait for labs to reproduce, I created a twitter list of folks who are following closely, feel free to follow along! AI agents protocol, discussion and state of for July 2023 * Participated in an e2b space with tons of AI builders (Full space and recap coming soon!) * Many touted AI agents as a category and discussed their own frameworks * Folks came up and talked about their needs from the agent protocol proposed by e2b * Agents need to be able to communicate with other agents/sub agents * Tasks payloads and artifacts and task completion can be async (think receiving a response email from a colleague) * The ability to debug (with timetravel) and trace and reproduce an agent run * Deployment, running and execution environment issues * Reliability of task finish reporting, and evaluation is hard Frontier model forum * OpenAI, Anthropic, Google, and Microsoft are forming the Frontier Model Forum to promote safe and responsible frontier AI. * The Forum will advance AI safety research, identify best practices, share knowledge on risks, and support using AI for challenges like climate change. * Membership is open to organizations developing frontier models that demonstrate safety commitment. * The Forum will focus on best practices, AI safety research, and information sharing between companies and governments. * Some have expressed concern that this could enable regulatory capture by the “Big LLM” shops that can use the lobbying power to stop innovation. StackOverflow AI - “The reports of my death have been greatly exaggerated” Stack overflow has been in the news lately, when a graphic of it’s decline in traffic has become viral. They have publicly disputed that information claiming they have moved to a different measuring and didn’t update the webpage, but then also… announced Overflow AI! * AI search and aggregation of answers + ability to follow up in natural language * Helps drafting questions * AI answers with a summary, and citations with the ability to “extend” and adjust for your coding level * VSCode integration! * Focusing on “validated and trusted” content * Not only for SO code, stack overflow for teams will also embed other sources (like your company confluence) and will give you attributed answers and tagging abilities on external content This has been an insane week in terms of news (👽 anyone?) and superconductors and AI releases! As always, I’m grateful for your attention! Forward this newsletter to 1 friend as a favor to me if you learned something new? Or alternatively, retweet us on twitter for bigger reach! Thank you! See you next ThursdAI (and on Sunday when I release the State Of Agents recap 😅 ) ThursdAI - Get in on this, and share w/ 1 friend 🫡 This is a public episode. If you'd like to discuss this with other subscribers or get access to bonus episodes, visit sub.thursdai.news/subscribe | |||
30 Jul 2023 | 🧪 LK99 - The superconductor that can change the world, and the K-drama behind it! | 00:50:15 | |
This is a free preview of a paid episode. To hear more, visit sub.thursdai.news First of all, let me address this from the get go, I’m not a material scientist! I am pretty good at finding information in twitter’s incredibly noisy info stream. (hey, this is how I bring you AI updates every ThursdAI) Since LK-99 is potentially groundbreaking and revolutionary, I’ve compiled a twitter list of everyone who I found credible, interested and a source of new information, and there’s now over 1.5K followers to this list alone! Since this clearly is interesting to a lot of you, I reached out to a few prominent people on this list, and asked them to join a twitter space, to try and stitch together an update on the current state of LK-99, replication attempts, history and lore, as it stands a week after the original papers release. If you found this interesting, you’re the type of person who wants to stay up to date, feel free to subscribe and keep this Substack alive! First of all, let’s do some level setting. Superconductors are real, we’ve used them in MRI machines for example, but the currently available superconductors need extremely low temperature and high pressure to well.., and the promise of a room temperature and ambient pressure superconductor is the holy grail of energy use. For a breakdown on what superconductors are, and what they can mean for the world, I strongly recommend this thread from Andrew Cote (published presciently a full two weeks before the LK-99 paper) or watch this incredible breakdown: July 22nd, the LK-99 arXiv day! On July 22nd, two papers describing “worlds first room temperature superconductor” were uploaded to arXiv: 2307.12008 - Sukbae Lee, Ji-Hoon Kim, Young-Wan Kwon (submitted by Kwon) and after 2 hours and 20 minutes another paper was uploaded 2307.12037 - Sukbae Lee, Jihoon Kim, Hyun-Tak Kim, Sungyeon Im, SooMin An, Keun Ho Auh (Submitted by Hyuntak Kim) You may notice that the first two authors on both papers are Sukbae Lee and Ji-Hoon Kim, and in fact LK stands for Lee and Kim and 99 in the LK-99 name stands for the year 1999 they have started research on this. You may also notice that YW Kwon who submitted the first paper, is not included on the second one, and in fact, is no longer part of the Quantum Energy Research Institute (Aka QCentre) where he was a CTO (he’s no longer listed on the site) If this shakes out, and SC is replicated, there’s definitely going to be a Netflix series on the events that led to YW Kwon to release the paper, after he was no longer affiliated with QCentre, with limited information so let’s try to connect the dots (a LOT of this connecting happened on the ground by Seo Sanghyeon and his friends, and translated by me. Their original coverage has a LOT of details and is available in Korean here Let’s go back to the 90s On the LinkedIn page of Ji-Hoon Kim (the page turned blank shortly before me writing this), JH Kim showed that he started working on this back in 1999, and they estimated they have a material that contained “very small amount of superconductivity” and together with Sukbae Lee, in 2018 they have established QCentre to complete the work of their Professor Emeritus of Chemistry at Korea University, the late Choi Dong-Sik (1943-2017) who apparently first proposed the LK-99 material (following the 1986 bonanza of the discovery of high temperature superconductors by IBM researchers). Fast forward to 2017, a wish expressed in a last will and testament starts everything again Professor Choi passed away, and in this will requested follow-up research on ISB theory and LK-99 and Quantum Energy Research Institute is now established by Lee and Kim (LK) and they continue their work on this material. In 2018, there’s a potential breakthrough, that could have been an accident that led to the discovery of the process behind LK-99? Here’s a snippet of Seo Sanghyeon explaining this: Kwon Young-Wan the ex-CTO Kwon is a Research Professor at Korea University & KIST, is the third author on the first arXiv paper, and the submitter, was previously the CTO, but at the time of the paper to arXiv he was not affiliated with QCentre for “some months” according to an interview with Lee. He uploads a paper, names only 3 authors (Lee, Kim and Himself) and then surprisingly presents LK-99 research at the MML2023 international conference held in Seoul a few days later, we haven’t yet found a video recording, however a few reports mention him asking for an interpreter, and talking about bringing samples without demonstration and proper equipment. Important to note, that Enter Hyun-Tak Kim H.T Kim is probably the most cited and well-known professor in academia among the folks involved. See his google scholar profile, with a D-index of 43 and has 261 publications and 11,263 citations. He’s a heavy hitter, and is the submitter and listed as the author of paper number 2 submitted to arXiv, 2 hours and 20 minutes after paper number 1 above. In the second paper, he’s listed as the third author (and the submitter to arXiv) and his contribution is acknowledged like so: An author, Hyun-Tak Kim (H. T. Kim),’s knowledge on mechanisms of both superconductivity and the metal-insulator (gap-nogap) transition highly contributed to writing the mechanism part. The knowledge was acquired over 20 years by processes of performing national projects including project [Grant 2017-0-00830] funded by Institute for Information and Communications Technology Promotion (IITP) in MSIT of Korea government in ETRI. H. T. Kim left ETRI on Nov. of 2022. In the first paper H.T. is not acknowledged, and is only mentioned in in reference no. 52 to his paper from 2021. Ok enough about the people Alex! Does the rock levitate? In January, QCentre youtube channel uploaded an unlisted video that showed magnetic properties of LK-99 and another video, with partial levitation is widely shared on social media. The partial levitation shown is attributed to the Meissner Effect and is a supposed proof of room temperature super conductivity. However, these two videos are inconclusive and are not enough for us to take QCentre claims at face value. The scientific community, having been stung by a recent incident surrounding a supposed room temp superconductor, where the evidence was apparently falsified (Dais et. al.) are not so easily swayed. Adding to that, the mess around the multiple papers, showing different theories, the lack of peer review, or independent replication, the surprised publication, and a rushed follow up publication, all makes people wonder, what is going on here? This doesn’t seem like a fabricated attempt. Summary of replication attempts so far (Sun, Jul 20) Given the importance of this discovery, and the “relative” triviality of replication, common enough materials, the process is not extremely complex (but kids, do not try this at home) so we can bet that “furnaces in solid-state materials labs around the world have been cooking yesterday and today to try to reproduce” [Science Magazine] We have reports from China that supplies of Led Apatite are running dry as many are trying to replicate quietly? Additional reports from India where Dr. VPS. Awana, the Chief scientist at CSIR-NPL and team are trying to replicate, with results expected as early as tomorrow (Monday, Jul 31) and has been emailing with Lee In addition to this, we’ve had Andrew McCalip from Varda space who has been live-tweetin, twitch streamin his “Meissner effect or bust” campaign to reproduce LK-99, while the world watches (Andrew joined the space as well) and provides ideas, materials and an outpour of support for this gung-ho, almost cowboy effort. We’ve also had folks from MIT who claimed that professors who want to remain anonymous, and went to MML2023 are also in contact with the team and are trying to test the material. Replication failure is … not a failure? Discussing the replication attempts with experts on stage, we all concluded that there are likely 2 ways for the world to know wether LK-99 is a superconductor. * Replication succeeds and scientists analyze the replicated sample * QCentre team provides a sample, and some very smart independent folks put it under a microscope, a magnetism analysis and a bunch of other measurements and confirm that it’s a superconductor at room temperature. While we wait for either of those, I encourage you to check out the resources, the space recording, and the list of folks I’ve collected to stay in the loop! Here’s a list of relevant links: * ChosunBiz Interview (Korean) And the list of folks who participated in the space, give them a follow: * Andrew McCalip (@andrewmccalip) * Danielle Fong (@DanielleFong) For your convenience, attached is an AI transcription of the space with speakers and timestamps (may be off by a few minutes) : [00:02:40] Alex Volkov (@altryne): Hello. Hello, everyone. There's a lot of you here, and I wanna welcome a shoot for up on stage while we wait for a few more guests, and then we can get started. Thank you so much for taking the time joining us. as you're as interested as all of us in this very exciting, very confusing, very potentially groundbreaking news. So I wanna introduce 2 folks up on stage 2 folks up on stage already, and bringing up another one just now. And hey, Andrew. Hey. [00:03:18] Alex Volkov (@altryne): Hey, How are you guys? [00:03:23] Ben (@BenShindel): Doing well. How are you? [00:03:27] Alex Volkov (@altryne): A little bit you know, the palms are a little bit sweaty. This is a insane turnout. Twitter is indeed a public space on because that we have. And, hopefully, spaces or two spaces, whatever they call it now, will hold. And I only invite Sam here to speak as well. Hey, Tobias. How are you? [00:03:51] Ate-a-Pi (@8teAPi): I'm good. I'm good. So good to good to, you know, hear from you guys in person, Alex. Thanks for putting the space together. [00:04:00] Alex Volkov (@altryne): Thirdly. Andrew, we're gonna introduce Andrew, but many folks who are here already follow you and and follow your work. How how's your evening going, Andrew? [00:04:12] Andrew McCalip (@andrewmccalip): Lee, this has been a wild ride. Thanks for putting all this together. It's gonna be great to get all the information in one place for the first time. This is my first time experiencing the full volume of the Internet, and just been a a lot of fun to see all the positivity around the progress. [00:04:29] Alex Volkov (@altryne): That's great. So I'll do my best that, you know, Mother think this. I will maybe preface this that I am not a scientist. Many of the terms that we'll hear today in the space I've heard for the first time a couple of days ago. What I am is a Twitter for many, many years, and I have collected a a list of folks who I I personally wanted to follow to kinda see the updates as they roll out, and we've seen many, many things roll out very quick. with a lot of confusion and different replication attempts from different places. And I just compiled the list for myself. I started following. [00:05:08] Alex Volkov (@altryne): 8 to buy had incredible incredible content diving into the the timeline. I found I I wanna introduce thank you. Am I saying this right? I think you need to hit the the mute button in a mute. If this is your first time talking on RESTASIS. let me know if you're able to do that. And if not, we'll try to solve this. And out as I was collecting folks, And I I started seeing that Andrew started doing their application attempts and even doing Twitch. [00:05:46] Seo Sanghyeon (@sanxiyn): Can you hear me? [00:05:47] Alex Volkov (@altryne): Can you hear me? We can hear you. Hey, Sam Kim. How are you? [00:05:57] Seo Sanghyeon (@sanxiyn): It it it's the noon in South Korea, and I'm fine. [00:06:01] Alex Volkov (@altryne): the afternoon. Right? [00:06:03] Seo Sanghyeon (@sanxiyn): It's 1. Yes. Yes. It's the 1 PM. [00:06:06] Alex Volkov (@altryne): Awesome. And so I was just doing an introduction maybe as you were telling up, you maybe not heard some of it. However, folks in the audience who followed this kind of thread and how we came to be here I have a a thread that I'll post on top here that has all the folks from the Twitter list that I forgot. And San Kyung and his his team is basically the reason for the space. Me and Nathan kind of found Sunqun. Am I saying Sunqun correctly? Is that is that the right way to say this? [00:06:41] Seo Sanghyeon (@sanxiyn): My name is. Your your, yeah, your pronunciation is not actually not. [00:06:48] Alex Volkov (@altryne): Okay. I'll I'll turn my best to put months at the at the right names. And so we both me and 8 to 5, a a 34 in Saint Kyung, who's in Seoul currently, and definitely speaks the language we don't speak, and so there's a lot of insight and translation. And so, yeah, I guess we'll will get started, so feel free to present yourself, and then talk a little bit about your last few days and how you came around getting in this topic. and then how kinda what you found so far. [00:07:28] Seo Sanghyeon (@sanxiyn): I I didn't really expect to to speak. [00:07:30] Alex Volkov (@altryne): That's okay. That's okay. [00:07:32] Seo Sanghyeon (@sanxiyn): That's put me put me on the spot. Yeah. [00:07:34] Alex Volkov (@altryne): I don't wanna put you on the spot, but give us maybe a brief summary. [00:07:44] Ate-a-Pi (@8teAPi): Maybe maybe do you do you want me to help Sanyon? [00:07:47] Seo Sanghyeon (@sanxiyn): Yes, please. Okay. You you have read my right top, so maybe maybe you can explain what's going on. [00:07:57] Ate-a-Pi (@8teAPi): Okay. So I'm I'm just gonna I'm just gonna just to preface everything, I I'm writing a work of fiction. So all of you guys are just participating in an experiment. So but I'm trying to keep everything to kinda, like, factual and trying to interpret what what is kind of happening on the ground. Right? Shyam is much more factual, and he he has actually been doing a primary source work. So he's been actually digging up the actual Korean language science papers. He's been sitting down with friends They've kinda, you know, summarized and kind of tried to understand what's going on. [00:08:36] Ate-a-Pi (@8teAPi): And he's really the one that's, you know, put together this that that the you know, the the the mentor, you know, whose name, I think, in some transliterations comes out to TS's chair, some Donsick He the mentor was basically in superconductors in this idea of this kind of 1 dimensional super and he had this theory. [00:09:00] Seo Sanghyeon (@sanxiyn): That so the name is che. che. Oh, sure. Yeah. Yeah. Yeah. He was a a professor in the Korean University's Department of Chemistry. [00:09:13] Ate-a-Pi (@8teAPi): Yeah. And and so he he had this idea, this theory, and he had graduate students. and one of those graduate students was Lee, and Lee kind of took up the mantle of this this theory. And then they, you know, tied up with who was an experiment list. [00:09:37] Ate-a-Pi (@8teAPi): And then they kinda discovered this trace this coast of a trace of a material in 1990 And at that point, what happens is having discovered this trace, their path kind of diverge this, and Kim, the experimentalist, goes on to do a masters, not in superconductors. So he does his masters in something else, and then he does the battery materials kind of PhD, and he graduates in 2008. [00:10:12] Ate-a-Pi (@8teAPi): while Lee continues on the superconductor path, does experimental any when he publishes his PhD. It's both a theory and synthesis of superconductors. And then he graduates, and then he he goes to work as a science adjunct professor, which we which we just found out. Like, a computer science adjunct professor, and he's there for about, you know, 4, 5 5 years. He doesn't publish. And and I'm guessing at this point, he kinda gets, like, you know, cashier out of out of academia completely, and he sets up a consulting firm, basically, Q Center. [00:10:50] Ate-a-Pi (@8teAPi): And they start taking on consulting work. And and then, again, the timeline is a little bit unclear on whether or not they continue to work on on the on on the product on what they discovered. And what happens then is in 2017, Chey Dongksik passes. [00:11:18] Ate-a-Pi (@8teAPi): And as he passes, he he gets his former students together, and he asked them to finish off what they started to find this superconducting material that they saw a ghost of a trace of in 1999. And he passes, and they have no money. basically. Song Young has done, again, primary source research, and, you know, the the office space is basically, like, like, a two story building, you know, somewhere in the you know, in in Seoul. It's a very modern kind of office. They don't have much money. [00:11:57] Ate-a-Pi (@8teAPi): My guess my guess is that they need Kim. because KIM is the experimentalist, and I'm guessing also that none of the theory works at this point. The only thing that they have to go on is that they actually did find something in 1999. And Kim, I'm guessing, is also quite practical because he didn't do he didn't pursue the superconductors for the PhD. Right? Because he's quite practical, he's like, dude, you get me money. I'll join you. You don't have money. I'm not joining you for your wild goose, Jason. Right? [00:12:36] Ate-a-Pi (@8teAPi): So Lee goes out and he recruits Kwan. And Kwan is kind of like you know, he's he's a US PhD. He has a research university, you know, position. recruit them, and they get funding. And I think I think Sam Young, you were you were saying that Kwon is the one on the, you know, National Science Foundation of Korea's like you know, list, like, grant. Right? I I think that's what you said. [00:13:08] Seo Sanghyeon (@sanxiyn): So the paper mentions the public grant from South Korea. called the National Resource Foundation, which is like National Science Foundation in United States. And Korn is listed as a primary invest mitigate our PI, if then. [00:13:25] Ate-a-Pi (@8teAPi): Right? [00:13:26] Alex Volkov (@altryne): Mhmm. [00:13:27] Ate-a-Pi (@8teAPi): Yeah. Yeah. That's right. Okay. So he he's the PI. So they recruit him as the PI, and Jade Kim, who is, you know, Lee's partner, basically leaves his very comfortable position as a research director in a hearing aid test. [00:13:44] Seo Sanghyeon (@sanxiyn): Yeah. [00:13:44] Alex Volkov (@altryne): Yeah. Yes. [00:13:45] Seo Sanghyeon (@sanxiyn): Yes. Yeah. Hearing aid Yeah. I Or the eye test there? Yeah. Yeah. For the ISER tech and in manufacture, the battery is specialized for the hearing aid. code. It is a medical device. They have a different standard from other batteries. And company a small business in South Korea, but seems competitive worldwide. [00:14:13] Alex Volkov (@altryne): So he leaves his let me let me -- Yeah. Go ahead. Just real quick and to give folks a quick summary. The main paper that we saw the explosion from that was published on July 22nd, so a week and and almost a day we're, like, almost 8 days into this. The three people that you you just said, besides the first professor, Choi or chair or Troy and or several places write it separately. So the the three people, SoftBank, Jihoon Kim, which is the LK in LK 99, right, Lee and Kim. And the third person you just mentioned is Young Wan, Kwan. Yes. [00:14:52] Alex Volkov (@altryne): Those are the the 3 authors on the paper that kind of was published on our side out of the blue. 8 days ago. Please continue. [00:15:03] Ate-a-Pi (@8teAPi): Right. And then so at this at this point, they're in 2017, And, you know, Lee goes out and does the fundraising. He recruits Kwan, who's the research professor, Kwon is basically he's on the paper. He he's he's the principal investigator on the grant, but he's still a professor at university. So he's basically, I'm guessing, like, a day a day in the, you know, in the office at Q Center, very modest place. I think the grand size is pretty small, and they get this ESR machine. [00:15:41] Ate-a-Pi (@8teAPi): And again, from what I can tell, the ESR machine only came knows how to use it. Because none of the other people are actually synthetic, you know, synthesis people. They're all like theory guys, Kuan is a physicist. And Kim himself, JH Kim himself, he's looking for something which you have to know what you're looking for, right? Because that's what he says in his LinkedIn. He's like, I'm looking for some if you don't know what you're looking for, then forget about it. Right? [00:16:19] Ate-a-Pi (@8teAPi): But he he knows what he's looking for, and they refine, they refine, and they refine, and he keeps doing experiments. He keeps refining the experiment, and he goes through, like, a 1000 iterations. And somehow, starting in 2018, somehow, By the middle of 2018, they find it. So that that's a surprising thing for me because they've I I I suspect they they've been working on it you know, before or, you know, Jay and Lee had a breakthrough on their theory, so they knew how to narrow the workspace down. But somehow in at the end of the day, Kim is the one grinding. [00:16:58] Ate-a-Pi (@8teAPi): Through that 1000 experiments, finally, to get, you know, a sample that works. [00:17:03] Seo Sanghyeon (@sanxiyn): And then they start by -- No. No. [00:17:05] Alex Volkov (@altryne): No. [00:17:05] Ate-a-Pi (@8teAPi): No. [00:17:05] Alex Volkov (@altryne): No. [00:17:05] Seo Sanghyeon (@sanxiyn): No. No. No. No. No. No? So so besides the two papers, there is a paper published in April returning query. And In their own words, they describe what what prompted their breakthrough in 2018. [00:17:27] Seo Sanghyeon (@sanxiyn): and it said that so so they are putting the material in a quartz tube And because they called it to best courts to cancel and Brooke, And the material left after the breaking of the glass was had the property they wanted. So so it was an accidental discovery. [00:18:02] Ate-a-Pi (@8teAPi): So can can you repeat that? Like, they what what happened? They put it in the quartz tube, and the quartz tube broke accidentally? [00:18:10] Seo Sanghyeon (@sanxiyn): Yes. [00:18:10] Alex Volkov (@altryne): Yes. Yes. [00:18:11] Seo Sanghyeon (@sanxiyn): I see. And and And that what's the breakthrough in 2018? I see. It's what I'm saying. [00:18:19] Alex Volkov (@altryne): Yeah. I just wanna confirm what I hear. The breaking of the course you led to the incidental discovery. This is this is the the breakthrough as it's written in the first paper in Korea? Yes. Yes. Okay. So I'll just call ASAP, I'll just give it back for some logistics. Folks, if you look up on on top of the space, there's a few tweets we're pinning. And as we go along, we're gonna add some information on top of this. The 3rd the third we pin from dystopian breaker has a link to the original kind of Korean paper. So please go ahead, Datapai. [00:18:54] Seo Sanghyeon (@sanxiyn): So so quick -- Okay. point. [00:18:56] Alex Volkov (@altryne): Yeah. [00:18:56] Ely Rabani (@radsci): Go ahead. Go ahead. This this could be important because, you know, as as soon as you expose it to the atmosphere, your getting hydration. And hydration, you know, might be harmful, might be helpful. From this, like, little account, it seems like it it it either didn't do anything or was helpful. But, like, no what temperature it was at when it broke, and and things like that could could actually be really pertinent. [00:19:30] Ate-a-Pi (@8teAPi): Yeah. So, absolutely, like so it's not they he does do the 1000 experiments, but the 1000 experiments, whether that gets him there or not, at one point in the experiment, the quartz tube breaks, that gets them there. They get lucky. Right? So they get they get lucky. And then after that, things proceed pretty quick They isolate they isolate it, and then they they get the crystallization. They start working on the papers. They start on the patents, and they start also trying to figure out the chemical vapor deposition process. They seem to have made some way some headway on the chemical vapor deposition process. [00:20:06] Ate-a-Pi (@8teAPi): And then, you know, sometime around September 2021, something start happening. Quant takes a position, sabbatical at, I think, Seoul University at that point. I'm not sure whether that means he's putting more time in the office or not. And then that fast forwards to yeah. Go go ahead, Sunggham. [00:20:33] Seo Sanghyeon (@sanxiyn): No. No. [00:20:33] Alex Volkov (@altryne): No. [00:20:33] Ate-a-Pi (@8teAPi): You go ahead. Okay. So that fast forward about March 2023 when basically the international patent has been filed. And Kuan leaves the team at this time. I'm not sure when Kim comes on board. That's not very to me at what point Yum Tuck comes on board. [00:20:57] Ate-a-Pi (@8teAPi): So I'm guessing it's after the nature, the nature paper gets dinged in 2020, And and and, you know, the the other thing that strikes me also is that every single person on the team is very aware of every single hoax in superconductors to date. Right? They they they all know the space well, They've seen every single hoax before. They know they know what the hoaxes look like. They know what to look for. They know what diamagmatism is. So I I I don't think yeah. [00:21:29] Seo Sanghyeon (@sanxiyn): Go ahead. So the date is So the day before the yesterday, Andrew McCully posted on his Twitter the translation of the Korean paper at Doctor Lloyd. Is that correct? And can can you so so how did you translate and can Can you say something about it? [00:21:59] Alex Volkov (@altryne): Andrew, I think he's Frank to you. So I can just ring to you. You posted a translated paper also. Right? [00:22:08] Andrew McCalip (@andrewmccalip): Yes. Now that was just a machine translation from Google. That was just a very cursory translation. [00:22:19] Seo Sanghyeon (@sanxiyn): Okay. [00:22:19] Ate-a-Pi (@8teAPi): So in basically, quantity is team in March, and then you have the kind of papers being released, you know, haphazardly. The next the next point that of them is that they had started releasing the papers app as early, like, late last week. [00:22:42] Alex Volkov (@altryne): And and then and then we have -- And by the way, I think it's it's important to highlight by Kwan, the guy who's no longer affiliated with with QCenter. Like, this this sole endeavor a business venture that's funded for for this for this purpose. Kwan is no longer affiliated with that. We've seen Sankyo posted an interview in Korea from Friday where I think both of the and Kim say that Kwan, the guy who published the first paper, is no longer affiliated. [00:23:12] Alex Volkov (@altryne): there were some speculation as to maybe the limit of three people on the paper is the limit of the Nobel Prize or 2 or 3 authors. I don't have this confirmed, but this is speculation going around. And it's important to note like, both of them say that the paper was not ready when it was released, and it was released by Juan, the guy who left the first paper. 2 hours later, 2 than 20 minutes later, another paper gets released in the in the same archive with, I wouldn't say, 5 authors. not including Kwan. Right? [00:23:48] Ate-a-Pi (@8teAPi): So Lee -- Yeah. And -- The user the the user name is TumTuk team, the the college professor from, you know, Virginia is the username who who pushes the r archive paper at that Yeah. [00:24:04] Seo Sanghyeon (@sanxiyn): Chantakim is a big name with the 18 days of 45, and If you look at the paper, there is an error message in Korean saying that Bloomberg could not be found. It is a neutral error message when you did the some of the typesetting wrong. [00:24:27] Seo Sanghyeon (@sanxiyn): And You just don't probably see the room temperature, sugar conductor paper with the error deaths that had to bookmark cannot be found if you are following if you are in not in emergency. [00:24:52] Alex Volkov (@altryne): So so it does feel to us at least from the summary so far that the paper that Quang released has different information than than the second paper, and the second paper feels like it was released in the Harry and included more people that currently work at Q Center, including Hyundai Kim. And Sonja, owner, you this question. You mentioned his h h score or something score. Can can you explain the importance of that score for him talking? [00:25:20] Seo Sanghyeon (@sanxiyn): creates someone else to the explanation. [00:25:24] Ate-a-Pi (@8teAPi): Okay. So so the h score is, you know, because we have a web web savvy audience here. It's kind of like a page rank for, you know, researchers. It shows you how influential how influential the researcher was, and so a higher score means that more people have been citing your paper. [00:25:45] Ben (@BenShindel): Go ahead, Ben. Yeah. More precisely. So, like, an h index of, say, 40 means you have 40 papers that each have 40 citations or more. That's a little tricky to understand. So, like, if I get another paper that has only 30 citations, it won't affect my h index at all. I have to get a 41st paper that has 41 citations to to to make it rise. [00:26:07] Alex Volkov (@altryne): So I think it's it's safe to say HUNTAKIM, the guy who submitted the second paper, potentially haphazardly. Correct? Like, we're we're we're saying there's 2 hours after the first one. So likely prompted by these events is a well well sighted very well sighted scientist with a very high kind of confidence score. It's not like a random person of the street that decide that there's now a superconductor of room temperature and, you know, verified it. [00:26:41] Seo Sanghyeon (@sanxiyn): Okay. Sorry for being side tracked, but I just checked the the motion related to Korean paper or not to talk through it by Andrew. And on the page 5, we clearly said that the quartz tube was destroyed due to internal pressure during rapid cooling of reaction and etcetera. So I think, in fact, nobody really read ready carefully. It is it is just there about the quartz tube once destroyed. [00:27:19] Ate-a-Pi (@8teAPi): Yeah. So I think I think it's yeah. Definitely, like, probably the the rest of us are are are not very close readers. of of that paper. [00:27:29] Seo Sanghyeon (@sanxiyn): So so We can we can continue on after the upload to the archive. [00:27:42] Ate-a-Pi (@8teAPi): Indeed. So okay. So they they they it goes into our our archive, and then all of the events of the last week happen you know, I don't think any of us expected any of the events to happen. So we've all just been kind of, like, following along and seeing what happens next. I had no idea that there was a metallics conference in South Korea, and I I definitely had, like, no idea that you know, one of the authors would show up there, and it gets posted on Twitter. And so and then and then Seung Young points it out on the FM Korea Football message board. [00:28:20] Ate-a-Pi (@8teAPi): And so we translate, you know, what the audience reaction was in in in a bad translation to get -- So -- -- whatever message was across. [00:28:30] Alex Volkov (@altryne): -- mind let me interject here because this is around the that I found out about this. Alex, frozen coffee. Alex, I forgot his nickname. We invited him here. He posted a a very long Twitter thread that got the attention of the algorithm and then boosted of this room template ambin pressure, superconductor paper from Korea. I think he only started talking about the first paper, and then after the second paper also came out. And I think at this point, or somewhere around there. Andrew, you found out about this. What what did you first hear about, you know, Twitter drama around LK 90 Right? [00:29:08] Alex Volkov (@altryne): And, Andrew, feel free to at least produce you know, introduce yourself officially and BARDA and how you're interacting with this. [00:29:16] Andrew McCalip (@andrewmccalip): Yeah. So I was just cruising the Internet at night, and this came across. I think my my Twitter feed And so I I'm incredibly curious. This is something that has been a bit of a a hobby for me. And so I was always interested in superconductors, so it it caught my attention. I'm a mechanical engineer. So full disclosure. I am not a subject matter expert. I am simply an aerospace engineer that has a lot of curiosity and some assets at his disposal. [00:29:50] Andrew McCalip (@andrewmccalip): And so reading this paper, it it struck me just the simplicity of of the process. And so I realized that I probably had the ability to replicate with full fidelity, the process that was described in the paper. And so that within about 30 minutes, I I realized I should simply start down this road that Twitter was already picking up at the time. [00:30:21] Andrew McCalip (@andrewmccalip): There's some conversations going back and forth and the it was the classic scenario where on every superconductor discussion, there is the same conversation that happens over and over again. And this synthesis appeared so simple that it seemed that the most expedient thing was to simply test it physically. And so my my work is very receptive of of after hours projects. I'm I'm known as the the guy that has really aggressive hobbies, let's say. [00:30:57] Andrew McCalip (@andrewmccalip): And so I'm always in the back doing something interesting with materials or automation. So within 30 minutes of reading the paper, I had ticked off orders to various chemical suppliers. I've reached out to overseas vendors. to try to procure a couple of the the elements. And so it was just kind of an offhand comment that I made on Twitter and and then the ball really started rolling, and I realized that everyone wanted to see this this made. [00:31:32] Andrew McCalip (@andrewmccalip): And so it was just supposed to be a a a fun little project, but I was really overwhelmed by the the response. Everyone wanted to to see this done. I think there's this incredible curiosity, there's this incredible drive. People wanna see, like, incredible things happen for the the the human race. And so something if this magnitude pops up, everyone's motivated to drop everything and investigate. And I think that's where we're at. [00:32:08] Alex Volkov (@altryne): And I think you met the algorithm at the right place where folks were excited about the future and think this could bring a lot of changes around the future, and you started saying, hey. You know? Here's a here's a direct approach. Let's try to replicate this. And I I wanna just highlight the fact the the materials involved in creating this. And the process, some folks say and please talk about this. Some folks say that has been an attempt at a hoax, it wouldn't be as simple. They wouldn't have released a simple instruction manual kind of quote, unquote simple that many labs around the work they replicate given the materials and and the right equipment. Right? [00:32:48] Ely Rabani (@radsci): So -- Yeah. [00:32:48] Alex Volkov (@altryne): So -- -- straightforwardness of this potentially shows some stuff. [00:32:51] Ely Rabani (@radsci): So this this is a good time for for a PSA. I mean, I know that that Andrew is well aware of this, and and and many of peep of the people who've been following it. But in case anybody who's listening isn't. The these compounds in vapor form at any rate are are highly talked music, and you you have to know lab safety. If you're gonna start trying to experiment with them, you need things like, a glove box and, you know, all kinds of PPE, a fume hood, everything else. Taking risks with this kind of thing is just really not worth it. [00:33:31] Alex Volkov (@altryne): I I I can't stress that. Absolutely. Don't try this at home. [00:33:36] Andrew McCalip (@andrewmccalip): kids definitely. Yeah. Absolutely. There's a lot of chatter in the beginning in the first couple hours about this can be replicated in a garage And, you know, I thought it was interesting. I thought maybe we've got the opportunity to to do it safely. we've got all the right equipment. We've got, you know, the the 1,000,000 of dollars of equipment that support our spacecraft business. that allow us to do some of these things safely. And so I thought Twitter wants to live vicariously through somebody why not do this? [00:34:12] Andrew McCalip (@andrewmccalip): I ended up being in sort of an interesting middle ground because I'm not in academia. I'm also not trying to commercialize any part of this tech. really just doing it for fun because it's incredibly interesting. So I've got no skin in the game except for making this work in a transparent manner. and then getting the materials into the hands of the experts. [00:34:34] Andrew McCalip (@andrewmccalip): So I thought if we can leverage some of our equipment and some of our, you know, very smart people that we have, to speed this timeline up, I didn't see anybody in the United States being vocal about trying to do replication there are so many stories coming out of other parts of the world that all the labs, there must be thousands of furnaces burning right now trying to replicate this. But I wanted to get material into the hands of some local experts in California. [00:35:09] Andrew McCalip (@andrewmccalip): And so that's really our our goal is, you know, can we can we sort of be the face of of the Internet do this experiment in a safe manner and then help advance the science and be sort of a forcing function to to doing this replication. [00:35:27] Alex Volkov (@altryne): So, Andrew, just before just a a small pause before you continue, I want to ask the other, Andrew, here. The Andrew code, if if you're able to unmute and and and talk us if you're available about the potential reasons why all of Twitter jumped on this. Andrew Kot, you had a thread on room temperature superconductors. About 2 weeks before this, like, almost a permanent is kind of a threat. And could you give us some summary first of all, feel free to introduce yourself, but also some summary of what this means if this replicates, what this means for the world. [00:36:07] Alex Volkov (@altryne): Applications, you know, give us, like, some excitement of what happens if this is an actual ambient pressure in room temperature superconductor? Andrew? Does not look like Andrew is Oh, hey. [00:36:33] Andrew Cote (@Andercot): Sorry. My my audio cut out for a second. I I missed the prompt. Oh, here you are. Let you only -- Sure. Yeah. Thanks. Thanks very much. [00:36:44] Alex Volkov (@altryne): So so folks so so I I explained to folks your thread about MBN, you know, pressure room temperature superconductors that you've offered, what, 2 weeks before the paper came out. And then suddenly, this dropped. And I wanted you to highlight some of the potential applications of superconductors and give us some of the highlights of what happens in this replicating. This is an actual, you know, real thing. [00:37:08] Andrew Cote (@Andercot): Yeah. Sure. So it's kind of a funny thing. Yeah. I put that thread out there 7 weeks before this story broke. You know, just I have worked with this kind of stuff in in a few different areas now, so it's very, you know, superconducting radio frequency cavities are standard technology in accelerator physics to fill these to work in. [00:37:31] Andrew Cote (@Andercot): Like, my first job in physics was actually in a condensed matter lab using a a scanning tunneling microscope to look at, you know, electronic structures of potential high temperature superconductors So this has always been sort of like a holy grail of material science, like sort of a holy grail of applied physics. It's one of these properties it's one of these materials where the bulk properties come from its quantum mechanical behavior. And and, you know, when quantum mechanics and its effects escape the realm of the very tiny, it can really manifest as as magical phenomenon at our scale in the world of the kind of the bulk matter or the big stuff. [00:38:10] Andrew Cote (@Andercot): So, you know, superconductors are used currently today, You know, it's it's they've reached engineering applicability through decades of continuous refinements and improvements. And and some of the biggest things to think about in what lets these things get used in industrial applications is their ability to superconducts at higher and higher temperatures And, also most also importantly, is to operate at higher and higher background magnetic field strengths. And so the way to think about this is that a superconductor, it's allowing current to move through it with zero resistance, but it also perfectly spells magnetic fields. [00:38:48] Andrew Cote (@Andercot): And there's an operating point of these materials where it's basically the current density and the temperature and the magnetic field kind of put the bounds or the performance envelope on the material. So some conductors can carry tons of current, but they can't exist in a very high field. And so, you know, those are hard to make as useful. You can use them for carrying, like, electricity, which is awesome, but often what you really wanna do is generate very strong magnetic fields. So I think maybe the most familiar to the most people here would be, like an MRI machine. Right? [00:39:27] Andrew Cote (@Andercot): Magnetic resonance imaging. So the idea there is you're generating very high strength field, and magnetic fields are measured in Tesla, for example. So just for just for context, you know, 3 Tesla is a is a pretty strong field, and that's what is about the strength using an MRI. So, you know, MRIs use these cryogenically cooled magnets, or or they're not I don't think cryogenically cooled. They're actually often just copper, but they do have cooling. But they generate this high strength field, and then, you know, it kind of sets all these little protons in your body spinning and dancing in a little, you know, kind of radiating energy. [00:40:03] Andrew Cote (@Andercot): And then you have a pickup coil, which is like an antenna, and the antenna is trying to pick up that energy and kinda reconstruct what's going on in your body. And this is how we can get, like, a really high detailed, high fidelity, three-dimensional image of what's going on inside someone without any invasive surgery. So it's, like, you know, MRIs are a real kind of amazing breakthrough in medical imaging. Superconductors if they could work without cryogenics would really simplify and make cheaper and more available, high resolution, high fidelity, three d images of people's bodies. [00:40:35] Andrew Cote (@Andercot): not just for making the magnetic fields, but also for picking up the signal emitted by the protons that get put into motion by the field in the first place. So it's kind of, like, one sort of off the shelf example. I think another one that's kind of under the radar, we don't think about it's not just in carrying electricity without resistance, which is useful for long range, like energy transmission, that kind of stuff. But if you look at the national grid, I mean, only 5, 7 percent of energy total, which is still significant, but it's, you know, single digit percentage ends up, you know, burning as weight You're suddenly muffled. [00:41:11] Alex Volkov (@altryne): I don't think yeah. You're suddenly a voice like your -- Oh, better. [00:41:18] Andrew Cote (@Andercot): Now it's better. Okay. Sorry about that. Yeah. So just gonna say so, you know, National Grid Scale Energy Production. Right? So trans transmitting the energy to its endpoint consumption, there's a bit of waste heat along the way. But what's what's also important to think about is how that energy is produced. It's produced also using high strength magnetic fields. And I was looking into this. There's a a experiment where these guys used sort of more modern high temperature superconducting tape to, you know, retrofit a large DC generator then it had, like, a 36 percent power improvement, right, which is pretty substantial. That's that's a that's a serious win. [00:41:58] Andrew Cote (@Andercot): Yeah. So there's there's, you know, sort of thousands of places this stuff could be used that would really just, like you know, it would either greatly improve the performance efficiency, reduce the cost, increase the accessibility of what we think of as, like, high technology like MRIs or particle accelerators. But it would also just decrease the cost of basic things like electricity generation and distribution And that's just the beginning. Right? So, you know, this kind of stuff there's a really good analogy here actually with the transistor, you know, for for years, scientists, then electrical engineers and physicists, they had this idea of a transistor. Right? [00:42:35] Andrew Cote (@Andercot): If only we could have some kind of simple, reliable, current model supplier. We could design all these wonderful things. We could design all these different kinds of logic functions and so forth. And so there was this search for the transistor people were searching for something that could do that, and they had anticipated all the places it could be used ahead of time. And it wasn't until at Bell labs, you know, a very kind of funny crossover here. One of the guys that's on the patent for the transistor is John Bardine. and John Bardeen's actually the only guy to win 2 Nobel Prizes. 1 was for the transistor. The other was for the theory of superconductivity, right, which is Barting Cooper Schiffer Theory, BCS. [00:43:14] Andrew Cote (@Andercot): So, again, it's one of it's one of those things where, you know, physicists, scientists, engineers kinda thought about this for a long time, realize this be amazing. And there's been this, you know, really complicated random walk through the configuration space of possible materials, right, which is so high dimensional. There's so many things you can construct. So I think it's I'm very optimistic about the field in general. I think one thing to think about with this particular result there's so much artisanal craft and and mastery that goes into producing these materials in a reliable, consistent way You know, science people don't often recognize. It's a lot of art involved too. Right? [00:43:52] Andrew Cote (@Andercot): Like like, things that are reduced to expert practice us and know how. And so I'd I'd just be cautious on, you know, jumping to conclusions either on this particular result, if it's if it's valid right now. But, also, if some labs can't fail to reproduce it, it doesn't actually rule it out entirely. I I think there's scientists that have traveled to Korea to work with the original authors. I look closely at that. You know, I'd also you know, I my internal odds are kind of like a 1 in 6 chance, this pans out, and it and it could be big. [00:44:21] Andrew Cote (@Andercot): But that doesn't mean that it's the end of the search or the end of the race, and I'm and I'm also optimistic that Getting people to understand what the massive long term and large scale social benefits of this kind of discovery could be could help direct a lot more basic science research towards this field. You know, I think we spend a lot of things on, like, how to make smartphone cameras better and not a lot of things on and not as much as we could spend on things like high temperature superconductors. And this is a final example. [00:44:48] Andrew Cote (@Andercot): I mean, so right now, you know, I work as a accelerator engineer, accelerator is a type of magnetic confinement fusion reactor The reason the company I work for can't exist, and and the reason there is this current burn and boom in nuclear fusion, is because we've engineered these high temperature superconductors to work in higher and higher magnetic fields, at at higher and higher temperatures. And and the big economic breakthrough there came when we can have these superconductors that can work at liquid nitrogen temperatures, right, which is 77 kelvin. And it's a lot cheaper to make liquid nitrogen and run that kind of cryogenics than it like liquid helium at, like, 4 Kelvin. [00:45:24] Andrew Cote (@Andercot): So, you know, we're already reaping some of the benefits of this sort of tech stack maturing over time. And I think really just getting started in terms of, like, the hunt for promising materials. I mean, I'm hoping this results in positive publicity and more effort, more energy, put into the field. I think if this doesn't pan out as the thing, you know, don't give up hope. Right? I mean, this is a long term game. Science sees by starts and stops. There's no fundamental physics here that's impossible. Right? There's no physical principle that says this can't work. Right? This isn't like a a momentumless or massless propulsion drive like the EM drive. [00:46:04] Andrew Cote (@Andercot): isn't, like, superluminal neutrinos. Right? Those things kind of break laws of physics. This is very much in the realm of, yeah, physically possible. seems seems very you know, in my mind, seems likely there could be something out there given the complexity of state space of electronic structures and given how you know, how large that space of exploration can be. And, yeah, so I think I'm just kind of you know, this is a great time to be interested in material science to appreciate basic science research and educating ourselves on on how good the future can be. You know, I think there's a lot of narratives right now in society and cultural in general. that kinda say, like, you know, you know, we we can't solve our way out of our biggest problems today. Right? [00:46:43] Andrew Cote (@Andercot): And and I'm very much on the other side of that debate. I think we can. I think it's through efforts like this. I think it's through people like Andrew at Varda that are willing to do stuff in their backyard or their garage or their fact or their their work workplace on their extra time. You know? I mean, this is the kind of this is the the let's build mentality. Right? And so I think we can build our way out of the world's greatest problems, and I its fundamental scientific advances like this discovery could be that that kind of paved the way out of there too. So, yeah, overall, very optimistic. [00:47:11] Andrew McCalip (@andrewmccalip): Andrew? That that's incredibly well said. That is an incredibly well balanced viewpoint. So how would you advise people to absorb the the next week of the new cycle? I mean, we're very much on a you know, we're we're back dead. We're back type of hype cycle. So how do you advise people to think about the results that they're seeing knowing that this is a a very difficult thing to replicate when it just because it a negative result is shown in a lab that doesn't mean it's not physically possible. [00:47:49] Andrew McCalip (@andrewmccalip): It's very difficult to prove the negative here. So tell us how we should absorb the new cycle coming up in the next few days. [00:47:59] Ate-a-Pi (@8teAPi): So I I I I I I might I might say something about that. I think I think this is basically tacit knowledge transfer, and you Kim Kim seems to have been this kind of, like, artisanal, like, you know, experiment list. So you need people to actually sit there in the lab with this guy, and he needs to demonstrate to them. And they need to pick up and and there might be things that he does, which he didn't write down. That that's the like, my my take on it given that He is the experiment list. He's the synthesis on on the team. [00:48:38] Ate-a-Pi (@8teAPi): Given that the team seems to have been only, like, 5 or 6 people, is that this guy is the maybe the only person in the world as of, like, you know, 18 months ago. I'm guessing that, you know, he managed to transfer some of that to the JungTux team. So I'm guessing that at at least one more one more team on on earth has this now. And I'm guessing that this knowledge transfer is now happening to a couple more people. So so you need to see this progress maybe 2 or 3 cycles for, like, a bunch of other people to have learned the skill, and then that's when that's when things get interesting. [00:49:14] Seo Sanghyeon (@sanxiyn): I mean, you don't really need to replicate to to verify this. There, the the team can just the team has the working samples. they can adjust the samples to the laps around the world. Hey, the rest of the episode is for paid subscribers to thursdai. I encourage you to subscribe or upgrade your subscription to access it, there’s almost 2 more hours of in depth conversation, stitching of facts, experts on material science, physics, electrical engineering and MIT folks chiming in. It’s really a great space, around 25K folks have listened to it on twitter so far. | |||
04 Aug 2023 | ThursdAI Aug 3 - OpenAI, Qwen 7B beats LLaMa, Orca is replicated, and more AI news | 00:26:03 | |
Hi, today’s episode is published on a Friday, it’s been a busy week with at least 4 twitter spaces, countless DMs and research! OpenAI announces UX updates * Example prompts: No more staring at a blank page! * Suggested replies: ChatGPT automatically synthesizes follow up questions. Then you just click a button * GPT-4 by default: When starting a new chat as a Plus user, ChatGPT will remember your previously selected model! * 4. Uploading multiple files is now supported in the Code Interpreter beta for all Plus users. * 5. Stay logged in: You’ll no longer be logged out every 2 weeks and if you do, we have a sweet new welcome page! * 6. Keyboard shortcuts: Work faster with shortcuts, Try ⌘ (Ctrl) + / to see the complete list. ThursdAI - I stay up to date so you don’t have to Alibaba releases Qwen7b * Trained with high-quality pretraining data. Qwen-7B pretrained on a self-constructed large-scale high-quality dataset of over 2.2 trillion tokens. The dataset includes plain texts and codes, and it covers a wide range of domains, including general domain data and professional domain data. * Strong performance. In comparison with the models of the similar model size, outperforms the competitors on a series of benchmark datasets, which evaluates natural language understanding, mathematics, coding, etc. * Better support of languages. New tokenizer, based on a large vocabulary of over 150K tokens, is a more efficient one compared with other tokenizers. It is friendly to many languages, and it is helpful for users to further finetune Qwen-7B for the extension of understanding a certain language. * Support of 8K Context Length. Both Qwen-7B and Qwen-7B-Chat support the context length of 8K, which allows inputs with long contexts. * Support of Plugins. Qwen-7B-Chat is trained with plugin-related alignment data, and thus it is capable of using tools, including APIs, models, databases, etc., and it is capable of playing as an agent. This is an impressive jump in open source capabilities, less than a month after LLaMa 2 release! GTE-large a new embedding model outperforms OPENAI ada-002 If you’ve used any “chat with your documents” app or built one, or have used a vector database, chances are, you’ve used openAI ada-002, it’s the most common embedding model (that turns text into embeddings for vector similarity search) This model is ousted by an OpenSource (nee. free) one called GTE-large with improvements on top of ada across most parameters! OpenOrca 2 preview Our friends from AlignmentLab including Teknium and LDJ have discussed the release of OpenOrca 2! If you’re interested in the type of finetuning things these guys do, we had a special interview w/ NousResearch on the pod a few weeks ago OpenOrca tops the charts for the best performing 13B model 👏 Hyper-write releases a personal assistant You know how much we love agents in ThursdAI, and we’re waiting for this field to materialize and I personally am waiting for an agent to summarize the whole links and screenshots for this summary, and… we’re not there yet! But we’re coming close, and our friends from HyperWrite have released their browser controlling agent on ThursdAI. Talk about a full day of releases! I absolutely love the marketing trick they used where one of the examples of how it works, is “upvote us on producthunt” and it actually did work for me, and found out that I already upvoted Superconductor continues I was absolutely worried that I won’t make it to this thursdAI or won’t know what to talk about because, well, I’ve become a sort of host and information hub and a interviewer of folks about LK-99. Many people around the world seem interested in it’s properties, replication attempts and to understand this new and exciting thing. We talked about this briefly, but if interests you (and I think it absolutely should) please listen to the below recording. ThursdAI - See ya next week, don’t forget to subscribe and if you are already subscribed, and get value, upgrading will help me buy the proper equipment to make this a professional endeavor and pay for the AI tools! 🫡 This is a public episode. If you'd like to discuss this with other subscribers or get access to bonus episodes, visit sub.thursdai.news/subscribe | |||
10 Aug 2023 | ThursdAI Aug 10 - Deepfakes get real, OSS Embeddings heating up, Wizard 70B tops tops the charts and more! | 00:15:42 | |
Hey everyone, welcome to yet another ThursdAI update! As always, I’m your host, Alex Volkov, and every week, ThursdAI is a twitter space that has a panel of experts, guests and AI enthusiasts who join to get up to date with the incredible fast pace of AI updates, learn together and listen to subject matter experts on several of the topics. Pssst, this podcast is now available on Apple, Spotify and everywhere using RSS and a new, long form, raw and uncut, full spaces recording podcast is coming soon! ThursdAI - Is supported by readers, and I promised my wife I’d ask, if you find this valuable, why not upgrade your subscription so I can keep this going? Get better equipment and produce higher quality shows? I started noticing that our updates spaces are split into several themes, and figured to start separating the updates to these themes as well, do let me know if the comments if you have feedback or preference or specific things to focus on. LLMs (Open Source & Proprietary) This section will include updates pertaining to Large Language Models, proprietary (GPT4 & Claude) and open source ones, APIs and prompting. Claude 1.2 instant in Anthropic API (source) Anthropic has released a new version of their Claude Instant, a very very fast model of Claude, with 100K, a very capable model that’s now better at code task, and most of all, very very fast! Anthropic is also better at giving access to these models, so if you’ve waited in their waitlist for a while, and still don’t have access, DM me (@altryne) and I’ll try to get you API access as a member of ThursdAI community. WizardLM-70B V1.0 tops OSS charts (source) WizardLM 70B from WizardLM is now the top dog in open source AI, featuring the same License as LLaMa and much much better code performance than base LLaMa 2, it’s now the top performing code model that’s also does other LLMy things. Per friend of the pod, and Finetuner extraordinaire Teknium, this is the best HumanEval (coding benchmark) we’ve seen in a LLaMa based open source model 🔥 Also from Teknium btw, a recent evaluation of the Alibaba Qwen 7B model we talked about last ThursdAI, by Teknium, actually showed that LLaMa 7B is a bit better, however, Qwen should also be evaluated on tool selection and agent use, and we’re waiting for those metrics to surface and will update! Embeddings Embeddings Embeddings It seems that in OpenSource embeddings, we’re now getting state of the art open source models (read: require no internet access) every week! In just the last few months: - Microsoft open-sourced E5 - Alibaba open-sourced General Text Embeddings - BAAI open-sourced FlagEmbedding - Jina open-sourced Jina Embeddings And now, we have a new metric MTEB and a new leaderboard from hugging face (who else?) to always know which model is currently leading the pack. With a new winner from this week! BGE (large, base and small (just 140MB) ) Embedding models are very important for many AI applications, RAG (retrieval augmented generation) products, semantic search and vector DBs, and the faster, smaller and more offline they are, the better the whole field of AI tools we’re going to get, including, much more capable, and offline agents. 🔥 Worth noting that text-ada-002, the OpenAI embedding API is now ranked 13 on the above MTEB leaderboard! Open Code Interpreter 👏 While we’re on the agents topic, we had the privilege to chat with a new friend of the pod, Shroominic who’s told us about his open source project, called codeinterpreter-api which is an open source implementation of code interpreter. We had a great conversation about this effort, the community push, the ability of this open version to install new packages, access the web, run offline and have multiple open source LLMs that run it, and we expect to hear more as this project develops! If you’re not familiar with OpenAI Code Interpreter, we’ve talked about it at length when it just came out here and it’s probably the best “AI Agent” that many folks have access to right now. Deepfakes are upon us! I want to show you this video and you tell me if you saw this not in an AI newsletter, would you have been able to tell it’s AI generated. This video was generated automatically, when I applied to the waitlist by HeyGen and then I registered again and tried to get AI Joshua to generate an ultra realistic ThursdAI promo vid haha. I’ve played with many tools for AI video generation and never saw anything come close to this quality, and can’t wait for this to launch! While this is a significant update for many folks in terms of how well deepfakes can look (and it is! Just look at it, reflections, HQ, lip movement is perfect, just incredible) this isn’t the only progress data point in this space. Play.ht announced version 2.0 which sounds incredibly natural, increased model size 10x and dataset to more than 1 million hours of speech across multiple languages, accents, and speaking styles and emotions and claims to have sub 1s latency and fake your voice with a sample of only… 3 seconds! 🤯 So have you and your loved ones chosen a code word to authenticate over the phone? Or switched to a verifiable communication style? While those of us with multiple accents don’t yet have to worry, everyone should stop believing any video or voice sample from now on, it’s just inevitable that all of that will be deepfaked and we should start coming up with ways to authenticate content. If you made it this far, and any of the above was new/important to you, why not support this pod/newsletter/community? If you’d like to sponsor us more directly, please ping me at altryne [at] gmail.com , I’m also open to consulting, and if you’re a great company, Developer Relations positions :) Finally, we’ve talked for a whopping 2 hours on the spaces, and that whole conversation can be heard on our Zealous page which has transcripts, AudioGrams of key moments, and space summarizations! And the Long form space recordings can be added to your podcatcher separately if you’d prefer the “ThursdAI raw feed” by using this RSS link, and will come as it’s own podcast very soon! Thanks to our friends at Zealous Thank you, Alex Volkov. Host ThursdAI - Recaps of the most high signal AI weekly spaces CEO @ Targum.video AI Consultant with free slots (Lets Talk) This is a public episode. If you'd like to discuss this with other subscribers or get access to bonus episodes, visit sub.thursdai.news/subscribe | |||
17 Aug 2023 | ThursdAI Aug 17 - AI Vision, Platypus tops the charts, AI Towns, Self Alignment 📰 and a special interview with Platypus authors! | 00:16:54 | |
Hey everyone, this is Alex Volkov, the host of ThursdAI, welcome to yet another recap of yet another incredibly fast past faced week. I want to start with a ThursdAI update, we now have a new website http://thursdai.news and a new dedicated twitter account @thursdai_pod as we build up the ThursdAI community and brand a bit more. As always, a reminder that ThursdAI is a weekly X space, newsletter and 2! podcasts, short form (Apple, Spotify) and the unedited long-form spaces recordings (RSS, Zealous page) for those who’d like the nitty gritty details (and are on a long drive somewhere). Open Source LLMs & Finetuning Honestly, the speed with which LLaMa 2 finetunes are taking over state of the art performance is staggering. We literally talk about a new model every week that’s topping the LLM Benchmark leaderboard, and it hasn’t even been a month since LLaMa 2 release day 🤯 (July 18 for those who are counting) Enter Platypus 70B (🔗) Platypus 70B-instruct is currently the highest ranked open source LLM and other Platypus versions We’ve had the great pleasure to chat with new friends of the pod Arielle Lee and Cole Hunter (and long time friend of the pod Nataniel Ruiz, co-author of DreamBooth, and StyleDrop which we’ve covered before) about this incredible effort to finetune LLaMa 2, the open dataset they curated and released as part of this effort and how quick and easy it is possible to train (a smaller 13B) version of Platypus (just 5 hours on a single A100 GPU ~= 6$ on Lambda 🤯) We had a great interview with Garage BAIND the authors of Platypus and we’ll be posting that on a special Sunday episode of ThursdAI so make sure you are subscribed to receive that when it drops. Open Orca + Platypus = OrctyPus 13B? (🔗) We’ve told you about OpenOrca just last week, from our friends at @alignment_lab and not only is Platypus is the best performing 70B model, the open source community comes through with an incredible merge and collaborating to bring you the best 13B model, which is a merge between OpenOrca and Platypus. This 13B model is now very close to the original LLaMa 70B in many of the metrics. LESS THAN A MONTH after the initial open source. It’s quite a remarkable achievement and we salute the whole community for this immense effort 👏 Also, accelerate! 🔥 Join the skunksworks Speaking of fast moving things, In addition to the above interview, we had a great conversation with folks from so called SkunksWorks OS discord, Namely Far El, Prateek Yadav, Alpay Ariak, Teknium and Alignment Labs, and our recurring guest hosts Yam Peleg and Nisten covered two very exciting community efforts, all happening within the SkunksWorks Discord. First effort is called MoE, Open mixture of experts, which is an Open Source attempt at replicating the Mixture of Experts model, which is widely attributed to why GPT-4 is so much better than GPT-3. The second effort is called Ablation studies, which is an effort Teknium is leading to understand once and for all, what is the best, cheapest and most high quality way to finetune open source models, whether it's Qlora or a full finetune or Loras. If you're interested in any of these, either by helping directly or provide resources such as GPU compute, please join the SkunksWorks discord. They will show you how to participate, even if you don't have prior finetuning knowledge! And we’ll keep you apprised of the results once they release any updates! Big Co LLMs + API updates In our Big CO corner, we start with an incredible paper from MetaAi, announcing: Self-Alignment w/ Backtranslation method + Humpback LLM - MetaAI Summarized briefly (definitely listen to the full episode and @yampeleg detailed overview of this method) it’s a way for an LLM to be trained on a unsupervised way of creating high quality datasets, for itself! Using not a lot of initial “seed” data from a high quality dataset. Think of it this way, fine-tuning a model requires a lot of “question → response” data in your dataset, and back-translation proposes “response → question” dataset generation, coming up with novel ways of saying “what would a potential instruction be that would make an LLM generate this result” This results in a model that effectively learns to learn better and create it’s own datasets without humans (well at least human labelers) in the loop. Here are some more reading material on X for reference. OpenAI new JS SDK (X link) OpenAI has partnered with StainlessAPI to released a major new version 4 of their TS/JS SDK with the following incredible DX improvements for AI engineers * Streaming responses for chat & completions * Carefully crafted TypeScript types * Support for ESM, Vercel edge functions, Cloudflare workers, & Deno * Better file upload API for Whisper, fine-tune files, & DALL·E images * Improved error handling through automatic retries & error classes * Increased performance via TCP connection reuse * Simpler initialization logic The most exciting part for me is, this is now very easy to get started with AI projects and get streaming on the incredible Cloudflare workers platform (Targum is part of the first Cloudflare workers launchpad but is not affiliated, we’re just superfans 🫶) Vision & Multi Modality There’s been some really cool stuff happening in computer vision and multi-modal AI recently. First up, a new method called 3D Gaussian Splatting that shows an incredibly clear and smooth way to generate 3d scenes from just a few images. Compared to neural radiance fields (NeRFs), Gaussian splatting produces much smoother results without the grainy voxel artifacts NeRFs often have. However, it achieves this improved quality without sacrificing the speed and performance of NeRFs. So Gaussian splatting gives a big boost in realism compared to NeRF renderings, while maintaining real-time speeds in cleaning up those “clouds” Supervision from Roboflow (and Piotr) Btw our own friend of the pod and AI Vision expert @skalskiP (who reviewed Gaussian Splatting for us) is also having a crazy ThursdAI week, with their open source library called SuperVision, which is a computer vision toolkit, and is trending #2 on Github 👏 Apple stepping in their Vision (not the headset) Transformer game Apple has open sourced ml-fastvit, which is their general purpose Vision Transformers model, which they claim runs at ~1ms on mobile devices, including code and pre-train weights available on Github 🔥 This is great to see from Apple ML teams, not only them open sourcing, but also them preparing all of us to the world of spatial computers (Vision Pro coming remember?) and many new Computer Vision heavy apps will be available at those incredible speeds. This is also great for on device inference running these models in node / on edge (as Friend of the pod @visheratin demonstrated with WebAI) Additional updates included Nvidia releasing a web playground for NeVa, which is their MLLM (Multimodal LLM, get used to seeing this term everywhere) and you can play with that here ), and Link-Context learning for MLLMs Agents OpenAi is also announced that Global Illumination joining OpenAI, that team is CEOd by the creator of Instagram stories algorithm and feed contributor and the team is behind a massive open world minecraft clone. Will we see OpenAI release agents into that world? We know that they are working on agents A16Z - AI Town (🔗) Speaking of agents roaming free and interacting, we covered the open sourcing of SmallVille just last week ↴ and now we see a new open source framework called AI Town of running letting agents roam and interact with each other from Andreessen Horowitz AI division. AI Town (Github) is a web framework, written in TypeScript and is built to run, get customized and run with different LLMs (even Open source ones) in mind and you can see the AI agents running around in a live demo here This ThursdAI was so packed with great information, that it’s really worth listening to the whole recording, you can do this on our Zealous page, RSS and on twitter (all those links can always be found on thursdai.news ) If you found this valuable, join our community and let your friends know? This is a great way to support us, as well as participate in the discussion on social, tag #thursdAI on anything you feel is worthwhile for us to summarize and This is a public episode. If you'd like to discuss this with other subscribers or get access to bonus episodes, visit sub.thursdai.news/subscribe | |||
20 Aug 2023 | 🎙️ThursdAI - LLM Finetuning deep dive, current top OSS LLMs (Platypus 70B, OrctyPus 13B) authors & what to look forward to | 00:52:26 | |
This is a free preview of a paid episode. To hear more, visit sub.thursdai.news Brief outline for your convenience: [00:00] Introduction by Alex Volkov[06:00] Discussing the Platypus models and data curation process by Ariel, Cole and Nathaniel[15:00] Merging Platypus with OpenOrca model by Alignment Labs* Combining strengths of Platypus and OpenOrca* Achieving state-of-the-art 13B model[40:00] Mixture of Experts (MOE) models explanation by Prateek and Far El[47:00] Ablation studies on different fine-tuning methods by Teknium Full transcript is available for our paid subscribers 👇 Why don’t you become one? Here’s a list of folks and models that appear in this episode please follow all of them on X: * ThursdAI cohosts - Alex Volkov, Yam Peleg, Nisten Tajiraj * Garage Baind - Ariel, Cole and Nataniel (platypus-llm.github.io) * Alignment Lab - Austin, Teknium (Discord server) * SkunkWorks OS - Far El, Prateek Yadav, Alpay Ariak (Discord server) I am recording this on August 18th, which marks the one month birthday of the Lama 2 release from Meta. It was the first commercially licensed large language model of its size and quality, and we want to thank the great folks at MetaAI. Yann LeCun, BigZuck and the whole FAIR team. Thank you guys. It's been an incredible month since it was released. We saw a Cambrian explosion of open source communities who make this world better, even since Lama 1. For example, LLaMa.Cpp by Georgi Gerganov is such an incredible example of how open source community comes together and this one guy in the weekend Took the open source weights and made it run on CPUs and much, much faster. Mark Zuckerberg even talked about this, how amazing the open source community has adopted LLAMA, and that Meta is also now adopting many of those techniques and developments back to run their own models cheaper and faster. And so it's been exactly one month since LLAMA 2 was released. And literally every ThursdAI since then, we have covered a new state of the art open source model all based on Lama 2 that topped the open source model charts on Hugging Face. Many of these top models were fine tuned by Discord organizations of super smart folks who just like to work together in the open and open source their work. Many of whom are great friends of the pod. Nous Research, with whom we've had a special episode a couple of weeks back Teknium1 seems to be part of every orgm Alignment Labs and GarageBaind being the last few folks topping the charts. I'm very excited not to only bring you an interview with Alignment Labs and GarageBaind, but also to give you a hint of two additional very exciting efforts that are happening in some of these discords. I also want to highlight how many of those folks do not have data scientist backgrounds. Some of them do. So we had a few PhDs or PhD studies folks, but some of them studied all this at home with the help of GPT 4. And some of them even connected via ThursdAI community and space, which I'm personally very happy about. So this special episode has two parts. The first part we're going to talk with Ariel. Cole and Natniel, currently known as GarageBaind, get it? bAInd, GarageBaind, because they're doing AI in their garage. I love it. 🔥 Who are now holding the record for the best performing open source model called Platypus2-70B-Instruct. And then, joining them is Austin from Alignment Labs, the authors of OpenOrca, also a top performing model, will talk about how they've merged and joined forces and trained the best performing 13b model called Open Orca Platypus 13B or Orctypus 13B This 13b parameters model comes very close to the Base Llama 70b. So, I will say this again, just 1 month after Lama 2 released by the great folks at Meta, we now have a 13 billion parameters model, which is way smaller and cheaper to run that comes very close to the performance benchmarks of a way bigger, very expensive to train and run 70B model. And I find it incredible. And we've only just started, it's been a month. And so the second part you will hear about two additional efforts, one run by Far El, Prateek and Alpay from the SkunksWorks OS Discord, which is an effort to bring everyone an open source mixture of experts model, and you'll hear about what mixture of experts is. And another effort run by a friend of the pod Teknium previously a chart topper himself with Nous Hermes models and many others, to figure out which of the fine tuning methods are the most efficient. and fast and cheap to run. You will hear several mentions of LORAs, which stand for Low Rank Adaptation, which are basically methods of keeping the huge weights of LAMA and other models frozen and retrain and fine tune and align some specific parts of it with new data, which is a method we know from Diffusion World. And it's now applying to the LLM world and showing great promise in how fast, easy, and cheap it is to fine tune these huge models with significantly less hardware costs and time. Specifically, Nataniel Ruiz, the guy who helped Ariel and Cole to train Platypus, the co-author on DreamBooth, StyleDrop and many other diffusion methods, mentioned that it takes around five hours on a single A100 GPU to fine tune the 13B parameter model. That, if you can find an A100 GPU, that's around $10. That's incredible. I hope you enjoy listening and learning from these great folks, and please don’t forget to checkout our website at thursdai.news for all the links, socials and podcast feeds. Brief outline for your convinience: [00:00] Introduction by Alex Volkov [06:00] Discussing the Platypus models and data curation process by Ariel, Cole and Nathaniel [15:00] Merging Platypus with OpenOrca model by Alignment Labs * Combining strengths of Platypus and OpenOrca * Achieving state-of-the-art 13B model [40:00] Mixture of Experts (MOE) models explanation by Prateek and Far El [47:00] Ablation studies on different fine-tuning methods by Teknium Full transcript is available for our paid subscribers 👇 Why don’t you become one? | |||
25 Aug 2023 | ThursdAI Aug 24 - Seamless Voice Model, LLaMa Code, GPT3.5 FineTune API & IDEFICS vision model from HF | 01:08:03 | |
Hey everyone, this week has been incredible (isn’t every week?), and as I’m writing this, I had to pause and go check out breaking news about LLama code which was literally released on ThursdAI as I’m writing the summary! I think Meta deserves their own section in this ThursdAI update 👏 A few reminders before we dive in, we now have a website (thursdai.news) which will have all the links to Apple, Spotify, Full recordings with transcripts and will soon have a calendar you can join to never miss a live space!This whole thing would have been possible without Yam, Nisten, Xenova , VB, Far El, LDJ and other expert speakers from different modalities who join and share their expertise from week to week, and there’s a convenient way to follow all of them now! TL;DR of all topics covered * Voice * Seamless M4T Model from Meta (demo) * Open Source LLM * LLaMa2 - code from Meta * Vision * IDEFICS - A multi modal text + image model from Hugging face * AI Art & Diffusion * 1 year of Stable Diffusion 🎂 * IdeoGram * Big Co LLMs + API updates * AI Tools & Things * Cursor IDE Voice Seamless M4t - A multi lingual, mutli tasking, multimodality voice model. To me, the absolute most mindblowing news of this week was Meta open sourcing (not fully, not commercially licensed) SeamlessM4T This is a multi lingual model that takes speech (and/or text) can generate the following: * Text * Speech * Translated Text * Translated Speech In a single model! For comparison sake, I takes a whole pipeline with whisper and other translators in targum.video not to mention much bigger models, and not to mention I don’t actually generate speech! This incredible news got me giddy and excited so fast, not only because it simplifies and unifies so much of what I do into 1 model, and makes it faster and opens up additional capabilities, but also because I strongly believe in the vision that Language Barriers should not exist and that’s why I built Targum. Meta apparently also believes in this vision, and gave us an incredible new power unlock that understands 100 languages and does so multilingually without effort. Language barriers should not exist Definitely checkout the discussion in the podcast, where VB from the open source audio team on Hugging Face goes in deeper into the exciting implementation details of this model. Open Source LLMs 🔥 LLaMa Code We were patient and we got it! Thank you Yann! Meta releases LLaMa Code, a LlaMa fine-tuned on coding tasks, including “in the middle” completion tasks, which are what copilot does, not just autocompleting code, but taking into account what’s surrounding the code it needs to generate. Available in 7B, 13B and 34B sizes, the largest model beats GPT3.5 on HumanEval, which is a metric for coding tasks. (you can try it here) In an interesting move, they also separately release a specific python finetuned versions, for python code specifically. Additional incredible thing is, it supports 100K context window of code, which is, a LOT of code. However it’s unlikely to be very useful in open source because of the compute required They also give us instruction fine-tuned versions of these models, and recommend using them, since those are finetuned on being helpful to humans rather than just autocomplete code. Boasting impressive numbers, this is of course, just the beginning, the open source community of finetuners is salivating! This is what they were waiting for, can they finetune these new models to beat GPT-4? 🤔 Nous update Friends of the Pod LDJ and Teknium1 are releasing the latest 70B model of their Nous Hermes 2 70B model 👏 We’re waiting on metrics but it potentially beats chatGPT on a few tasks! Exciting times! Vision & Multi Modality IDEFICS - a new 80B model from HuggingFace, was released after a years effort, and is quite quite good. We love vision multimodality here on ThursdAI, we’ve been covering it since we say that GPT-4 demo! IDEFICS is a an effort by hugging face to create a foundational model for multimodality, and it is currently the only visual language model of this scale (80 billion parameters) that is available in open-access. It’s made by fusing the vision transformer CLIP-VIT-H-14 and LLaMa 1, I bet LLaMa 2 is coming soon as well! And the best thing, it’s openly available and you can use it in your code with hugging face transformers library! It’s not perfect of course, and can hallucinate quite a bit, but it’s quite remarkable that we get these models weekly now, and this is just the start! AI Art & Diffusion Stable Diffusion is 1 year old Has it been a year? wow, for me, personally, stable diffusion is what started this whole AI fever dream. SD was the first model I actually ran on my own GPU, the first model I learned how to.. run, and use without relying on APIs. It made me way more comfortable with juggling models, learning what weights were, and we’ll here we are :) I now host a podcast and have a newsletter and I’m part of a community of folks who do the same, train models, discuss AI engineer topics and teach others! Huge thank you to Emad, Stability AI team, my friends there, and everyone else who worked hard on this. Hard to imagine how crazy of a pace we’ve been on since the first SD1.4 release, and how incredibly realistic the images are now compared to what we got then and got excited about! 🎂 IdeaoGram joins the AI art race IdeoGram - new text to image from ex googlers (announcement) is the new kid on the block, not open source (unless I missed it) it boasts significant text capabilities, and really great quality of imagery. It also has a remix ability, and is availble from the web, unlike… MidJourney! Big Co LLMs + API updates Open AI pairs with ScaleAI to let enterprises finetune and run finetuned GPT3.4 models! This is an interesting time for OpenAI to dive into fine-tuning, as open source models inch closer and closer to GPT3.5 on several metrics with each week. Reminder, if you finetune a GPT3.5 model ,you need to provide your own data to OpenAI but then also you have to pay them for essentially hosting a model just for you, which means it’s not going to be cheap. Use as much prompting as humanly possible before you consider doing the above fine-tuning and you may be able to solve your task much better and cheaper. Agents The most interesting thing to me in the world of agents actually came from an IDE! I installed Cursor, the new AI infused VsCode clone, imported my vscode settings, and off we went! It can use your own GPT-4 keys if you don’t want to send them our code or pay, it embeds your whole repo for easy import and code understand and does so much more, like adding a button to every error in console to “debug” and has an “new AI project” feature, which builds you a template just by typing a few words! Our friends Alessio and Swyx have interviewed the founder of Cursor on their podcast, a strong recommendation to check that episode out! After using Cursor for just a few days, I don’t want to go back to VSCode and even consider … maybe pausing my copilot subscription 🤯 That’s all for today folks! I wish you all a great week, and we’ll see you in the next ThursdAI 🫡 Thank you for reading ThursdAI - Recaps of the most high signal AI weekly spaces. This post is public so feel free to share it with a friend? Let’s get to 1K readers 🔥 This is a public episode. If you'd like to discuss this with other subscribers or get access to bonus episodes, visit sub.thursdai.news/subscribe | |||
07 Sep 2023 | ThursdAI Sep 7 - Falcon 180B 🦅 , 🔥 Mojo lang finally here, YaRN scaling interview, Many OSS models & more AI news | 00:29:19 | |
Hey ya’ll, welcome to yet another ThursdAI, this is Alex coming at you every ThursdAI, including a live recording this time! Which was incredible, we chatted about Falcon 180B,had a great interview in the end with 3 authors of the YaRN scaling paper and LLongMa 128K context, had 3 breaking news! in the middle, MOJO🔥 has been released and Adept released a LLaMa comparable OSS model (and friend of the pod) @reach_vb showed an open ASR leaderboard on hugging face! We also covered an incredible tiny model called StarCoder 1B that was finetuned by friend of the pod (who joined the space to talk to us about it!) As always, you can listen to the whole 3 hour long form conversation (raw, unedited) on our Zealous page (and add it to your podcatcher via this RSS) and this short-form pod is available on Apple, Spotify and everywhere. ThursdAI - Hey, if you enjoy these, how about subscribing for real? Would love to do this full time! Every paid subscriber is like a dear friend 🧡 TL;DR of all topics covered * Open Source LLM * Falcon 180B announced by TIIUAE (Announcement, Demo) * YaRN scaling paper - scaling LlaMa to 128K context (link) * OpenHermes-13B from @teknium1 (link) * Persimmon-8B from Adept.AI (link) * Starcoder-1B-sft from @abacaj (link) * Big Co LLMs + API updates * OpenAI first ever Dev conference (link) * Claude announces a $20/mo Claude Pro tier (link) * Modular releases Mojo🔥 with 68,000x improvement over python (Link) * Vision * Real time deepfake with FaceFusion (link) * HeyGen released AI avatars and AI video translation with lipsync (link, translation announcement) * Voice * Open ASR (automatic speech recognition) leaderboard from HuggingFace (link) * Tools * LangChain Hub (re) launched * Open Interpreter (Announcement, Github) Open Source LLM 🦅 Falcon 180B - The largest open source LLM to date (Announcement, Demo) The folks at the “Technology Innovation Institute” have open sourced the huge Falcon 180B, and have put it up on Hugging Face. Having previously open sourced Falcon 40B, the folks from TIIUAE have given us a huge model that beats (base) LLaMa 2 on several evaluations, if just slightly by a few percentages points. It’s huge, was trained on 3.5 trillion tokens and weights above 100GB as a file and requires 400GB for inference. Some folks were not as impressed with Falcon performance, given it’s parameter size is 2.5 those of LLaMa 2 (and likely it took a longer time to train) but the relative benchmarks is just a few percentages higher than LLaMa. It also boasts an embarrassingly low context window of just 2K tokens, and code was just 5% of it’s dataset, even though we already know that more code in the dataset, makes the models smarter! Georgi Gerganov is already running this model on his M2 Ultra because he’s the Goat, and co-host of ThursdAI spaces, Nisten, was able to run this model with CPU-only and with just 4GB of ram 🤯 We’re waiting for Nisten to post a Github on how to run this monsterous model on just CPU, because it’s incredible! However, given the Apache2 license and the fine-tuning community excitement about improving these open models, it’s an incredible feat. and we’re very happy that this was released! The complete open sourcing also matters in terms of geopolitics, this model was developed in the UAE, while in the US, the export of A100 GPUs was banned to the middle easy, and folks are talking about regulating foundational models, and this release, size and parameter model that’s coming out of the United Arab Emirates, for free, is going to definitely add to the discussion wether to regulate AI, open source and fine-tuning huge models! YaRN scaling LLaMa to 128K context window Last week, just in time for ThursdAI, we posted about the release of Yarn-Llama-2-13b-128k, a whopping 32x improvement in context window size on top of the base LLaMa from the folks at Nous Research, Enrico Shippole, @theemozilla with the help of Eluether AI. This week, they released the YaRN: Efficient Context Window Extension of Large Language Models paper which uses Rotary Position Embeddings to stretch the context windows of transformer attention based LLMs significantly. We had friends of the pod Enrico Shippole, theemozilla (Jeff) and Bowen Peng on the twitter space and an special interview with them will be released on Sunday, if you’re interested in scaling and stretching context windows work, definitely subscribe for that episode, it was incredible! It’s great to see that their work is already applied into several places, including CodeLLaMa (which was released with 16K - 100K context) and the problem is now compute, basically, context windows can be stretched, and the models are able to generalize from smaller datasets, such that the next models are predicted to be released with infinite amount of context window, and it’ll depend on your hardware memory requirements. Persimmon-8B from AdeptAI (announcement, github) AdeptAI, the company behind Act-1, a foundational model for AI Agent that does browser driving, and has a few co-founders that are the original transformers paper authors, have dropped a ThursdAI surprise, a fresh (read, not a LLaMa clone) model! Releasing an completely open source model called Persimmon-8B, with a full Apache 2 license, 16K context window (using custom RoPE scaling methods) and some interesting inference speedups with C++. A very interesting 8B model that can fit on most consumer hardware, with additional tricks and a huge context window, is definitely welcome! Additional interesting point is, they have 70K unused embeddings for multimodal extensions! Can’t wait to see what’s that about! Starcoder-1B-sft - tiny model that’s great at code Anton Bacaj (@abacaj) has finetuned StarCoder, to achieve some incredible results, for such a tiny model! Remember the first item, a whopping 180B parameter Falcon? We’ll, this is just 1B parameters model, finetuned on 65K sampled dataset of code, that’s outperforming Falcon, LLaMa2, Palm-2 (and Persimmon) on coding tasks, and runs on your device, so fast, that it’s hard to read! It boasts an incredible 39% on HumanEval task and 31% on MBPP! (Anton reran and updated the MBPP score later) and can run locally. Friend of the pod Xenova has already ported this model to transformers.js and it’ll soon run in your browser! OpenHermes-13B from @teknium1 (link) Our friend Teknium1 (who we’ve interviewed a few weeks ago) releases OpenHermes on top of LLaMa2, but this time it’s a completely open model and datasets, marking this the first time that Hermes models have been open! OpenHermes was trained on 242,000 entries of primarily GPT-4 generated data, from open datasets across the AI landscape, including: * GPTeacher - General Instruct, * Roleplay v1, Roleplay v2, and Code Instruct Datasets, by Teknium * WizardLM (v1, evol_instruct 70k), by WizardLM * Team/nlpxucan Airoboros GPT-4 (v1.0), by JonDurbin * Camel-AI's domain expert datasets, by the Camel-AI Team * CodeAlpaca, by Sahil2801 * GPT4-LLM and * Unnatural Instructions, by Microsoft Check it out folks! Big Co LLM + API updates Modular finally ships Mojo 🔥 (Announcement) I just knew it, that Mojo will finally be shipped during ThursdAI, and in fact, this was a great #BreakingNews moment on twitter spaces! Modular, and it’s co-founder Chris Lattner (author of LLVM, MLIR, Swift and many other things) have finally released their Mojo 🔥 language, for AI. Mojo 🔥 is like Python++, includes strong types, full interoperability with python ecosystem but is able to run basic vanilla python, and has so so much more in it, but the main thing Modular is claiming is a whopping 68,000x improvement over vanilla python! You didn’t misread this, 68,000 improvement, when using all the Modular inference compilers, and Mojo virtualization tricks and compilation improvements. It’s incredible. The beauty of Mojo is that it meets developers where they are and allows them to adopt new features to achieve high performance gradually. By combining the best of dynamic and static languages, Mojo can deliver performance up to 68,000 times faster than Python today. That's quite a leap! If you want to delve deeper into Mojo's origin story, you can find more information in their documentation. But for now, let me highlight a few key benefits that Mojo offers: Firstly, Mojo allows you to write everything in one language, merging the usability of Python with the systems programming features that typically require developers to rely on C, C++, or CUDA. This means that both research and deployment teams can work within a common codebase, streamlining the workflow from research to production. Secondly, Mojo unlocks Python's performance potential. While Python is widely used, it may not be the best tool for high-performance or specialized hardware tasks. However, Mojo bridges that gap by enabling high performance on CPUs and providing support for exotic accelerators like GPUs and ASICs. With Mojo, you can achieve performance levels on par with C++ and CUDA. Thirdly, and this is a big one, Mojo seamlessly integrates with the entire Python ecosystem. You can leverage the extensive library collection available in Python while making use of Mojo's features and performance benefits. This means you can easily combine libraries like NumPy and Matplotlib with your Mojo code – talk about flexibility! Finally, Mojo allows you to upgrade your AI workloads effortlessly. By tightly integrating with the Modular AI Engine, Mojo empowers you to extend your AI workloads with custom operations. This includes pre-processing and post-processing operations, as well as high-performance mathematical algorithms. You can even integrate kernel fusion, graph rewrites, shape functions, and more. Mojo is all about expanding the possibilities! Mojo’s playground has been around since May and I have a deep dive here but you should really watch for over 3 hours on everything from Why they chose to be a python superset, to why he thinks the community will pick it up, it’s an incredible watch and will make you excited about Mojo! WebGPU ships with support for FP16 in Chromium Chrome has shipped with WebGPU back in April of 23’, after years of development, it allows high performance 3D graphics (and of course, transformers inference) in the browser and on the web! However, for inference of models, GPU access is not enough, you also need to be able to run smaller models. Well, one way to make models smaller is to run them in fp16 format. Essentially cutting the precision of the weights numbers by half, we can use much smaller (read compressed) models with a slight loss in accuracy. Friends of the pod Nisten and Xenova (transformers.js author) have given us an update that a new, updated fp16 support has shipped in nightly of chromium, allowing for much much smaller models to be run on clientside! OpenAI first dev conference (Announcement) OpenAI has announced their first developer focused conference, to happen in SF during November 6th! In person only (with the keynote being streamed to all) and they also said that they won’t do any model announcement like GPT-5 😂 But we'll all expect at least a few API updates! Vision FaceFusion 1.1.0 - a deepfake faceswapper (Announcement, Github) We all know deepfakes are here, I mean, don’t we? But did you know that it’s now super easy to face swap your face into an image or a video? FaceFusion does just that, an incredibly fast way to deepfake someone’s face into an image or a video with a few clicks, works on CPU (I couldn’t make it work on GPU but it’s possible) and shows incredible results! Enjoy Steve Buschemi dance around as Harry Styles? 3 clicks and 10 minutes and you get this 🔥 Friend of the pod CocktailPeanut, has made it incredible easy to install with just 1 click with his pinokio.computer app, which I use and love! Facefusion also has a webcam mode that is able to deepfake any image onto a webcam stream for a lot of fun on zoom calls! (which I wasn’t able to test for some reason) HeyGen launches their deep AI face creator Many of us used 11Labs to clone voices, but what if you can clone a voice AND an image of a person? With just 2 minutes of their recording? That’s what HeyGen are claiming to be able to do, and we’ve previously reported that their incredible realistic AI avatar generation from videos/images + voice really blew us away. Heygen just launched their service and you can sign up and get a few minutes for free, here’s a sample (with the CEO avatar, they couldn’t make my own for some launch day errors) The video you see on top of just that, the CEO of HeyGen, thanking you for reading this weeks ThursdAI! Voice ASR leaderboard + New top ASR model from Nvidia I love doing ThursdAI, and one of the things I love most, is folks sending me stuff they worked on, and then coming to ThursdAI to chat about it. Friend of the pod Vaibhav (VB) Srivastav, who’s an incredible dev rel at HuggingFace, focusing on Audio, has shipped a new Open-ASR (automatic speech recognition) leaderboard on huggingface! Showing the top ASR models like Whisper and a new comer, Nvidia FastConformer, which I didn’t even know existed, and now it’s topping Whisper for english speech to text tasks! HuggingFace leaderboards like these are definitely a boon for the Open Source industry as they allow all of us to easily select open source models, but also allow the open source community to start racing towards the top, while we all benefit! Tools Open Interpreter (Announcement, Github) One tool that I’ve used this week, and is incredible, is OpenInterpreter from @heyitskillian It’s incredibly easy to install and run, and behaves like OpenAI Code Interpreter (renamed to Advanced Data Analytics) but on your computer, and is able to do things like control your apps, lower volume, edit images/files and tons more pip install open-interpreter And that’s it! Give it a try (and you have to approve each command that it runs) It’s a great agent, and hopefully we’ll get Killian to chat with us about it on next ThursdAI! LangChain hub has launched (link) If you’re into LangChain, and even if you aren’t, it’s undeniable the weight LangChain has in the ai engineer industry! They have a connector for everything, tons of folks use them, and they have raised a bunch of funding. They have just launched their new LangChain Hub and it’s exciting! Many folks are sharing their best prompts on there, and ways to work with langchain, with upvotes and sharable links! Also, worth nothing that our friends swyx and Alessio from Latent Space have recently released an episode with Harrison on Latent space, and it’s WELL worth listening (and reading) as swyx did a deep dive into Landchain, it’s nay-sayers and everything in between! Check it out below : Thank you, see you next time (with some incredible personal news I’ll have to share) ThursdAI - Recaps of the most high signal AI weekly spaces is a reader-supported publication. To receive new posts and support my work, consider becoming a free or paid subscriber. This is a public episode. If you'd like to discuss this with other subscribers or get access to bonus episodes, visit sub.thursdai.news/subscribe | |||
10 Sep 2023 | 🔥🎙️ ThursdAI Sunday special - Extending LLaMa to 128K context window (2 orders of magnitude) with YaRN [Interview with authors] | 00:54:21 | |
This is a free preview of a paid episode. To hear more, visit sub.thursdai.news Happy Sunday everyone, I am very excited to bring you this interview with the folks who took LLaMa 2 and made it LLoooooongMa! Extending LLaMa 2 context window from 4,000 to a whopping 128,000 tokens (Yarn-Llama-2-13b-128k on Hugging Face), these guys also came up with a paper called YaRN (Efficient Context Window Extension of Large Language Models) and showed that YaRN is not only requires 10x less tokens to create these long contexts, but also 2.5x less training steps! And, the models generalize so there’s now no need to collect extremely long sequences (think books length sequences) for the models to understand those context lengths. I have decided also to do something different (which took me half of Sunday so I can’t promise and am not committing to this format, but for the premium subscribers, you can now watch this interview with running Karaoke style subtitles and improved audio! This will be uploaded to Youtube in a week but aren’t you glad you subscribed and is getting this first?) Here’s a teaser preview: And here’s the chapter for your convenience (the only thing that’s ai generated 😂) 0:00 - Introduction 3:08 - Discussion of extending LLAMA2's context length from 4,000 tokens to 128,000 tokens using the YaRN method 8:23 - Explanation of rope scaling for positional encodings in transformers 13:21 - How the rope scaling idea allows for longer context through positional interpolation 18:51 - Using in-context learning to train models on shorter sequences but still handle long contexts 25:18 - Sourcing long-form data like books to train 128k token models 31:21 - Whether future models will natively support longer contexts 37:33 - New model from Adept with 16k context using rope scaling 42:46 - Attention is quadratic - need better algorithms to make long context usable 49:39 - Open source community pushing state of the art alongside big labs 52:34 - Closing thoughts As always, full (manually edited) transcription (and this time a special video version!) is reserved for the premium subscribers, I promise it’ll be worth it, so why not .. y’know? skip a cup of coffee from SB and support ThursdAI? | |||
15 Sep 2023 | 🔥 ThursdAI Sep 14 - Phi 1.5, Open XTTS 🗣️, Baichuan2 13B, Stable Audio 🎶, Nougat OCR and a personal life update from Alex | 01:31:41 | |
This is a free preview of a paid episode. To hear more, visit sub.thursdai.news Hey, welcome to yet another ThursdAI 🫡 This episode is special for several reasons, one of which, I shared a personal life update (got to listen to the episode to hear 😉) but also, this is the first time I took the mountainous challenge of fixing, editing and “video-fying” (is that a word?) our whole live recording! All 3 hours of it, were condensed, sliced, sound improved (x audio quality is really dogshit) and uploaded for your convenience. Please let me know what you think! Premium folks get access to the full podcast in audiogram format, and a full transcription with timestamps and speakers, here’s a sneak preview of how that looks, why not subscribe? 😮 TL;DR of all topics covered * Open Source LLM * Microsoft Phi 1.5 - a tiny model that beats other 7B models (with a twist?) (Paper, Model) * Baichuan 7B / 13B - a bilingual (cn/en) model with highly crafted approach to training (Paper, Github) * Big Co LLMs + API updates * Nothing major this week * Voice & Audio * Stable Audio 🎶 - A new music generation model from Stability AI. (Website) * Coqui XTTS - an open source multilingual text to speech for training and generating a cloned voice (Github, HuggingFace) * AI Art & Diffusion * Würstchen v2 - A new super quick 1024 diffusion model (Announcement, Demo, Github) * DiffBIR - Towards Blind Image Restoration with Generative Diffusion Prior (Annoucement, Demo, Github) * Tools * Nougat from Meta - open-source OCR model that accurately scans books with heavy math/scientific notations (Announcement, Github, Paper) * GPT4All Vulkan from Nomic - Run LLMs on ANY consumer GPUs, not just NVIDIA (Announcement) * Nisten’s AI ISO disk - Announcement And here are timestamps and chapter/discussion topics for your convenience: [00:05:56] Phi 1.5 - 1.3B parameter model that closely matches Falcon & LLaMa 7B [00:09:08] Potential Data Contamination with Phi 1.5 [00:10:11] Data Contamination unconfirmed [00:12:59] Tiny models are all the rage lately [00:16:23] Synthetic Dataset for Phi [00:18:37] Are we going to run out of training data? [00:20:31] Breaking News - Nougat - OCR from Meta [00:23:12] Nisten - AI ISO disk [00:29:08] Baichuan 7B - an immaculate Chinese model [00:36:16] Unique Loss Terms [00:38:37] Baichuan ByLingual and MultiLingual dataset [00:39:30] Finetunes of Baichuan [00:42:28] Philosophical questions in the dataset [00:45:21] Let's think step by step [00:48:17] Is breath related text in the original dataset? [00:50:27] Counterintuitive prompting for models with no breath [00:55:36] Idea spaces [00:59:59] Alex - Life update about ThursdAI [01:04:30] Stable Audio from Stability AI [01:17:23] GPT4ALL Vulkan [01:19:37] Coqui.ai releases XTTS - an open source TTS - interview With Josh Meyer [01:30:40] Summary Here’s a full video of the pod, and a full transcription, and as always, 🧡 thank you for bring a paid subscriber, this really gives me the energy to keep going, get better guests, release dope podcast content, and have 3 hours spaces and then spend 7 hours editing 🔥 | |||
17 Sep 2023 | 📅 ThursdAI - Special interview with Killian Lukas, Author of Open Interpreter (23K Github stars for the first week) 🔥 | 00:55:06 | |
This is a free preview of a paid episode. To hear more, visit sub.thursdai.news Hey! Welcome to this special ThursdAI Sunday episode. Today I'm excited to share my interview with Killian Lucas, the creator of Open Interpreter - an incredible new open source project that lets you run code via AI models like GPT-4 or local models like Llama on your own machine. Just a quick note, that while this episode is provided for free, premium subscribers enjoy the full write up including my examples of using Open Interpreter, the complete (manually edited) transcript and a video form of the pod for easier viewing, search, highlights and more. Here’s a trailer of that in case you consider subscribing If you haven’t caught up with GPT-4 Code Interpreter yet (now renamed to Advanced Data Analytics), I joined Simon Willison and swyx when it first launched and we had a deep dive about it on Latent Space and even at the day of the release, we were already noticing a major restricting factor, Code Interpreter is amazing, but doesn’t have internet access, and can’t install new packages, or use new tools. An additional thing we immediately noticed was, the surface area of “what it can do” is vast, given it can write arbitrary code per request, it was very interesting to hear what other folks are using it for for inspiration, and “imagination unlock”. I started a hashtag called #codeinterpreterCan and have since documented many interesting use cases, like comitting to git, running a vector DB, convert audio & video to different formats, plot wind rose diagrams, run whisper and so much more. I personally have all but switched to Code Interpreter (ADA) as my main chatGPT tab, and it’s currently the reason I’m still paying the 20 bucks! Enter, Open interpreter Just a week after open sourcing Open Interpreter, it already has over 20,000 stars on GitHub and a huge following. You can follow Killian on Twitter and check out the Open Interpreter GitHub repo to learn more. Installing is as easy as pip install open-interpreter. (but do make sure to install and run it inside a venv or a conda env, trust me!) And then, you just.. ask for stuff! (and sometimes ask again as you’ll see in the below usage video) Specifically, highlighted in the incredible launch video, if you’re using a mac, Code Interpreter can write and run AppleScript, which can run and control most of the native apps and settings on your mac. Here’s a quick example I recorded while writing this post up, where I ask Open Interpreter to switch system to Dark mode, then I use it to actually help me extract all the chapters for this interview and cut a trailer together! | |||
22 Sep 2023 | 📆 ThursdAI Sep 21 - OpenAI 🖼️ DALL-E 3, 3.5 Instruct & Gobi, Windows Copilot, Bard Extensions, WebGPU, ChainOfDensity, RemeberAll | 01:09:20 | |
Hey dear ThursdAI friends, as always I’m very excited to bring you this edition of ThursdAI, September 21st, which is packed full of goodness updates, great conversations with experts, breaking AI news and not 1 but 2 interviews ThursdAI - hey, psst, if you got here from X, dont’ worry, I don’t spam, but def. subscribe, you’ll be the coolest most up to date AI person you know! TL;DR of all topics covered * AI Art & Diffusion * 🖼️ DALL-E 3 - High quality art, with a built in brain (Announcement, Comparison to MJ) * Microsoft - Bing will have DALL-E 3 for free (Link) * Big Co LLMs + API updates * Microsoft - Windows Copilot 🔥 (Announcement, Demo) * OpenAI - GPT3.5 instruct (Link) * OpenAI - Finetuning UI (and finetuning your finetunes) (Annoucement, Link) * Google - Bard has extensions (twitter thread, video) * Open Source LLM * Glaive-coder-7B (Announcement, Model, Arena) * Yann Lecun testimony in front of US senate (Opening Statement, Thread) * Vision * Leak : OpenAI GPT4 Vision is coming soon + Gobi multimodal? (source) * Tools & Prompts * Chain of Density - a great summarizer prompt technique (Link, Paper, Playground) * Cardinal - AI infused product backlog (ProductHunt) * Glaive Arena - (link) AI Art + Diffusion DALL-E 3 - High quality art, with a built in brain DALL-E 2 was the reason I went hard into everything AI, I have a condition called Aphantasia, and when I learned that AI tools can help me regain a part of my brain that’s missing, I was in complete AWE. My first “AI” project was a chrome extension that injects prompts into DALL-E UI to help with prompt engineering. Well, now not only is my extension no longer needed, prompt engineering for AI art itself may die a slow death with DALL-E 3, which is going to be integrated into chatGPT interface, and chatGPT will be able to help you… chat with your creation, ask for modifications, alternative styles, and suggest different art directions! In addition to this incredible new interface, which I think is going to change the whole AI art field, the images are of mind-blowing quality, coherence of objects and scene elements is top notch, and the ability to tweak tiny detail really shines! Additional thing they really fixed is hands and text! Get ready for SO many memes coming at you! Btw, I created a conversational generation bot in my telegram chatGPT bot (before there was an API with stability diffusion and I can only remember how addicting this was!) and so did my friends from Krea :) so y’know… where’s our free dall-e credits OpenAI? 🤔 Just kidding, an additional awesome thing that now, DALL-E will be integrated into chatGPT plus subscription (and enterprise) and will refuse to generate any living artists art, and has a very very strong bias towards “clean” imagery. I wonder how fast will it come to an API, but this is incredible news! P.S - if you don’t want to pay for chatGPT, apparently DALL-E 3 conversational is already being rolled out as a free offering for Bing Chat 👀 Only for a certain percentage of users, but will be free for everyone going forward! Big Co LLM + API updates Copilot, no longer just for code? Microsoft has announced some breaking news on #thursdai, where they confirmed that Copilot is now a piece of the new windows, and will live just a shortcut away from many many people. I think this is absolutely revolutionary, as just last week we chatted with Killian from Open Interpreter and having an LLM run things on my machine was one of the main reasons I was really excited about it! And now we have a full on, baked AI agent, inside the worlds most popular operating system, running for free, for all mom and pop windows computers out there, with just a shortcut away! Copilot will be a native part of many apps, not only windows, here’s an example of a powerpoint copilot! As we chatted on the pod, this will put AI into the hands of so so many people for whom opening the chatGPT interface is beyond them, and I find it incredibly exciting development! (I will not be switching to windows for it tho, will you?) Btw, shoutout to Mikhail Parakhin who lead the BingChat integration and is now in charge of the whole windows division! It shows how much dedication to AI Microsoft is showing and it really seems that they don’t want to “miss” this revolution like they did with mobile! OpenAI releases GPT 3.5 instruct turbo! For many of us, who used GPT3 APIs before it was cool (who has the 43 character API key 🙋♂️) we remember the “instruct” models where all the rage, and then OpenAI basically told everyone to switch to the much faster and more RLHFd chat interfaces. Well now, they brought GPT3.5 back, with instruct and turbo mode, it’s no longer a chat, it’s a completion model, that is apparently much better at chess? An additional interesting thing is, it includes logprobs in the response, so you can actually build much more interesting software (by asking for several responses and then looking at the log probabilities), for example, if you’re asking the model for a multiple choice answer to a question, you can rank the answers based on logprobs! Listen to the pod, Raunak explains this really well! FineTune your finetunes OpenAI also released a UI for finetuning GPT3.5 and upped the number of concurrent finetunes to 3, and now, you can finetune your finetunes! So you can continue finetuning already finetuned models! Bard extensions are like chatGPT plugins but more native. While we wait for Gemini (cmon google, just drop it!) the multi modal upcoming incredible LLM that will beat GPT-4 allegedly, Google is shoving new unbacked features into Bard (remember Bard? It’s like the 5th most used AI assistant!) You can now opt in, and @ mention stuff like Gmail, Youtube, Drive and many more Google services and Bard will connect to them, do a search (not a vector search apparently, just a keyword search) and will show you results (or summarize your documents) inside Bard interface. The @ ui is really cool, and reminded me of Cursor (where you can @ different files or documentation) but in practice, from my 2 checks, it really didn’t work at all and was worse than just a keyword search. Open Source LLM Glaive-coder-7B reaches an incredible 63% on human eval Friends of the pod Anton Bacaj and Sahil Chaudhary have open sourced a beast of a coder model Glaive-coder-7B, with just 7B parameters, this model achieves an enormous 63% on HumanEval@1, which is higher than LLaMa 2, Code LLaMa and even GPT 3.5 (based on technical reports) at just a tiny 7B parameters 🔥 (table from code-llama released for reference, the table is now meaningless 😂) Yann Lecun testimony in front of US senate Look, we get it, the meeting of the CEOs (and Clem from HuggingFace) made more waves, especially on this huge table, who wasn’t there, Elon, Bill Gates, Sundar, Satya, Zuck, IBM, Sam Altman But IMO the real deal government AI thing was done by Yann Lecun, chief scientist at Meta AI, who came in hot, with very pro open source opening statements, and was very patient with the very surprised senators on the committee. Opening statement is worth watching in full (I transcribed it with Targum cause… duh) and Yann actually retweeted! 🫶 Here’s a little taste, where Yann is saying, literally “make progress as fast as we can” 🙇♂️ He was also asked about, what happens if US over-restricts open source AI, and our adversaries will … not? Will we be at a disadvantage? Good questions senators, I like this thinking, more of this please. Vision Gobi and GPT4-Vision are incoming to beat Gemini to the punch? According to The Information, OpenAi is gearing up to give us the vision model of GPT-4 due to the hinted upcoming release of Gemini, a multi modal model from Google (that’s also rumored to be released very soon, I’m sure they will release this on next ThursdAI, or the one after that!) It seems to be the case for both DALL-E 3 and the leak about GPT-4 Vision, because apparently Gemini is multi modal on the input (can take images and text) AND the output (can generate text and images) and OpenAI maybe wants to get ahead of that. We’ve seen images of GPT-4 Vision in the chatGPT UI that were leaked, so it’s only a matter of time. The most interesting thing from this leak was the model codenamed GOBI, which is going to be a “true” multimodal model, unlike GPT-4 vision. Here’s an explanation of the difference from Yam Peleg , ThursdAI expert on everything language models! Voice Honestly, nothing major happened with voice since last week 👀 Tools Chain of Density The Salesforce AI team has developed a new technique for improving text summarization with large language models. Called Chain of Density (CoD), this prompting method allows users to incrementally increase the informational density of a summary. The key insight is balancing the right amount of details and main ideas when summarizing text. With CoD, you can prompt the model to add more detail until an optimal summary is reached. This gives more control over the summary output. The Salesforce researchers tested CoD against vanilla GPT summaries in a human preference study. The results showed people preferred the CoD versions, demonstrating the effectiveness of this approach. Overall, the Salesforce AI team has introduced an innovative way to enhance text summarization with large language models. By tuning the density of the output, CoD prompts can produce higher quality summaries. It will be exciting to see where they take this promising technique in the future. RememberAll - extend your LLM context with a proxy We had Raunak from rememberAll on the pod this week, and that interview is probably coming on Sunday, but wanted to include this in tools as it’s super cool. Basically with 2 lines of code change, you can send your API calls through RememberAll proxy, and they will extract the key information, and embed and store it in a vectorDB for you, and then inject it back on responses. Super clever way to extend memory, here’s a preview from Raunak (demo) and a more full interview is coming soon! Cardinal has launched on ProductHunt, from my friends Wiz and Mor (link) Quick friendly plug, Wix and Mor are friends of mine and they have just launched Cardinal, an AI infused product backlog, that extracts features, discussion about feature requests, and more, from customer feedback, from tons of sources. Go give them a try, if you’re looking to make your product backlog work better, it’s really really slick! Hey, if you arrived here, do me a quick favor? Send me a DM with this emoji 🥔 , and then share this newsletter with 1 friend who like you, loves AI? Thanks, I expect many potatoes in my inbox! See you next ThursdAI 🫡 Here’s the full transcript (no video this time, I’m finishing this up at 10:30 and video will take me at least 3 more hours, apologies 🙇♂️) [00:10:21] Alex Introduces Yam Peleg [00:10:57] Alex Introduces Nisten Tahiraj [00:11:10] Alex Introduces Far El [00:11:24] Alex Introduces Xenova [00:11:44] Alex Introduces Roie S. Cohen [00:11:53] Alex Introduces Tzafrir Rehan [00:12:16] DALL-E 3 - An AI art model with a brain, coming to chatGPT plus [00:20:33] Microsoft c launches Windows CoPilot [00:30:46] Open AI leaks, GPT-4 Vision, Gobic [00:38:36] 3.5 instruct model from OpenAI [00:43:03] Raunak intro [00:43:25] Bard Extensions allow access to GMail, Youtube, Drive FULL transcript: [00:00:00] Alex Volkov: So, Thursday I is this wonderful thing that happened and happened organically as well. [00:00:26] And basically what happens is we have this live recording every Thursday, every ThursdAI on Twitter spaces. I am I'm very grateful to share the stage with experts in their fields, and we all talk about different things, because AI updates are so multidisciplinary right now. It's really hard for even experts in their one field to follow everything. [00:00:51] I find this mixture of experts type model on stage very conducive because we all go and find the most up to date things from the last week. And then we have folks who, it's their specification, for example, to comment on them. And you guys in the audience get the benefit of this. And it just happened organically through many conversations we had on, on Spaces since GPT 4 was launched. [00:01:16] Literally the day, March 14th, 2023 aka Pi Day. It was the first day we started these spaces, and since then the community has grown to just... An incredible amount of people who join quality experts, top of their field people. I'm, I'm just so humbled by all of this. And since then, many folks told me, like Roy here in the audience, that, Hey, Alex, you're doing this in this weirdest hour. [00:01:42] Thursday a. m. in San Francisco, nobody's gonna come. It's really hard to participate in the actual live recording. And so, I started a newsletter and a podcast for this. And so, if you aren't able to make it, I more than welcome you to register to the newsletter. You know what? Even if you are here every week, register to the newsletter, because why not? [00:02:03] Because, share it with your friends. We're talking about everything AI related. Hopefully, hopefully no hype. And I have friends here to reduce the hype when I'm getting too hypey. Definitely none of the, Hey, here's a new AI tool that will help you fix the thing you don't need fixing. [00:02:18] And I think that's, that's been resonating with the community. And so, as you now are here, you're also participant in this community. I welcome everybody to Tag Thursday AI on their news about ai or #thursdAI, or just like the Thursday iPod, which probably should join this so people get some more visibility. but you are part of the community. Now, those of you who come back, those of you who listen in, those of you who share all of them. All of these things are very helpful for the community to grow and for us to just know about more stuff. [00:02:49] It's actually an incredible signal when two or three or more of you react under a piece of news and say, hey, we probably should cover this in Thursday. It really helps, truly. I think with that, yeah, I think this intro is enough intro. Welcome. What's up, Tzafrir? How are you? [00:03:06] Tzafrir Rehan: All's well. Thank you very much. I wanted to, to strengthen your point about the time factor. So we expand. So anyone here who wants to be a little bit interested in generative technologies and breaking news and have some things to do in the meanwhile, and also looking to actually build something cool from all of this. [00:03:31] Time is the limiting factor here. That's like the, the hardest resource here. Having this group and having everyone explore everything together. It's a lifesaver. It's like a order of magnitude improvement on our ability to move forward each one individually. And that's a group together just to give examples. [00:03:53] So I'm interested in generative images, videos, and audio. And for each of these, there are hundreds of models right now available. With the availability to make fine tunes on specific datasets for some of these generating a single asset like a video can take hours. Training takes hours. If you want to explore a little bit like the effect of different prompts, just generating hundreds of samples takes hours. [00:04:26] So without this group, it would be impossible to even know. Where to go and where to invest my time And the name of the game right now is to just choose where you invest your time on To actually get things done and keep up. So thank you. Thank you. Thank you for you and for this group And let's have fun. [00:04:46] Alex Volkov: Thank you. Thank you everyone. I definitely feel super powered by the people in this group who can like back me up on, I read one tweet and then I saw some people react to this tweet, but I didn't have the time or the capability or the experience to dive in. [00:05:00] And then there's folks here who did, and then we're going to complete each other. And I think our model, I haven't shared since we started, but our motto is we stay up to date. So you don't have to and have to, I think is the operating word. You want to stay up to date and you're welcome to stay up to date and you're welcome to tag us and talk with us and leave comments here in the chat as well, but you don't have to anymore because, there's a, there's a newsletter that will update you and there's folks on stage who will talk about this. [00:05:26] I want to briefly cover one tiny thing that I did on the podcast that I think I will start doing as well. So, so far editing this hour and a half, two hours that we have here live was a pain, but I just decided to lean into this because. The conversation we're having here is so much more informative and interesting that any type of summary that I want to do or wanted to do is not going to do it justice. [00:05:50] And so I had some different feedback from different folks about the length of the podcast. Some people said, yeah, 25 minutes, just the updates is like the right spot. And yeah, the podcast is moving towards. This is going to be the live recording. I'm going to edit this don't worry. [00:06:04] But besides that, the podcast will be this conversation. Going forward as much as I'm able to edit this, and ship both the newsletter and the podcast in time on Thursday But with that Tzafrir thank you for the kind words, man. I appreciate you being here and sharing with us your expertise [00:06:20] I want to say hi to Zenova and Arthur. [00:06:22] We'll start with Zenova. Welcome Josh. How are you? [00:06:27] Xenova: Yeah. Hey Yeah, pretty good. Been busy, busy, busy [00:06:33] for those who Don't know. I'll just quickly introduce myself. I am the creator of Transformers. js, which is a JavaScript library for running HuggingFace Transformers directly in the browser, or Node, or Deno, or maybe Bunsoon. [00:06:49] Who knows when that gets sorted out properly, but any JavaScript environment that you're, that you're looking for. And, yeah, I recently joined HuggingFace, which is exciting. Now I'm able to sort of work on it basically full time. And yeah, lots of, lots of exciting things are, are in the pipeline. [00:07:06] Alex Volkov: It's been incredible to have you here and then see your progress with Transformer. [00:07:10] js and then you joining Hug and Faceman. I appreciate the time here. [00:07:13] Arthur, thank you for joining. Please feel free to introduce yourself. [00:07:18] Arthur Islamov: Okay. So, my name is Arthur and I'm fixing and making WebAssembly to work with big models. [00:07:25] So, soon you will be able to run anything huge in the browser, and I'm particularly interested in diffusion models, so right now I'm making the Staple Diffusion 2. 1 to work in the browser, and then have some plans to make SDXL, and maybe as well as Lama and other models too. With all that work done. [00:07:50] Alex Volkov: That's awesome. Thank you for joining. [00:07:52] Far El: Yo, what's up? Yeah, I'm my name is Farouk. I'm like founder of Nod. ai where we build autonomous agents and also working on skunkworks. ai, which is an open source group where we are pushing the boundaries of what we can do with LLMs and AI as a whole, really. [00:08:10] Our first, like, major project is this open source MOE architecture that we've been tinkering around with for the last couple months. We're also exploring even more, exotic AI arcs to try to get, to GPT 4 level capability for open source. [00:08:28] Alex Volkov: Awesome. Awesome. Awesome. And Nistan, welcome brother. [00:08:33] Yam Peleg: Yeah. Hey everyone, I'm Nistan Tahirai and I'm terminally online. That's the introduction. Thank you. Yeah, I, I'm also, I'm a dev in Toronto. I worked on the first doctor wrapper which is still doing pretty well. Like no complaints so far, six months later, knock on wood. And yeah, recently started doing a lot more open source stuff. [00:09:03] Put out a bunch of open source doctor models on, on HuggingFace, which I still need to write a benchmark for because there is no safety benchmarks that are public. And yeah, lately been working with Farouk to make the whole Sconcrooks AI mixture of experts model more usable because it's still, it's not even bleeding edge. [00:09:26] And this one is more like hemorrhaging edge technology. It takes like three people to get it to work. And yeah, I've been extremely interested on the web GPU side ever since Zenova on a random tweet just gave me the command to start Chrome Canary properly. And then I was able to load it. Whole seven B model. [00:09:48] And yeah, I'm thinking next for the future, if, if things go okay. I mean, my goal that I've set myself is to have some kind of distributed. Mixture of experts running via WebGPU and then having Gantt. js encrypts the connections between the, the different nodes and experts. And we'll see how that plays out because everything is changing so quickly. [00:10:14] But yeah, it's, it's good to be here. And I'm glad I found this Twitter space randomly way back in [00:10:21] Alex Introduces Yam Peleg [00:10:21] Alex Volkov: Yeah, for a long time. I just want to welcome Yam to the stage. And Yam doesn't love introducing himself, but I can do it for you Yam this time if you'd like. [00:10:31] All right. So, I will just run through the speakers on stage just real quick. Yam, thank you for joining us. Folks, Yam is our, I could say, resident... Machine learning engineer extraordinaire everything from data sets and training large language models understanding the internals of how they work and baking a few of his own definitely The guy who if we found the interesting paper, he will be able to explain this to us [00:10:57] Alex Introduces Nisten Tahiraj [00:10:57] Alex Volkov: Nisten. I call you like The AI engineer hacker type, like the stuff that you sometimes do, we're all in awe of being able to run stuff on CPU and doing different, like, approaches that, like, nobody thought of them before. [00:11:10] Alex Introduces Far El [00:11:10] Alex Volkov: Far El you're doing, like, great community organizing and we're waiting to see from the MOE and Skunkworks. [00:11:15] And folks should definitely follow Far El for that and join Skunkworks OS. It's really hard for me to say. Skunks. Works OS efforts in the discord. [00:11:24] Alex Introduces Xenova [00:11:24] Alex Volkov: Zenova is our run models on the client guy so Transformers. js, everything related to ONNX and everything related to quantization and making the models smaller. [00:11:35] All of that. All models, all modularities, but I think the focus is on, on the browser after you're new, but obviously you introduce yourself, WebGPU stuff. [00:11:44] Alex Introduces Roie S. Cohen [00:11:44] Alex Volkov: We have Roy, who's a DevRel in Pinecon, who he didn't say, but Pinecon and VectorDB is in Context Windows and, and discussion about RAG, like all of these things Roy is our go to. [00:11:53] Alex Introduces Tzafrir Rehan [00:11:53] Alex Volkov: And Tzafrir also introduced himself, everything vision, audio, and excitement. So a very well rounded group here. And I definitely recommend everybody to follow. And now with that, now that we are complete, let's please start with the updates because we have an incredible, incredible Thursday, literally every week, right folks? [00:12:12] Literally every week we have an incredible Thursday [00:12:16] DALL-E 3 - An AI art model with a brain, coming to chatGPT plus [00:12:16] Alex Volkov: so we'll start with, with two big ones. I want to say the first big update was obviously DALL-E 3. So I will just share briefly about my story with DALL-E and then I would love folks on stage also to chime in. Please raise your hand so we don't talk over each other. DALL-E when it came out, When the announcement came out for DALL-E 2, I want to say it was a year ago in, a year and a half ago, maybe, in January, February or something, this blew me away. [00:12:47] I have something called aphantasia, where, I don't know if you saw this, but like, I don't have like the visual mind's eye, so I can't like visually see things, and it's been a thing with me all my life, and then here comes the AI tool that can draw. Very quickly, then I turned my, I noticed stable diffusion, for example, and I just like. [00:13:04] It took away from there. Everything that I have, all my interest in AI started from DALL-E basically. And DALL-E 3 seems like the next step in all of this. And the reason I'm saying this is because DALL-E 3 is visually incredible, but this is not actually like the biggest part about this, right? We have mid journey. [00:13:22] I pinned somebody's comparison between DALL-E and mid journey. And Midrani is beautiful and Gorgias is a way smaller team. DALL-E 3 has this beautiful thing where it's connected to ChatGPT. So not only is it like going to be not separate anymore, you're going to have the chat interface into DALL-E 3. [00:13:41] ChatGPT will be able to help you. As a prompt engineer, and you'd be able to chat with the creation process itself. So you will ask for an image, and if you don't know how to actually define what you want in this image, which types, you'd be able to just chat with it. You will say, you know what, actually make it darker, make it more cartoony, whatever. [00:14:01] And then chatGPT itself with its brain is going to be your prompt engineer body in the creation. And I think. Quality aside, which quality is really, really good. The thing they're highlighting for, for DALL-E 3 is the ability to have multiple. Objects and subjects from your prompt in one image because it understands them. [00:14:23] But also definitely the piece where you can keep talking to an image is changing the image creation UI significantly where, mid journey. With all, all the love we have for Midjourney is still stuck in Discord. They're still working on the web. It's, it's taking a long time and we've talked about Ideogram to lead them from the side. [00:14:44] We know that Google has multiple image models like Imogen and different ones. They have like three, I think at this point, that they haven't yet released. And DALL-E, I think is the first. Multimodal on the output model that we'll get, right? So multimodal on the output means that what you get back towards you is not only text generation and we saw some other stuff, right? [00:15:06] We saw some graphs, we saw some code interpreter can run code, etc. But this is a multimodal on the output. And Very exciting. I, I, DALL-E 3 news took Twitter by storm. Everybody started sharing this, including us. We can't wait to play with DALL-E 3. I welcome folks on stage. I want to start with Zafreer reaction, but definitely to share what we think about this. [00:15:26] And the last thing I'll say... Say is that now that the community community is growing, suddenly people dmm me. So first of all, you're all welcome to DM me about different stuff. I see I see somebody in the audience with DM me. I think she's still here. So shout out about joining the better test for DALL-E three, which now they, they're able to share about Funny tidbit, it will, it's right now baked into the UI. [00:15:48] So Dally 3 is going to be baked into ChatGPT and ChatGPT Enterprise UIs. However, when they tested this, they tested it via a plugin. So OpenAI actually built a plugin and had like a restricted access to this plugin. And folks who like talked with this plugin, the plugin ran the Dally ChatGPT version behind the scenes. [00:16:06] And we don't have access to it yet. I don't know if anybody on stage has access. Please tell me if you do. The access is coming soon, which is interesting from OpenAI. And I think that's most of the daily stuff that I had. And I want to, please, please, buddy, I want to hear from Zafira, please. [00:16:23] And please raise your hand. I really need us to not talk over each other. [00:16:30] Thank you. [00:16:31] Tzafrir Rehan: So yeah, DALL-E 3 is looking amazing. I did see some examples that people with early [00:16:38] access were [00:16:38] generating, and it's far more detailed and coherent than the things we are used to seeing from stable diffusion. And much less randomness, I would say. And what's exciting here is a few changes in the paradigm of how it works. [00:16:56] For example, like you said, [00:16:59] it doesn't expect you to know all the intricacies. You can describe in [00:17:03] your natural language what you want to see [00:17:05] and it will use [00:17:07] GPT, however much they are powering the, for generating a prompt to make the whole image. That's the one thing. The other thing is that it's not [00:17:19] text to image. [00:17:21] It's more a conversation. Similar to how chat GPT is a conversation between you and the assistant. DALL-E 3 is a chat. So you can see in the video that they released. You generate one image and then you discuss if you want to make changes to it, if you want to make more variations, and that would be very interesting to see the flow. [00:17:44] From the AI artist perspective, I think it will be met with a little bit hesitation, at least not knowing how much fine control they are providing. If they are letting away... to influence all these various parameters that the model uses. That is a lot of the workflow for generating AI art. [00:18:06] And when you want to make a piece for release as an artist, you spend a lot of time fine tuning it. [00:18:13] And today with Stable Diffusion, and with Mid Journey, we have a lot of fine grained control over changing the parameters by a little bit, adding one more word, That's one thing, and another thing is that artists usually actually want to have that control over the prompt. For example, this week I saw an interesting example, I'll try to find it for you, where the artist adds the words Event horizon to an image. [00:18:44] Now the image is not of space, but the model does take that idea of the event horizon shape, and makes the image more shaped like an event horizon. So those are the kinds of tricks that right now prompt engineers use to make very specific changes in the image. So I'm interested to knowing if DALL-E 3 will allow that kind of control. [00:19:08] And most of all, finally, we had DAL E2 very early in the game, before Stable Diffusion even gave the first clunky models, before everything, and there was so much work and mid journey. And so many much interesting things coming out in image generation and open AI will always like hanging back. [00:19:30] We have this very basic value too, which sometimes works and usually doesn't gives you very weird results. So yeah, good to see that they are still working on actually [00:19:43] innovating [00:19:44] and thinking of the next step and how we can combine all of these technologies. To make something that's much more fun to the user experience. [00:19:53] Alex Volkov: Absolutely. And I will remind some folks the internals behind kind of diffusion models, like stable diffusion, et cetera. OpenAI actually made the whole field happen, I think, with some was it VIT? Vision Transformer that they released and, [00:20:05] Yam Peleg: they released the first diffusion. The first diffusion model. [00:20:08] Alex Volkov: Yes. And so like the whole field is all to open the eye and it's great. I, it's a fair, I joined you in the, it's super great to see them innovate and give us some new UIs for this because. I heard from multiple people who have access to this, that this, you can get lost in just chatting to a picture, to the creation process. [00:20:26] It's like a whole new creation process, basically, like prompting, but chatting. I'm very excited about this, very excited. [00:20:31] , so we'll definitely talk more about this. [00:20:33] Microsoft c launches Windows CoPilot [00:20:33] Alex Volkov: I want to move on to the next thing, which is exciting. And so. Until today, basically, the word co pilot meant GitHub co pilot, at least for those of us with VS Code, those of us who write code. GitHub co pilot obviously is the auto complete engine that, gives you code abilities. [00:20:50] And many of us use it, many of us don't use it. But, today, I think, Microsoft who owns GitHub and who is very close with OpenAI has announced Copilot for Windows. And it's coming soon with the Windows update. And we've seen some previews about this in some discussions. And I find it very interesting that Microsoft is innovating in AI, whereas we're waiting for Google to come up with Gemini. [00:21:18] We're waiting for Google to, we're going to talk about Bard updates as well. But Copilot for Windows will be able To be just like a shortcut away. I think windows C is the new shortcut and you'd be able to ask it like he asked you for different things. And for those of us in the audience who didn't join us in the previous ThursdAIs, we. [00:21:40] Talked with Killian from this open source called Open Interpreter. And one of the things that we all like in Open Interpreter is that it runs on my machine and it generates code, and some of that code could be AppleScript. And so it's very easy to run stuff on the Mac using AppleScript. You can open Calendar, you can send emails, you can do a bunch of stuff. [00:21:58] And so it was beautiful to see that, like, even an open source agent like Open Interpreter is able to Run code and then, activate stuff on your computer. Having, and I think Kilian mentioned, like, Microsoft's Copilot is coming. And not just a week later, exactly a week later after that discussion, we now have Windows Copilot. [00:22:16] Which is going to be able to run Windows for you. It's going to be able to open apps and shut down apps. It's going to be able to just like... Be a, chat GPT, but living inside windows. And I think it's going to be based on GPT 4. It only makes sense with the Microsoft OpenAI collaboration. And like I can't understate this for a second. [00:22:38] GPT 4 was released on March, right? Chat GPT was released less than a year ago on November something. And now the next version of world's probably most. Common operating system, Windows, is going to have AI built in as a companion. How insane is this, folks? I, I, I, I have a Windows machine, because I have an NVIDIA GPU, blah, blah, blah, and not only I'm not only on the Mac and I'm really excited to, like, play with this. [00:23:09] An additional thing that they've announced together with this update is connecting to the previous thing that we said, which is Bing, Chat, and Windows Copilot will both have DALL-E 3 built in for free. So DALL-E 3 is going to be possible on GPT Plus subscribers, the ones of us who paid the 20 bucks. [00:23:32] However... For, through Bing, you'll be able to get it for free, and it's going to be part of Windows. Right, so, my mom, who probably doesn't use Windows, okay, her husband, my mom's husband uses Windows, he'd be able to use GPT 4 to run his Windows and also generate images. I think that's incredible, and, only Microsoft can give it out for free. [00:23:52] I think that's mostly it in... The Microsoft update. However, it's breaking news. Literally, they released the tweet once we started the space So I'm sure more stuff will come out of there But I invite folks on stage to chime in with Windows Copilot news What do you think about this whether or not, you know This is going to change multiple people's usage of Windows or Or not [00:24:16] Nisten Tahiraj: I mean the whole Using software thing is all up in the air now, right? Everyone's in creative mode. Yeah, it's pretty hard to predict what's going to be the, the better interface voice is getting really good. Open interpreter show that it can do a whole bunch of stuff. You can also delete all the Jason files on your computer accidentally, but I think those, those will be worked out those issues. [00:24:43] Yeah, it is hard to, it's hard to call because again, being is still a free beta service, they haven't quite figured out how to fully monetize that, because that's not cheap to run especially considering that it is the multimodal image one, so. Yeah, don't have that much an opinion. [00:25:05] I think it's still too early to call as to how interfaces will change. [00:25:09] Alex Volkov: I agree. I just, I'm excited that AI that we've come to known for less than a year is now baked into an operating system for everyone, right? Even going to a website like chatGPT registering is not for everyone and they will. They will definitely , lower the bar for usage here. What's up, Yam [00:25:28] Yam Peleg: hi I just want to say that we've seen, because everything is so early, we've seen really great infrastructure for RAG but we haven't seen a wide scale product using RAG on this scale. So, and, and it makes sense at the end. [00:25:47] I mean, you have a lot of information scattered around all different software and different devices. It's, I think it's the perfect idea to just merge everything with the RAG and just allow you to chat with whatever information you have everywhere. And Microsoft is perfectly positioned to do that. And I'm looking forward. [00:26:13] I think that I think it's a great idea. I don't know if the implementation. Will be great. It's, we need to see, I think it will, but we need to see, but I think that's it. As a concept is a great concept. [00:26:26] Alex Volkov: Something that I saw from a person who's very close with the Microsoft team, for some reason, the guy behind being his name is Michael Perakin, and he has this like very non branded Twitter account that barely has an avatar image. [00:26:43] And he's been doing, he's open. Yeah. He's been doing, he's been doing like customer support basically on Twitter. Like people will say, Oh, Bing has this, has that. And he's like been very, very responsive to some people. And so two things that he did say, first of all, Dally three is already part of Bing for some percentage of population. [00:27:00] So if you use Bing, and we've talked about Bing before about image and vision. If you use Bing, go try and generate images with it. It used to be Dally too, but if you get. Good ones. You may get value three, which is incredible. You may already have this. And the second thing is I saw somebody commented that he is now head of windows, right? [00:27:17] So the guy behind being the guy who pushed a I into being is now moving to be ahead of windows. And I think this together with this release shows us that. How just how much Microsoft is serious about a I everywhere and is determined to not miss this new wave like they missed the mobile wave. And everybody says that, Apple overtook Microsoft and Microsoft was like late to mobile. [00:27:37] And And it just goes to show like how much they invest in this whole thing. And I find it like very, very good because for many people, even going to a website is a barrier of entry. And then when it's just like one click in their operating system of choice, I think it's going to be very it's going to shove AI into way more people's faces. [00:27:54] I also want to say that Microsoft out of the big ones is fairly based in terms of. Safety and regulation, which we usually don't talk about we can talk about in maybe the next space, but like, we can have worse than Microsoft, which is surprising for me because I used to hate on the Internet Explorer most of my life. [00:28:12] And so now Microsoft is very based. I think less comments on Windows Copilot here, folks, and then we can move on to the next stuff from OpenAI, actually. [00:28:22] Nisten Tahiraj: So my last one is I've started using Edge Canary as my daily browser just because of the sidebar and the splitting. So if you have a widescreen monitor, it's actually very handy because you can have code interpreter on one side, and I'll show an image of it very quickly. [00:28:39] And I have Bing, which has an excellent voice back and forth. And it has really good voice generation, which normally would be very expensive if you're paying for it, but it's in beta And then I have the actual work and on the sidebar you can have Anyway, this interface is a bit convoluted and edge browser is it's still a little bit clunky, but Overall, it's been working pretty well for me. [00:29:06] So I I don't know. I sort of see the browser as being more and more important. That's your operating system. Some people disagree. They're trying like Sean is, is trying to do more of a OS native stuff with his tool that lets you run multiple ones. But Yeah, you can see the screenshot of how I started using it with voice, so. [00:29:28] In general, I see it as you'll just talk to it back and forth. I think That's, [00:29:32] Alex Volkov: at least that's what I want. Were you referring to Swix's Godmode app where you can run all the LLMs in like a window? [00:29:39] Nisten Tahiraj: Yes, but that one, for example, on the Mac is right, there's an icon right beside the clock. And you just click that and it pops up, so it's unintrusively there. [00:29:49] And it adds to your experience instead of getting in the way. And I, I do like that part because it is using real estate on the screen efficiently, but again, if you have a. If you use a wider monitor, so can Edge with all of its right sidebar shortcuts, because then you can add your discord, your outlook and stuff there too, right where the GPT like right where I use the code interpreter window and even have some completion and document writing stuff too now. [00:30:19] So that's how I see it. I, it's again, it's up in the air, what people will find most helpful [00:30:25] Alex Volkov: absolutely. And I've been using Bing somewhat as well. And yes. The sidebar can also read from the page, right? So the Bing chat in the sidebar has access to the page if you give it. [00:30:37] And that for like summarization and different things, that's really, really excellent as well. Like it completes your browsing experience. So I'm assuming that they're doing some stuff with the co pilot. [00:30:46] Open AI leaks, GPT-4 Vision, Gobic [00:30:46] Alex Volkov: All right, folks, we're moving forward because we have much to cover. And, there's more news from OpenAI. [00:30:52] They actually came before DALL-E, and we were supposed to talk about them first, and then DALL-E, but sorry, and then DALL-E came out. And now let's cover some news from OpenAI. So... It feels like the theme behind all of these news is OpenAI is trying to rush stuff to the door or to announce some stuff to the door because they know or they hear or they saw the information from Google breaking out about Gemini, the multi model wolf. [00:31:19] Huge model from, from Google that is potentially GPT 4 like and can do images in the input and output is multimodal on the output as well. And so we don't know many sorry, we don't know much information about Gemini so far, but we do know that the information kind of the publication called the information released that Gemini is coming very soon. [00:31:40] And we see the response from OpenAI in multiple places, right? So DALL-E 3 is one of them. OpenAI released so the information also leaked. about open the eye gearing up to give us vision for those of you who remember pretty much every space since march we're talking about gpt4 that is also multi model on the input and yeah we can probably go into the details whether or not it's fully multi model versus gobby and i would love for you to participate in this but basically gpt4 when they announced they showed the demo of it they gave it some screenshot they gave it like a sketch of a website that was able to code that and then we didn't get That feature, the Multimodality from GPT 4, we didn't get it. [00:32:20] The only people who got it, and me and Nisten interviewed the CEO of this, is Be My Eyes, which is this app for blind folks, and they just like shove GPT 4 vision in there to help those with eyesight issues. And it seems that now Google has finally stepping into the arena, sorry for the pun, and that we may get GPT 4 vision very soon. [00:32:42] I actually saw some screenshots how it looks inside the GPT 4 chat GPT interface. And the additional exciting thing is, they have a different model. With the code name Gobi, that as apparently it works in OpenAI. And that one is going to be multi modal and like fully. So, Yam, I would love to, if you can repeat what we talked about last night, about the differences and how GPT 4 is multi modal, but not fully. [00:33:06] I would love for you to expand on this. [00:33:09] Yam Peleg: Yeah. First it's important to understand that there is a huge difference in infrastructure between the two companies. And the infrastructure dictates what is possible or not possible, what is hard or not hard. From the rumors nothing is confirmed, but from the rumors the, the structure and the size of GPT 4 is. [00:33:34] It was chosen to fit the hardware, the infrastructure to actually run the model. It doesn't matter if you have the best model in the world, if you cannot just serve it. So Google is using its own hardware, which is not sharing with anyone else. And it's important to understand this. So when we see that Google is doing according to the rumors. [00:33:58] And, and insane training run or, or preparing to ship or, or serve an insane model that is multimodal on the input and on the output. It, the reason we didn't see, I think, I think the reason open AI I didn't release a GPT with the image head is simply because it's. It's probably expensive. It's not that easy to deploy something like this, especially not with the amount of people that use OpenAI services. [00:34:31] And, and I think this is this is what we see. This is the reason for what we see at the moment. Now it's important to understand that according to rumors, again, nothing is confirmed, take with a grain of salt, according to the rumors, which makes sense, GPT 4 is first a language model. It was trained as a language model, just language model. [00:34:53] And once it was trained. It, there was they added an image head to the frozen model. This basically, this reduced the risk of something going wrong with full multimodality end to end. And moreover it allows you to just use the model on its own. And if you want, you can plug the head so you can use them. [00:35:14] You can, it's flexible. You can use them with or without a head. Now, the thing is that there is you do pay a price because again, with a grain of salt, there, there is there is, there are caveats to this, but we have already seen multiple times that multimodality, when done right, benefits both modalities. [00:35:36] So GPT 4 allegedly did not benefit from the multimodality. And this is the difference between GPT 4 and the new rumored model that we have. According to the rumors, the rumored model was trained end to end images and text throughout the whole training. So, we should, if it's true, if everything is true we should expect a better model only if you just use it for text, we should expect a better model because the, the images just influence the text and text influence the images and So on and so forth. [00:36:12] Alex Volkov: That's great. That's what I have. One follow up question. You spoke about benefits from training on text and vision. And I remember Ilya Asatkov also talked about this. I think with the Jensen CEO of NVIDIA. He talked about different other places. Could you speak to some of those potential benefits of how multi model trains on text and images is actually better? [00:36:37] Yam Peleg: If I remember correctly Ilya said Ilya gave the perfect example for this. You can, if you really want, you can describe what the color red mean with text or what, what objects are red. All of this will be nothing like just seeing the color red. So there is a difference between actually training on images. [00:37:04] Versus training on text that describe the images which is just, it's just a different sensation. So the whole you can say the world model inside the, the language model is influenced by, by the images. And I think color is, is just a great example. And if I remember correctly, that was Example he gave in this interview. [00:37:27] Alex Volkov: Yeah, absolutely. And I think the other one he said is It's obviously better at stuff like math or physics where it's able to actually read different, the graphs and everything. It's like, it just arrives at the question faster, but also like Yam you correctly pointed out the world model of this model is way better because it's able to see basically. [00:37:50] So We have potentially exciting news. One thing I will add is that Yam I think you're correct opening. I just didn't want to spend the kind of this GPU cycles on the vision model and the being able to attach a head with vision. I think it's exciting. I do want to highlight that Microsoft likely has. [00:38:08] The bandwidth for that, because being has the ability to have vision. Now, I don't know if it's like the full one. I don't know if they did some work because the examples that I tested with being vision gave less quality like responses on images than I was expecting GPT four from the example. [00:38:25] So maybe they know if they, maybe they did some stuff for optimization speed, but yeah, definitely it feels like infrastructure was gearing up for this and hopefully we'll see it soon. From OpenAI. [00:38:36] 3.5 instruct model from OpenAI [00:38:36] Alex Volkov: Another thing we saw from OpenAI, and I think this is this last one, we have a bunch of OpenAI updates, is the 3. [00:38:42] 5 Instruct model. And unlike the ChagGPT model, 3. 5 Instruct is very similar to how OpenAI APIs We're actually working before the ChatGPT explosion, right? Before you were able to like do back and forth conversation. Before it was RLHF for conversation purposes. And I saw many, many folks get very excited about 3. [00:39:05] 5 Instruct. Because it's very similar to what we had before ChatGPT. But it's much faster. Now we don't know if it's faster because way less people use this because it's new. Or it's faster because they actually did some TurboMagic on it. But, we'd love to invite folks on stage, maybe Roy, maybe Mr. [00:39:21] Yang to talk about the instruct and the difference between kind of this end point in the API versus the regular chat end point. If you have anything to, to, to, to add here from what you read, please feel free to, to add. [00:39:36] Nisten Tahiraj: I used it in the playground to just like write An agreement for the site, like a privacy agreement. [00:39:41] It was pretty good for that. It just it's annoying that the context window is so small. It's only a 4k context window. And it's more like only three and a half K because some of it will be your prompt. I think it has some very other very good usability uses, which we haven't experimented with yet. [00:40:02] Like the one person got it to play chess very well. And I think it's, yeah, it's really worth looking at for stuff like doing automation or you're continuing some work on your desktop, for example, with open interpreter, and it'll be able to continue generating in that regard. So there are quite a few things to explore there. [00:40:26] I'm just glad it's cheap and it's good. So that's that's what we want at the end of the day [00:40:34] Alex Volkov: Yeah it's it's cheap and I think for many folks they were surprised with like the chat interface They had to switch for chgpt to get like the benefits and the speed and now they're happy that they have the instruct model of old They also added log props. [00:40:47] So I, I would love to ask folks on stage because I'm not entirely sure what like logprops is in the API response. And I saw Alex Gravely and some other folks are getting excited about logprops. And I want to say, just before, I want to say hello to Ronak, if I'm pronouncing this correctly. Ronak. And we're going to talk about RamboRole in a second, or in a few minutes, but if you have comments on the Instruct API and LogProps, feel free to share. [00:41:18] Raunak Chowdhuri: Yeah, I do. LogProps is awesome. It basically gives you like, token level probability distributions on, in terms of the model. So normally when you are using GPT 4 or GPT 3, You just get words back when you're, when you're querying the model. But what LogProbs allows you to do is, is see the probability distribution that's outputted by the model that is normally sampled by, like, the temperature parameter. [00:41:43] And you can use that to do a lot of, like, really interesting things. Like, for example, if you're, if you're asking GPT to solve a multiple choice question, for example it's really useful to actually understand. the model's confidence in whether it's A, B, C, or D. And you can actually get that directly from the model by examining that probability distribution from the log prop. [00:42:05] So it actually provides... A lot more insight into what the model is thinking and I think that's a pretty useful technology. You can actually do a lot of clever things with it, like someone built something called like JSONformer which is basically like a tool that allows you to if you have a model that exposes log props, you can only sample the tokens. [00:42:24] That basically are valid JSON tokens, and construct a response that is very much aligned with like a certain format that you want. So I think that's a pretty powerful tool. [00:42:36] Alex Volkov: Thank you, Ronak. Thank you. And I remember JSONformer and did not know that they use log, log prox for that. So here you have it, folks. [00:42:43] There's a new endpoint for you, your usages that now exposes the token probabilities. So you can use this to build better tools and different types of tools. And yeah, Ronak, would you care to introduce yourself briefly? I will ask again once we record kind of your section, but feel free to introduce yourself. [00:43:03] Raunak intro [00:43:03] Raunak Chowdhuri: Yeah, absolutely. So I'm a senior at MIT. I'm graduating in a couple months. My background's in machine learning, artificial intelligence. I've been doing research in this area for quite a few years now. Yeah, I'm working on some interesting projects that we'll dive into later, but basically building long term memory for Language models. [00:43:25] Bard Extensions allow access to GMail, Youtube, Drive [00:43:25] Alex Volkov: Awesome, awesome. Thank you. Thank you for coming up and thank you for explaining log, log props as well. All right. So the next thing I want to talk about briefly, really briefly, because it's not that great is bard from Google. Before we get to Gemini, before we hear from Googles like Explosive, GPT 4, Combating Model, etc. Right now we have Bard. For some reason we also have Google Assistant, which I'm not sure what's the involvement with LLMs there. But Bard is something that some folks on stage here use. And I was never like very, very excited about Bard for some reason. [00:44:00] However, they just released a few updates to Bard and they say like this is the best Bard ever. And it feels like very googly, very like product manager y to me, at least. What they released is something called extensions, right? So if you use Bard before and you haven't touched it in a while, like I haven't, if you go to Bard right now, what you will have is the chance to... [00:44:22] Updated with extensions. Those extensions could access your Gmail, all of it, your Google Drive, all of it, YouTube. I think some other ones that I'm trying to remember. And the cool thing about this, which I actually like, is the UI. You can do at sign, like like you mentioned somebody on Twitter. [00:44:38] And then you have access to those extensions. It's a different take on the plugins with ChagPT, where like ChagPT plugins, you have to be in that mode, it decides for you, blah, blah, blah. So here you can actually say like, add Gmail, and then ask it questions. It will actually go and do a search in your Gmail account and give you back answers with, with natural text. [00:44:56] So. Conceptually pretty cool, right? We all use Gmail, or like at least most of us use Gmail. And so to be able to like get summaries of the latest emails, blah, blah, blah. So conceptually very cool. Google Docs as well. You can tag Google Docs. You can do Google Drive. Oh, Google Maps is the, is the other one. [00:45:10] So you can actually say like, hey, what are some of the stuff that, in San Francisco, Seattle, whatever it will give you. The thing that I was really surprised by is just how bad it is, just honestly not to... If there's folks in the audience who work on Bard, I apologize. And sometimes we say these things, but there's like so many, so many people working on this stuff. [00:45:31] And like, it's, the, the nights and weekends, they don't see family. So like, I apologize. Just network from the comparison point in my experience, I was really disappointed in how... Google, who's this like huge company that like created Transformers for us, like they, they're not afraid to release something this bad. [00:45:50] And what is bad, I mean, specifically, I literally used two of the extensions. One is Gmail. To ask it about my upcoming flight to San Francisco, which I told you guys about. I'm going to be at the AI engineer event as, as a media person. And it couldn't find any information from this flight and just gave me flights from the past. [00:46:07] I literally asked, give me flights from the future or like, give me my upcoming flights. And it gave me flights from the past. It also gave me two trips to the Denver museum, which is, which are not flights. And so, yeah, we know LLM hallucinates, blah, blah, blah. But if you, if you put your brand behind this and you're Google and you put Gmail in there and you cannot like do a basic search, that's upsetting. [00:46:30] And so I said, all right, I'll give it another try. I did YouTube. And I asked, Hey, what does MKBHD, Marques Brownlee, if you guys don't follow him, he's like this great tech reviewer. What does he think about the latest iPhone? And it went to YouTube and it searched and it gave me. Marquez's videos from last year about the iPhone 14, and I literally took the same string that I pasted into Barg, went to the YouTube interface, pasted it in the YouTube search, and got like the latest videos that he had about the iPhone 15. [00:46:58] And so I was thinking there like why would I ever use this if like the first two searches did not work, where this is the whole promise of this. So again, not to be negative. I don't love being negative. It's just like from a comparison standpoint. It's really I really got to wonder how many folks in Google are trying to rush through the LLM craze. [00:47:19] We remember Sundar Pichai saying, AI, AI, AI, AI, AI, AI, AI, AI, AI, on the stage like 48 times, right? And they're shoving AI into everywhere. It's just, for me, it wasn't that useful. So I would love to hear, Safrir, I see your hand up. I would love to hear from folks on stage about your experience with BARD and those specific kind of extension new things. [00:47:41] Tzafrir Rehan: So I don't have much experience with it, actually, for the same reasons that you said. But I want to give the perspective that I think what we're seeing here is Google jumped early to stay in the game. Maybe they didn't expect ChatGPT to go viral that big so fast. Well, this was developed like a sci fi technology and suddenly it's a household item overnight. [00:48:09] But, if you're talking about Google, and I worked at Google actually for three years, about a decade ago, it's a company that can make very big moves very slowly. That means, if Gmail data, Drive data, it's the holiest of holy of privacy. If you want as an engineer at Google, if you want to touch that data to read even a single bite, you need to go through quarters of legal meetings. [00:48:41] So the fact that they are going in this direction indicates a course heading that they took the time to think of it through and decide, yes, we are doing this very risky move in terms of privacy and user expectations. Because they believe in the value. So let's see where they get to when they actually, when they are actually fully implemented. [00:49:05] Because I think right now, what we are seeing is a rushed out version. [00:49:09] Alex Volkov: I agree. I think that's how it definitely feels where the basic stuff, like. A keyword search works better than like this search and they're basically hitting the API, which they have behind it definitely feels rushed very polished UI wise, very safe, very like protective, like googly, but very, it's not super helpful. [00:49:27] I think at this point Yeah, I think this is most of the news unless I'm missing some so let me look and see in my template that I already Drafted for myself. Let's see if we have any more things to cover before we before we move on to the interviews So yes, one last thing I wanted to find I'll just find this thing. [00:49:48] It's called chain of density So, I saw this, I think it was a paper first, and then yeah, I'll share this in the chat. I'm sorry, not in the chat, in the, in the Jumbotron. I saw somebody release a paper on this, and then I think Harrison from LangChain reposted this and actually put it up on their website with the prompt sharing, where you can play with prompts, is this new method called chain of density, which is actually really, really good at getting summarizations from From ChatGPT and different other places like Cloud as well. [00:50:21] And I think it's really cool because I just posted it on top. It it asks for four summarizations with more and more density, right? So it starts with like, hey, summarize this text or article. And then it says give me like a JSON file in response with like four summarizations. The second [00:50:37] one, give me a summarization. [00:50:40] Extract from the first one that you just gave me, extract the entities that were missing, and give me another summarization with those entities, and then do it again and again. And I think there's, like, some cool prompt magic in there that says something to the tune of, make sure that this is understood on its own, and the person doesn't have to read the article to understand the summarization. [00:51:00] I personally have gotten really good summarizations based on this technique, so much so that I've added it to my snippets. Where, where I have different snippets for prompts. And if you are doing any type of summarization, definitely check it out. Nistan, I saw your hand briefly up if you want to comment on this thing. [00:51:16] Nisten Tahiraj: Yeah. Like the first person that I knew who got a contract as a prompt engineer actually used this technique a lot last year. And the way he was explaining it was when you do, when you compress an idea and then you extrapolate, that's how creativity happens in general. Like you, you compress. You extrapolate out of it, you compress and then you extrapolate. [00:51:36] So it's pretty interesting that someone did this in a much more systematic way. I'm, I'm going to check it out. [00:51:43] Alex Volkov: Chain of density. And I wanted to ping back real quickly on the compressing part, because yeah, I saw your tweet and there was a paper about compression as well. And Ilya gave a talk about compression recently. [00:51:55] And I wanted to see if you want to talk about that compression part and paper. Briefly and if not, that's also okay. We can move on but I just like I think this is also this week. [00:52:07] Yam Peleg: Yeah, I got I Had some controversial opinions in the last couple of weeks And as it turns out there are papers that support them coming up after them. [00:52:19] But yeah, I highly, highly suggest reading the compression paper. Basically, basically what it says is that it just it just conveys the idea that what, what we are actually doing is I want to say. Reversing the process that generates the data and by reversing the process that generates the data. [00:52:39] If you think about it, the process that generates the data is us. So, I don't, I don't want to, I don't wanna say the, the the words that I shouldn't. I got a, I got some heat for them, but you can find in my tweets. It's it's a really good paper. It's really It's much more scientific, you can say versus other papers that talk about intelligence, about general intelligence, and poke on this idea, and I highly recommend reading this paper if you're interested in this part of what we're doing. [00:53:13] It doesn't prove anything because, general intelligence is a, is a big thing, but it. It is it is interesting the ideas there are, are, are solid and great to see. [00:53:24] Alex Volkov: Yeah, I, I, I heard this multiple times this comparison or metaphor that intelligence is compression and, compressing a lot of ideas into, First of all, it compares to natural language, the ability of us to understand something, to put it into words, that's compression. [00:53:39] Obviously, Feynman's quote, where like, you really understand something if you can explain this to a five year old, is also like, compressing down and also being able to explain some stuff. And so I heard this multiple times, and it's great to see that, there's now papers to talk about this. And continuous compression, like Nissen said, actually... [00:53:54] Actually brings out better results and it's also good to see on the topic of literal compression. I know this like it's confusing There was also another paper that's worth checking out from this week Where they actually used llms and different transformers for an actual compression to compare to like png or or jpeg, etc And I think they saw very interesting compression results as well I don't remember if I have a tweet for that But yeah, be on the lookout for the for multiple types of different compression, uh as we as we move forward Thank you I think With that, I think we are ready to move on to our guests here on stage and to talk about two exciting things. [00:54:30] So, first of all, actually three exciting things. One of them is, Nisten you had a visit to Geoffrey Hinton's lab that I wanted to hear from you a brief story about. After that, we're going to talk with Arthur and Zenova about WebGPU and going to do like a brief interview about like... Running models locally in the browser. [00:54:47] And then at the end, we're going to talk about remember all with Ronak and his exciting approach to extending complex windows. So with that, I'll just give a brief kind of summary of the spaces we had today and some logistics, and then we can get started with the second part of Thursday. So again, everybody in the audience, we're just drawing the joint in the middle or have joined us from week to week. [00:55:08] Thursday is about. Staying up to date together and give giving updates every week so that folks don't have to follow everything because it's almost impossible. I'm very happy to be joined by multiple folks from different disciplines and folks who can answer stuff and complete and find new things to get excited about about AI. [00:55:28] From different fields every week here on stage. We also have a podcast and newsletter. If you're here and you're new and you just like just joined us and you can join next week, you can sign up for the newsletter as well. We stay up to date. So you don't have to, this is the model. And the first part of this is usually updates from last week, breaking news. [00:55:46] There's another breaking news with YouTube something, but I think we'll cover this next time unless folks here want to read up on this and then give us an update at the end. But the second part of it is usually A deep dive into different conversations and, and guests. And today we have Arthur and we have Ronak to, to talk about different, very exciting things. [00:56:05] And we'll start with Nistan's brief foray into the lab, AKA yeah, Nistan, give us, give us like a few minutes on, on your, your excursions. [00:56:16] Nisten Tahiraj: Well, I've been going as a guest to Vector Institute for. Over a year and a year and a half and this time I, I went in and I'd never met Pharrell in real life. [00:56:28] I didn't even know what he looked like. It was just some dude. He was GitHub. And yeah, so I, I invited him in and we were going to work on making the bootable. Bootable OS that just boots straight into a GDML model and then hopefully gets Chromium running with WebGPU. And essentially I just, I made before a, a tiny 4. [00:56:54] 7 gig ISO that includes an entire Llama 7b model and an entire Linux distro. I use Slackworks, that's the smallest, and I used that for like 20 years. And yeah, so we were in the lab and Eventually, he's like, let's just try and get the whole thing working. So let's just try and get the mixture of experts. [00:57:14] Let's just do it all at once and see where, where we get stuck. And anyway, I had to call another friend who was an extremely good DevOps engineer. To help and, and yeah, anyway, long story short, I couldn't get it to run on the GPU because there were no instances and I only had an A10 24 gig and MixtureFX needs more than that because it's 32 experts. [00:57:39] So I had to run it on the CPU and that's what we spent the entire day and evening on. And it was really slow, but then we realized, yeah, this is probably like the first time someone has effectively ran Mixture of experts model on on, on a CPU. And again, it's, you can check out the REPL. [00:57:58] I made a CPU branch and it's the V1 branch if you really want to get it to work. But yeah, that was the story. I just met with a random person from Twitter for the first time who was in their discord and yeah, it was, it was fun. And we also, the funniest part was that. Happened to be there a call in Rafael, who has been teaching about mixture of experts and writing a lot of the papers, and then we look behind and he's just like literally like five dusks away. [00:58:30] And I was just like, taking a back. It's like, Oh, holy cow. He's here. And he had no idea who we were or anything. So, yeah, that was that was fun. [00:58:39] Alex Volkov: There, if you don't mind me completing this story from what you told me multiple times, because I think it's like way more colorful than you, than you let on. First of all, VectorLab is the lab of Geoffrey Hinton, the grandfather of AI, right? [00:58:52] This is the lab. This is like, he's widely considered the person who like, have kickstarted this whole field, basically. Is that, is that, that lab? Was he there? [00:59:02] Nisten Tahiraj: Yeah, yeah, yeah. Ilyas Iskever has been a student. He wasn't there. He's rarely there. He only has like one PhD, one student under his wing this year. [00:59:12] So he comes in very rarely. But yeah, Ilya Suskever was not in, in the smaller lab before they moved here. Also Adrian Gomez, the, one of the writers of the Transformers paper still comes there every once in a while. He was there regularly up until Cohere got funded last year. And yeah, this is, this is the lab and it's it's pretty funny because everyone's very, very academic and we're just straight up hacking and whatever we can find. [00:59:45] Alex Volkov: So the second thing that I wanted to cover here is that exactly what you built in the lab of Geoffrey Hinton, because He's now very public about the AI kind of doomerism and AI different kind of potential bad things that will happen with AI and how to not open source, how to regulate. He's very public. [01:00:04] He's on every news. And here you are, you and Pharrell are working on an ISO, a bootable AI disc that you literally can run offline that has Lama and offline LLM. The, that basically will say, even if they regulate, you can just like take an old. CPU based machine and run this thing. So you basically get democratizing AI in the lab of the person who's now like very, very vocal about like stopping it. [01:00:27] So that's, that's the second part that I personally like very enjoy. [01:00:31] Nisten Tahiraj: It's not just that. Also, if you listen further than what the news media. Shows it's a lot more complex than that. He, he wants people to acknowledge that the risks are real and show that they are mitigating them. But at the same time, he's been doing research to do molecularly grown chips. [01:00:51] And that architecture first didn't work. So. They're still going full speed ahead. They're just making, the reason that they went that way was just saying to a lot of the community, just don't act like idiots, just regulate yourselves. That, that was why they were challenging that. [01:01:09] It's it was a lot more complex than people realize. And the professor there, Colin, he's been a big pusher for demarketizing and open sourcing. Model C in general, and so, yeah, it's a lot more, it's a lot more nuanced than what you see in the media, and when you think about it, the safest form of AI that you can have is one that you can just literally unplug, and you have full control over, so there is nothing safer than that. [01:01:40] Otherwise, you're just trusting some incompetent politician with regulatory or whatever legal hacks to control it. So, it's yeah. It's a lot, I want to say, it's a lot more nuanced than people, than what you've just seen in media snippets and reactionary Twitter checks. [01:01:58] Alex Volkov: Yeah, I hear you. And definitely we'll, we'll, we'll check out the nuances and Jeffrey Hinton on the topic very briefly before after our apologies, we'll get to in just a second, just like something that also happened this week. Yan Likun, the GOAT, aka, the chief meta AI chief scientist, well, went in front of the Senate. I think a couple of days ago, and he, I just pinned the tweet on the top that he actually retweeted, which was like, sent by notices, and he gave an incredible opening statement, talking about how open sourcing is very important, why the open source LLAMA, talking about the fact that, the open source LLAMA1 and the sky didn't fall, and all of these things, and he also outlined a bunch of the safety protocols that they have into account when they release LLAMA2, and I think it's a First of all, very important to have somebody like Jan in front of Senate and talking about legislators and regulators and about regulation, because we see more and more Jan. [01:02:52] I think you brought up last week about there was another discussion and Elon Musk was there and Sundar Pichai was there. Everybody was there talking about AI and how to regulate. And I think it's very important to have voices like Jan LeCun talk about like, talk with different things with clarity and safety. [01:03:07] And so definitely. Recommend everybody to check out his opening statement because you know the doomers it's very easy to scare Especially on like the engaged baiting networks like x and etc It's very easy to like take something that people don't understand use it to scare folks And I think it's very important to have very clear very Credentialed and very like, understanding people from this world to actually explain that there's benefits and explain how open source can benefit as well. [01:03:36] And I think you also mentioned how excited the open source community was about the Lama to release. And I want to believe that we all had like a small, tiny part to play in, in this. And so, yeah, we're definitely on Yam's map sorry Yam Likun's map and definitely worth checking this out. I think with that listen, thank you for sharing your story. [01:03:52] Tell us more escapades from vector lab. And if you get to meet Geoffrey Hinton, tell him about Thursday night and also Colin. [01:03:59] [01:03:59] Alex Volkov: All right folks this actually concludes the two hours that we've allotted for thursday night today I I know there's like many folks. I see dave in the audience. What's up, dave? I haven't I see. I see other folks just stepping in with, with all the sadness of, I want to keep talking with all of you. [01:04:20] There's also now a need to, transcribe this and, and, and put this into a newsletter, a podcast form Thursday is here every week. We're here literally every week since GPT four came out, I think. Mr. Did I miss one week on vacation? Yeah, newsletter came out, but we didn't talk that week. I felt like, oh, I miss my guys. [01:04:37] I miss my friends. We need to get up to date together. So we're here every Thursday. I there's so much always to talk about. I want to just like to highlight how much boring this would have been without friends like distance and Nova Arthur, now the new friend of the pod, it's a freer and some other folks who stepped away young and far and for real, like many, many other folks who joined this week to week. [01:04:58] And Help us bring you, the audience, the best AI news roundup possible on, on X slash Twitter. Now almost six, seven months already into this. This has opened many, many opportunities for many folks on stage, including myself. I'm going to the AI Engineer Conference. As a media person, I'm going to do some spaces from there. [01:05:19] If you're in the AI Engineer Conference in a couple of weeks, definitely reach out and, we'll talk over there. With that, I want to just say... Without the audience here, this also would be very, very boring. So thank you for joining from week to week. Thank you for listening. Tuning in. Thank you for subscribing. [01:05:34] Thank you for sharing with your friends. And thank you for leaving comments as well. And with that, I wanna wish you a happy Thursday. I, I'm sure there's going to be many, many, many new things we're listening just today. But you know, we can only cover so much. With that, thank you folks. Have a nice rest of your Thursday. [01:05:49] I, and we'll meet you here next week. And yeah. Cheers. Have a good one. This is a public episode. If you'd like to discuss this with other subscribers or get access to bonus episodes, visit sub.thursdai.news/subscribe | |||
29 Sep 2023 | 📅🔥ThursdAI Sep 28 - GPT4 sees, speaks and surfs, Cloudflare AI on GPUs,Mistral 7B, Spotify Translates, Meta AI everywhere, Qwen14B & more AI news from this INSANE week | 01:41:01 | |
[00:00:00] Intro and welcome everyone [00:00:52] GPT4 - Vision from OpenAI [00:05:06] Safety concern with GPT4-V [00:09:18] GPT4 can talk and listen as well [00:12:15] Apple rumors, on device inference, and Siri [00:17:01] OpenAI Voice Cloning Tech used in Spotify to translate podcasts [00:19:44] On the risks of Voice Cloning tech being open sourced [00:26:07] Alex statement on purpose of ThursdAI [00:27:53] “AGI has been achieved internally”; [00:32:10] OpenAI, Jonny Ive and Masa are rumored to be working on a hardware device [00:33:51] Cloudflare AI - Serverless GPU on global scale [00:37:13] Cloudflare AI partnership with HuggingFace to allow you to run many models in your own [00:40:34] Cloudflare announced the Vectorize DB and embedings on edge [00:46:52] Cloudflare AI gateway - proxy LLM calls, caching, monitoring, statistics and fallback [00:51:15] Part 2 - intro an recap [00:54:14] Meta AI announcements, bringing AI agents to 3 billion people next month [00:56:22] Meta announces EMU image model to be integrated into AI agent on every platform [00:59:38] Meta RayBan glasses upgraded to spatial computing, with AI and camera access [01:00:39] On the topic os smart glasses, GoogleGlass, and the acceptance society wide to have [01:05:37] Safety and societal implications of everyone having glasses and recording everything [01:12:05] Part 3 - Open Source LLMs, Mistral, QWEN and CapyBara [01:21:27] Mistral 7B - SOTA 7B general model from MIstralAI [01:23:08] On the topic of releasing datasets publically and legal challenges with obtaining that [01:24:42] Mistral GOAT team giving us a torrrent link to a model with Apache 2 license. Truly, as I’ve been doing these coverages in one form or another for the past 9 months, and I don’t remember a week this full of updates, news, state of the art open source models and more. So, here’s to acceleration (and me finally facing the fact that I need a niche, and decide what I’ll update on and what I won’t, and also be transparent with all of you about it) On a separate note, this past two weeks, ThursdAI had exposure to Yann Lecun (RTs), joined on stage by VP of DevRel in Cloudflare and their counterpart in HuggingFace, CEO of Anaconda joined us on stage this episode and we’ve had the chief scientist of Mistral join in the audience 😮 ThursdAI really shapes to be the place where this community meets, and I couldn’t be more humbled and prouder of the show, the experts on stage that join from week to week, and the growing audience 🙇♂️ ok now let’s get to the actual news! ThursdAI - Weeks like this one highlight how important it is to stay up to date on many AI news, subscribe, I’ve got some cool stuff coming! 🔥 All right so here’s everything we’ve covered on ThursdAI, September 28th: (and if you’d like to watch the episode video with the full transcript, it’s here for free): Show Notes + Links * Vision * 🔥 Open AI announces GPT4-Vision (Announcement, Model Card) * Meta glasses will be multimodal + AI assistant (Announcement) * Big Co + API updates * Cloudflare AI on workers, serverless GPU, Vector DB and AI monitoring (Announcement, Documentation) * Cloudflare announces partnerships with HuggingFace, Meta * Claude announces $4 billion investment from Amazon (Announcement) * Meta announces AI assistant across WhatsApp, Instagram * Open Source LLM * 🔥 Mistral AI releases - Mistral 7B - beating LLaMa2 13B (Announcement, Model) * Alibaba releases Qwen 14B - beating LLaMa2 34B (Paper, Model, Vision Chat) * AI Art & Diffusion * Meta shows off EMU - new image model * Still waiting for DALL-E3 😂 * Tools * Spotify translation using Open AI voice cloning tech Vision GPT 4-Vision I’ve been waiting for this release since March 14th (literally) and have been waiting and talking about this on literally every ThursdAI, and have been comparing every open source multimodality image model (IDEFICS, LlaVa, QWEN-VL, NeeVa and many others) to it, and none came close! And here we are, a brief rumor about the upcoming Gemini release (potentially a multimodal big model form Google) and OpenAi decided to release GPT-4V and it’s as incredible as we’ve been waiting for! From creating components from a picture of UI, to solving complex math problems with LaTex, to helping you get out of a parking ticket by looking at a picture of a complex set of parking rules, X folks report that GPT4-V is incredibly helpful and unlocks so many new possibilities! Can’t wait to get access, and most of all, for OpenAI to land this in the API for developers to start building this into products! On the pod, I’ve talked about how I personally don’t believe AGI can work without vision, and how personal AI assistants are going to need to see what I see to be really helpful in the real world, and we’re about to unlock this 👀 Super exciting. I will add this one last thing, here’s Ilya Sutskever, OpenAI chief scientist, talking about AI + Vision, and this connects with our previous reporting that GPT-4 is not natively multimodal (while we’re waiting for rumored Gobi) If you need more use-cases, check out this great breakdown by friend of the pod, SkalskiP (Pyotr) who is a vision engineer at RoboFlow which got really high Hacker News rankings. https://blog.roboflow.com/gpt-4-vision/ Meta RayBan smartglasses will have multimodal AI 👀 To add to the above increased interest about AI (and to rumors about OpenAI working with Jonny Ive from Apple + Masayoshi San about a rumored hardware device) Meta has announced a new iteration of their RayBan glasses, that will include a camera that will help you go live, include an AI agent in the Glasses and most of all, will be multimodal, by which they mean, the AI agent in there (we don’t know if it’s LLaMa based or something else) will have access to the camera, and to what you see. Given how well this works, it may be revolutionary on it’s own right! I’ve been on a MultiModality kick since that incredible March 14th day, and I’m very excited that it’s here! 🙌 Big CO + API updates Cloudflare AI - Serverless GPU inference, VectorDB and AI Gateway I was blown away by this, so much so, that I’ve hopped on an emergency space on Wednesday, to talk all about this. Some of you know, I’ve created https://targum.video a year ago, and it’s been accepted to CloudFlare workers launchpad. The whole website and backend is on workers, but the GPU and inference, I had to build in python and put on a LambdaLabs GPU machine. So starting today, folks could build something like Targum, end to end on Cloudflare with the announcement of GPU inference. If you’d like all the details, I was really humbled to host Ricky Robinette (VP Developer Experience @ Cloudflare) and Phillip Schmidt from Hugging Face join the X space on launch day (to my complete surprise) and you can find that conversation here (it’s going to be on the pod soon after I find some time to edit this 😅) Here’s my notes from that conversation: * Inference on edge is here * Serverless GPUs on cloudflare edge network * Integrated with Workers platform * What is the workers platform * Give example of the many tools it has * Targum example for what is done on workers and what is done on GPU * Easy to get started and deploy * Will have a free tier 🔆 * Models and Usecases * LLMs - LLaMa 7B * Embeddings - BGE-base * Text Classification - DistillBert * Translation - m2m100 * ASR - Whisper * Preselected models right now * Vectorize - an edge native vector DB * Integrates with wrangler and ecosystem * Supports existing vectors from OpenAI Ada (importable) * Metadata can include R2 objects, KV storage and more! * Build and deploy full RAG apps, including your own local models all inside 1 platform * AI - gateway * Proxy for OpenAI (and other providers calls) * Shows a usage dashboard * Global Coverage: * Plan to be in 100 data centers by the end this year * And nearly everywhere by the end of 2024 * WebGPU in workers * Many HF models support ONNX * WebGPU is now supporting FP-16 * This could open a new path to run smaller models within workers even without CFAI * Partnership with HuggingFace * 1 click deploy in a dropdown on half a million models * Serverless inference - no more downloading and uploading * Way faster as well * Cloudflare will have a de-facto proxy/mirror of HF? 🤔 I’m very very excited by the HuggingFace partnership and you can hear it in the recording! Meta announces AI assistant across chat apps, Instagram, WhatsApp, Messenger I haven’t tested this yet, but this is going to be incredible to make AI experiences to over 3B people around the world! In addition to just “chat with AI” , Meta has partnered with many celebs to “Embody” them into AI characters, which I found.. a bit unsettling? But I guess we’ll see how much this will affect the “personas” of the AI assistants. Open Source LLM Qwen 14B with chat and vision versions QWEN model from Alibaba, which we’ve already talked about multiple times, then was taken down from the web, comes back, with a vengeance! Qwen team comes back with a 14B model, that beats LlaMa2 34B on most evaluations, including a VL version (only 7B), which according to my tests, was the best performing open source vision model even at 7B It was really cool to see the Qwen authors interact with Yam and I on Twitter, it’s like crossing the great firewall and hopefully we’ll have that team on ThursdAI recording at some point! 🔥 Mistral 7B (torrent tweet) - SOTA LLM Mistral team have made news when they raised $113 million without a product, just 3 co-founders, back in Jun, and the “takes” on twitter were, “we’re in a bubble, bla bla bla” and yesterday, this Goated team just posted a tweet with a magnet torrent link, and no description. So of course everybody downloaded it and found the best SOTA 7B model, that outperforms a much larger LLaMa 2 13B and MUCH larger LLaMa 34B on several benchmarks! It even comes very close to the Code LLaMa performance benchmarks on code, while being a general model, which is incredible. Needless to say, the team delivered the promise, and to see them commit this fully to OpenSource, by dropping a modal with Apache 2 license, straight to bit-torrent, is a great sight to see! Also, we caught glimpes of Guillaume Lample in the audience while we were gassing Mistral up, and potentially at some point we may get Mistral folks to join a ThursdAI live space? 🫡 AI Art + Diffusion Meta introduced EMU - A diffusion model integrated into it’s AI offerings with a /imagine command, available for free, in all their products, and it looks really good! I wonder if it will do the same “chat with image” thing as DALL-E3 was announced to do, but in any case, giving this, for free, in this quality, to so many people, is remarkable 🙇♂️ Kudos to the team at Meta for ALL the releases today! Can’t wait to play with them. Tools Spotify translates podcasts using stealth OpenAI tech Spotify announced translations for podcast, using some secret OpenAI voice cloning tech, and we had a long discussion about the implication of voice cloning, deep fakes and everything in between with Peter Wang and other folks on the pod, definitely recommended listening! I love this, absolutely, not just because you may want to listen to ThursdAI pod in your native language (and I could finally show my mom who doesn’t speak English what I’m doing!) but also because language barriers should NOT exist, and Targum.video and this and all the models that Meta is releasing are a great testament to how fast language barriers are coming down! I’m very very happy with this development and will keep you guys posted on these developments. With that, I should probably stop here, it’s been an absolutely insane week, and if this summary helped, like, share and consider a premium subscription? ThursdAI - Recaps of the most high signal AI weekly spaces is a reader-supported publication. To receive new posts and support my work, consider becoming a free or paid subscriber. P.S - If you scrolled all the way to here, send me 🧨 in a DM on any platform 😉, I may have something for you This is a public episode. If you'd like to discuss this with other subscribers or get access to bonus episodes, visit sub.thursdai.news/subscribe | |||
05 Oct 2023 | 📅 ThursdAI Oct 4 - AI wearables, Mistral fine-tunes, AI browsers and more AI news from last week | 01:28:21 | |
Boy am I glad that not all AI weeks are like last week, where we had so much news and so many things happening that I was barely able to take a breath for the week! I am very excited to bring you this newsletter from San Fancisco this week, the AI mecca, the arena, the place where there are so many AI events and hack-a-thons that I don’t actually know how people get any work done! On that topic, I’m in SF to participate in the AI.engineer (by swyx and Benjamin Dunphy) next week, to host spaces and interviews with the top AI folks in here, and to discuss with the audience, what is an AI engineer, if you have any questions you’d like me to ask, please comment with them and I’ll make sure I’ll try to answer. ThursdAI - subscribe eh? ↴ Here’s a table of contents of everything we chatted about: [00:00:00] Intro and welcome [00:04:53] Alex in San Francisco - AI Engineer [00:07:32] Reka AI - Announcing a new multimodal Foundational model called Yasa-1 [00:12:42] Google adding Bard to Google Assistant [00:18:56] Where is Gemini? [00:23:06] Arc browser adding Arc Max with 5 new AI features [00:24:56] 5 seconds link AI generated previews [00:31:54] Ability to run LLMs on client side with WebGPU [00:39:28] Mistral is getting love from Open Source, [00:48:04] Mistral Open Orca 7B [00:58:28] Acknowledging the experts of ThursdAI [01:01:14] Voice based always on AI assistants [01:09:00] Airchat adds voice cloning based translation tech [01:14:23] Effects of AI voice cloning on society [01:21:32] SDXL IKEA LORA [01:23:17] Brief Recap Show notes: Big Co * Google - adding Bard to Google Assistant (Announcement)Come on google, just give us Gemini already! * Reka AI - Multimodal Yasa-1 from Yi Tay and team (Announcement)With Yi Tay from Flan/Bard fame as chief scientist! But I wasn’t able to test myself! * Arc - first browser AI features (My thread, Brief video review, Arc Invite)I love Arc, I recommend it to everyone I meet, now with AI preview features it’s even more a non brainer, strongly recommend if you like productivity Open Source LLMs * Mistral vs LLaMa 2 boxing match (link)A fun little battle arena to select which responses you personally find better to see the difference between Mistral 7B and LLaMa 13B * Mistral-7B-OpenOrca (announcement)The folks from Alignment labs do it again! Great finetune that comes very close (98%) to LLaMa 70B on benchmarks! * SynthIA-7B-v1.3 - (Huggingface)An uncensored finetune on top of Mistral that Reddit claims is a great model, especially since a chain of thought is somehow built in apparently VISION * Radiologists thread about GPT-4 V taking over radiology (or maybe not?) (Thread) Voice * AirChat added voice clone + translation features (Room, Demo)I’ve been an avid AirChat user (It’s Naval’s social media platform that’s voice based) for a while, and am very excited they are destroying language barriers with this feature! * Tab was revealed in a great demo by Avi Schiffman (Demo)Go Avi! Rooting for you brother, competition makes folk stronger! * Rewind announced Rewind Pendant (Announcement)I ordered one, but Rewind didn’t announce a date of when this hits the market, going to be interesting to see how well they do! Ai Art and Diffusion - IKEA Lora generate IKEA style tutorials for everything with SDXL (Announcement, HuggingFace) * DALL-E3 seems to be available to all Plus members now This weeks pod was generated by talking to chatGPT, it’s so fun, you gotta try it! No longer breakdown this week ,but we covered a bunch of it in the show, and I highly recommend listening to it! Don’t forget to follow me on X to be aware of the spaces live from ai.engineer event in SF, the conference will be live-streamed as well on youtube! See you next week 🫡 ThursdAI - Recaps of the most high signal AI weekly spaces is a reader-supported publication. To receive new posts and support my work, consider becoming a free or paid subscriber. This is a public episode. If you'd like to discuss this with other subscribers or get access to bonus episodes, visit sub.thursdai.news/subscribe | |||
13 Oct 2023 | A week of horror, an AI conference of contrasts | 01:29:16 | |
A week of horror, an AI conference of contrasts Hi, this is Alex. In the podcast this week, you'll hear my conversation with Miguel, a new friend I made in AI.engineer event, and then a recap of the whole Ai.engineer event I had with Swyx after the end. This newsletter is a difficult one for me to write, honestly, I wanted to skip this one entirely, struggling to fit the current events into my platform and the AI narrative, however, decided to write one anyway, as the events of the last week have merged into 1 for me in a flurry of contrasts. Contrast 1 - Innovation vs Destruction I was invited (among a few other Israelis or Israeli-Americans) to the ai.engineer summit in SF, to celebrate the rise of the AI engineer, and I was looking forward to that very much. Meeting many of you (Shoutout to everyone who listens to ThursdAI who I've met face to face!) and talking to new friends of the pod, interviewing speakers, meeting and making connections was a dream come true. However a few days before the conference began, in a stark contrast to this dream, I had to call my mom, who was sheltering, 20km from the Gaza strip border, to ask if our friends and family are alive and accounted for, and to hear sirens as rockets flying above her head, as Hamas terrorists murder, pillage and kidnap, in what seems to be the 10x equivalent of 9/11 terror attack, relative to population size. I grew up in Ashkelon, rocket attacks are nothing new to me, we've learned to live with them (thank you Iron Dome heroes) but this was something else entirely, a new world of terror. So back to the conference, given that there's not a lot to be gained by doom scrolling, and watching (basically snuff) films coming out of the region, given that all my friends and family were accounted for, I decided to not give the terrorists what they want (which is to get people in state of terror) and instead to choose to have compassion, without empathy towards the situation and not bring sadness to every conversation I had there (over 200 I think) So participating at an AI event, which hosts and celebrates folks who are literally at the pinnacle of innovation, building the future, using all the latest tools while also hurting and holding the dear ones in my thoughts was a very stark contrast between past and future, and huge credit goes to Dedy Kredo, CTO of Codium, who was in the same position, and gave a hell of a talk, with a kick-ass (no backup recording!) demo live, and then shared this image: This is his co-founder, Itamar, who was called to reserve duty to protect his family and country, sitting with his rifle and his dashboard, seeing destruction + creation, past and future, negativity and positivity all at once. As Dedy masterfully said, we will prevail 🙏 Contrast 2 - Progress // Fear At the event, Swyx and Benjamin gave me a media pass and a free reign, and I asked to be teamed with a camera-person to go around the event and do some (not live) interviews. I was teamed with the lovely Stacey, from Chico, CA. Stacey has nothing to do with AI, in fact she's a wedding photographer, however she definitely listened with interest to the interviews I was holding, and to speakers on stage. While we were taking a break, I looked out the window, and saw a driverless car (waymo) zip by, and since they only started operating after I left SF 3 years ago, I didn't yet have a chance to ride in one. So I asked Stacey and some other folks, if they'd like to go for a ride, and to my complete bewilderement, Stacey said "no 😳" and when I asked why not, she didn't want to admin but then said that it's scary. This struck me and since that moment, I've had as many conversations with Stacey as I had with other folks who came to be AI.engineers, since this was such a stark contrast between progress and fear. I basically was walking, almost hand in hand, with a person who doesn't use or understand AI, and fears it, amongst the folks who are building the future, exist at the pinnacle of innovation and discuss how to connect more AI to more AI, and how to build complete autonomous agents to augment human productivity and bring about the world of abundance. This contrast was supported by several new friends of mine, who came to the AI.engineer and SF for the first time, from countries where English is not the first language, and where Waymo's are not zipping about on the streets freely, and it highlighted for me, how much of this shift is global, and how concentrated the decision making, the building, the innovation is, within the arena, SF, California and US. It's almost expected that AI is going to speak english, and to use/build it, we have to speak it as well, while most of the world doesn't use English as their first language. Contrast 3 - Technological // Spiritual This contrast was intimate and personal to me. You see, this ai.engineer event was the first such sized event, professional, with folks talking "my language" since I had burned out this summer. If you've followed for a while, you may remember we talked about Lk-99 and superconductor, and I overclocked myself back then so much (scaling a whole another podcast, hosting 7 spaces in 2 weeks, creating a community of 1,500 and following all the news 24/7) that I had didn't want to go on speaking, doing spaces, recording podcasts... I was just done. Luckily my friend Junaid sent me a meditation practice recording with the saying "fill your own cup, before you give out to others" That recording led me to discover effortless meditation (via AirChat, DM me for details), and going on a 5 day free virtual retreat, which changed my life in several very significant ways and just keeps going. So back to the conference, coming from that place, a place of center, a place where I balance my own inner piece + interact with 150+ people (I think, I haven't really counted) and equalizing energy among them, connecting many folks to many other folks, introducing and getting introduced, giving interviews, remembering names and faces, this was an incredible experience to me, and many of my new friends asked me, what's going on, and why and how I meditate for an hour every day. So the contrast between my own spiritual journey, and the technological journey was not a very strong one, beyond 1 very... interesting and weird conversation I had, everyone else seemed to be into self improvement, spiritual growth, I've met many folks who went on vipasana retreats, had meditation practices themselves or used to have one and wanted to come back to it. Maybe it was self selection, but I really enjoyed seeing and hearing all the stories from folks, as I believe that given contrast number 2, the way we talk about AI, the way we build AI, the way we then show AI to the world, needs to take into account compassion towards those who don't understand it, who fear it, and want nothing to do with it. Not to mention, that I doubt that any of the doomers are walking on their own spiritual path. I wanted to just send this newsletter but as I always do, I pasted it into Claude.ai, and asked for a review. And Claude suggested I end it on a positive note, because the middle was heavy, and even gave me some suggestion as to how to end it on a positive note. So instead of me doing it, here's an AI, doing a human job on ending something on a positive note: [Claude] Here are some examples of how you could end your newsletter on a more hopeful, forward-looking note: * Remind readers of the optimism and excitement you felt at the AI conference, and how seeing the potential of technology firsthand gives you hope for the future. * Share an insight or realization you had about how to stay grounded and centered even amidst chaos - something your spiritual practices have taught you. * Affirm that while destruction and violence will always be part of the human experience, you believe innovative thinkers will lead us to a brighter tomorrow. * Note how connecting deeply with other humans, even strangers, fills you with hope. Finding common ground and shared humanity counterbalances the forces that divide. * Point to a specific AI-related project or breakthrough that gives you hope for progress. e.g. medical advancements, climate change solutions, etc. * Share an inspirational quote or verse that reflects resilience and perseverance in difficult times. * End by expressing gratitude - for your community of readers, your loved ones, the chance to have profound human experiences, or your inner stillness amidst outer turmoil. The key is ending on a note of hope, possibility, love, resilience - something that uplifts readers after processing weighty material. Let me know if these spark any ideas for how to conclude your newsletter on a high note! P.S - I know this newsletter is not what you expected, and maybe not what you signed up for, and I deliberated if I even should write it and what if anything should I post on the podcast. However, this week was an incredibly full of contrast, of sadness and excitement, of sorrow and bewilderment, so I had to share my take on all this. P.P.S - as always, if you read all the way to the end, dm me the ☮️ emoji This is a public episode. If you'd like to discuss this with other subscribers or get access to bonus episodes, visit sub.thursdai.news/subscribe | |||
20 Oct 2023 | 🔥 ThursdAI Oct 19 - Adept Fuyu multimodal, Pi has internet access, Mojo works on macs, Baidu announces ERNIE in all apps & more AI news | 01:29:56 | |
Hey friends, welcome to ThursdAI Oct - 19. Here’s everything we covered + a little deep dive after the TL;DR for those who like extra credit. ThursdAI - If you like staying up to date, join our community Also, here’s the reason why the newsletter is a bit delayed today, I played with Riffusion to try and get a cool song for ThursdAI 😂 ThursdAI October 19th TL;DR of all topics covered: * Open Source MLLMs * Adept open sources Fuyu 8B - multi modal trained on understanding charts and UI (Announcement, Hugging face, Demo) * Teknium releases Open Hermes 2 on Mistral 7B (Announcement, Model) * NEFTune - a "one simple trick" to get higher quality finetunes by adding noise (Thread, Github) * Mistral is on fire, most fine-tunes are on top of Mistral now * Big CO LLMs + APIs * Inflection Pi got internet access & New therapy mode (Announcement) * Mojo 🔥 is working on Apple silicon Macs and has LLaMa.cpp level performance (Announcement, Performance thread) * Anthropic Claude.ai is rolled out to additional 95 countries (Announcement) * Baidu AI announcements - ERNIE 4, multimodal foundational model, integrated with many applications (Announcement, Thread) * Vision * Meta is decoding brain activity in near real time using non intrusive MEG (Announcement, Blog, Paper) * Baidu YunYiduo drive - Can use text prompts to extract precise frames from video, and summarize videos, transcribe and add subtitles. (Announcement) * Voice & Audio * Near real time voice generation with play.ht - under 300ms (Announcement) * I'm having a lot of fun with Airpods + chatGPT voice (X) * Riffusion - generate short songs with sound and singing (Riffusion, X) * AI Art & Diffusion * Adobe releases Firefly 2 - lifelike and realistic images, generative match, prompt remix and prompt suggestions (X, Firefly)DALL-E 3 is now available to all chatGPT Plus uses (Announcement, Research paper!) * Tools * LMStudio - a great and easy way to download models and run on M1 straight on your mac (Download) * Other * ThursdAI is adhering to the techno-optimist manifesto by Pmarca (Link) Open source mLLMs Welcome to multimodal future with Fuyu 8B from Adept We've seen and covered many multi-modal models before, and in fact, most of them will start being multimodal, so get ready to say "MLLMs" or... we come up with something better. Most of them so far have been pretty heavy, IDEFICS was 80B parameters etc' This week we received a new, 8B multi modal with great OCR abilities from Adept, the same guys who gave us Persimmon 8B a few weeks ago, in fact, Fuyu is a type of persimmon tree (we see you Adept!) In the podcast I talked about having 2 separate benchmarks for myself, one for chatGPT or any MultiModal coming from huge companies, and another for open source/tiny models. Given that Fuyu is a tiny model, it's quite impressive! It's OCR capabilities are impressive, and the QA is really on point (as well as captioning) An interesting thing about FuYu architecture is, because it doesn't use the traditional vision encoders, it can scale to arbitrary image sizes and resolutions, and is really fast (large image responses under 100ms) Additionally, during the release of Fuyu, Arushi from Adept authored a thread about visualQA evaluation datasets are, which... they really are bad, and I hope we get better ones! NEFTune - 1 weird trick of adding noise to embeddings makes models better (announcement thread) If you guys remember, a "this one weird trick" was discovered by KaiokenDev back in June, to extend the context window of LLaMa models, which then turned into RoPE scaling and YaRN scaling (which we covered in a special episode with the authors) Well, now we have a similar "1 weird trick" that by just adding some noise to embeddings at training time, the model performance can grow by up to 25%! The results very per dataset of course, however, considering how easy it is to try, literally: It's as simple as doing this in your forward pass if training: return orig_embed(x) + noise else: return orig_embed(x) We should be happy that the "free lunch" tricks like this exist. Notably, we had a great guest, Wing Lian the maintainer of Axolotl, a very popular tool to streamline fine-tuning, chime in and say that in his tests, and among the discord folks, they couldn't reproduce some of these claims (as they are adding everything that's super cool and beneficial for finetuners to their library) so it remains to be seen how far this "trick" scales, and what else needed to be done here. Similarly, back when the context extend trick was discovered, there was a lot of debates about it's effectiveness from Ofir Press (author of ALiBi, another context scaling methond) and futher iterations of the trick made into a paper and a robust method, so this develompment is indeed exciting! Mojo 🔥 now supports Apple silicon Macs and has LLaMa.cpp level performance! I've been waiting for this day! We've covered Mojo from Modular a couple of times and it seems that the promise behind it starts to materialize. Modular promises an incredible unbelieavable 68,000X boost over vanilla python, and it's been great to see that develop. Today (October 19) they have released their support of Mojo Lang on Apple silicon which most developers use, and it's a native one and you can use it right now via CLI. A friend of the pod Aydyn Tairov, hopped on the live recording and talked to use about his LLama.🔥 project (Github) that he ported to the Apple silicon, and showed an incredible, LLaMa.cpp like performance, without crazy optimizations! Aydyn collected many LLaMa implementations, including Llama.cpp, LLama.c by Karpathy and many others, and included his LLama.mojo (or Llama.🔥) and saw that the mojo one is coming very very close to LLama.cpp and significantly beats Rust and Go and Julia examples (on specific baby llama models) The Mojo future is bright, and we'll keep updating with more, but for now, go play with it! Meta is doing near-real time brain → image research! 🤯 We've talked about fMRI signals (and EEG) signals being translated to diffusion imagery before, and this week, Meta has shown that while fMRI signals to brain imagery is pretty crazy on it's own, using something called MEG (non invasive Magnetoencephalography) they can generate and keep generating images based on the brain signals, in near real time! [TK video here] I don't have a LOT to say about this topic, besides the fact that as an Aphant (I have Aphantasia) I can't wait to try this on myself and see what my brain actually "sees" Baidu announces ERNIE and a bunch AI native products including maps, drive, autonomous ride hailing and more. Baidu has just wrapped up their biggest conference of the year, BaiduWorld, where they announced a new version of their foundational model called ERNIE4, which is a multimodal (of unknown size) and is now integrated into quite a few of their products, many of which are re-imagined with AI. A few examples beyond a basic LLM chat like interface are, a new revamped map experience with an AI assistant (with voice) built in to help you navigate and find locations, a new office management app that handles appointments and time slots called InfoFlow, and it apparently even does travel booking, to an AI "google drive" like product called YunYidou, that is able to find video content, based on what was said and when, and even pinpoint specific frames, summarize and do a bunch fo other incredible AI stuff, here's a translated video of someone interacting with YunYinou and asking for a bunch of stuff one after another. Disclosure: I don't know if the video is edited or in real time. Voice & Audio Real time voice for agents is almost here, chatGPT voice mode is powerful I've spent maybe 2 hours this week, with chatGPT in my ear, using the new voice mode + AirPods. It's almost like... being on a call with chatGPT. I started talking to it in the store, asking for different produce to buy for a recipe, then drove home and ask it to "prepare" me for the task (I don't usually cook this specific thing) and then during my cooking, I kept talking to it, asking for next steps. With the new IOS the voice mode shows up as a live activity and you can pause it and resume without opening the app: It was literally present in my world, without me having to watch the screen or type. It's a completely new paradigm of interactions when you don't have to type anymore, or pick up a screen and read, and it's wonderful! Play.ht shows off an impressive <300ms voice generation for agents After spending almost 2 hours talking to chatGPT, I was thinking, why aren't all AI assistants like this, and the answer was, well... generating voice takes time, which takes you out of your "conversation flow" And then today, play.ht showed off a new update to their API that generates voice in <300ms, and that can be a clone of your voice, with your accent and all. We truly live in unprecedented times. I can't wait for agents to start talking and seeing what I see (and remember everything I heard, via Tab or Pendant or Pin) Riffusion is addictive, generate song snippets with life-like lyrics! We've talked about music gen before, however, Riffusion is a new addition and is now generating short song segments with VOICE! Here are a few samples, and honestly, I've procrastinated writing this newsletter because it's so fun to generate these, and I wish they went for longer! AI Art & Diffusion Adobe releases Firefly2 which is significantly better at skin textures, realism, and hands. Additionally they have added a style transfer which is wonderful, upload a picture of something with a style you'd like, and your prompt will be generated in that style, it works really really well. The extra details on the skin is just something else, though I did cherry pick this example, the other hands were a dead give-away, still, the hands are getting better across the board! Plus they have a bunch of prompt features, like prompt suggestion, ability to remix other creations and more, it's really quite developed at this point. Also: DALL-E is now available to 100% of plus users and enterprise, have you tried it yet? What do you think? Let me know in replies! That’s it for October 19. If you're into AI engineering, make sure you listen to the previous weeks podcast where Swyx and I recapped everything that happened on stage and off it in the seminal AI Engineer summit. And make sure to share this newsletter with your friends who like AI! For those who are 'in the know', emoji of the week is 📣, please DM or reply with it if you got all the way here 🫡 and we'll see you next week (where I will have some exciting news to share!) This is a public episode. If you'd like to discuss this with other subscribers or get access to bonus episodes, visit sub.thursdai.news/subscribe | |||
26 Oct 2023 | 📅 ThursdAI Oct-26, Jina Embeddings SOTA, Gradio-Lite, Copilot crossed 100M paid devs, and more AI news | 01:39:42 | |
ThursdAI October 26th Timestamps and full transcript for your convinience ## [00:00:00] Intro and brief updates ## [00:02:00] Interview with Bo Weng, author of Jina Embeddings V2 ## [00:33:40] Hugging Face open sourcing a fast Text Embeddings ## [00:36:52] Data Provenance Initiative at dataprovenance.org ## [00:39:27] LocalLLama effort to compare 39 open source LLMs + ## [00:53:13] Gradio Interview with Abubakar, Xenova, Yuichiro ## [00:56:13] Gradio effects on the open source LLM ecosystem ## [01:02:23] Gradio local URL via Gradio Proxy ## [01:07:10] Local inference on device with Gradio - Lite ## [01:14:02] Transformers.js integration with Gradio-lite ## [01:28:00] Recap and bye bye Hey everyone, welcome to ThursdAI, this is Alex Volkov, I'm very happy to bring you another weekly installment of 📅 ThursdAI. ThursdAI - Recaps of the most high signal AI weekly spaces is a reader-supported publication. To receive new posts and support my work, consider becoming a free or paid subscriber. TL;DR of all topics covered: * Open Source LLMs * JINA - jina-embeddings-v2 - First OSS embeddings models with 8K context (Announcement, HuggingFace) * Simon Willison guide to Embeddings (Blogpost) * Hugging Face - Text embeddings inference (X, Github) * Data Provenance Initiative - public audit of 1800+ datasets (Announcement) * Huge open source LLM comparison from r/LocalLLama (Thread) * Big CO LLMs + APIs * NVIDIA research new spin on Robot Learning (Announcement, Project) * Microsoft / Github - Copilot crossed 100 million paying users (X) * RememberAll open source (X) * Voice * Gladia announces multilingual near real time whisper transcriptions (X, Announcement) * AI Art & Diffusion * Segmind releases SSD-1B - 50% smaller and 60% faster version of SDXL (Blog, Hugging Face, Demo) * Prompt techniques * How to use seeds in DALL-E to add/remove objects from generations (by - Thread) This week was a mild one in terms of updates, believe it or not, we didn't get a new State of the art open source large language model this week, however, we did get a new state of the art Embeddings model from JinaAI (supporting 8K sequence length). We also had quite the quiet week from the big dogs, OpenAI is probably sitting on updates until Dev Day (which I'm going to cover for all of you, thanks to Logan for the invite), Google had some leaks about Gemini (we're waiting!) and another AI app builder thing, Apple is teasing new hardware (but nothing AI related) coming soon, and Microsoft / Github announced that CoPilot has 100 million paying users! (I tweeted this and Idan Gazit, Sr. Director GithubNext where Copilot was born, tweeted that "we're literally just getting started" and mentioned November 8th as... a date to watch, so mark your calendars for some craziness next two weeks) Additionally, we covered the Data provenance initiative that helps sort and validate licenses for over 1800 public datasets, a massive effort led by Shayne Redford with assistance from many folks including friend of the pod Enrico Shippole, we also covered another massive evaluation effort by a user named WolframRavenwolf on the LocalLLama subreddit, that evaluated and compared 39 open source models and GPT4. Not surprisingly the best model right now is the one we covered last week, OpenHermes 7B from Teknium. Two additional updates were covered, one of them is Gladia AI, released their version of whisper over web-sockets, and I covered it on X with a reaction video, it allows developers to stream speech to text, with very low latency and it's multi-lingual as well, so if you're building an agent that folks can talk to, definitely give this a try, and finally, we covered SegMind SSD-1B, a distilled version of SDXL, making it 50% smaller in size and 60% faster in generation speed (you can play with it here) This week I was lucky to host 2 deep dive conversations, one with Bo Wang, from Jina AI, and we covered embeddings, vector latent spaces, dimensionality, and how they retrained BERT to allow for longer sequence length, it was a fascinating conversation, even if you don't understand what embeddings are, it's well worth a listen. And in the second part, I had the pleasure to have Abubakar Abid, head of Gradio at Hugging Face, to talk about Gradio, it's effect on the open source community, and then joined by Yuichiro and Xenova to talk about the next iteration of Gradio, called Gradio-lite that runs completely within the browser, no server required. A fascinating conversation, if you're a machine learning engineer, AI engineer, or just someone who is interested in this field, we covered a LOT of ground, including Emscripten, python in the browser, Gradio as a tool for ML, webGPU and much more. I hope you enjoy this deep dive episode with 2 authors of the updates this week, and hope to see you in the next one. P.S - if you've been participating in the emoji of the week, and have read all the way up to here, your emoji of the week is 🦾, please reply or DM me with it 👀 Timestamps and full transcript for your convinience ## [00:00:00] Intro and brief updates ## [00:02:00] Interview with Bo Weng, author of Jina Embeddings V2 ## [00:33:40] Hugging Face open sourcing a fast Text Embeddings ## [00:36:52] Data Provenance Initiative at dataprovenance.org ## [00:39:27] LocalLLama effort to compare 39 open source LLMs + ## [00:53:13] Gradio Interview with Abubakar, Xenova, Yuichiro ## [00:56:13] Gradio effects on the open source LLM ecosystem ## [01:02:23] Gradio local URL via Gradio Proxy ## [01:07:10] Local inference on device with Gradio - Lite ## [01:14:02] Transformers.js integration with Gradio-lite ## [01:28:00] Recap and bye bye Full Transcription: [00:00:00] Alex Volkov: Hey, everyone. Welcome to Thursday. My name is Alex Volkov, and I'm very happy to bring you another weekly installment of Thursday. I. This week was actually a mild one in terms of updates, believe it or not. Or we didn't get the new state of the art opensource, large language model this week. However, we did get a new state of the art embeddings model. And we're going to talk about that. we got very lucky that one of the authors of this, a medics model, gold Gina embeddings V2, Bo Wang joined us on stage and gave us a masterclass in embeddings and share some very interesting things about this, including some stuff they haven't charged yet. So definitely worth a listen. Additionally recovered the data provenance initiative that helps sort and validate licenses for over 1800 public data sets. A massive effort led by Shane Redford with assistance from many folks, including a friend of the pod. Enrico Shippole. [00:01:07] we also covered the massive effort by another user named Wolf from Ravenwolfe on the local Lama subreddit. Uh, that effort evaluated and compared to 39 open source models ranging from 7 billion parameters to 70 billion parameters and threw in the GPT4 comparison as well. Not surprisingly, the best model right now is the one we covered last week from friends of the politic new called open Hermes seven B. [00:01:34] Do additional updates we've covered. One of them is Gladia AI, a company that offers transcription and translation APIs release their version of whisper over WebSockets. So live transcription, and I covered it on X with a reaction video. And I'll add that link in the show notes. It allows developers like you to stream speech, to text and. Very low latency and high quality and it's multi-lingual as well. So if you're building an agent that your users can talk to. Um, definitely give this a try. And finally Segmind segued mind accompany that just decided to open source a distilled version of. SDXL, making it 50% smaller in size and the in addition to that 60% faster in generation speed. The links to all these will be in the show notes. [00:02:23] But this week I was lucky to host two deep dives, one with Bo Weng which I mentioned. Uh, we've covered the embeddings vector led in spaces that dimensionality and how they retrained Bert model to allow for a longer sequence length. It was a fascinating conversation. Even if you don't understand what embeddings are, it's well worth the listen. And, , I learned a lot. Now I hope you will, as well. And the second part, I had the pleasure to have a Brubaker a bit. The head of grandio at hugging face to talk about gradient. What is it? Uh, its effect on the open source community. And then joined by utero. And Sunnova to talk about the next iteration of Grigio called Grigio light that runs completely within the browser. No Serra required. We also covered a bit of what's coming to Gradio in the next release. on October 31st. [00:03:15] A fascinating conversation. If you're a machine learning engineer, AI engineer, or just somebody who's interested in this skilled. You've probably used radio, even if you haven't written any Gradio apps, every model and hugging face usually gets a great deal demo. [00:03:30] And we've covered a lot of ground, including M scripting. Then by filling the browser. As a tool for machine learning, web GPU, and so much more. [00:03:38] Again, fascinating conversation. I hope you enjoy this deep dive episode. Um, humbled by the fact that sometimes the people. Who produced the updates we cover actually come to Thursday and talk to me about the things they released. And I hope this trend continues, and I hope you enjoyed this deep dive over an episode. And, um, I'll see you in the next one. And now I give you thursday october 26. oh, awesome. It looks like Bo, you joined us. Let's see if you're connecting to the audience, and can you unmute yourself, can you see if we can hear you? [00:04:22] Bo Wang: Hi, can you hear me? Oh, we can hear you fine, awesome. this, this, this feature of, of Twitter. [00:04:30] Alex Volkov: That's awesome. This, this usually happens, folks join and it's their first face and then they can't leave us. And so let me just do a little, maybe... Maybe, actually, maybe you can do it, right? Let me just present yourself. [00:04:42] I think I followed you a while ago, because I've been mentioning embeddings and the MTB dashboard and Hug and Face for a while. And, obviously, embeddings are not a new concept, right? We started with Word2Vec ten years ago, but now, with the rise of LLMs, And now with the rise of AI tools and many people wanting to understand the similarity between the user query and an actual thing they, they, they stored in some database, embeddings have seen a huge boon. [00:05:10] And also we've saw like all the vector databases pop up like mushrooms after the rain. I think Spotify just released a new one. And my tweet was like, Hey, do we really need another vector database? But Boaz, I think I started following you because you mentioned that you were working on something that's. [00:05:25] It's coming very soon, and finally this week this was released. So actually, thank you for joining us, Beau, and thank you for doing the first ever Twitter space for yourself. How about can we start with your introduction of who you are and how are you involved with this effort, and then we can talk about Jina. [00:05:41] Bo Wang: Yes, sure. Basically I have a very different background. I guess I was oriJinally from China, but my bachelor was more related to text retrieval. I have a retrieval experience rather than pure machine learning background, I would say. Then I came to the Europe. I came to the Netherlands like seven or eight years ago as a, as an international student. [00:06:04] And I was really, really lucky and met my supervisor there. She basically guided me into the, in the world of the multimedia information retrieval, multimodal information retrieval, this kind of thing. And that was around 2015 or 2016. So I also picked up machine learning there because when I was doing my bachelor, it's not really hot at that moment. [00:06:27] It's like 2013, 2014. Then machine learning becomes really good. And then I was really motivated, okay, how can I apply machine learning to, to search? That is, that is my biggest motivation. So when I was doing my master, I, I collaborated with my friends in, in, in the US, in China, in Europe. We started with a project called Match Zoo. [00:06:51] And at that time, the embedding on search is just a nothing. We basically built a open source. Software and became at that time the standard of neural retrieval or neural search, this kind of thing. Then when the bird got released, then our project basically got queue because. Everyone's focus basically shifted to BERT, but it's quite interesting. [00:07:16] Then I graduated and started to work as a machine learning engineer for three years in Amsterdam. Then I moved to Berlin and joined Jina AI three years ago as a machine learning engineer. Then basically always doing neural search, vector search, how to use machine learning to improve search. That is my biggest motivation. [00:07:37] That's it. [00:07:38] Alex Volkov: Awesome. Thank you. And thank you for sharing with us and, and coming up and Gene. ai is the company that you're now working and the embeddings thing that we're going to talk about is from Gene. ai. I will just mention the one thing that I missed in my introduction is the reason why embeddings are so hot right now. [00:07:53] The reason why vectorDB is so hot right now is that pretty much everybody does RAG, Retrieval Augmented Generation. And obviously, For that, you have to store some information in embeddings, you have to do some retrieval, you have to figure out how to do chunking of your text, you have to figure out how to do the retrieval, like all these things. [00:08:10] Many people understand that whether or not in context learning is this incredible thing for LLMs, and you can do a lot with it, you may not want to spend as much tokens on your allowance, right? Or you maybe not have enough in the context window in some in some other LLMs. So embeddings... Are a way for us to do one of the main ways to interact with these models right now, which is RAC. [00:08:33] And I think we've covered open source embeddings compared to OpenAI's ADA002 embedding model a while ago, on ThursDAI. And I think It's been clear that models like GTE and BGE, I think those are the top ones, at least before you guys released, on the Hugging Face big embedding model kind of leaderboard, and thank you Hugging Face for doing this leaderboard. [00:09:02] They are great for open source, but I think recently it was talked about they're lacking some context. And Bo, if you don't mind, please present what you guys open sourced this week, or released this week, I guess it's open source as well. Please talk through Jina Embeddings v2 and how it differs from everything else we've talked about. [00:09:21] Bo Wang: Okay, good. Basically, it's not like embeddings for, how can I say, maybe two... point five years. But previously we are doing at a much smaller scale. Basically we built all the algorithm, all the platform, even like cloud fine tuning platform to helping people build better embeddings. So there is a not really open source, but a closed source project called fine tuner, which we built to helping user build better embeddings. [00:09:53] But we didn't, we found it okay. Maybe we are maybe too early. because people are not even using embeddings. How could they find embeddings? So we decided to make a move. Basically, we basically scaled up our how can I say ambition. We decided to train, train our own embeddings. So six months ago, we started to train from scratch, but not really from scratch because in binding training, normally you have to train in two stages. [00:10:23] The first stage, you need to pre train on massive scale of like text pairs. Your objective is to bring these text pairs as closer as possible, as possible, because these text pairs should be semantically related to each other. In the next stage, you need to fine tune with Carefully selected triplets, all this kind of thing. [00:10:43] So we basically started from scratch, but by collecting data, I think it was like six months ago, we working with three to four engineers together, basically scouting every possible pairs from the internet. Then we basically created like one billion, 1. 2 billion sentence pairs from there. And we started to train our model based on the T5. [00:11:07] Basically it's a very popular encoder decoder model. This is on the market. But if you look at the MTB leaderboard or all the models on the market, the reason why they only support 512 sequence lengths is constrained actually by the backbone itself. Okay, we figure out another reason after we release the V1 model. [00:11:31] Basically, if you look at. And the leaderboard or massive text embedding leaderboard, that is the one Alex just mentioned. Sorry, it's really bad because everyone is trying to overfitting the leaderboard. That naturally happens because if you look at BGE, GTE, the scores will never that high if you don't add the training data into the, into the, That's really bad. [00:12:00] And we decided to take a different approach. Okay. The biggest problem we want to solve first, improving the quality of the embeddings. The second thing we want to solve is. Enable user to making longer context lens. If we want to making user make user have longer context lens, so we have to rework the BERT model, because every basically the embedding model, the backbone was from BERT or T5. [00:12:27] So we basically started from scratch. Why not we just borrow the latest research from large language model? Every large language model wants large context. Why not we just borrow the research ideas? into the musk language modeling modelings. So we basically borrowed some ideas, such as rotary position embeddings or alibi, maybe you did, and reworked BERT. [00:12:49] We call it JinaBERT. So basically now the JinaBERT can handle much longer sequence. So we trained BERT from scratch. Now BERT has been a byproduct of our embeddings. Then we use this JinaBERT to contrastively train the models on the semantic pairs and triplets that finally allow us to encode 8K content. [00:13:15] Alex Volkov: Wow, that's impressive. Just, just to react to what you're saying, because BERT is pretty much every, everyone uses BERT or at least use BERT, right? At least in the MTB leaderboard. I've also noticed many other examples that use BERT or distilled BERT and stuff like this. You're saying, what you're saying, if I'm understanding correctly, is this was the limitation for sequence length? [00:13:36] for other embedding models in the open source, right? And the OpenAI one that's not open source, that does have 8, 000 sequence length. Basically, sequence length, if I'm explaining correctly, is just how much text you can embed without chunking. [00:13:51] Yes. And you're basically saying that you, you guys saw this limitation and then retrained BERT to use rotary embeddings. We've talked about rotary embeddings multiple times here. We had folks behind the yarn paper for extending context windows. Alibi is we follow Ophir Press. [00:14:08] I don't think Ophir ever joined ThursdAI, but Ophir, if you hear this, you're welcome to join as well. So Alibi is another way to extend context windows and I think Mosaic folks used Alibha and some other folks as well. Bo, could you speak more about like borrowing the context from there and retraining BERT to JinaBERT and whether or not JinaBERT is also open source? [00:14:28] Bo Wang: Oh, we actually want to make JinaBERT open source, but I need to align with my colleagues. That's, that's, that's really, that's a decision to be made. And the, the idea is quite naive. If you didn't know, I don't want to dive into too much about technical details, but basically the idea of Alibi basically removed the position embeddings from the large language model pre training. [00:14:55] And the Alibi technique allow us to train on the shorter sequences. But inference at every very long sequence. So in the end, I think if I, my remember is correct, the author of alibi paper, basically trained model on 512 sequence lens and 1,024 sequence lens, but he's able to inference on 16 K. 16 K, like sequence lens. [00:15:23] If you further expand it, you are not capable because that's the limitation of hardware, that's the limitation of GPE. So he, he actually tested 16 K like a sequence lens. So what we did is just. Borrowed this idea from the autoregressive models into the mask language models. And integrate Alibi, remove the position embeddings from the bird, and add this Alibi slope and all the Alibi stuff back into the bird. [00:15:49] And just borrowed the things how we train bird or something Roberta, something from Roberta, and retrained the bird. I never imagined bird could be a by product of our embedding model, but this... This happened. We could open source it. Maybe I have to discuss with my colleague. [00:16:09] Alex Volkov: Okay. So when you talk to your colleagues, tell them that first of all, you already said that you may do this on ThursdAI Stage. [00:16:15] So your colleagues are welcome also to join. And when you open source this, you guys are welcome to come here and tell us about this. We love the open source. The more you guys do, the better. And the more it happens on ThursdAI Stage, the better, of course, as well. Bo, you guys released the Jina Embedding Version 2, correct? [00:16:33] Gene Embedding Version 2 has a sequence length of 8k tokens. So that actually allows to, if, just for folks in the audience, 8, 000 tokens is, I want to say, maybe like 6, 000 words in English around, right? And different languages as well. Could you talk about multilinguality as well? Is it multilingual, is it only English? [00:16:53] How that how that appears within the embedding model? [00:16:57] Bo Wang: Okay, actually, our Jina Embedding V2 is only English, so it's a monolingual embedding model. If you look at the MTV benchmark or all the public multilingual models, they are multilingual. But to be frankly, I don't think this is a fair solution for that. [00:17:18] I think at least every major language. [00:17:24] We decided to choose another hard way. We will not train a multilingual model, but we will train a bilingual model. Our first target will be German and Spanish. What we are doing at Jina AI is we basically Fix our English embedding model as it is just keep it at is, but we are continuously adding the German data, adding the Spanish data into the embedding model. [00:17:51] And our embedding model cares two things. We make it bilingual. So it's either German, English or German English, Spanish, Spanish, English, German, English, or Japanese, English, whatever. And what we are doing is we want to build this embedding model to make it monolingual. So imagine you are, you have a German English embedding model. [00:18:12] So if you search for German, you'll get German results. If you use English, you'll get English results. But we also care about the cross linguality of this bilingual model. So imagine you, you, you encode two, two sentences. One is in German, one is in English, which they are With the same meaning, we also want these vectors to be mapped into the similar semantic space. [00:18:36] Because I, I'm a foreigner myself, sometimes, imagine I, I, I buy some stuff in the supermarket. Sometimes I have to translate, use Google Translate, for example, milk into Milch in German, then, then, then put it into the search box. I really want this bilingual model happen. And I believe every, at least, major language deserves such an embedding model. [00:19:03] Alex Volkov: Absolutely. And thanks for clarifying this because one of the things that I often talk about here on Thursday Night is as a founder of Targum, which is inside videos, is just how much language barriers are preventing folks from conversing to each other. And definitely embeddings are... The way people extend memories parallel lines, right? [00:19:21] So like a huge, a huge thing that you guys are working on and especially helpful. The sequence length is, and I think we have a question from the audience is what is the sequence lengths actually allow people to do? I guess Jina and I worked with some, some other folks in the embedding space. Could you talk about what is the longer sequence lengths now unlocking for people who want to use open source embeddings? [00:19:41] Obviously. My answer here is, well, OpenAI's embeddings is the one that's most widely used, but that one you have to do online, and you have to send it to OpenAI, you have to have a credit card with them, blah, blah, blah, you have to be from supported countries. Could you talk about a little bit of what sequence length allows unlocks once you guys release something like this? [00:20:02] Bo Wang: Okay, actually, we didn't think too much about applications. Most of the vector embeddings applications, you can imagine search and classification. You build another layer of, I don't know, classifier to classify items based on the representation. You can build some clustering. You can do some anomaly detection on the NLP text. [00:20:22] This is something I can imagine. But the most important thing I I have to be frankly to you because we are, we are like writing a technical report as well. Something like a paper maybe we'll submit to academic conference. Longer embeddings doesn't really always work. That is because sometimes if the important message is in in the front of the document you want to embed, then it makes most of the sense just to encode let's say 256 tokens. [00:20:53] or 512. But sometimes if you you have a document which the answer is at the middle or the end of the document, then you will never find it if the message is truncated. Another situation we find very interesting is for clustering tasks. Imagine you want to visualize your embeddings. Longer longer sequence length almost always helps and for clustering tasks. [00:21:21] And to be frankly, I don't care too much about the application. I think people, we, what we're offering is the, how can I say, offering is, is like a key. We, we unlock this 512 sequence length. To educate and people can explore it. People, let's say I, I only need two K then, then people just set tokenize max lens to two k. [00:21:44] Then, then embed. Based on their needs, I just don't want to be, people to be limited by the backbone, by the 500 to 12 sequence lengths. I think that's the most important thing. [00:21:55] Alex Volkov: That's awesome. Thank you. Thank you for that. Thank you for your honesty as well. I love it. I appreciate it. The fact that, there's research and there's application and you not necessarily have to be limited with the application set in mind. [00:22:07] We do research because you're just opening up doors. And I love, I love hearing that. Bo maybe last thing that I would love to talk to you about as the expert here on the topic of dimensions. Right. So dimensionality with embeddings I think is very important. Open the eye, I think is one of the highest ones. [00:22:21] The kind of the, the thing that they give us is like 1200 mentioned as well. You guys, I [00:22:26] think [00:22:26] Jina is around 500. Or so is that correct? Could you talk a bit about that concept in broad strokes for people who may be not familiar? And then also talk about the why the state of the art OpenAI is so far ahead? [00:22:39] And what will it take to get the open source embeddings also to catch up in dimensionality? [00:22:46] Bo Wang: You mean the dimensionality of the vectors? Okay, basically we follow a very standard BERT size. The only thing we modified is actually the the alibi part and some training part. [00:22:58] And our small model dimensionality is 512, and the base model is 768 and we have also a large model, haven't been released because of the training is too slow. We have so much data to change. Even the model size is small, but we have so much data and so large model dimensionality size is 1,024. And if my memory is correct, so are I embedding 0 0 2? [00:23:23] Have but dimensionality of. 1, 5, 3, 6, something like that, which is a very strange dimensionality, I have to say, but I would say the dimensionality is, is, is the longer might be more Better or more expressive, but shorter, which means when you are doing the vector search, it's gonna be much more faster. [00:23:48] So it's something you have to balance. So if you think the speed query speed, or the retrieval speed or whatever is more important to you. And if I, if I know correct, some of the Vector database, they make money by the dimensionality, let's say. They, they charge you by the dimensionality, so it's actually quite expensive if your dimensionality is too high. [00:24:13] So it's a balance between expressionist and the, the, the, the speed and the, the, the, the cost you want to invest. So it's. It's very hard to determine, but I think 512, 768, and 1024 is very common as BERT. [00:24:34] Alex Volkov: So great to hear that a bigger model is also coming, but it hasn't been released yet. So there's like the base model and the small model for embeddings, and we're waiting for the next one as well. [00:24:46] I wanted to maybe ask you to maybe simplify for the audience, the concept of dimensionality. What does it mean between, what is the difference between embeddings that were stored with 512 and like 1235 or whatever OpenAI does? What does it mean for quality? So you mentioned the speed, right? It's easier to look up nearest neighbors, maybe within the 512 dimension space, what does it actually mean for quality of look up of different other ways that strings can compare? Could you maybe simplify the whole concept, if possible, for people who don't speak embeddings? [00:25:19] Bo Wang: Okay maybe let me quickly start with the most basic version. [00:25:24] If you imagine, if you type something in the search box right now, when doing, doing the matching, and it's actually also embedding, but it's something like if I make a simple version, it's a binary embedding. Imagine there 3, 000 words in English. Maybe there are much more, definitely. Imagine it's 3, 000 words in English, then the vector is 3, 000 dimensionality. [00:25:48] Then what current solution of searching or matching do is just making... If the query has a token, if your document has a token, if your document has this token, then your occurrence will be one. If you query has the token, and this one will match your document token. But it's also about the, the frequency it appears, it's how, how rare it is. [00:26:12] But the current solution is basically matching by the. By the English word, but with neural network, basically if you know about this, for example, ResNet know about a lot of different, for example, classification models, basically the output class of item, but if you chop up the classification layer, it will give you some a vector. [00:26:36] Basically this vector is It's the representation of the information you want to encode. Basically it's a compressed version of the information in a certain dimensionality such as 512, 768, something like this. So it's a compressed list of non numerical numbers, which we normally call it dense vectors. [00:26:57] because it's much more how can I say in English dense, right? Compared to the traditional way we store vectors, it's much more sparse. There is a lot of zero, there is a lot of one, because zero means not exist, one means exist. When one exists, then there is a match, then you've got the search result. [00:27:16] So these dense vectors capture more about semantics, but if you match by the occurrence, then you might lose the semantics. But only matching by the occurrence of a token or a word. [00:27:31] Alex Volkov: Thank you. More dimensions, basically, if I'm not saying it correctly, more dimensions just have more similarity vector. So like more things two strings or tokens can be similar on. And this basically means higher match rate. For more similarity things. And I think the basic stuff I think is covered in the Simon Wilson, the first pin tweet here, Simon Wilson did a basic, basic intro into what do dimensions embeddings mean and why they matter. [00:28:00] And I specifically love the fact that there's arithmetic that can be done. I think somebody reads the paper even before this whole LLM thing, where if you take embeddings for Spain and embeddings for Germany, and then you take you, you can subtract like the embedding for Paris and then you get something closer to, to like Berlin, for example, right? [00:28:19] So there's like concepts in, inside these things that are they're even arithmetic works and if you take like King and you subscribe male, then you get something closer to Queen and stuff like this. It's really, really interesting. And also Bo, you mentioned visualization as well. It's really impossible to visualize. [00:28:36] 10, 24, et cetera, dimensions, right? Like we humans, we have perceived maybe three, maybe three and a half, four with time, whatever. And usually what happens is those multiple dimensions get down scaled to 3D in order to visualize in neighborhoods. And I think we've talked with folks from ARISE. They have a software called Phoenix that allows you to visualize embeddings for clustering and for semantics. [00:29:02] Atlas does this as well, right? Nomic AI's Atlas does this as well. You can provide dimensions as well. And so you can provide embeddings and see clustering for concepts. And it's really pretty cool. If you haven't played with this, if you only did VectorDBs and you stored your stuff after you've done chunking, but you've never visualized how this looks, I strongly recommend you to do and I think well, thank you so much for joining us and explaining to us, the internals and sharing with us some exciting things about what's to come. Jina Burt is hopefully hopefully is coming, a, a retrained version of Burt, the, the, the, the, the... The grease of all how should I say, I can't, it's hard for me to define a verb, but I see it everywhere it's, it's the big base bone of a lot of NLP tasks, and it's great to see that you guys are about to first of all, retrain it for longer sequences, using tricks like Alibi and and I think you said Positional Embeddings, and hoping to see some open source action from this, but also that Jina Embedding's large model is coming as well with more dimensions waiting for that. Hopefully you guys didn't stop training that. And I just want to tell folks why I'm excited for this. And this kind of will take us to the next. [00:30:08] Point as well is because, while I love OpenAI, I honestly do, I'm going to join their Dev Day, I'm going to report from their Dev Day and tell you all the interesting things that OpenAI does. We've been talking about we've been talking and we'll be talking today about local inference, about running models on edge, about running models of your own. [00:30:28] Mistin is here, he even works on some bootable stuff that you can like completely off the grid run. And, so far, we've been focused on open source LLMs, for example, right? So we've had I see Pharrell in the audience from Skunks Works, and many other fine tuners, like Tignium, Alignment Labs, all these folks are working on local LLMs, and they never get to GPT 4 level yet. [00:30:51] We're waiting for that, and they will. But the whole point of them is, you run them locally, they're uncensored, you can do whatever you want, you can fine tune them on whatever you want. However, the kind of the embeddings part Is the glue to connect it to an application and the reason is because there's only so much context window also context window is expensive and even if theoretically the yarn paper that we've talked with the authors of allows you to extend the context window to 128, 000 tokens The hardware requirements for that are incredible, right? [00:31:22] Everybody in the world of AI engineers, they switch up to, to, to retrieval of data generation. Basically, instead of shoving everything in the context, they switched Hey, let's use a vector database. Let's say a Chroma. Or Pinecone, or Waviate, like all of those, vectorized from Cloudflare, and the other one from Spotify there, I forget its name or even Superbase now has one. [00:31:43] Everybody has a vector database it seems these days, and the reason for that is because all the AI engineers now understand that you need to put some text into some embeddings, store them in some database. And many pieces of that were still requiring internet, requiring OpenAI API calls, requiring credit cards, like all these things. [00:32:03] And I think it's great that we've finally got to a point where, first of all there are embeddings that are matching whatever OpenAI has given us. And now you can run them locally as well. You don't have to go to OpenAI. If you don't want to host, you can probably run them. I think though GeneEmbedding's base is very tiny. [00:32:20] Like it's half like the small model is 770 megabytes, I think. Maybe a little bit more, if [00:32:27] Bo Wang: I'm looking at this correctly. Sorry, it's half precision. So you need to double it to make it FP32. [00:32:33] Alex Volkov: Oh yeah, it's half precision. So it's already quantized, you mean? [00:32:37] Bo Wang: Oh no, it's just to store it as FV16, [00:32:39] Alex Volkov: if you store it as FV16. [00:32:43] Oh, if you store it as FV16. But the whole point is the next segment in ThursdAI today is going to be less about updates and more about the very specific things. We've been talking about local inference as well, and these models are tiny, you can run them on your own hardware, on Edge via Cloudflare, let's say, or on your computer. [00:32:58] And you now can do almost end to end application wise. From the point of your user inputting a query embedding this query, running a match, a vector search, KNNN and whatever you want nearest neighbor search for that query for the user. Retrieve that all from like local open source. You basically you, you can basically go offline. [00:33:20] And this is what we want in, in the era of upcoming regulation towards what AI can be and cannot be. And the era of like open source models getting better and better. We've talked last week where Zephyr and I think Mistral News from Technium is also matching some GPT 3. 5. All of those models you can download and nobody can tell you not to run inference on them. [00:33:40] Hugging Face open sourcing a fast Text Embeddings Inference Server with Rust / Candle [00:33:40] Alex Volkov: But the actual applications, they still require the web or they used to. And now I'm, I'm loving this like new move towards. Even the application layer, even the RAG systems, which are augmented generation, even the vector databases, and even the embeddings are now coming to, to open source, coming to your local computer. [00:33:57] And this will just mean like more applications either on your phone or your computer. And absolutely love that. Bo, thank you for that. And thank you for coming to the stage here and talking about the things that you guys open sourced and hopefully we'll see more open source from Jina and everybody should follow you and, and Jina as well. [00:34:13] Thank you. It looks like. Thank you for joining. I think the next thing that I wanna talk about is actually in this vein as well. Let me go find this o Of course, we love hug and face and the thing that I think that's already on top if you look, yeah if you look at the last thing, last tweet that's pinned it's a tweet from Jeri Lou from Lama Index, obviously. [00:34:33] Well, well, well, we're following Jerry and whatever they're building and doing over at Lama Index because they implement everything like super fast. I think they also added support for Jina like extremely fast. He talks about this thing where HugInFace opensource for us something in Rust and Candlestick? [00:34:51] Candlelight? Something like that? I forgot that they're like iteration on top of Rust. Basically, the open source is a server that's called TextEmbeddingsInferenceServer that you can run on your hardware, on your Linux. boxes and basically get the same thing that you get from OpenAI Embeddings. [00:35:07] Because Embeddings is just one thing, but it's a model. And I think you could use this model. You could use this model with transformers but it wasn't as fast. And as Bo previously mentioned, there's considerations of latency for user experience, right? If you're building an application, you want it to be as responsive as possible. [00:35:24] You need to look at all the places in your stack and say, Hey. What slows me down? For many of us, the actual inference, let's say use GPT 4, waiting on OpenAI to respond and stream that response is what slows many applications down. And but many people who do embeddings, let's say you have a interface of a chat or a search, you need to embed every query the user sends to you. [00:35:48] And one such slowness there is how do you actually How do you actually embed this? And so it's great to see that Hackenface is working on that and improving that. So you previously could do this with transformers, and now they released this specific server for embeddings called TextEmbeddings Inference Server. [00:36:04] And I think it's four, four times faster. than the previous way to run this, and I absolutely love it. So I wanted to highlight this in case you are interested. You don't have to, you can use OpenAI Embeddings. Like we said, we love OpenAI, it's very cheap. But if you are interested in doing the local embedding way, if you want to go end to end, complete, like offline, you want to build like an offline application, using their internet server I think is a good idea. [00:36:29] And also it shows what HuggingFace is doing with Rust and I really need to remember what language there is but definitely a great attempt from Hug and Face, and yeah, just wanted to highlight that. Let's see. Before we are joined from the Grad. io folks, and I think there's some folks in the audience who are ready from Grad. [00:36:48] io to come up here and talk about local inference which 15 minutes left, [00:36:52] Data Provenance Initiative at dataprovenance.org [00:36:52] Alex Volkov: I wanted to also mention the Data Provenance Initiative. Let me actually find this announcement, and then quickly... Quickly paste this here, and I was hoping that Enrico can be here. . There's a guy named Shane Longfree, [00:37:05] and he released this massive, massive effort, included with many people. And basically what this effort is, it's called the Data Provenance Initiative. Data Provenance Initiative is now existing in dataprovenance. org. And hopefully can somebody maybe send me the, the direct link to the suite to add this. [00:37:23] It... It is a massive effort to take 1, 800, so 1, 800 Instruct and Align datasets that are public, and to go through them to identify multiple things. You can filter them, exclude them, you can look at creators, and the most important thing, you can look at licenses. Why would you do this? Well, I don't know if somebody who builds an application needs this necessarily, but everybody who wants to fine tune models, the data is the most important key for this, and building data sets and running them through your fine tuning efforts is basically the number one thing that many people do in the fine tune community, right? [00:38:04] Data wranglers, and now, thank you, Nishtan, thank you so much, and a friend of the pod, Enrico. is now pinned to the top of the tweet. Thank you for to the top of the space, the nest, whatever it's called. A friend of Enrico Cipolla, who we've talked previously in the context of extending I think Lama to first 16k and then 128k. [00:38:24] I think Enrico is part of the team on yarn paper as well. I joined this effort, and I was hoping Enrique could join us to talk about this. But basically, if you're doing anything with data, this seems like a massive, massive effort. Many datasets from Lion, and we've talked about Lion, and Alpaca, GPT 4L Gorilla, all these datasets. [00:38:46] It's very important when you release your model as open source that you have the license to actually release this. You don't want to get exposure, you don't want to get sued, whatever. And if you're in finding data sets and creating different mixes to fine tune different models, this is a very important thing. [00:39:03] And we want to shout out, Shane Longpre, Enrico, and everybody who worked on this because I think... Just, I love these efforts for the open source, for the community, and it just makes, it's easier to fine tune, to train models. It makes it easier for us to advance and get better and smaller models, and it's worth celebrating and ThursdAI is the place to celebrate this, right? [00:39:27] LocalLLama effort to compare 39 open source LLMs + GPT4 [00:39:27] Alex Volkov: On the topic of extreme, how should I say efforts that are happening by the community on the same topic, I want to add another one, and this one I think I have a way to pull it up, so give me just a second give me just a second, yes. A Twitter user named Wolfram Ravenwolf who is a participant of the local Lama community on Reddit and now is pinned to the nest at the top of the tweet did this massive effort of comparing open source LLMs and tested 39 different models ranging from 7 billion parameters to 70 billion, and also compared them to chat GPT, GPT 4. [00:40:06] And I just want to circle back to something we've said. In the previous space as well, and I welcome like folks on stage also to jam in here. I've seen also the same kind of concepts from Hug and Face folks. I think Glenn said the same thing. It's really unfair to, to take a open source model like Mistral7b and then start comparing this to GPT 4. [00:40:26] It's unfair for several reasons. But also I think it, it, it can obfuscate to some people when they do this comparison of how, just how advanced we've come for the past year in open source models. OpenAI has the infrastructure, they're backed by Microsoft, they have the pipelines to serve these models way faster. [00:40:47] And also, those models don't run on like local hardware, they don't run on like one GPU. It's like a whole, a whole... Amazing MLOps effort to bring you this speed. When you're running local source model open source models locally when they're open source, they're, they're, they're small, there's drawbacks and there's like takeaways that you have to bake in into your evaluation. [00:41:09] So comparing to the GPT 4, which is super general in many, many things, that will just lead to your disappointment. However, and we've been talking about this like with other open source models If you have a different benchmark in your head of if you're comparing open source to open source, then it's a whole completely different ballgame. [00:41:26] And then you start seeing things like, Hey, we're noticing that the 7 billion parameter model is, beating 70 billion. We're noticing that size is not necessarily the king because if you guys remember, Three months ago, ni, I wanna say we've talked about Falcon 180 B. 180 B was like, three times the size of like the, the, the next largest model. [00:41:47] And it was incredible the Falcon Open source this, and then it was like, like a wo like, no, nobody really was able to run 180 B because it's huge. But also once, once we did run it, we saw that like the difference between that and LAMA are not great at all. Maybe a few percentage points on, on the valuations. [00:42:04] However, the benefits that we see are from local, like for tinier and tinier models from like 7D Mistral, for example, which is the, the one that the fine tuners of the world are now preferring to everything else. And so the kind of, when you're about to evaluate whatever next model that's coming up that we're going to talk about please remember that Comparing to large, open, big companies backed by billions of dollars that run on multiple split hardware, it's just going to lead to disappointment. [00:42:34] However, when you do some comparisons, like the guy did, that is now pinned to the tweet this is the way to actually do this. However, on specific tasks, like for, say, coding go ahead, [00:42:46] Nisten Tahiraj: Nisam. I was going to say, we're still a bit early to judge for example, Falcon could have used a lot more training. [00:42:53] There's also other. parts where larger models play a big effect stuff like if you want to do very long context summarization then you want to use the 70b and as far as i'm getting it and this is probably inaccurate right now but the more tokens you have the more meat you have in there the Then the larger the thoughts can can be. [00:43:23] So that's the principle which are going by. Well, Mistral will do extremely well in small analytical tasks and in benchmarks, and it's amazing as a tool. It doesn't necessarily mean that it'll be good at thinking big. You still need The meat there, the amount of tokens to do that. Now you could chop it up and, and do it one, one at a time, but anyway, just something to keep in mind because lately we also saw the announcement from Lama70Blong, which started getting really good at at summarization. [00:44:04] So again, there's one particular part. Which is summarization where you, it looks like you need longer you need bigger models for that. And I've tested it myself with Falcon and stuff, and it's pretty good at summarization, I just want to also give them the benefit of the doubt that there is still something that could be done there. [00:44:28] I wouldn't just outright dismiss. [00:44:31] Alex Volkov: Yeah, absolutely, absolutely, and I want to join this non dismissal. Falcon open sourced fully commercially like Falcon 70B before, and this was the biggest open source model at the time. And then they gave us 180B, they didn't have to, and we appreciate like open sourcing. [00:44:46] We're not going to say no. Bigger models have more information, more, more, maybe world model in them, and there's definitely place for that, for sure. The, the next thing you mentioned also, and I think I, I strongly connect to that, Nissen, and thank you, is GPT 4, for example, is very generalized. It does many, many, many things well. [00:45:08] It's like kind of impressive and whatever Gemini is going to be from Google soon, hopefully, we're always waiting on ThursdAI, that the breaking news will come on Thursday. We're gonna be talking about something else and then Google suddenly drops Gemini on us. There's also other rumors for Google's other stuff. [00:45:22] Whatever OpenAI's Arrakis was, and then they stopped training, and whatever next they're coming from OpenAI, will probably blow everything we expect in terms of generality out of the water. And this, the open source models, as, as they currently are, they're really great at... Focused tasks, right? So like the coder model, for example that recently Glaive Coder was released by Anton Bakaj, I think is doing very well on the evaluations for code. [00:45:51] However, on general stuff, it's probably less, less good. And I think for open models expecting generality on the same level as GPT 4, I think, is, is going to lead to disappointment. But for tasks, I see, I think we're coming close to different things that a year ago seemed state of the art. If you guys remember, it's not even a year since JetGPT was released, right? [00:46:14] I think JetGPT was released in November? No, not as an API even, just the UI, like middle of November. So we're coming up on one year, I think the Dev Day will actually be one year. That was 3. 5. 3. 5 now, many people use 3. 5 for applications, but, you want to go for 4. If you're paying for Chattopadhyay Plus and you have a task to solve, you're not going to go 3. [00:46:35] 5 just because you feel like it. You know that 4 is better. But now we're having open source models way smaller. They're actually getting to some levels of 3. 5, and the above effort is actually an effort to try to figure out which ones. And so I strongly recommend, first of all, to get familiar with local Llama subreddit. [00:46:54] If you don't use Reddit, I feel you, and I've been a Reddit user for a long time, and I stopped. Some parts of Reddit are really annoying. This is actually a very good one, where I get a bunch of my information outside of Twitter. And I think Andrej Karpathy also recommended this recently, which... Then became an item on that subreddit. [00:47:12] It was really funny. And this massive effort was done by this user and he, he did like a full comparison of just 39 different models. And he outlined the testing methodology as well. We've talked about testing and evaluation methodology. Between ourselves, it's not easy to evaluate these things. A lot of them are like gut feeling. [00:47:31] A lot of the, the evaluation, and Nathan and I have like our own prompts that we try on every new model, right? It's, it's like a lot, a lot of this for many people is like gut feel. And many people also talk about the problem with evals and I think Bo mentioned the same thing with the embedding leaderboards that then, you know. [00:47:48] It then becomes like a sport for people to like fine tune and, and release models just to put their name on the board to overfit on whatever whatever metrics and evaluations happen there. And then there's a whole discussion on Twitter whether or not this new model that beats that model on, on some, some score actually, was trained on the actual evaluation data. [00:48:09] But... Definitely the gut feeling variation is important and definitely having different things to test for is important. And you guys know, I think, those of you who come to ThursdAI, my specific gut feels are about like translation and multilingual abilities, for example, and direction following some other people like Jeremy, Jeremy Howard from ThursdAI have his own like approach. [00:48:29] Everybody has their own approach. I think what's interesting there is... Kind of the community provides, right? We're like this decentralized brain of evaluating every new model. And for now, the community definitely landed on Mistral as being like the top. At least a model in the 7b range, and Falcon, even though it's huge and can do some tasks like Nissan said is less, less and Lama was there before. So if you start measuring the community responses to open source models, you start noticing better what does what. And this effort from this guy, he actually outlined the methodology, and I want to shout out... Friends of the pod, Tignium being the go to many, many things, specifically because Open Hermes, which, Hermes, which we've talked about before which was fine tuned from Mistral7b is probably like getting the, the, the top leaderboard from there, but also based on my experiences, right? [00:49:22] So we've talked last week about Open Hermes being able, you're able to run Open Hermes on your... Basically, M1, M2, Max with LM Studio, which also shout out to LM Studio, they're great, and I've tested this, and this seems to be, like, a very, very well rounded model, especially for one that you can run yourself and comparing to GPT 4 and other stuff, this model for specific things is really great. [00:49:45] It's good for coding. It's not the best for coding. I think there's a coding equivalent. And I just really encourage you, if you're interested, like figuring out what to use. And we've talked about this before. What to use. Is an interesting concept, because if you come to these spaces every week and you're like, Oh, this model is now state of the art, that model is state of the art. [00:50:05] You may end up not building anything, because you just won't have the, you always keep chasing the latest and greatest. The differences are not vast from week to week, we're just seeing like better scores. But it's well worth checking out this effort for the methodology, for the confirmation that you have. [00:50:21] Let's say you, you felt that Mistral is better and now you can actually understand. And also for friends of the pod I think John Durbin is also, Error Boris model is really great and it's also up there. And what Nistan highlighted is that bigger models sometimes excel at different things some summarization or just more knowledge. [00:50:38] It's also outlined there as well. And You can also see models that are not that great, that maybe look good on the leaderboards, but don't necessarily perform as well, and you can see them as well in that effort. [00:50:49] So maybe actually let me reset the space. Everybody who joined in the middle of me speaking is like, why is this guy speaking? [00:50:56] And what's going on here? You are welcome. You, you're in the space of ThursdayAI. ThursdayAI we are meeting every week to talk about everything that happens in the world of AI. If you're listening to this and you're enjoying, you're the target audience, but generally we talk about everything from open source LLMs and now embeddings. [00:51:13] We, we talk about big company APIs. There's not a lot of updates from OpenAI this week. I think they're quiet and they're going to release everything in a week and a half in their dev day. And, and Tropic obviously, and, and... Cloud and Microsoft and Google, like all these things we cover as much as possible. [00:51:29] We also cover voice and audio. And in that vein, I want to shout out to friends from Gladia and I'll pin there actually, let me just pin this right now. Gladia just released a streaming of Whisper and I've been waiting for something like this to happen. Sometimes for AI engineers, you don't want to host everything yourself. And you want to trust that, the, the WebSocket infrastructure is going to be there when you don't want to build it out. And I'm not getting paid for this. [00:51:53] This is like my, my personal, if I had to implement like something like the voice interface with ChatGPT, I would not build it myself. I would not trust my own MLOps skills for that. And so for that Gladia is, I've been following them since I wanted to implement some of their stuff and they just implemented like a WebSocket. [00:52:11] Whisper transcription streaming, and it's multilingual, and it's quite fast, and I definitely recommend folks to check it out. Or check out my review of it, and try out the demo, and if you want it, use it. Because we've talked last week about the interface for ChatGPT that's voice based, and you can actually have a FaceTime call with ChatGPT, and that's incredible. [00:52:30] And I think more and more removing the screen out of this talking to your AI agents, I think, with the latest releases also in text to speech, like 11 Labs and XTTS that we've covered as well. With advances there, with speed, you can actually start getting interfaces where you can talk, and the AI listens and answers back to you very fast. [00:52:52] Worth checking out, and definitely an update. Thank you. [00:52:57] Nisten Tahiraj: Okay. So this is a complete product. I was, [00:53:00] Alex Volkov: yeah, this is a full, pay a little bit, get a WebSocket and then you use this WebSocket to just like stream and you can embed this into your applications like very fast. Setting that up, I think Koki, you can do this with Koki, which we also covered. [00:53:13] Gradio Interview with Abubakar, Xenova, Yuichiro [00:53:13] Alex Volkov: Alright, I think it's time to again, reset the space. ThursdAI I wanna thank Bo who is still on stage. Bo, you're welcome to keep, stay with us a little bit and now we're moving on to the second part of this. [00:53:30] Welcome, Abubakar. Welcome, Zinova, Joshua. Welcome some folks in the audience from Hugging Face. It's great to see you here on ThursdAI, well, Zinova is always here, or hopefully, but Abubakar, I think this is your first time. [00:53:41] I'll do a brief intro, and then we can, we can go and talk about Gradio as well. [00:53:45] I my first inference that I ran on a machine model was a year and something ago, and this was via Gradio, because I, I got this weights file, and I was like, okay, I can, I can probably run something with CLI, but how do I actually visualize this? And back then, Gradio was... was the way and I think since then you already guys you were already part of Hug and Face and Everybody who visited a model page and tried a demo or something probably experienced Gradua even without knowing that this is what is behind all the demos So welcome, please feel free to present yourself. [00:54:17] Give us Maybe two line, three line of how you explain Gradio to folks, and then we can talk about some exciting stuff that you guys have released this week. [00:54:25] Abubakar Abid: Awesome. Yeah, first of all, thank you again for, for having me and for having several folks from the Gradio team here. I've known you, Alex, for a long time. [00:54:32] I think you were one of the early users of Gradio or at least one of the early users of Gradio blocks and, and some of these viral demos. So I've seen, this podcast develop over time and it's It's a real honor to be to be able to come here and to be able to talk about Gradio. [00:54:45] Yeah. Hi everyone. I'm Abu Bakr. I'm, I lead the Gradio team at Hugging Face. So Gradio is basically the way we describe it is it's the fastest way to, to build a GUI or an app from a machine learning model. So traditionally have, taking a machine learning model to production or at least letting... [00:55:01] Users try it out has meant that you need to know a lot of front end. You need to know how to, setting up a server, web hosting. You have to figure all of these things out so that other people can play around with your machine learning model. But Gradio lets you do all of that with just a few lines of Python as I think Joshua was mentioning earlier. [00:55:18] And Gradio has been used by a lot of people. We're very lucky that, we kind of coincide. We started Gradio a few years ago late 2019. It grew out of A project at Stanford, and then spun out to be a startup, and then we got acquired by Hugging Face, and we've been growing Gradio within that kind of ecosystem. [00:55:32] But we're very lucky because during this time has coincided with a lot of real developments in machine learning. I come from an academic background, so before 2019 I was doing my PhD at Stanford. And, everyone's been doing, machine learning for a while now, but... [00:55:45] The types of machine learning models that people wanted to build, you built it, you published a paper and that was it, but, since then, recently people are building machine learning models that other people actually want to use, other people want to play around with, things have gone very, exciting, and so that's led to a lot of people building radio demos I think, I was looking at the the stats recently we have something around more than three, four million demos, Gradio demos that have been built since we started the, library. [00:56:09] And yeah, so recently we released something called Gradio Lite, which lets you run... [00:56:13] Gradio effects on the open source LLM ecosystem [00:56:13] Alex Volkov: Wait, before, before, Abubakar, if you don't mind, before Gradio Lite, let's not I just want to highlight how important this is to the ecosystem, right? I'm oriJinally a front end engineer I do component libraries for breakfast, and basically, I don't want to do them it's really nice to have a component library maybe Tailwind UI, or ShadCN, like, all these things, so even front end engineers, they don't like building things from scratch. [00:56:35] Switching to machine learning folks who like build the model, let's say, and want to run some inference, that's not their cup of tea at all. And, just thinking about like installing some JavaScript packages, like running NPM, like all these things, it's not like where they live at all. And so what Gradio allows us to do this in Python. [00:56:51] And I think this is, let's start there. That's on its own is incredible and lead, led to so many demos just look to happen in Gradio. And you guys built out pretty much everything else for them, like everything that you would need. And I think recently you've added stuff, before we get to Gradual Light, like components like chat, because you notice that, many people talk to LLMs, they need the chat interface, right? [00:57:10] There's a bunch of multi modal stuff for video and stuff. Could you talk about, the component approach of how you think about providing tools for people that don't have to be designers? [00:57:20] Abubakar Abid: Yeah, absolutely. So yeah, that's exactly right. Most of the time when you're, machine learning, developer you don't want to be thinking about writing front end, components that coupled with some, an interesting insight that we had with machine learning models. [00:57:31] It's much more like the components from machine learning models are tend to be much more usable than in other kinds of applications, right? So one thing I want to be clear is that Gradio is actually not meant to be like a, build web apps in general in Python. That's not our goal. Our goal, we're heavily optimized toward building machine learning apps. [00:57:50] And what that means is, the types of inputs and outputs that people tend to work with are a little bit more, contained. So we have a library right now of about 30 30, Different types of like inputs and outputs. So what does that mean? So things like images, image editing video inputs and outputs, chatbots as outputs JSON, data frames, various types of inputs and outputs that components that come prepackaged with Gradio. [00:58:15] And then when you build a Gradio application, you basically say, Hey, this is my function. These are my inputs, and these are my outputs. And then Gradio takes care of everything else, stringing everything together sending them, message back and forth, and pre processing, post processing everything in the right way. [00:58:29] So yeah, you just have to define your function in the backend, and your inputs and your outputs, and then Gradio spins up a UI for you. [00:58:36] Alex Volkov: And so I really find it funny and I sent the laughing emoji and said that Gradio was not meant to build web apps, like full scale web apps, because I think the first time that we've talked, you reached out, because I joined whatever open source that was running for stable diffusion, this was before automatic, I think, and you told me hey, Alex, You did some stuff that we didn't mean for you to do, so I injected like a bunch of JavaScript, I injected a bunch of CSS, like I had to I had to go with my full on like front end developer, I was limited with this thing, and so I, even despite the limitation, I think we did like a bunch of stuff with just like raw JavaScript injection, and since then I think it's very interesting, you're mentioning like Gradio demos, Gradio demos, Automatic 1. [00:59:16] 1. 1, which is maybe for most people, is the only way they know like how to run stable diffusion, is now getting investments from like NVIDIA and getting right, I saw like a bunch of stuff that Automatic does, so it's very interesting like how you started and how the community picked it up. So can you talk about like the bigger parts of this, like Automatic and some other that are like taking Gradio and pushing it to the absolute limit? [00:59:37] Abubakar Abid: Yeah, absolutely. So that's yeah we're, we're, I'm, I'm, like, perpetually shocked by Automatic 111, every time I see a plug in, or, kind of the, the I think, like you said NVIDIA, now IBM, or something, released a plug in for Automatic 111? It's crazy. But yeah, so basically it's ever since we started Gradio, we've been noticing that, okay, okay, Gradio seems to work for 90 percent of the use cases, but then the last 10 percent people are pushing the limits of, of what's possible with Gradio. [01:00:06] And so we've progressively increased what's possible. So in the early days of Gradio, there was actually just one class called Interface. And what that did was it allowed you to Specify some inputs and some outputs and a single function. And we quickly realized, okay, people are trying to do a lot more. [01:00:20] So then about a year and a half ago, we released grad your Blocks, which allow you to like have arbitrary layouts. You can have multiple functions, string together connect inputs and in different ways. And that is what kind of allowed these very, very complex apps like automatic 1 1 1 SSD Next, and the equivalence in other domains as well. [01:00:36] Of course, like the, the text, the text web, the, the UBA Booga. Text UI as well and then there's also similar kind of, very complex demos in the audio space as well. And music generation as well. So like these super complex, multiple tabs, all of that, that's possible with this new kind of architecture that we laid out called GradioBlox. [01:00:55] And GradioBlox is this whole system for specifying layouts and and, and functions. And it's defined in a way that's intuitive to. Python developers, the, we like a lot of these like web frameworks in Python have, have popped up. And one of the things that I've noticed as someone who knows Python, but really not much JavaScript is that they're very much coming in from the perspective of a JavaScript engineer, and so like this kind of React inspired kind of frameworks and, and stuff like that. [01:01:21] And, and what, that's not very intuitive to a Python developer, in my opinion. And so we've defined this whole thing. Where you can, have these, build these arbitrary web, kind of web apps, but still in this Pythonic way. And we're actually about to take this a step farther, and maybe I can talk about this at some point but next week we're going to release Gradio 4. [01:01:38] 0, which takes this idea of being able to control what's happening on the page. To the next level. You can have arbitrary control over the ui, ux of any of our components. You can build your own components and use them within a Grady app app and get all of the features that you want in a grad app. [01:01:52] Like the, the, API usage, pre-processing, post-processing. Everything just works out of out of out of the box. But now with your own kind of level of control, yeah. Awesome. [01:02:01] Alex Volkov: And it's been honestly great to see just how much enablement. Something like as simple as Gradio for folks who don't necessarily want to install npm and css packages. [01:02:11] There's not much enablement this gave the open source community because People release, like you said, different significant things. Many of them, maybe you are not even aware of, right? They're running in some Discord, they're running in some Reddit. It's not like you guys follow everything that happens. [01:02:23] Gradio local URL via Gradio Proxy [01:02:23] Alex Volkov: Additional thing that I want to just mention that's very important that. When you run Gradio locally, you guys actually expose it via like your server, basically my local machine. And that's been like a blast that that's been like a very, very important feature that people may be sitting behind the proxy or everything. [01:02:39] You can share your like local instance with some folks, unfortunately only for 72 hours. But actually [01:02:44] Abubakar Abid: that's about to change. So in 4. 0, one of the things that we're trying to get, so actually, we've been very lucky because Gradio has been developed along with the community. Like you said, like often times we don't know what people are using Gradio for until, they come to us and tell us that this doesn't work, and then they'll link to their repo and it's this super complex Gradio app and we're like, what? [01:03:01] Okay, why are you even trying that? That's way too complicated. But, but, then we'll realize like to the extent to what people are building. And so this you mentioned the share, these share links as well, which I want to just briefly touch upon. So one, one of the things that we released in like the early days of, of, of Gradio is we realize People don't want to worry about hosting their machine learning apps. [01:03:19] Oftentimes you want to share your machine learning app with your colleague. Let's say you're like the engineer and you have a colleague who's a PM or something who wants to try it out. Or it might be if you're in academia, you want to share it with fellow researchers or your professors, whatever it may be. [01:03:33] And like, why do all of this hosting stuff if you just are, are, like, building an MVP, right? So we built this idea of a share link. So you just, when you launch your Gradio app, you just say share equals true. And what that does is it creates a it uses something called Fast Reverse Proxy to actually expose your local port to a to this FRP server which is running in a public... [01:03:53] Machine, and what that does is it forwards any request from a public URL to your local, port. And, what the, in a, the long story short, what that does is it makes your Gradio app available on the web for anyone to try. It runs for 72 hours by default, but now what we're doing as part of 4. [01:04:08] 0, we'll, announce this, is you can actually build your own share servers. So we have instructions for how to do that very easily and you can point your Gradio instance to that share server. So if you have an EC2 instance running somewhere, just point to it and then you can have that share link running for as long as you want and you can, share your share servers with other people at your company or your organization or whatever it may be and they can use that share link and, again, they can run for however they want. [01:04:30] Wait, [01:04:31] Nisten Tahiraj: wait, wait, is this out? Which branch is this? Is [01:04:34] Abubakar Abid: this going to be out? This is going to be out on Tuesday for Gradio 4. 0 we're, we're going to launch on Tuesday. [01:04:41] Nisten Tahiraj: It's like the most useful feature I'd say of, of Gradio, especially when you make a Google collab that you want people to just run in one click. [01:04:49] And like, how are they going to even use this model? And you just throw the entire Gradio interface in there and you share equals true. And then they know, they can just give it, give the link to their friends and stuff. It's really, it makes it really easy, especially with Google Colab. But now that you can host your own, this is huge. [01:05:09] This is going to... to another level. I have more questions for [01:05:14] Alex Volkov: Google. I think I just Nissen, thank you. I just want to touch upon the Google collab thing. I think at some point Google started restricting how long you can run like a collab for, and I think you guys are the reason. This exact thing that Nissen said. [01:05:30] People just kept running the Gradio thing with the URL within the Google collab and exposing like stable diffusion. They didn't build collab for that, and I think they quickly had to figure out how to go around this. [01:05:41] Abubakar Abid: Yeah. And their approach is like literally blacklisting the name of the the of specific, GitHub repos, which, I, I completely understand where, where Colab is coming from, right? [01:05:50] They're giving these GPUs for free. They have to have to prioritize certain use cases, but we're working with the Colab team and we're seeing if, there's ways, like right now it's like a blacklist on, on automatic one on one, and some other repos. So we're hoping we can find another way that's not That's not so restrictive. [01:06:05] Nisten Tahiraj: No, but it still works. You can just fork the repo. It works for everything else. It works for LLMs. So if anybody else really needs it. Gradio works on Colab. Well, as far as language stuff goes, I haven't done that much. [01:06:18] Abubakar Abid: Yeah, so Gradio works on Colab for sure. And, and that's, and that's early on, like one of the decisions we had to make actually was... [01:06:25] Should we use like, the default python runtime or should we like change, like the interpreter and stuff like that? Because building GUIs is not necessarily python's like strength, and like oftentimes you wanna render re-render everything, and you, you wanna do certain things that may not be like what Python is suited for. [01:06:42] But early on we decided, yeah, we wanna stick with the default python runtime because. One of the reasons was things like Colab, because we wanted people to be able to run Gradio wherever they normally run Python without having to change their workflows. And Colab, Gradio works in Colab. [01:06:56] We had to do a lot of... Trickery to make it work. But yeah, it works. It's just like these certain very, very specific apps that have become too popular and apparently consume too many resources. They're blacklisted by Colab right now. [01:07:10] Local inference on device with Gradio - Lite [01:07:10] Alex Volkov: Alright thank you for this intro for Gradio. To continue this, we have on stage Zinova who introduced himself, authors of TransformerJS, we've been talking with Bo in the audience, also somebody who's like just recently open sourced, with Jina, the embeddings model, and everything that we love to cover in ThursdAI, a lot of it is talking about As open source, as local as possible, for different reasons, for, not getting restricted reasons. [01:07:36] And you guys just recently launched Gradio Lite, and actually we have Yuichiro here on stage as well. So I would love to have you, Abubakar introduce and maybe have Yuichiro then follow up with some of the stuff about what is Gradio Lite? How does it relate to running models on, on device and open source? [01:07:52] And yeah, please, please introduce it. [01:07:54] Abubakar Abid: Yeah, absolutely. Yeah. Like you mentioned, I think one of the things that we think about a lot about at Gradia is like, it's the open source ecosystem and, right now, for example, where can open source LMs, for example, really shine and things like that. [01:08:06] And one of the places is on device, right? On device or in browser is, open source has a huge, edge over proprietary models. And so we were thinking about how can Gradio be useful in this setting. And we were thinking about the in browser application in particular. And we were very, very lucky to have Yuchi actually reach out to us. [01:08:25] And Yuchi has this, fantastic tracker, but if you don't already don't know Yuchi, he built Streamlit Lite, which is a way to run Streamlit apps in the browser. And then he reached out to us and basically had this idea of doing something similar with Gradio as well. And basically, I, almost like single handedly refactored much of the Gradio library so that it could run. [01:08:43] In, with Pyodide, in WebAssembly, and basically just run in the browser. I'll let Yuchi talk more about that, but basically, if you know how to use Gradio, then you know how to use Gradiolite. You just write the same Python code, but wrap it inside Gradiolite tags, and then it runs within the browser, like in the front end. [01:08:59] You can execute arbitrary Python, and it just works. Yuchi, if you want to share a little bit more. About that, yeah, or introduce yourself. [01:09:08] Yuichiro: All right, Hey, can you hear me? Well, thank you very much, thank you very much for the quick short interaction about Gradual Light and Streaming Light 2. [01:09:15] Well, as Abhakal explained about it, [01:09:18] there was [01:09:18] Sorry. OriJinally there was kind of a, a tech technological movement about edge computing or Python. It was started by uh ide that was c Python runtime compiled for web assembly that can completely run on web browsers. It started, it triggers the big band of edge computational Python, random starting with project that was. [01:09:43] That was already deported to Web Assembly runtime as D Light, and it inspired many other Python frameworks, including Streamlet and any other existing Python frameworks. I dunno uh pet script or. HoloVis panel or Shiny for Python, something like that. So there was a huge movement about to, to make Python frameworks to compatible with WebAssembly or web browser environment. [01:10:13] And I thought that was a great, opportunity to make machine learning or data science stuff completely run on web browser, including transformer things or many more stuff existing in the stream machine learning ecosystem. And I first created a Streamlit Lite that was forked version of Streamlit to WebAssembly. [01:10:36] And yeah, the, remaining story were the same as what Abhakaal introduced. So technically it was not, my oriJinal stuff, but there was a huge movement about such kind of stuff. And I, simply followed that. flow and my the transfer such kind of analogies to gradial repository. [01:10:58] Alex Volkov: Yeah, that's it. Awesome. Thank you so much. And okay. So can we talk about what actually do we do with now the ability to run Gradio all in the browser? Could maybe both of you give some examples and then I would like also to to add Zenova to the conversation because much of the stuff is using Transformers. [01:11:18] js, correct? Can we maybe go and talk about what is now actually possible compared to like when I run Gradio on my machine with a GPU and I can run like Stable Diffusion? I just [01:11:27] Nisten Tahiraj: want to say that this is crazy that this can happen at all for the audience to [01:11:32] Abubakar Abid: prepare. Yeah, I was honestly blown away the first time Yuchi showed me a demo as well. [01:11:36] Imagine you have a, any sort of machine learning model. Practically, not almost anything, but a super, really good speech recognition model running completely in your browser. Meaning that, for example, now you can take that demo, you can put it inside GitHub Pages. [01:11:51] You can host it inside. We've seen people embed Gradio demos now with Gradio Lite inside Notion. So you have a Notion whatever page, you can take that demo, you can embed it inside Notion. One of the things that we launched when we launched Gradio Lite at the same time is we also launched something called the Gradio Playground. [01:12:07] Now the Gradio Playground, you can actually just Google this, you can find this. But basically what it allows you to do is it allows you to write code in the browser. And as you're editing the code, you can see live previews of your Gradio application. And, and basically what's happening is, is taking that Gradio code, it's wrapping it inside Gradio Lite tags, and it's just running it. [01:12:27] It's just straightforward application of Gradio Lite. And, we're excited by this personally just because if one, it opens up, it allows us to write interactive documentation. You can write, you can try stuff, you can, you can immediately, see the results. We're also excited because we've seen interest from other libraries including, for example, SacketLearn, who want to embed Gradio demos within their documentation. [01:12:49] Within their docs, right? But they're they were hesitant before because they didn't want to have a separate server running these radio applications and have to worry about maintaining those servers, making sure they were up all the time, making sure they could handle the load. Now they can write it in their docs and they're like, their, their demos and everything, they'll just run in the user's browser. [01:13:07] They won't have to worry about maintaining everything since it's, in the same code base and everything. So I think that's another kind of cool application that we're excited by is just... These potential for interactive documentations that, maybe potentially other, other maintainers or other libraries might want to include. [01:13:22] So yeah, so stuff like, security, privacy, serverless type stuff, hosting, and all of that. And then also like these interactive documentations. [01:13:30] Alex Volkov: I think the demo that you mentioned with the transition within Notion from VB from Hive Interface, I think that was great. I'm trying to find the actual link, but basically, because Notion allows to embed like basically iframes, right? [01:13:42] So he embedded this whole Gradio Lite interface to translate. I think using... Burt or something like very similar that all runs within like the notion page. I think that's awesome. Joshua, you want to chime in here and say how transformers is built into this and now this allows for way more people to use transformers in like a UI way. [01:14:02] Transformers.js integration with Gradio-lite [01:14:02] Xenova: Yeah, sure. So first of all, literally nine, like almost, I would say the whole. Everything that we are talking about now has been, like, [01:14:12] Abubakar Abid: led by the Gradio team. [01:14:14] Xenova: And I am here piggybacking and be like, Whoa, look at this, Transformers JS is now working. That's really not what we're talking about today. [01:14:23] It's the amazing work that the team has been able to do. To achieve the past, this has been going on for, for quite a while. It's been like codenamed like Gradioasm and now finally being released as as GradioLite and now. Sort of like the Transformers J side of it. Just oh, by the way there's this library called Transformers J you can sort of use it and, and with the, the Transformers. [01:14:48] Oh, was that ? Sorry. You've been way too humble. [01:14:51] Abubakar Abid: No, no, absolutely [01:14:52] Xenova: not. I think it's, it's, it's so much has been done by, by you and your, and, and the amazing radio team that's it, it just so happens to be that these things are like coinciding. And now you can end up using Transformers. js with, with Gradio and Gradio Lite. [01:15:07] And obviously this is also made possible by, okay, everyone, everyone stick with me. It's going to be a little, get a little complicated when I try to explain this. But Transformers. js Pi, which is, are you ready? A JavaScript port of a Python library turned into a JavaScript library so that I can run in a Python environment. [01:15:29] Okay. We all caught up? That's, that's Transformers. js. py, which is which, which Yushi wrote in the audience obviously with his experience with streamlets bringing streamlets to the browser. It's sort of his, his invention, which is quite funny, but that's sort of how Transformers. js is able to be run. [01:15:49] Inside Gradiolites there are other ways, but from what you'll see in the documentation, that's sort of like the, the go to way. And it's [01:15:57] Alex Volkov: Yeah, I wanna ask about this, because I saw from Transformers. js, import import on Discord Transformers. js. [01:16:04] So maybe you should, could you talk about this part that, that Zenova tried to explain? It was, like, a little A little complex Transformer. js is you can install it through npm and then run this, right? And then it runs in the, in the node environment and browser environment. Gradualite is basically Python within JavaScript. [01:16:19] So then you have to turn Transformers into Python in order to get it into Gradualite so that it runs within the JavaScript context again? Is that, is that correct? Am I getting this right? [01:16:30] Nisten Tahiraj: If I could say something for the audience, what has, what's happening here is that there's a layer called Pyodide and that uses kind of like what WebAssembly uses to run Python at native speeds. [01:16:44] So it runs in the browser. And it goes down that stack, there's like a virtual machine and compiler, all that stuff in there. And then that's how Python is able to run at native speed. And this means that with PyScript, you can have inside the same index. html, just your regular index. html, you can have your JavaScript code and your objects and stuff. [01:17:06] And you can have just straight Python code in there. Like you just add the tag. You just dump the Python as is nothing else. And the crazy part is that it can access JavaScript objects now. So you can do the math in Python in the browser, 'cause JavaScript can do math well again, but, and then you can access those objects. [01:17:30] So this is a whole crazy stack here with PIO IDE and EM scripting. And again, that's only WebAssembly. So that's CPU. Only for now, because there's still a mountain of work to get, and to finish it off, Emscripten is like your POSIX layer, like your Unix layer. It's like there's an operating system being built inside the browser here that's going on. [01:17:54] So that's why things are getting complicated, but yeah, just to keep that in mind, that's the base. [01:17:59] Yuichiro: Yeah, but, what Nisten talked about was everything, because we can access the JS object from Python world inside the browser if you import transformer. [01:18:10] js. py on Gradle Lite, under the hood, transformer. js is now still being imported in the browser environment. And what... When you write a Python code as a Gradle like application on the browser, what you do is, simply using the oriJinal JavaScript, JavaScript version of Transformer. js, just proxy from the Python code through the, proxying mechanism provided by Pyodite. [01:18:42] What Transformer. js. py does is just a, thin, Proxying layer or some glue code between bridging these two, two words, Python and JavaScript. That's it. [01:18:56] Abubakar Abid: Yeah, I just zooming out a little bit. So basically what, what Transformers. js underscore pi does, it lets you run everything that Transformers. [01:19:03] js does. And what Transformers. js does, it lets you run a lot of the models, a lot of the tasks. There's a lot of the models there, you can now run in your browser, right? We're talking about all of the NLP related tasks, like things like translation, LLMs, but also, a lot of the vision tasks, a lot of the audio stuff. [01:19:22] We're talking about speech recognition that's powered by Transformers, what Josh has been doing with Transformers. js. And I think Transformers. js just released, even for example, speech generation. Text to speech. And so now you can do that within Transformer. js, which means you can do it within Transformer. [01:19:34] js Pi, which means now you can do it within Gradial Light as well. [01:19:40] Alex Volkov: That's incredible. And I think the biggest part for me is that... Now that you guys ported Gradio, which is ubiquitous in machine learning, and everybody who releases the model uses either this or Streamlit, but I think it's, it's a clear winner between the two, as this is, as I'm concerned and as I see, then now you basically ported the same thing towards the browser, and the more we see, Models getting smaller and we've been always talking about this models getting smaller, models being uploaded to the browser. [01:20:08] Browsers [01:20:09] Abubakar Abid: getting more powerful and, [01:20:10] Alex Volkov: and WebP is more browser getting more powerful. Yeah. And yeah, I'm getting, I'm getting to web GPU because we have backend here, Arthur, on stage. And I would love to introduce you guys if, unless you're already familiar. The more we see this move, the more like the need for something like a component library that's built in is, is very interesting. [01:20:25] Even though this world already has a bunch of libraries. But you're basically, with this, you're also porting the people with the experience of Gradio, right? With the existing with the existing frameworks, with the existing Gradio interfaces, to this world. I find it very exciting, so thank you. [01:20:38] And I want to introduce Arthur. Arthur, feel free to unmute yourself and maybe introduce yourself, briefly, and then yeah, feel free to chime in to this conversation. [01:20:46] Arthur Islamov: Okay, so I did quite a lot of things with ONNX to create the Diffuser. js library and to load stable diffusion in the browser, and now I'm working on the SDXL version. [01:20:58] So I was going to ask, do you know if there are some plans on adding WebGPU backends for PyTorch? Because when it happens... It'll be so much easier as web GPU backend can be launched on any platform, not even in the browser, but also locally without the browser, just using the metal backend the direct ticks or Vulcan or Linux. [01:21:28] So I guess when that happens, we'll go to a whole new era as you'll be able to run those PyTorch models in the browser with GPU acceleration. [01:21:40] Xenova: I can tag on to this. The TLDR of it is it's not at the point... [01:21:46] Where, sort of, I'm sort of comfortable with upgrading the ONNX Runtime web Runtime, basically, to support the WebGPU backend right now, just because there's quite a few issues still left, like left, so we'll see. To solve before we get to the point where you can start running these models completely on WebGPU. [01:22:07] The main, I think, the current issue at the moment is with, like, when you're generating text a lot of the... The buffers aren't reused properly during when you, when you start decoding. That's sort of leading to quite a massive performance bottleneck just because you're transferring memory between CPU and GPU every single time you're you're decoding. [01:22:31] So that's, that's not quite there yet, however, with things like image image classification and I guess models with encode only, encoder only models, those are getting quite good, like births pretty fast we've, segment anything when you're just doing the encoding step, we the Onyx Runtime team has got to the point where it used to take around 40 seconds and now it takes around 4 seconds. [01:22:55] And that's currently being worked on in like a dev branch, basically, of Transformers. js, just like making sure the integration's working. But it's, it's almost there. I keep, I keep saying it's almost there, but the amazing Microsoft team has been, has been really working hard on this. And if you just look at the commit history of on GitHub Microsoft slash Onyx Runtime and you go to the web version. [01:23:18] There's just so many amazing people working on it and it's slowly getting to a point where and this will sort of be released with Transformers. js version 3. When we upgrade the Onyx runtime version to probably 1. 17, which will be, which will be the next one. It's currently 1. 16. 1. And then they'll, it's, and, and literally from the user's perspective, it's as simple as adding a line of code just saying, Basically use web GPU instead of web assembly. [01:23:46] And, and also in the case where it's not supported, it'll fall back to the web assembly implementation. And, and that's, this will completely be transferable to how grid your light works, just because as was mentioned, it's sort of use as transformers js under the hood. So you any benefits that you'll see in Transformers j you'll see in transformers Jss pie, which you'll see in radio lights, which is, which is great. [01:24:11] TLDR coming soon, it's an, it's an annoying answer to give, but it's, it's so close. And I guess this is also good because it sort of aligns with the time that more browsers will support WebGPU, sort of like without flags. I know Chrome is sort of leading the charge and other Chromium based browsers. [01:24:30] But if you look at things like Safari and Firefox, it's quite far behind to the point that you it's. It's not, it's not ready for like mass adoption yet, but once it is, and once the ONNX Runtime backend has the WebGPU support has improved, you'll definitely be seeing that in Transformers Jest. [01:24:48] So hopefully that answers the question. I [01:24:52] Nisten Tahiraj: think stuff's about to get crazy on the front end because of this because the thing about you have all your WebGL stuff, you have all your maps, all your 3D, all your games. Now you can have an LLM even generate code for them, manipulate those objects, move stuff around on screen in 3D, and like the, the AI. [01:25:14] Does that at all, all within your machine, but I do want to say that for, for Pyodide itself, it might take a long time for a WebGPU support because it depends on EM scripting. And if you want to do anything with Python, like open a file, write a file, output a file, you only can do what EM scripting gives you and EM scripting is like the base layer. [01:25:39] Of the operating system, like it pretends, it fools your apps into thinking that there's an operating system there when, when there isn't. And as far as I've seen, like two, three months ago, WebGPU support was like really, really early on and might take a while for Emscripten to support that. So you're going to have to do that other ways by going straight to using WebGPU versus using it with that layer. [01:26:06] So it might get a bit complex [01:26:09] Alex Volkov: there. I agree about the stuff is about to get crazy. Go ahead, Arthur, and then we'll follow up on Gradio 4 and then we'll conclude. [01:26:18] Nisten Tahiraj: Yeah, I [01:26:18] Arthur Islamov: just wanted to tell that as yesterday or a few days ago I have seen that This distilled stable diffusion model I saw that they have previously released not Excel version, but the ordinary 2.1 or something like that, the distilled one. [01:26:35] So I'm thinking to try to make my edema work with that distilled model without 64 beats. So for just ordinary 32 bit that will work in almost any browser without any additional flags or launching with some special parameters. [01:26:54] Alex Volkov: Yeah. Arthur, you can't just mention an item on my updates list and not talk about this, right? [01:26:59] Folks, let me just briefly cover what Arthur just said. Just on the fly SegMind, a company called SegMind, introduced like a distilled version of SDXL. And it's okay, Diffusion Excel, something they released a while ago. We've covered this multiple times. Understand like way better. Quality, obviously, generations and diffusion, but also way better text understanding, right? [01:27:20] And it has two parts there's like a refiner part in addition. And so this company basically distilled that distillation we've talked about multiple times before. It's when you train your own model, but then you steal data from GPT 4 and you create the data so that GPT 4, you basically distill, it's like smartness to your own models. [01:27:37] So they basically did this for SDXL they call it SegMind Stable Diffusion 1B, and it's a. 50 percent smaller and 60 percent faster than SDXL. Again, just to put in some time frames what Abubakar and I talked about, where I first experienced Gladio, this was Stable Diffusion 1. 4, a year ago, a year and a couple of months ago. [01:27:57] Since then, we got Stable Diffusion multiple iterations of Stable Diffusion, then there is SDXL, which is like the Excel version. It it generates 124 by 124 images. And, and then now a few months after they released that, now we have a version that's 50 percent smaller and 60 percent faster. [01:28:16] And so what Arthur is like now talking about diffusers JS is the ability to like load stable diffusion in the browser. Now there's a model that's half the size, and 60 percent has passed, which is good for the browser context. So I pinned it to the top of the tweet check out SegMind, it's definitely super cool. [01:28:34] And the advancements that we see from week to week, and this is obviously super cool as well. And Arthur, sorry to interrupt with this, but you had one of my tasks that I had to finish before we finish and talk about this. So are you, have you already introduced it to diffusers? Have you tried it? [01:28:52] Nisten Tahiraj: I have [01:28:52] Arthur Islamov: tried it to convert it to omx, but it didn't work or maybe some of my code didn't work. So I guess I will try again on the weekend and yeah, most likely I will make it running. [01:29:06] Alex Volkov: I think we had some folks on segment react and we will, let's try to connect there and, and hopefully get it running on, on as well so that we all benefit and I guess, maybe as the last part of this conversation Abukar and thank you for joining Uchi ABA Ali and the folks from Hugging Face. It is great to see all of you. I think you mentioned some folks that joined before like Uhb and some other folks on the Hugging Face. We're big fans here on ThursdAI, and we're like, always welcome you guys. [01:29:33] Could you talk about what's coming in version four, because I think you, you, you gave us like one tidbit. But give us an update on that. I would love to hear. [01:29:40] Abubakar Abid: Yeah, yeah, definitely. Yeah, so we're launching Gradio 4. 0 on Tuesday October 31st. And basically, the, the team has been working very, you mentioned earlier that, people are, are building these very, very complex apps with, with Gradio and, and really, honestly, stuff that we did not anticipate when we were designing Gradio. [01:29:57] And more and more, what we want to do is almost take ourselves out of this feed, feedback loop. And let people build what they want to build, but let the community build stuff that, whatever you imagine, kind of just be, just be able to put that in a Gradio app. Let me be a little bit more concrete. [01:30:11] So what is Gradio 4. 0 going to introduce? For example it's going to introduce the idea of custom components. So if you know a little bit of Python, a little bit of JavaScript, you can build your own component. You can use that within a Gradio app, just like you do normally, just like you use our built in, 30 or so built in components. [01:30:27] Speaking of the built in components, we're redesigning some of the components from scratch, particularly the media components. So things like image audio video, they're going to be much, much nicer and they're going to be fully accessible. So one of the things that we're realizing is that, we're not, at Gradio, we're not just building a product for a specific audience, but we're building tools that let people build, apps for many different audiences. [01:30:50] And so we want to make sure that all of the core components are accessible. That way it's easy to do the right thing and build accessible web applications. So we're redesigning that we're switching over from WebSockets to as server side events. There's several reasons for this and, and we'll talk about more about this on, on Tuesday. [01:31:07] We're, we're having a little long live stream as well, but there's several reasons why server side events is the way to go for Gradio. And so there's, that's more of an internal refactor. You probably won't notice things. You might notice some speed ups in certain situations. It'll unlock a lot of things later on. [01:31:22] We're open sourcing the the the sharing links process, the share servers at Gradio. So everyone will be able to set up their own, custom share links. So instead of, whatever dot Gradio dot live, you can have, you can have, some, some code, dot turjom dot video if you want, you can have whatever URL, custom URL you want for your share links. [01:31:42] And then a lot of other changes as well we'll, we'll, we'll talk more about that on Tuesday. The team has been working super hard, so I'm, I'm excited to, to get it out for you guys to try out. [01:31:51] Alex Volkov: That's so awesome, and, and can't, can't wait to see this. I I think the share links is like such a powerful virality thing, that once people start adding this to their domains, and, and start running different Gradio interfaces within Colab, outside of Colab, with their own domains. [01:32:08] I think it's going to be super cool, especially if they don't expire. I absolutely received many of these links over DMs from multiple people. I think even people in the audience so far. And I think adding them to the custom domains. Thank you for open sourcing that. That's great. [01:32:21] Abubakar Abid: I think part of it is also we want to reduce the load on our shared servers. [01:32:25] We're getting too many of these links being created [01:32:27] and stuff. [01:32:27] Alex Volkov: Yes, absolutely. And I think the accessibility features are great. Folks, definitely check out follow Abu Bakr, follow Yuchi, and folks on stage, do Ali as well. To stay tuned to what's coming up to Gradio and then make sure to update your Gradio interfaces to the new accessible ones because you're building it's no longer demos. [01:32:46] Everybody's using every new model is, is getting a Gradio interface and accessibility is very important for the level of web applications. I think... With that, I want to thank you guys for coming up and sharing with us Radio Light, which is very, very in accordance to what we love to talk about here, open source, open source LLM, on device inference, and taking control of your own, LLMs. [01:33:07] I think, Nistan, you briefly, briefly talked about how crazy it's going to be. Where there is an LLM built into your website or web application that runs on the GPU of your device and is able to do stuff. And you can interact with it without basically offline. That's great. I think Nishtan, something that I want to talk about, but maybe I'll let you talk about this. [01:33:27] I will say this thing. Now that we've concluded the interview with Gladio folks, one of the things that we love. Most of all, on Thursday, I have breaking news, and we actually have some breaking news. Nissen, go ahead, please, present the breaking news that you just sent. [01:33:39] Nisten Tahiraj: I pasted a Gradius space above. [01:33:43] If you click on it, that's what it is. And it's it's Kokui's, new release, new voice model. This is huge because they're allowing fine tuning on their voice model. And one criticism of the open source voice models has been that the dataset for training them has been of poor quality, like the, the microphone and, and stuff and the, the dataset that people use to, to train the models has been bad. [01:34:12] So this is. Pretty important in this regard, because it's one of the very few, there's the one that Zenova released, and the Kokui one, that are open source and usable when it comes to text to speech, and that are like somewhat, somewhat pleasant, and that run relatively fast. Otherwise, it's pretty hard to have text to speech. [01:34:37] Yeah, the, the part that you can fine tune, they, they open source the fine tuning code. Yeah, go there and [01:34:43] Alex Volkov: get that, yeah. Thank you, Nissan. The, the folks from Cochlea, when they released XTTS, which is the open source text to speech that kind of, we know 11 Labs, we know Play. ht, we know OpenAI has one that Spotify uses to translation, and OpenAI haven't released any. [01:34:59] We'll see next week if they're gonna give us an API for that. All of those require... A lot of money, just a lot of money 11 labs is basically rolling in cash because everybody wants to get their AIs to talk, right? And so we previously here, we talked about the listen part, we've talked about the streaming from Gladiator, but now you can have Whisper basically streaming. [01:35:18] The other part of that was, hey, well, once your LLM listens and thinks, which is the inference part, you also want it. to talk to you. And TTS Texas speech is the way to do that. And Kokui, we had a chat with Joshua when they released TTS, which was very exciting. And now live on stage, live on Thursday. [01:35:34] I, because this is why Thursday exists. Many people release stuff on Thursday. There is their own fine tuning with minutes of data. So you can create a voice, let's say maybe this is going to be disappointing for folks here on stage, but everybody here who spoke on stage more than a minute now is basically public for everybody else to take your voice and clone it with XTDS. [01:35:56] It was possible before, somebody just had to pay money for it, but now... And Ali's laughing because Ali didn't talk yet, but everybody's basically now is, is going to get voice cloned. It's very easy. We're going towards this future. If this future scares you, there's no escape from that. Even I think VAL E from Microsoft, when it was released, they talked about like maybe 30 seconds of voice is enough to clone. [01:36:18] But XTTS now gives us basically a framework and, and even the new language they said to, to add to Kokui, to the XTTS, and then you can use this. Within Transformers. Zinova, can we use Cochlear within Transformers. js or not yet? I think, I think we can. Not yet. Not yet. Okay, so soon, soon you'll be able to even do this all completely all within the browser. [01:36:41] Hopefully once integration with WebGPU lands. So here we have it folks. We had an incredible Thursday I today. We started with talking with Bo and the guys from the embeddings team that released like the, the most kind of up how should I say, most comparable to OpenAI embeddings in open source. [01:36:59] And that was great. Bo actually gave us like a masterclass in how embeddings work and Jina embedding models are available within, talked with Bakar and Yuchi and ANOVA and Arthur and all on stage the team behind Grado that if you haven't used Grado, you probably have used Grado. You just didn't know that it's Grado And, actually, this interface or slash library that started for demos only scaled all the way up to something like automatic, where like multiple people compute, contribute thousands of contributions including like NVIDIA and and I think IBM contribute now. It's like a full businesses run on this quote unquote component library. [01:37:37] And I I just want to invite you to join Thursday and next week as well because... Some of this was planned, but definitely not all of this. But also, this is the way to stay up to date. And next week we're going to see some more incredible things. I think some very interesting things are coming up. [01:37:52] I will have a personal announcement to make that's going to be very surprising to some folks here on stage. But definitely we'll keep ThursdAI going significantly, significantly more. And with that, I just want to thank you for joining us. It's been a pleasure to have these. It's been a pleasure to like have a space where, the graduate folks and the Jina folks can come and talk about what they released. [01:38:12] And we can actually ask them questions. I want to thank everybody who joined on stage. Nistan, thank you always for joining, Zinova, Arthur. We, we were joined by new folks that we'll introduce next. Thank you so much for joining us this week because we just don't have the time and obviously thank you for folks in the audience who join every week and I see Enrico in there and Junaid and Tony and some other folks that like I love to see from week to week. [01:38:33] If you missed any part of this, any part at all, or if the internet connection for you got stuck ThursdAI is about a live recording, but then it's getting released as a podcast episode, so you will get, if you're subscribed, and you should be already subscribed to ThursdAI on Apple or Spotify, you'll get this episode hopefully very quickly edited, if I'm not getting lost in some other interesting stuff, like Kokui. [01:38:57] Thank you. And we will also release a newsletter with all the links and the conversations with Guardia team and, and Bo and all the updates as well in the form of links. And with that, I thank you for joining. It's been two hours. It's been a lovely time and now I need to go and actually edit the podcast. [01:39:12] See you here next week. Thank you and yeah, please share with your friends as much as possible. The more crowd there is, the better these will be. And yeah, help and participate. Thank you all and have a good rest of your week. Bye bye. This is a public episode. If you'd like to discuss this with other subscribers or get access to bonus episodes, visit sub.thursdai.news/subscribe | |||
03 Nov 2023 | 📅 ThursdAI Nov 02 - ChatGPT "All Tools", Bidens AI EO, many OSS SOTA models, text 2 3D, distil-whisper and more AI news 🔥 | 01:36:01 | |
ThursdAI November 2nd Hey everyone, welcome to yet another exciting ThursdAI. This week we have a special announcement, the co-host of and I will be hosting a shared X space live from Open AI Dev Day! Monday next week (and then will likely follow up with interviews, analysis and potentially a shared episode!) Make sure you set a reminder on X (https://thursdai.news/next) , we’re going to open the live stream early, 8:30am on Monday, and we’ll live stream all throughout the keynote! It’ll be super fun! Back to our regular schedule, we covered a LOT of stuff today, and again, were lucky enough to have BREAKING NEWS and the authors of said breaking news (VB from HuggingFace and Emozilla from Yarn-Mistral-128K) to join us and talk a little bit in depth about their updates! [00:00:34] Recap of Previous Week's Topics [00:00:50] Discussion on AI Embeddings [00:01:49] Gradio Interface and its Applications [00:02:56] Gradio UI Hosting and its Advantages [00:04:50] Introduction of Baklava Model [00:05:11] Zenova's Input on Distilled Whisper [00:10:32] AI Regulation Week Discussion [00:24:14] ChatGPT new All Tools mode (aka MMIO) [00:35:45] Discussion on Multimodal Input and Output Models [00:36:55] BREAKING NEWS: Mistral YaRN 7B - 128K context window [00:37:02] Announcement of Mistral Yarn Release [00:46:47] Exploring the Limitations of Current AI Models [00:47:25] The Potential of Vicuna 16k and Memory Usage [00:49:43] The Impact of Apple's New Silicon on AI Models [00:51:23] Introduction to New Models from Nius Research [00:51:39] The Future of Long Context Inference [00:53:42] Exploring the Capabilities of Obsidian [00:54:29] The Future of Multimodality in AI [00:58:48] The Exciting Developments in CodeFusion [01:06:49] The Release of the Red Pajama V2 Dataset [01:12:07] The Introduction of Luma's Genie [01:16:37] Discussion on 3D Models and Stable Diffusion [01:17:08] Excitement about AI Art and Diffusion Models [01:17:48] Regulation of AI and OpenAI Developments [01:18:24] Guest Introduction: VB from Hug& Face [01:18:53] VB's Presentation on Distilled Whisper [01:21:54] Discussion on Distillation Concept [01:27:35] Insanely Fast Whisper Framework [01:32:32] Conclusion and Recap Show notes and links: * AI Regulation * Biden Executive Order on AI was signed (Full EO, Deep dive) * UK AI regulation forum (King AI speech, no really, Arthur from Mistral) * Mozilla - Joint statement on AI and openness (Sign the letter) * Open Source LLMs * Together AI releases RedPajama 2, 25x larger dataset (30T tokens) (Blog, X, HF) * Alignment Lab - OpenChat-3.5 a chatGPT beating open source model (HF) * Emozilla + Nous Research - Yarn-Mistral-7b-128k (and 64K) longest context window (Announcement, HF) * LDJ + Nous Research release Capybara 3B & 7B (Announcement, HF) * LDJ - Obsidian 3B - the smallest open source multi modal model (HF, Quantized) * Big CO LLMs + APIs * ChatGPT "all tools" MMIO mode - Combines vision, browsing, ADA and DALL-E into 1 model (Thread, Examples, System prompt) * Microsoft CodeFusion paper - a tiny (75M parameters) model beats a 20B GPT-3.5-turbo (Thread, ArXiv) * Voice * Hugging Face - Distill whisper - 2x smaller english only version of Whisper (X, paper, code) * AI Art & Diffusion & 3D * Luma - text-to-3D Genie bot (Announcement, Try it) * Stable 3D & Sky changer AI Regulation IS HERE Look, to be very frank, I want to focus ThursdAI on all the news that we're getting from week to week, and to bring a positive outlook, so politics, doomerism, and regulation weren't on the roadmap, however, with weeks like these, it's really hard to ignore, so let's talk about this. President Biden signed an Executive Order, citing the old, wartime era Defence Production act (looks like the US gov. also has "one weird trick" to make the gov move faster) and it wasn't as bombastic as people thought. X being X, there has been so many takes pre this executive order even releasing about regulatory capture being done by the big AI labs, about how open source is no longer going to be possible, and if you visit Mark Andressen feed you'll see he's only reposting AI generated memes to the tune of "don't tread on me" about GPU and compute rights. However, at least on the face of it, this executive order was mild, and discussed many AI risks and focused on regulating models from huge compute runs (~28M H100 hours // $50M dollars worth). Here's the relevant section. Many in the open source community reacted to the flops limitation with a response that it's very much a lobbyist based decision, and that the application should be regulated, not only the compute. There's much more to say about the EO, if you want to dig deeper, I strongly recommend this piece from AI Snake oil : and check out Yan Lecun's whole feed. UK AI safety summit in Bletchley Park Look, did I ever expect to add the King of England into an AI weekly recap newsletter? Surely, if he was AI Art generated or something, not the real king, addressing the topic of AI safety! This video was played for the attendees of a few day AI safety summit in Blecheley park, where AI luminaries (Yan Lecun, Elon Musk, Arthur Mensch Mistral CEO, Naveen Rao) attended and talked about the risks and benefits of AI and regulation. I think Naveen Rao had a great recap here, but additionally, there were announcements about Safety Institute in the UK, and they outlined what actions the government can take. In other regulation related news, Mozilla has a joint statement on AI safety and openness (link) that many signed, which makes the case for openness and open source as the way to AI safety. Kudos on mozilla, we stand by the letter 🤝 Big CO LLMs + APIs OpenAI - ChatGPT "all tools" aka MMIO mode (that's now dubbed "confidential") Just a week before the first Dev Day from OpenAI, we were hanging out in X spaces talking about what the regulation might bring, when a few folks noticed that their ChatGPT interface looks different, and saw a very specific popup message saying that ChatGPT can now talk with documents, and "use tools without switching", see and interact with DALL-E and Advanced Data Analysis (FKA Code Interpreter) all in one prompt. While many X takes focused solely on just how many "chat with your PDF" startups OpenAI just "killed", and indeed, the "work with PDFs" functionality seemed new, chatGPT could now get uploads of files, had the ability to search, to go to a specific page, even do a basic summary on PDF files, I was interested in the second part! Specifically because given GPT-4V is now basically enabled for everyone, this "combined" mode makes ChatGPT the first MMIO model that we have, which is a multi modal on input (Text, Voice, Images) and output (Text, Images). You see, most MultiModal Models so far have been only multimodal on the input, ie, take in text or images or a combination, and while playing around with the above, we noticed some incredible use-cases that are now available! ChatGPT (for some lucky folks) can now do all these things in one prompt with shared context: * Read and interact with PDFs * See and understand images + text * Browse & Search up to date info with Bing * Write and execute code with ADA * Generate images with DALL-E All in the same prompt, one after another, and often for several steps and iterations. One such example was, I asked for "get the current weather in Denver and generate an image based on the conditions" and we got this incredible, almost on the fly "weather" UI, showing the conditions (it was the first snow in CO this year), weather, humidity and everything. Now, DALL-E is ok with text but not great, but it's incredible with scenery, so having this "on the fly UI" that has real time info was super great to show off the capabilities of a general model. We also saw prompts from folks who uploaded a picture of an obscure object, and asked DALL-E to "add" this object to an already generated image, so DALL-E now has eyes, and can understand and "draw" some of the objects and add them to other images, which was an amazing thing to see, and I can't wait to play around with this functionality. We noticed a few more things, specifically that DALL-E images are now stored on the same disk that you get access to with ADA, so you can then ask ChatGPT to upscale, crop and do things with those images for example, and generate code with those images as a background! There are so many new potential use-cases that have opened up, that we spent a long evening / night on X spaces trying to throw the kitchen sink onto this mode, in the fear that it was a fluke by OpenAI and they weren't meant to release this, and we were right! Today on ThursdAI live recording, some users reported that they no longer have access to it (and they miss it!) and some reported that it's now called something like "Confidential" Someone also leaked the full prompt for this "all tools" mode and it's a doosy! The "All Tools" omni-prompt takes a whopping 2,756 tokens, but it's also using the GPT-4 32k model, with a 32,767 token context window. (link) I guess we're going to see the announcement on Dev Day (did you set a reminder?) This new mode that we saw and played with, added to the many many leaks and semi-confirmed modes that are coming out of Reddit make it seem like ChatGPT is going to have an all out Birthday party next week and is about to blow some people's minds! We're here for it! 👏 Open Source LLMs CodeFusion - 75M parameters model based on Diffusion Model for Code Generation Code-fusion was taken down from ArXiv, claimed 3.5 is 20B params (and then taken down saying that this was unsubstantiated) X link The paper itself discusses the ability to use diffusion to generate code, and has much less data to get to a very good coding level, with a model small enough to fit on a chip's cache (not even memory) and be very very fast. Of course, this is only theoretical and we're going to wait for a while until we see if this replicates, especially since the PDF was taken down due to someone attributing the 20B parameters note to a forbes article. The size of the model, and the performance score on some coding tasks make me very very excited about tiny models on edge/local future! I find the parameter obsession folks have about OpenAI models incredible, because parameter size really doesn’t matter, it's a bad estimation anyway, OAI can train their models for years and keep them in the same parameter size and they would be vastly different models at the start and finish! Together releases a massive 30T tokens dataset - RedPajama-Data-v2 (Announcement, HF) This massive massive dataset is 25x the previous RedPajama, and is completely open, deduplicated and has enormous wealth of data to train models from. For folks who were talking the "there's no more tokens" book, this came as a surprise for sure! It's also multi-lingual, with tokens in English, French, Italian, German and Spanish in there. Kudos to Together compute for this massive massive open source effort 👏 Open source Finetunes Roundup This week was another crazy one for open source fine-tuners, releasing SOTA after SOTA, many of them on ThursdAI itself 😅 Barely possible to keep up (and that's quite literally my job!) Mistral 7B - 128K (and 64K) (HF) The same folks who brought you the YaRN paper, Emozilla, Bowen Peng and Enrico Shippole (frequent friends of the pod, we had quite a few conversations with them in the past) have released the longest context Mistral fine-tune, able to take 64K and a whopping 128K tokens in it's context length, making one of the best open source model now compatible with book length prompts and very very long memory! Capybara + Obsidian (HF, Quantized) Friend of the pod (and weekly cohost) LDJ releases 2 Nous research models, Capybara (trained on StableLM 3B and Mistral 7B) and Obsidian, the first vision enabled multi modal 3B model that can run on an iPhone! Capybara is a great dataset that he compiled and the Obsidian model uses the LLaVa architecture for input multimodality and even shows some understanding of humor in images! Alignment Lab - OpenChat-3.5 a chatGPT beating open source model (Announcement, HF) According to friends of the pod Alignment Lab (of OpenOrca fame) we get a Mistral finetune that beats! chatGPT on many code based evaluations (from march, we all think chatGPT became much better since then) OpenChat is by nature a conversationally focused model optimized to provide a very high quality user experience in addition to performing extremely powerfully on reasoning benchmarks. Open source is truly unmatched, and in the face of a government regulation week, open sources is coming out in full! Voice HuggingFace Distill Whisper - 6x performance of whisper with 1% WER rate (Announcement, HF) Hugging face folks release a distillation of Whisper, a process (and a paper) with which they use a "teacher" model like the original Open AI whisper, to "teach" a smaller model, and in the process of distillation, transfer capabilities from one to another, while also making the models smaller! This makes a significantly smaller model (2x smaller) with comparative (and even better) performance on some use-cases, while being 6x faster! This distill-whisper is now included with latest transformers (and transformers.js) releases and you can start using this faster whisper today! 👏 That's it for today folks, it's been a busy busy week, and many more things were announced, make sure to join our space and if you have read all the way until here, DM me the 🧯 emoji as a reply or in a DM, it’s how I know who are the most engaged users are! ThursdAI - Recaps of the most high signal AI weekly spaces is a reader-supported publication. To receive new posts and support my work, consider becoming a free or paid subscriber. This is a public episode. If you'd like to discuss this with other subscribers or get access to bonus episodes, visit sub.thursdai.news/subscribe | |||
09 Nov 2023 | 📅 ThursdAI - OpenAI DevDay recap (also X.ai grōk, 01.ai 200K SOTA model, Humane AI pin) and a personal update from Alex 🎊 | 01:56:20 | |
Hey everyone, this is Alex Volkov 👋 This week was an incredibly packed with news, started strong on Sunday with x.ai GrŌk announcement, Monday with all the releases during OpenAI Dev Day, then topped of with Github Universe Copilot announcements, and to top it all of, we postponed the live recording to see what hu.ma.ne has in store for us as AI devices go (Finally announced Pin with all the features) In between we had a new AI Unicorn from HongKong called Yi from 01.ai which dropped a new SOTA 34B model with a whopping 200K context window and a commercial license by ex-Google China lead Kai Fu Lee. Above all, this week was a monumental for me personally, ThursdAI has been a passion project for the longest time (240 days), and it led me to incredible places, like being invited to ai.engineer summit to do media, then getting invited to OpenAI Dev Day (to also do podcasting from there), interview and befriend folks from HuggingFace, Github, Adobe, Google, OpenAI and of course open source friends like Nous Research, Alignment Labs, and interview authors of papers, hackers of projects, and fine-tuners and of course all of you, who tune in from week to week 🙏 Thank you! It's all been so humbling and fun, which makes me ever more excited to share the next chapter. Starting Monday I'm joining Weights & Biases as an AI Evangelist! 🎊 I couldn't be more excited to continue ThursdAI mission, of spreading knowledge about AI, connecting between the AI engineers and the fine-tuners, the Data Scientists and the GEN AI folks, the super advanced cutting edge stuff, and the folks who fear AI with the backing of such an incredible and important company in the AI space. ThursdAI will continue as a X space, newsletter and podcast, as we'll gradually find a common voice, and continue bringing folks awareness of WandB incredible brand to newer developers, products and communities. Expect more on this very soon! Ok now to the actual AI news 😅 TL;DR of all topics covered: * OpenAI Dev Day * GPT-4 Turbo with 128K context, 3x cheaper than GPT-4 * Assistant API - OpenAI's new Agent API, with retrieval memory, code interpreter, function calling, JSON mode * GPTs - Shareable, configurable GPT agents with memory, code interpreter, DALL-E, Browsing, custom instructions and actions * Privacy Shield - Open AI lawyers will protect you from copyright lawsuits * Dev Day emergency pod with Latent Space with Swyx, Allesio, Simon and Me! (Listen) * OpenSource LLMs * 01 launches YI-34B, a 200K context window model commercially licensed and it tops all HuggingFace leaderboards across all sizes (Announcement) * Vision * GPT-4 Vision API finally announced, rejoice, it's as incredible as we've imagined it to be * Voice * Open AI TTS models with 6 very-realistic, multilingual voices, no cloning tho * AI Art & Diffusion * <2.5 seconds full SDXL inference with FAL (Announcement) OpenAI Dev Day So much to cover from OpenAI that this has it's own section today in the newsletter. I was lucky enough to get invited, and attend the first ever OpenAI developer conference (AKA Dev Day) and it was an absolute blast to attend. It was also incredible to attend it together with all 8.5 thousand of you who tuned into our live stream on X, as we were walking to the event, and then watched the keynote together (Thanks Ray for the restream) and talked with OpenAI folks about the updates. Huge shoutout to LDJ, Nisten, Ray, Phlo, Swyx and many other folks who held the space, while we were otherwise engaged with deep dives and meeting folks and doing interviews! So now for some actual reporting! What did we get from OpenAI? omg we got so much, as developers, as users (and as attendees, I will add more on this later) GPT4-Turbo with 128K context length The major thing that was announced is a new model, GPT-4-turbo, which is supposedly faster than GPT-4, while being 3x cheaper (2x on output) and having a whopping 128K context length while also being more accurate (with significantly better recall and attention throughout this context length) With JSON mode and significantly improved function calling capabilities, updated cut-off time (April 2023), and higher rate limits, this new model is already being implemented across all the products and is a significant significant upgrade to many folks GPTs - A massive shift in agent landscapes by OpenAI Another (semi-separate) thing that Sam talked about was the GPTs, their version of agents not to be confused with the Assistants API, which is also Agents, but for developers, and they are not the same and it's confusing GPTs I think is a genius marketing move by OpenAI and replaces Plugins (that didn't even meet product market fit) in many regards. GPTs are instances of well... GPT4-turbo, that you can create by simply chatting with BuilderGPT, and they can have their own custom instruction set, and capabilities that you can turn on and off, like browse the web with Bing, Create images with DALL-E and write and execute code with Code Interpreter (bye bye Advanced Data Analysis, we don't miss ya). GPTs also have memory, you can upload a bunch of documents (and your users as well) and GPTs will do vectorization and extract the relevant information out of those documents, so think, your personal Tax assistant that has all 3 years of your tax returns And they have eyes, GPT4-V is built in so you can drop in screenshots, images and all kinds of combinations of things. Additionally, you can define actions for assistants (which is similar to how Plugins were developed previously, via an OpenAPI schema) and the GPT will be able to use those actions to do tasks outside of the GPT context, like send emails, check stuff in your documentation and much more, pretty much anything that's possible via API is now possible via the actions. One big thing that's missing for me is, GPTs are reactive, so they won't reach out to you or your user when there's a new thing, like a new email to summarize or a new task completed, but I'm sure OpenAI will close that gap at some point. GPTs are not Assistants, they are similar but not the same and it's quite confusing. GPTs are created online, and then are share-able with links. Which btw, I created a GPT that uses several of the available tools, browsing for real time weather info, and date/time and generates an on the fly, never seen before weather art for everyone. It's really fun to play with, let me know what you think (HERE) the image above is generated by the Visual Weather GPT Unified "All tools" mode for everyone (who pays) One tiny thing that Sam mentioned on stage, is in fact huge IMO, is the removal of the selector in chatGPT, and all premium users now have access to 1 interface that is multi modal on input and output (I call it MMIO) - This mode now understands images (vision) + text on input, and can browse the web and generate images, text, graphs (as it runs code) on the output. This is a significant capabilities upgrade to many folks who will use these tools, but previously didn't want to choose between DALL-E mode and browse or Code Interpreter mode. The model now intelligently selects what tool to use for a given task, and this means more and more "generality" for the models, as they are learning and getting new capabilities in the form of tools. This in addition to a MASSIVE 128K context window, means that chatGPT has now been significantly upgraded, and you still pay $20/mo 👏 Gotta love that! Assistant API (OpenAI Agents) This is the big announcement for developers, we all got access to a new and significantly improved Assistants API, which improves on several our experience on several categories: * Creating Assistants - Assistants are OpenAI's first foray into the world of AGENTS, and it's quite exciting! You can create an assistant via API (not quite the same as GPTs, we'll cover the differences later), you can create each assistant with it's own set of instructions (that you don't have to pass each time with the prompt), tools like code interpreter and retrieval, and functions. Also you can select models, so you don't have to use the new GPT-4-turbo (but you should!) * Code Interpreter - Assistants are able to write and execute code now, which is a whole world of excitement! Having code abilities (that executes in a safe environment on OAI side) is a significant boost in many regards and many tasks require bits of code "on the fly", for example time-zone tasks. You will no longer have to write that code yourself, you can ask your assistant * Retrieval - OpenAI (and apparently QDrant!) have given all the developers a built in RAG (retrieval augmented generation) capabilities + document uploading and understanding. You can upload files like documentation via the API or let your users upload files, and parse and extract information out of! This is an additional huge huge thing, basically memory is built in for you now * Stateful API - this API introduces the concept of threads, where OpenAI will manage the state of your conversation, and you can assign 1 user per thread and then just send the responses back to the user, and send the user queries to the same thread. No longer do you have to send the whole history back and forth! It's quite incredible, however it raises the question of pricing, and calculating tokens. Per OpenAI (I asked), if you would like to calculate costs on the fly, you'd have to use the get thread endpoint, and then count the amount of tokens that's already in the thread (and it can be a LOT since there's now 128K tokens in the context length) * JSON and Better functions calling - You can now set the API to respond in JSON mode! Which is an incredible improvement for devs, and which we only were able to do via Functions before, and even functions got an upgrade, with an ability to call multiple functions. Functions are added as "actions" in the assistant creation, so you can give your assistant abilities that it will execute by returing to you functions with the right parameters. Thing "set the mood" will return a function to call the smart lights, and "play" will return a function that will call Spotify API * Multiple Assistants can join a thread - you can create specific assistants that can all join the same thread with the user, each with a set of custom instructions and capabilities and tools * Parallel Functions - this is also new, the assistant API can now return several functions for you to execute, which could lead to the creation of scenes, for example in a smart home, you want to "set the mood" and several functions would be returned from the API, one that will turn of the lights, one that will start the music, and one that will turn on mood lighting. Vision GPT-4 Vision Finally, it's here, multimodality for developers to implement, the moment I personally have been waiting for since GPT-4 was launched (and ThursdAI started) back on March 14 (240 days ago, but who's counting) GPT-4 vision is able to take images, and text, and respond with many vision related tasks, like analysis, understanding, summarization of captions. Many folks are splitting videos frame by frame and analyzing whole videos already (in addition to whispering the video to get what is said) Hackers and developers like friend of the pod Robert, created quick hacks like a browser extension that lets you select any screenshot on the page and ask GPT4 vision things about it, another friend of the pod SkalskiP created a hot dog classifier Gradio space 😂 and is maintaining an awesome list of experiments with vision on Github Voice Text to speech models OpenAI decided to help us all build agents properly, and agents need not only ears (for which they gave us whisper, and released V3 as well) but also voice, and we finally got the TTS from OpenAI, 6 very beautiful, emotional voices, that you can run very easily, and cheaply. You can't generate more or clone yet (that's only for friends of OpenAI like Spotify and others) but you can use the 6 we got (plus a secret pirate one apparently they trained but never released!) They sound ultra-realistic, and are multi-linugal as well, you can just pass different languages and voila. Friend of the pod Simon Willison created a quick CLI tool called ospeak to pipe text into and it'll use your OAI key to read that text out with those super nice voices! Whisper v3 was released! https://github.com/openai/whisper/discussions/1762 The large-v3 model shows improved performance over a wide variety of languages, and the plot below includes all languages where Whisper large-v3 performs lower than 60% error rate on Common Voice 15 and Fleurs, showing 10% to 20% reduction of errors compared to large-v2: HUMANE Humane AI pin is ready for pre-order at 699 HUMANE pin was finally announced, and here is the break-down, they have a clever way to achieve "all day battery life" by having a hot swap system, with a magnetic booster that you can swap when you get low on battery (pretty genius TBH) It's passive so it's not "always listening" but there is a wake word apparently, and you can activate by touch. Runs on the T-mobile Network ( which sucks for folks like me where T-mobile just doesn't have reception in their neighborhood 😂 ) No apps, just AI experiences powered by OpenAI, with a laser powered projector UI on your hand, and voice controls AI voice input will allow interactions like asking for information (which has browsing) and is SIGNIFICANTLY better than "Siri" or "Ok Google" from the demo, being able to rewrite your messages for you, catch you up on multiple messages and even search through them! You can ask for retrieval from previous messages Pin is multimodal, voice input and vision Holding the microphone on Tab while someone's speaking to you in a different language will automatically translate that language for you and then translate you back to that language with your own intonation! Bye bye language barriers! And with vision, you can do things like tracking calories from showing it what you ate, or buy things you're seeing in the store, but online, take pictures and videos and then store all of them transcribed in your personal AI memory Starting at $699, with a $24/mo payment that comes with unlimited AI queries, storage and service (again, just T-mobile), Tidal music subscription and more. I think it's lovely that someone tries to take on Google/Apple duopoly with a completely re-imagined AI device, and can't wait to pre-order mine and test it out. It will be an interesting experience of balance with 2 phone numbers, but also a monthly payment that basically makes the device use-less if you stop paying. Phew, this was a big update, not to mention there's a whole 2 hour podcast I want you to listen to on top of this, thank you for reading, for subscribing, for participating in the community and I can't wait to finally relax after this long week (still Jet-lagged) and prepare for my new Monday! I want to send a heartfelt shoutout to my friend swyx who not only let me on to Latent Space from time to time (including the last recap emergency pod), but also is my blood-line to SF, where everything happens! Thanks man, I really appreciate all you did for me and ThursdAI 🫡 Can't wait to see you all on the next ThursdAI, and as always, replies, comments, congratulations, are welcome as replies, DMs and send me the 🎉 for this one, I'd really appreciate it! — Alex This is a public episode. If you'd like to discuss this with other subscribers or get access to bonus episodes, visit sub.thursdai.news/subscribe | |||
17 Nov 2023 | 📅 ThursdAI Nov 16 - Live AI art, MS copilots everywhere, EMUs from Meta, sketch-to-code from TLDraw, Capybara 34B and other AI news! | 01:46:42 | |
Hey yall, welcome to this special edition of ThursdAI. This is the first one that I'm sending in my new capacity as the AI Evangelist Weights & Biases (on the growth team) I made the announcement last week, but this week is my first official week at W&B, and oh boy... how humbled and excited I was to receive all the inspiring and supporting feedback from the community, friends, colleagues and family 🙇♂️ I promise to continue my mission of delivering AI news, positivity and excitement, and to be that one place where we stay up to date so you don't have to. This week we also had one of our biggest live recordings yet, with 900 folks tuned in so far 😮 and it was my pleasure to again to chat with folks who "made the news" so we had a brief interview with Steve Ruiz and Lou from TLDraw, about their incredible GPT-4 Vision enabled "make real" functionality and finally got to catch up with my good friend Idan Gazit who's heading the Github@Next team (the birthplace of Github Copilot) about how they see the future. So definitely definitely check out the full conversation! TL;DR of all topics covered: * Open Source LLMs * Nous Capybara 34B on top of Yi-34B (with 200K context length!) (Eval, HF) * Microsoft - Phi 2 will be open sourced (barely) (Announcement, Model) * HF adds finetune chain genealogy (Announcement) * Big CO LLMs + APIs * Microsoft - Everything is CoPilot (Summary, copilot.microsoft.com) * CoPilot for work and 365 (Blogpost) * CoPilot studio - low code "tools" builder for CoPilot + GPTs access (Thread) * OpenAI Assistants API cookbook (Link) * Vision * 🔥 TLdraw make real button - turn sketches into code in seconds with vision (Video, makereal.tldraw.com) * Humane Pin - Orders are out, shipping early 2024, multimodal AI agent on your lapel ( * ) * Voice & Audio * 🔥 DeepMind (Youtube) - Lyria high quality music generations you can HUM into (Announcement) * EmotiVoice - 2000 different voices with emotional synthesis (Github) * Whisper V3 is top of the charts again (Announcement, Leaderboard, Github) * AI Art & Diffusion * 🔥 Real-time LCM (latent consistency model) AI art is blowing up (Krea, Fal Demo) * 🔥 Meta announces EMU-video and EMU-edit (Thread, Blog) * Runway motion brush (Announcement) * Agents * Alex's Visual Weather GPT (Announcement, Demo) * AutoGen, Microsoft agents framework is now supporting assistants API (Announcement) * Tools * Gobble Bot - scrape everything into 1 long file for GPT consumption (Announcement, Link) * ReTool state of AI 2023 - https://retool.com/reports/state-of-ai-2023 * Notion Q&A AI - search through a company Notion and QA things (announcement) * GPTs shortlinks + analytics from Steven Tey ( https://chatg.pt * ) This Week's Buzz from WandB (aka what I learned this week) Introducing a new section in the newsletter called "The Week's Buzz from WandB" (AKA What I Learned This Week). As someone who joined Weights and Biases without prior knowledge of the product, I'll be learning a lot. I'll also share my knowledge here, so you can learn alongside me. Here's what I learned this week: The most important things I learned this week is just how prevelant and how much of a leader Weights&Biases is. W&B main product is used by most of the foundation LLM trainers including OpenAI. In fact GPT-4 was completely trained on W&B! It's used by pretty much everyone besides Google. In addition to that it's not only about LLMs, W&B products are used to train models in many many different areas of the industry. Some incredible examples are a pesticide dispenser that's part of the John Deere tractors that only spreads pesticides onto weeds and not actual produce. And Big Pharma who's using W&B to help create better drugs that are now in trial. And it's just incredible how much machine learning that's outside of just LLMs is there. But also I'm absolutely floored by just the amount of ubiquity that W&B has in the LLM World. W&B has two main products, Models & Prompts, Prompts is a newer one, and we're going to dig into both of these more next week! Additionally, it's striking how many AI Engineers, API users such as myself and many of my friends, have no idea of who W&B even is, of if they do, they never used it! Well, that's what I'm here to change, so stay tuned! Open source & LLMs In the open source corner, we have the first Nous fine-tune of Yi-34B, which is a great model that we've covered in the last episode and now is fine-tuned with the Capybara dataset by ThursdAI cohost, LDJ! Not only is that a great model, it now tops the charts for the resident reviewer we WolframRavenwolf on /r/LocalLLama (and X) Additionally, Open-Hermes 2.5 7B from Teknium is now second place on HuggingFace leaderboards, it was released recently but we haven't covered until now, I still think that Hermes is one of the more capable local models you can get! Also in open source this week, guess who loves it? Satya (and Microsoft) They love it so much that they not only created this awesome slide (altho, what's SLMs? Small Language Models? I don't like it), they also announced that LLaMa and Mistral are coming to Azure services as inference! And they gave us a little treat, Phi2 is coming. They said OpenSource (but folks looking at the license saw that it's only for research capabilities) but supposedly it's a significantly more capable model while only being 2.7B weights (super super tiny) Big Companies & APIs Speaking of Microsoft, they announced so much during their Ignite event on Wednesday (15th) that it's impossible to cover all of it in this newsletter, but basically here are the main things that got me excited! CoPilot everywhere, everything is CoPilot Microsoft rebranded Bing Chat to Copilot and it now lives on copilot.microsoft.com and it's basically a free GPT4, with vision and DALL-e capabilities. If you're not signed up for OpenAI's plus membership, this is as good as it gets for free! They also announced CoPilot for 365, which means that everything from office (word, excel!) to your mail, and your teams conversations will have a CoPilot that will be able to draw from your organizations knowledge and help you do incredible things. Things like help book appointments, pull in relevant people for the meeting based on previous documents, summarize that meeting, schedule follow ups, and like a TON more stuff. Dall-e integration will help you create awesome powerpoint slides. (p.s. all of this will be allegedly data protected and won't be shared with MS or be trained on) They literally went and did "AI everywhere" with CoPilot and it's kinda incredible to see how big they are betting the farm on AI with Microsoft while Google... where's Google™? CoPilot Studio One of the more exciting things for me was, the CoPilot Studio announcement, a low-code tool to extend your company's CoPilot by your IT, for your organization. Think, getting HR data from your HR system, or your sales data from your SalesForce! They will launch with 1100 connectors for many services but allow you to easily build your own. One notable thing is, Custom GPTs will also be a connector! You will be literally able to connect yyour CoPilot with your (or someone's) GPTs! Are you getting this? AI Employees are coming faster than you think! Vision I've been waiting for cool vision demos since GPT-4V API was launched and oh boy did we get them! From friend of the pod Robert Lukoshko Auto screenshot analysis which will take screenshots periodically and will send you a report of all you did that day, to Charlie Holtz live webcam narration by David Attenborough (which is available on Github!) But I think there's 1 vision demo that takes the cake this week, by our friends (Steve Ruiz) from TLDraw, which is a whiteboard canvas primitive. They have added a sketch-to-code button, that allows you to sketch something out and GPT-4 Vision will analyze this, and GPT-4 will write code, and you will get live code within seconds. It's so mind-blowing that I'm still collecting my jaw of the floor here. It also does coding, so if you ask it nicely to add JS interactivity, the result will be interactive 🤯 GPT4-V Is truly as revolutionary as I imagined it to be when Greg announced it on stage 🫡 P.S - Have you played with it? Do you have cool demos? DM me with 👁️🗨️ emoji and a cool vision demo to be included in the next ThursdAI AI Art & Diffusion & 3D In addition to the TLDraw demo, one mind-blowing demo after another is coming this week from the AI Art world, using the LCM (Latent Consistency Model) + a whiteboard. This is yet another see it to believe it type thing (or play with it) (video from Linus) Dear friends from Krea.ai were the first to implement this insanity, that allows you to see real time AI art generation almost as fast as you type your prompts, and then followed up by the wizards at Fal to get the generations down to several mili-seconds (shoutout Gorkem!), the real time drawing thing is truly truly mind-blowing. It's so mind-blowing that folks add their webcam feeds into this, and see almost real time generation on the fly of their webcam feeds. Meta announcing new Emus (Video & Edit) Meta doesn't want me to relax, and during the space, announced their text-to-video and textual-editing models. Emu Video produces great videos from a prompt, and emu-edit is really interesting, it allows you to edit parts of images by typing, think "remove the tail from this cat" or "remove the hat from this person" They have this to say, which... dayum. In human evaluations, our generated videos are strongly preferred in quality compared to all prior work– 81% vs. Google’s Imagen Video, 90% vs. Nvidia’s PYOCO, and 96% vs. Meta’s Make-A-Video. Our model outperforms commercial solutions such as RunwayML’s Gen2 and Pika Labs It's really compelling, can't wait to see if they open source this, video is coming ya'll! Audio & Sound Deepmind + Youtube announced Lyria (blogpost) This new music model is pretty breathtaking, but we only got a glimpse, not even a waitlist for that one, however, check out the pre-recorded demoes, folks at deep mind have a model you can hum into, sing into, and it'll create a full blown track for you, with bass, drums, and singing! Not only that, it will also license vocals from mucisians (al-la Grimes) and will split the revenue between you and them if you post it on Youtube! Pretty cool Google, pretty cool! Agents & Tools Look, I gotta be honest, I'm not sure about this category, Agent and Tools, if to put them into one or not, but I guess GPTs are kinda tools, so I'm gonna combine them for this one. GPTs (My Visual Weather, Simons Notes) This week, the GPT that I created called Visual Weather GPT has blown up, with over 5,000 chats opened with it, and many many folks using this and texting me about this. super cool way to just like check all the capabilities of a GPT. If you remember, I thought of this idea a few weeks ago when we got a sneak preview to the "All tools" mode, but now I can share it with you all in the form of a GPT, that will browser the web for real time weather data, and create a unique art piece for that location and weather conditions! It's really easy to make as well, and I do fully expect everyone to start making their own versions very soon, and I think we're inching towards the era of JIT (just in time) software, where you'll create software as you require it, and it'll be as easy as talking to a chatGPT! Speaking of, friend of the pod Steven Tey from Vercel (who's dub.sh I use and love for thursdai.news links) has released a GPT link shortener, called chatg.pt and you can register and get your own cool short link like https://chatg.pt/artweather 👏 And it'll give you analytics as well! Pro tip for weather GPT, you can ask for a specific season or style in parentesises and then those as greeting cards for your friends. Happy upcoming Thanks giving everyone! Speaking of Thanks giving, we're not taking a break, next ThursdAI, November 23, join us for a live discussion and podcast recoding! We'll have many thanks, cool AI stuff, and much more! This is a public episode. If you'd like to discuss this with other subscribers or get access to bonus episodes, visit sub.thursdai.news/subscribe | |||
23 Nov 2023 | 🦃 ThursdAI Thanksgiving special - OpenAI ctrl+altman+delete, Stable Video, Claude 2.1 (200K), the (continuous) rise of OSS LLMs & more AI news | 01:56:09 | |
ThursdAI TL;DR - November 23 TL;DR of all topics covered: * OpenAI Drama * Sam... there and back again. * Open Source LLMs * Intel finetuned Mistral and is on top of leaderboards with neural-chat-7B (Thread, HF, Github) * And trained on new Habana hardware! * Yi-34B Chat - 4-bit and 8-bit chat finetune for Yi-34 (Card, Demo) * Microsoft released Orca 2 - it's underwhelming (Thread from Eric, HF, Blog) * System2Attention - Uses LLM reasons to figure out what to attend to (Thread, Paper) * Lookahead decoding to speed up LLM inference by 2x (Lmsys blog, Github) * Big CO LLMs + APIs * Anthropic Claude 2.1 - 200K context, 2x less hallucinations, tool use finetune (Announcement, Blog, Ctx length analysis) * InflectionAI releases Inflection 2 (Announcement, Blog) * Bard can summarize youtube videos now * Vision * Video-LLaVa - open source video understanding (Github, demo) * Voice * OpenAI added voice for free accounts (Announcement) * 11Labs released speech to speech including intonations (Announcement, Demo) * Whisper.cpp - with OpenAI like drop in replacement API server (Announcement) * AI Art & Diffusion * Stable Video Diffusion - Stability releases text2video and img2video (Announcement, Try it) * Zip-Lora - combine diffusion LORAs together - Nataniel Ruiz (Annoucement, Blog) * Some folks are getting NERFs out from SVD (Stable Video Diffusion) (link) * LCM everywhere - In Krea, In Tl;Draw, in Fal, on Hugging Face * Tools * Screenshot-to-html (Thread, Github) Ctrl+Altman+Delete weekend If you're subscribed to ThursdAI, then you most likely either know the full story of the crazy OpenAI weekend. Here's my super super quick summary (and if you want a full blow-by-blow coverage, Ben Tossel as a great one here) Sam got fired, Greg quit, Mira flipped then Ilya Flipped. Satya played some chess, there was an interim CEO for 54 hours, all employees sent hearts then signed a letter, neither of the 3 co-fouders are on the board anymore, Ilya's still there, company is aligned AF going into 24 and Satya is somehow a winner in all this. The biggest winner to me is open source folks, who got tons of interest suddenly, and specifically, everyone seems to converge on the OpenHermes 2.5 Mistral from Teknium (Nous Research) as the best model around! However, I want to shoutout the incredible cohesion that came out of the folks in OpenAI, I created a list of around 120 employees on X and all of them were basically aligned the whole weekend, from ❤️ sending to signing the letter, to showing how happy they are Sam and Greg are back! Yay This Week's Buzz from WandB (aka what I learned this week) As I’m still onboarding, the main things I’ve learned this week, is how transparent Weights & Biases is internally. During the whole OAI saga, Lukas the co-founder sent a long message in Slack, addressing the situation (after all, OpenAI is a big customer for W&B, GPT-4 was trained on W&B end to end) and answering questions about how this situation can affect us and the business. Additionally, another co-founder, Shawn Lewis shared a recording of his update to the BOD of WandB, about out progress on the product side. It’s really really refreshing to see this information voluntarily shared with the company 👏 The first core value of W&B is Honesty, and it includes transparency outside of matters like personal HR stuff, and after hearing about this during onboarding, it’s great to see that the company lives it in practice 👏 I also learned that almost every loss curve image that you see on X, is a W&B dashboard screenshot ✨ and while we do have a share functionality, it’s not built for viral X sharing haha so in the spirit of transparency, here’s a video I recorded and shared with product + feature request to make these screenshot way more attractive + clear that it’s W&B Open Source LLMs Intel passes Hermes on SOTA with a DPO Mistral Finetune (Thread, Hugging Face, Github) Yes, that intel, the... oldest computing company in the world, not only comes out strong with the best (on benchmarks) open source LLM, it also does DPO, and has been trained on a completely new hardware + Apache 2 license! Here's Yam's TL;DR for the DPO (Direct Policy Optimization) technique: Given a prompt and a pair of completions, train the model to prefer one over the other. This model was trained on prompts from SlimOrca's dataset where each has one GPT-4 completion and one LLaMA-13B completion. The model trained to prefer GPT-4 over LLaMA-13B. Additionally, even tho there is custom hardware included here, Intel supports the HuggingFace trainer fully, and the whole repo is very clean and easy to understand, replicate and build things on top of (like LORA) LMSys Lookahead decoding (Lmsys, Github) This method significantly improves the output of LLMs, sometimes by more than 2x, using some jacobian notation (don't ask me) tricks. It's copmatible with HF transformers library! I hope this comes to open source tools like LLaMa.cpp soon! Big CO LLMs + APIs Anthropic Claude comes back with 2.1 featuring 200K context window, tool use While folks on X thought this was new, Anthropic actually announced Claude with 200K back in the May, and just gave us 100K context window, which for the longest time was the largest context window around. I was always thinking, they don't have a reason to release 200K since none of their users actually wants it, and it's a marketing/sales decision to wait until OpenAI catches up. Remember, back then, GPT-4 was 8K and some lucky folks got 32K! Well, OpenAI releases GPT-4-turbo with 128K so Anthropic re-trained and released Claude to gain an upper hand. I also love the tool use capabilities. Re: longer context window, there were a bunch of folks testing if 200K context window is actually all that great, and it turns out, besides being very expensive to run (you pay per tokens) it also loses a bunch of information at lengths over 200K using needle in the haystack searches. Here's an analysis by Greg Kamradt that shows that: * Starting at ~90K tokens, performance of recall at the bottom of the document started to get increasingly worse * Less context = more accuracy - This is well know, but when possible reduce the amount of context you send to the models to increase its ability to recall. I had similar issues back in May with their 100K tokens window (source) Voice & Audio ElevenLabs has speech-to-speech Creating a significant jump in capabilities, ElevenLabs now allows you to be the actor behind the voice! With speech to speech, they would transfer the pauses, the intonation, the emotion, into the voice generation. Here's my live reaction and comparison: * Notable: Whisper.CPP now supports a server compatible with OpenAI (Announcement, Github) AI Art & Diffusion Stable Video diffusion - text-2-video / img-2-video foundational model (Announcement, Hugging Face, Github, DEMO) Stable has done it again, Stable Video allows you to create increidbly consistent videos with images or just text! They are short now, but working on extending the times, and they videos look incredible! (And thanks to friends at Fal, you can try right now, here) And here’s a quick gif I created with DALL-E 3 and Fal to celebrate the Laundry Buddy team at OAI while the outage was happening) Tools Screenshot to HTML (Github) I… what else is there to say? Someone used GPT4-Vision to … take screenshots and iteratively re-create the HTML for them. As someone who used to spend month on this exact task, I’m very very happy it’s now automated! Happy Thanksgiving 🦃 I am really thankful to all of you who subscribe and come back every week, thank you! I would have been here without all your support, comments, feedback! Including this incredible art piece that Andrew from spacesdashboard created just in time for our live recording, just look at those little robots! 😍 See you next week (and of course the emoji of the week is 🦃, DM or reply!) This is a public episode. If you'd like to discuss this with other subscribers or get access to bonus episodes, visit sub.thursdai.news/subscribe | |||
30 Nov 2023 | 🎉 ThursdAI Nov 30 // ChatGPT 1 year celebration special episode // covering the past 1 year in LLM/OSS AI 🥳 | 01:24:37 | |
🎶 Happy birthday to you, happy birthday to you, happy birthday chat GPT-eeeeeeee, happy birthday to you. Hey everyone, welcome to this special edition of ThursdAI where you're probably gonna have two emails and two podcast episodes today and you can choose which one you want to but we actually recorded both of them live it just they went a little long. ThursdAI - The only podcast that brings you yearly recaps since chatGPT was released (😂) This one is the more celebratory one, today is one year from the release of chat GPT and we (and by we I mean I, Alex) decided to celebrate it by recapping not just the last week in AI but the last year (full timeline posted at the bottom of this newsletter) Going month by month with a swoosh sound in the editing and covering the most important thing that happened in LLM and open source LLMs since chatGPT was released and imagination unlocked the capability for everyone! We also covered Meta stepping in with Lama and then everything that happened since then in the multi modality and vector databases and agents and everything everything everything, it was a one hell of an hour and a half, we had almost 1K audience members! and so I recommend you listen to this one first and then the week updates later because there were some incredible releases this week as well! (as there are every week) I think it's important to do like a Spotify wrapped type thing for AI, for something like a one year for chat GPT and I think we'll be doing this every year so hopefully in the year we'll see you here on November 30th covering the next year in AI. And hopefully the next year in AI system will actually help me summarize all this because it's a lot of work but with that I will just leave you with the timeline and no notes and you should listen to everything because we talked about everything live! I hope you enjoy this special birthday celebration! (OpenAI sure did, check out this incredibly cute little celebration video they just posted) Here’s the full timeline with everything important that happened month by month that we’ve covered: * December 2022 - ChatGPT becomes the fastest growing product in history * GPT3.5 with 4K context window, instruction finetuning and conversational RLHF * January * Microsoft invests additional $10B into OpenAI (Jan 23, Blog) * February * LLaMa 1 - Biggest Open Source LLM (February 24 - Blog) * No commercial license * 30% MMLU * No instruction fine-tuninig (RL;HF) * ChatGPT unofficial APIs exist * March (the month of LLM superpowers) * ChatGPT API (March 1, announcement) * Developers can now build chatGPT powered apps * All clones so far were completion based and not conversation based * LLama.cpp from ggerganov + Quantization (March 10, Blog) * Stanford - Alpaca 7B - Finetune on self-instruct GPT3.5 dataset (March 13, Blog) * GPT4 release + chatGPT upgrade (March 14 - GPT-4 demo) * 67.0% HumanEval | 86.4% MMLU * 8K (and 32K) context windows * Anthropic announces Claude + Claude instant (March 14 - Blog) * 56.0% HumanEval * Folks previously form OAI leave to open Anthropic as research, then pivot from research into commercial * LMSYS Vicuna 13B - Finetuned based on shareg.pt exports (March 30, Blog) * April (Embedings & Agents) * AutoGPT becomes the fastest github starred project + writes it's own code (April 1, Blog) * Agents start to pop up like mushrooms after the rain * LLaVa - Multimodality open source begins (April 18, Blog) * CLIP + Vicuna smushed together to get LLMs eyes * Bard improvements * May (Context windows) * Mosaic MPT-7B with 64K context, 1T parameters, commercial license (May 5, Blog) * Anthropic updates Claude with 100K context window (May 11, Blog) * LLongBoi summer begins (Context windows are being stretched) * Nvidia shows Voyager agents that play Minecraft + Memory stored in Vector DB (May 27, Blog) * June * GPT-3.5-turbo + functions API (June 6, Blog) * GPT3.5 and 4 got a boost in capabilities and steer-ability * Price reduction on models + 75% reduction on ada embeddings model * LLaMa context window extended to 8K with RoPE scaling * AI Engineers self determination essay by swyx * July * Code Interpreter GA - ChatGPT can code (July 11, Blog) * Anthropic Claude 2 - (July 11 - Blog) * 200K context window * 71% HumanEval * LLaMa 2 (July 18 - Blog) * Base & Chat models (RLHF) * Commercial license * 29.9% Human Eval | 68.9% MMLU * August * Meta releases Code-LlaMa, code finetune models * September * DALL-E 3 - Adds multi-modality on output and chat to image gen (Sep 20, Blog) * Mistral 7B top performing open source LLM via torrent link (Sep 27, Blog) * GPT4-V (vision & voice) - Adds multimodality on input (Sep 27, Blog) * October * OpenHermes - Mistral 7B finetune that tops the charts from Teknium / Nous Research (Oct 16, Announcement) * Inflection PI gets connected to the web + supportPi mode (Oct 16, Blog) * Adept releases multimodal FuYu 8B (Oct 19, blog) * November * Grok from Xai - with realtime access to all of X content * OpenAI dev day * Combined mode for MMIO (multi modal on input and output) * GPT-4 Turbo with 128K context, 3x cheaper than GPT-4 * Assistants API with retrieval capabilities * Share-able GPTs - custom versions of GPT with retrieval, DALL-E, Code Interpreter and vision * Chatbots with real business use-cases, for example WandBot (that we just launched today! Blog) * Has vector storage memory * Available via Discord/Slack * And custom GPT! * Microsoft has copilot everywhere in office Aaaand now we’re here! What an incredible year, can’t imagine what the next year holds for all of us, but 1 thing is for sure, ThursdAI will be here to keep you all up to date! P.S - If you scrolled all the way to here, DM me the 🎊 emoji so I know you celebrated with us! It really helps me to know that there is at least a few folks out of the thousands that get this newsletter that scrolls all the way through! This is a public episode. If you'd like to discuss this with other subscribers or get access to bonus episodes, visit sub.thursdai.news/subscribe | |||
08 Dec 2023 | 🌉 ThursdAI Dec 7th - Gemini is out-ish, Grok is out, OSS AI Event in SF, Waymo rides, and more AI news from the past week 👏 | 01:51:37 | |
ThursdAI December 7th TL;DR Greetins of the day everyone (as our panelist Akshay likes to sometimes say) and Happy first candle of Hannukah for those who celebrate! 🕎 I'm writing this newsletter from the back of an Waymo self driving car, in SF, as I'm here for just a few nights (again) to participate in the Open Source AI meetup, that was co-organized by Ollama and Nous Research, Alignment Labs and hosted by A16Z in their SF office. This event was the highlight of this trip, it was quite a packed meetup in terms of AI talent, and I got to meet quite a few ThursdAI listeners, mutuals on X, and AI celebs We also recorded the podcast this week from the arena, thanks to Swyx and Alessio from latentspace pod for hosting ThursdAI this week form their newly built out pod studio (and apologies everyone for the rocky start and the cutting out issues, luckily we had local recordings so the pod version sounds good!) Google finally teases Gemini Ultra (and gives us Pro) What a week folks, what a week, as I was boarding the flight to SF to meet with Open Source folks, Google announced (finally!) the release of Gemini, their long rumored, highly performant model with a LOT of fanfare! Blogposts authored by Sundar and Demis Hassabis, beautiful demos of unseen before capabilities, comparisons to GPT-4V which the Ultra version of Gemini outperforms on several benchmarks, and rumors that Sergey Brin, the guy who's net worth is north of 100Bn is listed as the core contributor on the paper and reports on benchmarks (somewhat skewed) show Ultra beaing GPT-4 on many coding and reasoning evaluations! We've been waiting for Gemini for such a long time, that we spend the first hour of the podcast discussing it and it's implications basically. We were also fairly disillusioned by the sleight of hand tricks Google marketing department played with the initial launch video, where it purportedly shows Gemini being a fully multi-modal AI, that reacts to a camera feed + user voice in real time, when in fact, it was quickly clear (from their developer blog) that it was not video+audio but rather images+text (the same two modalities we already have in GPT-4V and given some prompting, it's quite easy to replicate most of it. We've also discussed how we again, got a tease, and not even a waitlist for the "super cool" stuff, while getting a GPT3.5 level of a model today in Bard upgrade. To me, the most mind-blowing demo video was actually one of the other ones in the announcement, which showed that Gemini has agentic behavior in understanding user intent, asks for clarifications, creates a PRD (Product Requirement Document) for itself, and then, generates Flutter code to create a UI on the fly, based on what the use asked it! This is pretty wild, as we all should expect that Just In Time UI will come to many of these big models! Tune in to the episode if you want to hear more takes, opinions and frustrations as none of us actually got to use Gemini Ultra, and the experience with Gemini Pro (which is now live on Bard) was at least for me, underwhelming This weeks buzz (What I learned in Weights & Biases this week) I actually had a blast talking about W&B to many of the open source and fine-tuners community this and past week. I already learned that W&B doesn't only help huge companies (like OpenAI, Anthropic, Meta, Mistral and tons more) to train their foundational models, but is widely used by the open source fine-tuners community as well. I've met with folks like Wing Lian (aka Caseus), maintainer of Axolotl, who uses W&B together with Axolotl, and got to geek out about W&B, met with Teknium and LDJ (Nous Research, Alignment Labs) and in fact, got LDJ to walk me through some of the ways he uses and used W&B in the past, including how it's used to track model runs, show artifacts in the middle of runs, and run mini-benchmarks and evaluations for LLMS as they finetune. If you're interested in this, here's an episode of a new “series” of me learning publicly (from scratch) so if you want to learn from scratch with me, welcome to check it out: Open Source AI in SF meetup This meetup was the reason I flew in to SF, I was invited by dear friends in the open source community, and couldn't miss it! There was such a talent density there, it was quite remarkable. Andrej Karpathy who's video about LLM I just finished re-watching, Jeremy Howard, folks from Mistral, A16Z, and tons of other startups, open source collectives, and enthusiasts, all came together to listen to a few lightning talks, but mostly to mingle and connect and share ideas. Nous Research announced that they are a company (not anymore just a discord collective of rag tag open sourcers!) and that they are working on Forge, a product offering of theirs, that runs local AI, has a platform for agent behavior, and is very interesting to keep an eye for. I've spent most of my time going around, hearing what folks are using (Hint: a LOT of axolotl), what they are finetuning (mostly Mistral) and what is the future (everyone's waiting for next Llama or next Mistral). Funnily enough, there was not a LOT of conversation about Gemini there at all, at least not among the folks that I talked to! Overall this was really really fun, and of course, being in SF, at least for me, especially now as an AI Evangelist, feels like coming home! So expect more trip reports! Here's a recap and a few more things that happened this week in AI: * Open Source LLMs * Apple released MLX - machine learning framework on apple silicon * Mamba - transformers alternative architecture from Tri Dao * Big CO LLMs + APIs * Google Gemini beats GPT-4V on a BUNCH of metrics, shows cool fake multimodal demo * Demo was embellished per the google developer blog * Multimodal capabilities are real * Dense model vs MOE * Multimodel on the output as well * For 5-shot, GPT-4 outperforms Gemini Ultra on MMLU * AlphaCode 2 is here and Google claims it performs better than 85% competitive programmers in the world and it performs even better, collaborating with a competitive programmer. * Long context prompting for Claude 2 shows 27% - 98% increase by using prompt techniques * X.ai finally released grok to many premium+ X subscribers. (link) * Vision * OpenHermes Vision finally released - something there was not right there, back to drawing board * Voice * Apparently Gemini beats Whisper v3! As part of a unified model no less * AI Art & Diffusion * Meta - releases a standalong EMU AI art generator websites https://imagine.meta.com * Tools * Jetbrains finally releases their own AI native companion + subscription That's it for me this week, this Waymo ride took extra long as it seems that in SF, during night rush hour, AI is at a disadvatage against human drivers. Maybe I'll take an Uber next time. P.S - here’s Grok roasting ThursdAI See you next week, and if you've scrolled all the way here for the emoji of the week, it's hidden in the middle of the article, send me that to let me know you read through 😉 This is a public episode. If you'd like to discuss this with other subscribers or get access to bonus episodes, visit sub.thursdai.news/subscribe | |||
14 Dec 2023 | 📅 ThursdAI - Live @ NeurIPS, Mixtral, GeminiPro, Phi2.0, StripedHyena, Upstage 10B SoTA & more AI news from last (insane) week | 01:49:25 | |
Wow what a week. I think I’ve reached to a level that I’m not phased by incredible weeks or days that happen in AI, but I… guess I still have much to learn! TL;DR of everything we covered (aka Show Notes) * Open Source LLMs * Mixtral MoE - 8X7B experts dropped with a magnet link again (Announcement, HF, Try it) * Mistral 0.2 instruct (Announcement, HF) * Upstage Solar 10B - Tops the HF leaderboards (Announcement) * Together -Striped Hyena architecture and new models (Announcement) * EAGLE - a new decoding method for LLMs (Announcement, Github) * Deci.ai - new SOTA 7B model * Phi 2.0 weights are available finally from Microsoft (HF) * QuiP - LLM quantization & Compression (link) * Big CO LLMs + APIs * Gemini Pro access over API (Announcement, Thread) * Uses character pricing not token * Mistral releases API inference server - La Platforme (API docs) * Together undercuts Mistral with serving Mixtral by 70% and announces OAI compatible API * OpenAI is open sourcing again - Releasing Weak-2-strong generalization paper and github! (announcement) * Vision * Gemini Pro api has vision AND video capabilities (API docs) * AI Art & Diffusion * Stability announces Zero123 - Zero Shot image to 3d model (Thread) * Imagen 2 from google (link) * Tools & Other * Optimus from Tesla is coming, and it looks incredible This week started on Friday, as we saw one of the crazier single days in the history of OSS AI that I can remember, and I’ve been doing this now for .. jesus, 9 months! In a single say, we saw a new Mistral model release called Mixtral, which is a Mixture of Experts (like GPT4 is rumored to be) of 8x7B Mistrals, and beats GPT3.5, we saw a completely new architecture that competes with Transformers called HYENA from Tri Dao and Together.xyz + 2 new models trained with that architecture, we saw a new SoTA 2-bit quantization method called QuiP from cornell AND a new 3x faster decoding method for showing tokens to users after an LLM has done “thinking”. And the best thing? All those advancements are stackable! What a day! Then I went to NeurIPS2023 (which is where I am right now, writing these words!), which I cover at length at the second part of the podcast, but figured I’d write about it here as well, since it was such a crazy experience. NeurIPS is the biggest AIML conference, I think they estimated 15K people from all over the world attending! Of course this brings many companies to sponsor, raise booths, give out swag and try to record! Of course with my new position at Weights & Biases I had to come as well and experience this for myself! Many of the attendees are customers of ours, and I was not expecting this amount of love given, just an incredible stream of people coming up to the booth, and saying how much they love the product! So I manned the booth, did interviews and live streams, and connected with a LOT of folks and I gotta say, this whole NeurIPS thing is quite incredible from the ability to meet people! I hung out with folks from Google, Meta, Microsoft, Apple, Weighs & Biases, Stability, Mistral, HuggingFace and PHD students and candidates from most of the top universities in the world, from KAIST to MIT and Stanford, Oslo and Shaghai, it's really a worldwide endeavor! I also got to meet many of the leading figures in AI, all of whom I had to come up to and say hi, shake their hand, introduce myself (and ThursdAI) and chat about what they or their team released and presents at the conference! Truly an unforgettable experience! Of course, This Weeks’ Buzz is that, everyone here loves W&B, from the PHD students, to literally every big LLM lab! They all came up to us (yes yes, even researches at Google who kinda low-key hate their internal tooling) and told us how awesome the experience was! (besides Xai folks, Jimmy wasn’t that impressed haha) and of course I got to practice the pitch so many times, since I manned the W&B booth! Please do listen to the above podcast, there’s so much detail that’s in there that doesn’t get up on the newsletter, as it’s impossible to cover all, but it was a really fun conversation, including my excited depiction of this weeks NOLA escapades! I think I’ll end here, cause I can go on and on about the parties (There were literally 7 at the same time last night, Google, Stability, OpenAI, Runway, and I’m sure there were a few more I wasn’t invited to!) and about New Orleans food (it’s my first time here, I ate a soft shell deep fried crab and turtle soup!) and I still have the poster sessions to go to and workshops! I will report more on my X account and the Weights & Biases X account, so stay tuned for that there, and as always, thanks for tuning in, reading and sharing ThursdAI with your friends 🫡 P.S - Still can’t really believe I get to do this full time now and share this journey with all of you, bringing you all with me to SF, and now NeurIPS and tons of other places and events in the future! — Alex Volkov, AI Evangelist @ Weights & Biases, Host of ThursdAI 🫡 This is a public episode. If you'd like to discuss this with other subscribers or get access to bonus episodes, visit sub.thursdai.news/subscribe | |||
22 Dec 2023 | 🎄ThursdAI - LAION down, OpenChat beats GPT3.5, Apple is showing where it's going, Midjourney v6 is here & Suno can make music! | 01:21:36 | |
Hey everyone, happy ThursdAI! As always, here's a list of things we covered this week, including show notes and links, to prepare you for the holidays. TL;DR of all topics covered: * Open Source AI * OpenChat-3.5-1210 - a top performing open source 7B model from OpenChat team beating GPT3.5 and Grok (link, HF, Demo) * LAION 5B dataset taken down due to CSAM allegations from Stanford (link, full report pdf) * FLASK - New evaluation framework from KAIST - based on skillset (link) * Shows a larger difference between open/closed source * Open leaderboard reliability issues, vibes benchmarks and more * HF releases a bunch of MLX ready models (LLama, Phi, Mistral, Mixtral) (link) * New transformer alternative architectures - Hyena & Mamba are heating up (link) * Big CO LLMs + APIs * Apple - LLM in a flash paper is making rounds (AK, Takeaways thread) * Anthropic adheres to the messages API format (X) * Microsoft Copilot finally has plugins (X) * Voice & Audio * AI Music generation Suno is now part of Microsoft Copilot plugins and creates long beautiful songs (link) * AI Art & Diffusion * Midjourney v6 is out - better text, great at following instructions (link) Open Source AI We start today with a topic I didn't expect to be covering, the LAION 5B dataset, was taken down, after a report from Stanford Internet Observatory found instances of CSAM (Child Sexual Abuse material) in the vast dataset. The outlined report had identified hundreds to thousands of instances of images of this sort, and used something called PhotoDNA by Microsoft to identify the images by hashes, using a sample of NSFW marked images. LAION 5B was used to train Stable Diffusion, and 1.4 and 1.5 were trained on a lot of images from that dataset, however SD2 for example was only trained on images not marked as NSFW. The report is very thorough, going through the methodology to find and check those types of images. Worth noting that LAION 5B itself is not an image dataset, as it only contains links to images and their descriptions from alt tags. Obviously this is a very touchy topic, given the way this dataset was scraped from the web, and given how many image models were trained on it, the report doesn't allege anything close to influence on the models it was trained on, and outlines a few methods of preventing issues like this in the future. One unfortunate outcome of such a discovery, is that this type of work can only be done on open datasets like LAION 5B, while closed source datasets don't get nearly to this level of scrutiny, and this can slow down the advancement of multi-modal open source multi modal models while closed source will continue having these issues and still prevail. The report alleges they found and validated between hundreds to a few thousand of CSAM verified imagery, which considering the size of the dataset, is infinitesimally small, however, it still shouldn't exist at all and better techniques to clean those scraping datasets should exist. The dataset was taken down for now from HuggingFace and other places. New version of a 7B model that beats chatGPT from OpenChat collective (link, HF, Demo) Friend of the pod Alpay Aryak and team released an update to one of the best 7B models, namely OpenChat 7B (1210) is a new version of one of the top models in the 7B world called OpenChat with a significant score compared to chatGPT 3.5 and Grok and with very high benchmark hits (63.4% on HumanEval compared to GPT3.5 64%) Scrutiny of open source benchmarks and leaderboards being gamed We've covered State of the art models on ThursdAI, and every time we did, we covered the benchmarks, and evaluation scores, Whether that's the popular MMLU (Multi-Task Language Understanding) or HumanEval (Python coding questions) and almost always, we've referred to the HuggingFace Open LLM leaderboard for the latest and greatest models. This week, there's a long thread on the hugging face forums that HF eventually had to shut down, that alleges that a new contender for the top, without revealing methods, used something called UNA to beat the benchmarks, and folks are suggesting that it must be a gaming of the system, as a model that's trained on the benchmarks can easily top the charts. This adds to the recent observations from friend of the pod Bo Wang from Jina AI, that the BGE folks have stopped focusing on the MTEB leaderboard (Massive Text Embedding Benchmark) benchmarks as well, as those are also seem to be gamed (link) This kicked off a storm of a discussion about different benchmarks and evaluations, ability to score and check wether or not we're advancing, and the openness of these benchmarks. Including one Andrej Karpathy that chimed in that the only way to know is to read the r/LocalLlama comment section (e.g. vibes based eval) and check the ELO score on the LMSys chatbot arena, which pits 2 random LLMs behind the scenes and lets users choose the best answer/score. LMsys also has a leaderboard, and that one only includes models they have explicitly added to their Arena, and also merges 3 different scores, the ELO score by human raters, the MTBench score and the MMLU. This is the latest benchmark, showing that Mixtral is the highest ranking open source model at this point, and that a few other Apache 2.0 models like OpenChat (the previous version, the one from today should score even higher) and OpenHermes are inching closer as well and have honorable mentions given their license and size! However, given the latest releases in HuggingFace lineage, where you could track the model finetunes to what models they were fine-tuned on, it's still a good place to check out those leaderboards, just... self evaluation and running models on your own tasks is always a good idea! Also a good idea is additional benchmarks, like the one proposed by KAIST this week called FLASK that shows quite a significant distance between closed source models and open source ones based on several skills. This weeks Buzz (What I learned this week in Weights & Biases) This week we kicked off a buildweek internally, which unfortunately I wasn’t able to be a super active participant in, due to lying on my couch with a fever for most of the week, but regardless, I noticed how important is it to have these build weeks/hack weeks from time to time to actually use some of the new techniques we often talk about, like chain-of-density prompting techniques, or agent fine-tunes. I also got paired with my colleague Anish on our project, and while we work on our project (to be revealed later) he gave a kick ass webinar on the famous deeplearning.ai platform on the topic of enhancing performance for LLM agents in automation that more than 5K folks tuned into! Anish is a wealth of knowledge, so check it out if this topic interests you 👏 Big CO LLMs + APIs Apple - LLM in a Flash + MLX stuff Apple has been more and more in the AI news lately, having recently released MLX framework for running models directly on apple silicon devices, without a lot of dependencies, which was always possible, but is not optimized. This got many folks to start converting models to an MLX compatible format and there's no even a new tag on HF for those converted models But the main news this week don't stop there, folks from Apple also released the LLM in a flash paper, which shows advances in running LLMs in hardware restricted environments like smartphones, where memory is limited, and shows interesting promise, and also a glimpse that Apple is likely moving towards on device or partially on device inference at some point if we combine the MLX stuff and this paper attempts. Anthropic moves towards messages API Anthropic Claude finally gives us some DX and introduces a similar to OpenAI messages API. Voice Microsoft copilot now has plugins and can create songs! Microsoft copilot (FKA Bing Chat) now has Plugins (probably not new from this week, but we haven't yet reported on this) and one of the coolest ones is SUNO, which is an audio generation platform that has been around. And now it's super easy to create whole songs, directly from the Microsoft Copilot interface! Here’s my 1 shot attempt and creating a holiday jingle for ThursdAI, it’s not good, but it’s fun 😂 And I’ve seen some quite decent examples like return to monkey AI Art & Diffusion Midjourney v6 looks stunning and follows prompts very well Midjourney finally dropped their version 6, and it looks, really really good. Notably, it's likely the highest quality / fidelity diffusion model out there that we can use, has better support for text, and follows prompts closely. DALL-E is still very impressive for folks given that the iteration via chatGPT interface is very easy and convinient, but still ,just look at some of these MJv6 generations 😻 Nick gave it a very details prompt with 8 specific color assingments and besides the image looking insane, MJ nailed the super complex prompt! 35mm film still, two-shot of a 50 year old black man with a grey beard wearing a brown jacket and red scarf standing next to a 20 year old white woman wearing a navy blue and cream houndstooth coat and black knit beanie. They are walking down the middle of the street at midnight, illuminated by the soft orange glow of the street lights --ar 7:5 --style raw --v 6.0 And just for fun, here’s a comparison of all previous versions of MJ for the same prompt, just to… feel the progress 🔥 Thanks for reading all the way through, I think I got more than I bargained for during NeurIPS and I came back with a fever and was debating wether to even record/send this weeks newletter, but now that I’m at the end of it I’m happy that I did! Though, if you listen to the full recording, you may hear me struggling to breathe a bit 😅 So I’ll go rest up before the holidays, wishing you merry Christmas if you celebrate it 🎄 See you next week 🫡 This is a public episode. If you'd like to discuss this with other subscribers or get access to bonus episodes, visit sub.thursdai.news/subscribe | |||
29 Dec 2023 | 📅 ThursdAI - Dec 28 - a BUNCH of new multimodal OSS, OpenAI getting sued by NYT, and our next year predictions | 01:33:51 | |
Hey hey hey (no longer ho ho ho 🎄) hope you had a great Christmas! And you know that many AI folks have dropped tons of OpenSource AI goodies for Christmas, here’s quite a list of new things, including at least 3 new multi-modal models, a dataset and a paper/technical report from the current top model on HF leaderboard from Upstage. We also had the pleasure to interview the folks who released the Robin suite of multi-modals and aligning them to “good responses” and that full interview is coming to ThursdAI soon so stay tuned. And we had a full 40 minutes with an open stage to get predictions for 2024 in the world of AI, which we fully intent to cover next year, so scroll all the way down to see ours, and reply/comment with yours! TL;DR of all topics covered: * Open Source LLMs * Uform - tiny(1B) multimodal embeddings and models that can run on device (HF, Blog, Github, Demo) * Notux 8x7B - one of the first Mixtral DPO fine-tunes - (Thread, Demo) * Upstage SOLAR 10.7B technical report (arXiv, X discussion, followup) * Capybara dataset open sourced by LDJ (Thread, HF) * Nous Hermes 34B (finetunes Yi34B) - (Thread, HF) * Open Source long context pressure test analysis (Reddit) * Robin - a suite of multi-modal (Vision-Language) models - (Thread, Blogpost, HF) * Big CO LLMs + APIs * Apple open sources ML-Ferret multi-modal model with referring and grounding capabilities (Github, Weights, Paper) * OpenAI & Microsoft are getting sued by NewYorkTimes for copyright infringement during training (Full Suit) * AI Art & Diffusion & 3D * Midjourney v6 alpha is really good at recreating scenes from movies (thread) Open Source LLMs Open source doesn't stop even during the holiday break! Maybe this is the time to catch up to the big companies? During the holiday periods? This week we got a new 34B Nous Hermes model, the first DPO fine-tune of Mixtral, Capybara dataset but by far the biggest news of this week was in Multimodality. Apple quietly open sourced ml-ferret, an any to any model able to compete in grounding with even GPT4-V sometimes, Uform released tiny mutli-modal and embeddings versions for on device inference, and AGI collective gave NousHermes 2.5 eyes 👀 There's no doubt that 24' is going to be the year of multimodality, and this week we saw an early start of that right on ThursdAI. Ml-Ferret from Apple (Github, Weights, Paper) Apple has been in the open source news lately, as we've covered their MLX release previously and the LLM in a flash paper that discusses inference for low hardware devices, and Apple folks had 1 more gift to give. Ml-Ferret is a multimodal grounding model, based on Vicuna (for some... reason?) which is able to get referrals from images (this highlighted or annotated areas) and then ground the responses with exact coordinates and boxes. The interesting thing about the referring, is that it can be any shape, bounding box or even irregular shape (like the ferred in the above example or cat tail below) Ferret was trained on a large new dataset called GRIT containing over 1 million examples of referring to and describing image regions (which wasn't open sourced AFAIK yet) According to Ariel Lee (our panelist) these weights are only delta weights and need to be combined with Vicuna weights to be able to run the full Ferret model properly. Uform - tiny (1.5B) MLLMs + vision embeddings (HF, Blog, Github, Demo) The folks at Unum have released a few gifts for us, with an apache 2.0 license 👏 Specifically they released 3 vision embeddings models, and 2 generative models. Per the documentation the embeddings can yield 2,3x speedup improvements to search from Clip like models, and 2-4x inference speed improvements given the tiny size. The embeddings have a multi-lingual version as well supporting well over 20 languages. The generative models can be used for image captioning, and since they are tiny, they are focused on running on device, and are already converted to ONNX format and core-ML format. Seen the results below compared to LLaVa and InstructBLIP, both at the 7B range I've tried a few images of my own (you can try the demo here), and while there was hallucinations, this tiny model did a surprising amount of understanding for the size! Also shoutout to Ash Robin suite of multimodal models (Thread, Blogpost, HF) The folks at the CERC-AAI lab in MILA-quebec have released a suite of multi-modal models, that they have finetuned and released a fork of NousHermes2.5 that can understand images, building on top of CLIP, and SigLIP as the image encoder. In fact, we did a full interview with Irina, Kshitij, Alexis and George from the AGI collective, that full interview will be released on ThursdAI soon, so stay tuned, as they had a LOT of knowledge to share, from fine-tuning the clip model itself for better results, to evaluation of multimodal models, to dataset curation/evaluation issues and tips from Irina on how to get a government supercomputer compute grant 😈 Big CO LLMs + APIs OpenAI is being used by NYT for copyright infringement during training (Lawsuit) New York times is suing OpenAI and Microsoft for copyright infringement, seeking damages (amount unclear) and removal of NYT data from OpenAI models. The full lawsuit is a worthwhile read, and in includes a whopping 100 pages of examples of GPT4 completing NYT articles verbatim. I personally wasn't able to reproduce this behavior in the chatGPT app, but some folks on X suggested that it's possible in the OpenAI playground, with the right prompt and NYT URL in the prompt. This lawsuit came after a round of attempted negotiations between NYT and OpenAI, which apparently failed, and it's worth noting a few things. First, OpenAI (with almost every other AI company) have a "Copyright shield" feature, where they protect the user of these services from getting sued for copyright violations. So there is no direct exposure for customers of OpenAI. Additional thing of note is, the NYT information is compiled not by OpenAI directly, rather, OpenAI (and almost every other LLM) have used the CommonCrawl dataset (among others) which did the crawling and collection of text itself. Per the CommonCrawl license, OpenAI should have reached out to each individual URL in that dataset and worked out the copyright on their own, which is a bit difficult to do, as CommonCrawl includes 3-5 billion pages collected each month. Regardless of the claims, the hottest takes I saw in regards to this are, that by the time anything moves with this lawsuit, we will be on GPT-6 or so and it won't matter by then, or that OpenAI will have to retrain a model without NYT data, which I find quite ludicrous personally and very unlikely to happen. If this lawsuit actually sets a precedent, this will IMO be a very bad one for the US, considering other countries like Japan are already getting ahead of this, declaring all scraped data as fair us if used for training (source) Of course, all of X became IP experts overnight, and the debates are very interesting, some are confusing technical terms, some are claiming that OpenAI will just cave and pay NYT, while some super libertarian ones take it all the way down to: if AI has human rights, and if it does, then preventing it learning from copyright material is like preventing people to read Hemingway. This weeks buzz (What I learned in WandB this week) This week, we sent out our annual emails of wrapped cards for everyone who used Weights & Biases to train models this year. This is a yearly tradition, similar to Spotify, however, for ML purposes, and this year the cards were generated with stable diffusion XL, generating hundreds of thousands of images based on autogenerated model run names! The interesting thing I noticed also, is just how many folks shared their stats screenshots right from the email we send, including not only how many hours they spend training models this year, but also how many other features they used, like reports and sweeps. And I noticed just how many folks don't use reports, which is a shame, as it's such a cool feature! WandB literally has a built in blogging platform for all your ML needs and it includes live widgets of every metric you're tracking in your runs, it's really great. AI Art & Diffusion Midjourney v6 is incredible at recreating actual movie stills and scenes (Thread) Another potential lawsuit is waiting to happen? We already saw lawsuits against StabilityAI for supposed copyright infringement and stability did a lot of work to exclude proprietary art from their training datasets, however, the new incredible version of Midjourney, shows just.. a mind-blowing accuracy in recreating scenes from movies, and cartoon styles. Just look at some of these examples (collected by some folks on X) This + the above lawsuit news coming for OpenAI & Microsoft from New York Times is setting up 24' to be the year where copyright law and AI finally meet for real. And we'll keep reporting on the outcomes. Predicitons for 24' In the last 20 minutes of the pod recording we opened up the floor to folks giving us their predictions for AI developments in the year 2024, and I also asked this question on X itself. The idea was, to come back next year during our yearly summary and see which predictions we hit, and which predictions we were not even remotely thinking about! Here's a list of predictions with their category (Thanks to AI to help me sort these from different sources and transcription) * Open Source LMs * 1GB models with Mix Trail performance levels - Nisten * Continual pretraining and building on top of each other's work - Irina Rish * Smaller models trained on more data - Irina Rish * Consolidation and standardization of models - Irina Rish * Agents running on 7B models with capabilities like web search and code interpretation - Shroominic * End of dominance of transformer architecture - Far El * Marriage of reinforcement learning and language models - Far El * New benchmarking standards - Far El * Plug and play weights for expertise - Umesh * Self-improving pipeline framework - Umesh * Big Companies/APIs * Mistral to become a major player, surpassing companies like Anthropic - Alex Volkov * Apple AI device with multimodal capabilities - Umesh * Google Gemini Pro commoditizing APIs - Umesh * Model that can ace undergrad computer science curriculum - George Adams * Extremely good but expensive model (~$1 per response) - Shroominic * Apple spatial computing + AI product innovation - John Doe * Real-time multilingual translation app/device - Umesh * Vision/Video * AI-generated full length feature film - Umesh * Artist AI model galleries for art generation - Umesh * Real-time video understanding and multimodal models - Alex Volkov * Public release of high quality, fast voice cloning tech - Alex Volkov * 3D model/animation generation for video games - tobi * Meta will outperform most companies in video AI and mixed reality - Alex Volkov * Other * Localized national AI models - Ravi * Rise in use of deepfakes - Ravi * Surge in metadata embedding for ownership identification - R.AI.S.E * Advances in AI for biology/healthcare - Ravi, Ash Vardanian * A model capable of completing an undergrad CS curriculum at an A level by the end of the year - George Adams * AI device, fully capable of multimodal capabilities, from Apple - Educated Guess * Development in domain-specific LMs for bio applications, especially in synthetic biology - Ravi * Twitter Prediction * CodeInterpreterAPI V2 - Shroominic * Gemini will NOT outperform ChatGPT - Alex Northstar * Tech slowdown in mass adoption, human creativity as bottleneck - “charles harben” * Biology and Robots - Sinan * Code LLMs near junior developer productivity - Karthik Kannan * Tokenizers will work - Geronimo * LLM curve plateaus, focus on refining and multimodal, OpenAI settles with NYT - hokiepoke * Fully generated, rigged, voiced game characters, minimal human intervention - Rudzinski Maciej * AI affects politics - 𝕄𝕏𝕊ℍℝ🤖 * Audio reaches DallE3 level, video and 3D advancements, new cool modality - Darth thromBOOzyt * Synthetic data will be huge - Leo Tronchon Ok now that our predictions are here, we'll come back here next year and see who hit what predictions! If you have predicitons if your own, please reply to this email/substack and post them here as well, so we'll have a record 🫡 With that, I want to wish you a happy new year, and as always, see you here next week 👏 This is a public episode. If you'd like to discuss this with other subscribers or get access to bonus episodes, visit sub.thursdai.news/subscribe | |||
05 Jan 2024 | 📅 ThursdAI Jan 4 - New WizardCoder, Hermes2 on SOLAR, Embedding King? from Microsoft, Alibaba upgrades vision model & more AI news | 01:38:57 | |
Here’s a TL;DR and show notes links * Open Source LLMs * New WizardCoder 33B V1.1 - 79% on HumanEval (X, HF) * Tekniums Hermes 2 on SOLAR 10.7B (X, HF) * Microsoft - E5 SOTA text embeddings w/ Mistral (X, HF, Paper, Yams Thread) * Big CO LLMs + APIs * Samsung is about to announce some AI stuff * OpenAI GPT store to come next week * Perplexity announces a $73.6 Series B round * Vision * Alibaba - QWEN-VL PLUS was updated to 14B (X, Demo) * OCU SeeAct - GPT4V as a generalist web agent if grounded (X, Paper) * Voice & Audio * Nvidia + Suno release NeMo Parakeet beats Whisper on english ASR (X, HF, DEMO) * Tools & Agents * Stanford - Mobile ALOHA bot - Open source cooking robot (Website, X thread) Open Source LLMs WizardCoder 33B reaches a whopping 79% on HumanEval @pass1 State of the art LLM coding in open source is here. A whopping 79% on HumanEval, with Wizard Finetuning DeepSeek Coder to get to the best Open Source coder, edging closer to GPT4 and passing GeminiPro and GPT3.5 👏 (at least on some benchmarks) Teknium releases a Hermes on top of SOLAR 10.7B Downloading now with LMStudio and have been running it, it's very capable. Right now SOLAR models are still on top of the hugging face leaderboard, and Hermes 2 now has 7B (Mistral) 10.7B (SOLAR) and 33B (Yi) sizes. On the podcast I've told a story of how this week I actually used the 33B version of Capybara for a task that GPT kept refusing to help me with. It was honestly kind of strange, a simple request to translate kept failing with an ominous “network error”. Which only highlighted how important the local AI movement is, and now I actually have had an experience myself of a local model coming through when a hosted capable one didn’t Microsoft releases a new text embeddings SOTA model E5 , finetuned on synthetic data on top of Mistral 7B We present a new, easy way to create high-quality text embeddings. Our method uses synthetic data and requires less than 1,000 training steps, without the need for complex training stages or large, manually collected datasets. By using advanced language models to generate synthetic data in almost 100 languages, we train open-source models with a standard technique. Our experiments show that our method performs well on tough benchmarks using only synthetic data, and it achieves even better results when we mix synthetic and real data. We had the great please of having Bo Wang again (One of the authors of the Previously SOTA Jina embeddings and a previous podcast gust) to do a deepdive into embeddings and specifically E5 with it's decoder only architecture. While the approach Microsoft researchers took here are interesting, and despite E5 claiming a top spot on the MTEB leaderboard (pictured above) this model doesn't seem to be super practical for most purposes folks use embeddings right now (RAG) for the following reasons: * Context length limitation of 32k, with a recommendation not to exceed 4096 tokens. * Requires a one-sentence instruction for queries, adding complexity for certain use cases like RAG. * Model size is large (14GB), leading to higher costs for production use. * Alternative models like bge-large-en-v1.5 are significantly smaller (1.35GB). * Embedding size is 4096 dimensions, increasing the cost for vector storage. Big CO LLMs + APIs OpenAI announces that the GPT store is coming next week! I can't wait to put the visual weather GPT I created and see how the store prompts it and if I get some revenue share like OpenAI promised during dev day. My daughter and I are frequent users of Alice - the kid painter as well, a custom GPT that my Daughter named Alice, that knows it's speaking to kids over voice, and is generating coloring pages. Will see how much this store lives up to the promises. This weeks Buzz (What I learned with WandB this week) This week was a short one for me, so not a LOT of learnings but I did start this course from W&B, called Training and Fine-tuning Large Language Models (LLMs). It features great speakers like Mark Sarufim from Meta, Jonathan Frankle from Mosaic, and Wei Wei Yang from Microsoft along with W&B MLEs (and my team mates) Darek Kleczek and Ayush Thakur and covers the end to end of training and fine-tuning LLMs! The course is available HERE and it's around 4 hours, and well well worth your time if you want to get a little more knowledge about the type of stuff we report on ThursdAI. Vision SeeAct - GPT4V as a generalist web agent if grounded (X, Paper) In June OSU NLP released Mind2Web which is a dataset for developing and evaluating web acting agents, LLMs that click buttons and perform tasks with 2350 tasks from over 130 website, stuff like booking flights, finding folks on twitter, find movies on Netflix etc' GPT4 without vision was terrible at this (just by reading the website html/text) and succeeded at around 2%. With new vision LMMs, websites are a perfect place to start training because of the visual (how website is rendered) is no paired with HTML (the grounding) and SeeAct uses GPT4-V to do this SeeAct is a generalist web agent built on LMMs like GPT-4V. Specifically, given a task on any website (e.g., “Compare iPhone 15 Pro Max with iPhone 13 Pro Max” on the Apple homepage), the agent first performs action generation to produce a textual description of the action at each step towards completing the task (e.g., “Navigate to the iPhone category”), and then performs action grounding to identify the corresponding HTML element (e.g., “[button] iPhone”) and operation (e.g., CLICK, TYPE, or SELECT) on the webpage. SeeAct achieves a 50% score on the Mind2Web evaluation task! QWEN-VL was updated to PLUS (14B) and it's pretty good compared to GPT4V Capabilities include: image captioning, visual question answering, visual grounding, OCR, visual reasoning. We had a chat with Junyang Lin, the tech lead for Qwen with Alibaba on the pod, and he mentioned specifically that they noticed that adding a larger "brain" (as in, LLM) to vision models, significantly increases the performance and vision understanding of the LMMs. While this model is not yet released, you can demo it here, and Junyang told us that it is coming to a release, like the previous QWEN models did before. I noticed the advanced OCR capabilities and understanding, this example was really spot on. Notice the "logo for Browser company" , the model understood that this text was in fact a logotype! (which even GPT4V failed at in my test) Voice Parakeet from NVIDIA beats Whisper on English with a tiny model (blog) Brought to you by @NVIDIAAI and @suno_ai_, parakeet beats Whisper and regains its first place. The models are released under a commercially permissive license! The models inherit the same FastConformer architecture and come in 2 flavors: 1. RNNT (1.1B & 0.6B) 2. CTC (1.1B & 0.5B) Each model is trained on 65K hours of English data (40K private proprietary data by Suno & NeMo teams) over several hundred epochs. Key features of the parakeet model: 1. It doesn't hallucinate (if the audio sample has silence, the output is silent). 2. It is quite robust to noisy audio (if the audio sample has non-vocal sounds, it outputs silence). We had the great please to have VB from the Audio team at HuggingFace, and he went in depth into the way in which Parakeet is better than Whisper (higher quality transcriptions while also being much much faster), it was trained on only 65K hours vs a few million with whisper, and we also covered that because of this different architecture, Parakeet is not able to receive any guidance for words that are hard for it to understand. For example, with whisper, I often provide ThursdAI in initial_prompt parameter to help guide whisper to know what it should say. Regardless, having a model that's superfast, and can beat whisper, and is commercially licensed to build on top of is incredible! Here's a demo for you to try it out and it's available with the NVIDIA NeMO framework. Coqui shuts down :( We've had Josh from Coqui on our pod before, when they released XTTS, and they have been friends ever since. It's sad to see Coqui shut down, and we want to wish all the team an easy and great transition 👏 You guys did a great job and we're rooting for each and every one of you. * Coqui is closing down. * The team is praised for being small yet impactful, competing with big tech despite limited resources. * Coqui began as the Machine Learning Group at Mozilla, creating DeepSpeech, Common Voice, and TTS. * Spun out as Coqui in 2021 to accelerate their mission. * Major achievement: XTTS, with openly released model weights for versions 1 and 2. * 2021: Coqui STT v1.0 released, Coqui Model Zoo and SC-GlowTTS launched. * 2022: YourTTS became viral, numerous open-source releases, team expansion. * 2023: Coqui Studio webapp and API launched, XTTS open release, first customers acquired. * Acknowledgment of the community, investors, customers, and partners for their support. * Partners include HuggingFace, Mozilla, Masakhane, Harvard, Indiana University, Google, MLCommons, Landing AI, NVIDIA, Intel, and Makerere University. * Future of generative AI in 2024 predicted to grow, with open-source playing a significant role. * Coqui TTS remains available on Github for further innovation. Tools Stanford Mobile ALOHA bot open sources, shows cooking Back in March, Stanford folks introduced ALOHA, (A Lowcost Open Hardware system for Bimanual Teleoperation) Basically a 4 arm robot, that a human operator can operate tasks and do fine motor skills like break an egg or tie ziptie. Well now, just 10 months later, they are introducing the Mobile version. A mounted ALOHA gear, that uses the human to perform tasks like cooking, calling the elevator and is able to learn from those actions, and then perform them.The operating gear can be easily detached for self operation, it's mobile so compute and battery pack are on the wheel base. Recently Meta released a huge dataset of first person operations called Ego-Exo 4D which combines first person and third person perspective for a big variety of tasks, such as cooking, cleaning, sports, healthcare and rock climbing, and this open hardware from Stanford is an additional example of how fast robotics advances into the physical world ThursdAI - Recaps of the most high signal AI weekly spaces is a reader-supported publication. To receive new posts and support my work, consider becoming a free or paid subscriber. And just like that, the first ThursdAI of the year is done! 🫡 Thank you for being a subscriber, see you next week 👏 This is a public episode. If you'd like to discuss this with other subscribers or get access to bonus episodes, visit sub.thursdai.news/subscribe | |||
12 Jan 2024 | 📅 ThursdAI Jan 11 - GPTs store, Mixtral paper, Phi is MIT + Phixtral, 🥯 by Jon Durbin owns the charts + Alex goes to SF again and 2 deep dive interviews 🎙️ | 01:16:41 | |
Hey hey everyone, how are you this fine ThursdAI? 👋 I’m gud thanks for asking! I’m continuing my experiment of spilling the beans, and telling you about everything we talked about in advance, both on the pod and in the newsletter, so let me know if this is the right way to go or not, for the busy ones it seems that it is. If you don’t have an hour 15, here’s a short video recap of everything we chatted about: ThursdAI - Jan 11 2024 TL;DR TL;DR of all topics covered + Show notes * Open Source LLMs * 🔥 Donut from Jon Durbin is now top of the LLM leaderboard (X, HF, Wolframs deep dive and scoring) * OpenChat January Update - Best open source 7B LLM (X, Hugging Face) * Our friends at NousResearch announce a seed round of 5.2M as their models pass 1.2 million downloads (X) * Argilla improved (Distillabeled?) the DPO enhanced Neural Hermes with higher quality DPO pairs (X) * New MoEs are coming out like hotcakes - PhixTral and DeepSeek MoE (X, Omar Thread, Phixtral Thread) * Microsoft makes Phi MIT licensed 👏 * Big CO LLMs + APIs * OpenAI adds personalization & team tiers (Teams announcement) * OpenAI launches GPT store (Store announcement, Store link) * Mixtral medium tops the LMsys human evaluation arena, is the best LLM overall after GPT4 👏 (X) * Hardware * Rabbit R1 is announced, $200/mo without a subscription, everybody has a take (X) * This weeks Buzz from Weights & Biases * Hackathon with Together, Langchain and WandB (and ME!) this weekend in AGI house (X, Signup) * Video * Bytedance releases MagicVideo-V2 video gen that looks great and passes Pika labs in human tests (X) * AI Art & Diffusion & 3D * Luma launched their online version of Genie and it's coming to the API (X) * Show notes and links mentioned * MergeKit (github) * Jon Durbins Contextual DPO dataset (HuggingFace) * Phixtral from Maxime Lebonne (X, HuggingFace) * WandGPT - out custom Weights & Biases GPT (GPT store) * Visual Weather GPT by me - https://chatg.pt/artweather * Ask OpenAI to not train on your chats - https://privacy.openai.com/policies AI Hardware It seems that the X conversation had a new thing this week, the AI hardware startup Rabbit, showcased their new $200 device (no subscriptions!) at CES and everyone and their mom had an opinion! We had quite a long conversation about that with (his first time on ThursdAI 👏) as we both pre-ordered one, however there were quite a few red flags, like for example, GPUs are costly, so how would an AI device that has AI in the cloud just cost a 1 time 200 bucks?? There were other interesting things they showed during the demo, and I’ll let you watch the full 30 minutes and if you want to read more, here’s a great deeper dive into this from . UPDATE: Ss I’m writing this, the CEO of Rabbit (who’s also on the board of Teenage Engineering, the amazing company that designed this device) tweeted that they sold out the initial first AND second batch of 10K unites, netting a nice $2M in hardware sales in 48 hours! Open Source LLMs Mixtral paper dropped (ArXiv, Morgans take) Mistral finally published the paper on Mixtral of experts, the MoE that's the absolutel best open source model right now, and it's quite the paper. Nisten did a full paper reading with explanations on X space, which I co-hosted and we had almost 3K people tune in to listen. Here's the link to the live reading X space by Nisten. And here's some notes courtecy Morgan McGuire (who's my boss at WandB btw 🙌) Strong retrieval across the entire context window Mixtral achieves a 100% retrieval accuracy regardless of the context length or the position of passkey in the sequence. Experts don't seem to activate based on topic Surprisingly, we do not observe obvious patterns in the assignment of experts based on the topic. For instance, at all layers, the distribution of expert assignment is very similar for ArXiv papers (written in Latex), for biology (PubMed Abstracts), and for Philosophy (PhilPapers) documents. However... The selection of experts appears to be more aligned with the syntax rather than the domain Datasets - No info was provided to which datasets Mixtral used to pretrain their incredible models 😭 Upsampled multilingual data Compared to Mistral 7B, we significantly upsample the proportion of multilingual data during pretraining. The extra capacity allows Mixtral to perform well on multilingual benchmarks while maintaining a high accuracy in English Mixtral Instruct Training We train Mixtral – Instruct using supervised fine-tuning (SFT) on an instruction dataset followed by Direct Preference Optimization (DPO) on a paired feedback dataset and was trained on @CoreWeave Jon Durbin Donut is the 🤴 of open source this week 6 of the top 10 are donut based models or merges of it. If you remember Auroborous, Donut includes that dataset, and there are two varieties there, the DPO and the non DPO versions of Bagel, including two merges from Cloudyu, which are non trained merges with mergekit, based on Donut. Jon pro tip for selecting DPO vs Non DPO models is FYI, the DPO version is more factual, truthful, better at math, etc., but is not great for RP, creative writing, etc. Use non-DPO for those tasks! Donut includes an impressive amount of dataset mixed together, which are all linked from the model card but here they are: "ai2_arc, airoboros, apps, belebele, bluemoon, boolq, capybara, cinematika, drop, emobank, gutenberg, lmsys_chat_1m, mathinstruct, mmlu, natural_instructions, openbookqa, pippa, piqa, python_alpaca, rosetta_code, slimorca, spider, squad_v2, synthia, winogrande, airoboros 3.1 vs airoboros 2.2.1, helpsteer, orca_dpo_pairs" Jon also shared his end of the year WandB report nad has trained a whopping 917 models this year for a total of ~2500 hours and is in the top 10% of the top active users (among 800K or so users) I didn't know that Jon is going to join, but was so happy that he joined the live recording that we ended up chatting for 20 minutes, and there was so many nuggets in that conversation, about how to prepare DPO datasets, which other ones Jon has been releasing, and just a bunch more gold, that I decided to CUT that out and post it as a separate special deepdive episode that's going to get released on the Sunday special. Stay tuned for that! Nous Research announces $5.2 million funding seed round as they cross 1.1 million model downloads on the hub Congrats to Karan, Emozilla, Teknium, Bowen, Shivani and the rest of the Nous team on this great news! 👏 We expect to hear more from them in the coming year, with a consistent commitment to open source, keep open sourcing the best models, and the upcoming Forge news! With investors like Balaji, OSS capital, Vipul from Together, Nous completes the $5.2M seed round, and we had Karan (one of the co-founders of Nous) on the pod to chat to use about what they are planning to do with that money and what are their continuous commitments to open source! In addition, they just recently passed 1.1 million downloads on the hub with Nous-Hermes-2-34B being their best model! 🤴 OpenChat Jan update becomes the leading open source 7B model (X, Hugging Face) This update mainly enhanced training methodology, in-context learning & coding skills, outperforming the last 1210 release on 7 out of 8 benchmarks! and scores 71.3 on HumanEval, 65.8% on MMLU 👏 The previous version of OpenChat trails just behind OpenHermes on the human evals on Lmsys arena, but both are incredible 7B models. Argilla - Argilla used their Distilabel tool to build a preference dataset from ratings and critiques of AI response pairs, taking around 3 hours - The original dataset assumed the GPT-4/3.5 responses were always best, but Argilla found this was not always the case - Their dataset confirmed ~4,000 pairs had the same rating, 7,000 pairs were unchanged, and ~2,000 times the rejected response was preferred - Improving existing DPO datasets with higher quality pairs is important for model fine-tuning - They are releasing an improved version of the popular Orca Pairs DPO dataset from Intel, and a new OpenHermes model outperforming baselines with 54% fewer DPO pairs Big CO LLMs + APIs OpenAI has a big week, launches GPTs store and team pro accounts (Blog) Things of note about the store: * My GPTs are getting feedback and crossed 10K chats , was #6 on lifestyle and the disappeared, but has gained 2x more chats in 24 hours since the store has launched! * Discoverability is great, trending GPTs are shown clearly, and folks are getting a lot of exposure * Copycats already started copying a bunch of the great GPTs, see this example of what happens when you search for Gymstreak, most of the top GPTs are already being copy-catted. Team accounts: $25/mo per user for annual plans and at least 2 teams The biggest confusion was from folks who didn't understand that OpenAI trains on Pro conversations, and there's an option to Opt-out! This weeks Buzz (What I learned with WandB this week) Weights and Biases (and ME!) are going to AGI house to lead a Rag vs Finetune hackathon with cool prizes! There's still time to RSVP, will incredible guests speakers, this Hackathon is organized together with... LangChain, TogetherCompute and AGI house - If you're in the SF area, and you wanna hack on some cool RAG things and get awesome prizes (and meet me!) join the waitlist here https://partiful.com/e/AlntdLtxh9Jh1J6Pcsma Vision & Video Luma released GENIE on Web and IOS, if you remember, we covered the GENIE text-to-3d model they first released on discord a while ago, and now it's incorporated into the luma website, and is significantly higher quality 3D assets. The generations are free for now, and they look awesome! Here are some of mine, I created a Bee holding a Wand (get it? WandB? 😆) and a polish bear (internal joke) and they look so cool! Friend of the pod and recent LUMA hire Arthur Islamov jumped on and also told us that this is coming to the API, so developers would be able to automate asset creation and generate tons of 3D objects programmatically, and use cool prompt techniques to make sure they are a bit better every time maybe? Great news! AI Art & Diffusion Bytedance announces MagicVideo-V2 (Arxiv, Project) We didn't get anything besides quite a few cherry picked videos and a paper, so we can't use this yet, but wow some of these videos look incredible! MagicVideo-V2 that integrates the text-to-image model, video motion generator, reference image embedding module and frame interpolation module into an end-to-end video generation pipeline. Benefiting from these architecture designs, MagicVideo-V2 can generate an aesthetically pleasing, high-resolution video with remarkable fidelity and smoothness. It demonstrates superior performance over leading Text-to-Video systems such as Runway, Pika 1.0, Morph, Moon Valley and Stable Video Diffusion model via user evaluation at large scale Lastly, I had the greatest time to interview my new friend João Moura, the creator of Crew AI, which been popping off, was the #1 trending on Github and #2 of the day on Product hunt, and is essentially an AI framework that lets you create a crew of AI agents to do tasks for you. I will be polishing up that conversation and post it together with the deep dive with Jon, so stay tuned, but here’s a sneak preview of how cool this is and expect that episode to drop soon! This is a public episode. If you'd like to discuss this with other subscribers or get access to bonus episodes, visit sub.thursdai.news/subscribe | |||
15 Jan 2024 | 🔥 ThursdAI Sunday special - Deep dives into Crew AI with Joao then a tasty Bagel discussion with Jon Durbin | 00:42:23 | |
ThursdAI - Sunday special deep dive, interviews with Joao, and Jon, AI agent Crews and Bagel Merges. Happy Sunday dear reader, As you know by now, ThursdAI pod is not a standard interview based podcast, we don't focus on a 1:1 guest/host conversation, but from time to time we do! And this week I was very lucky to have one invited guest and one surprise guest, and I'm very happy to bring you both these conversations today. Get your Crew together - interview with João Moura, creator of CrewAI We'll first hear from João Moura, the creator of Crew AI, the latest agent framework. João is a director of AI eng. at Clearbit (acquired by Hubspot recently) and created Crew AI for himself, to automate many of the things he didn't want to keep doing, for example, post more on Linkedin. Crew has been getting a lot of engagement lately, and we go into the conversation about it with João, it's been trending #1 on Github, and received #2 product of the day when Chris Messina hunted this (to João's complete surprise) on Product Hunt. CrewAI is built on top of Langchain, and is an agent framework, focusing on Orchestration or role-playing, autonomous agents. In our chat with João we go into the inspiration, the technical challenges and the success of CrewAI so far, how maintenance for crew is now partly a family effort and what's next for crew Merges and Bagels - chat with Jon Durbin about Bagel, DPO and merging The second part of today's pod was a conversation with Jon Durbin, a self described AI tinkerer and software engineer. Jon is a Sr. applied AI researcher at Convai, and is well known in our AI circles as a master finetuner and dataset curator. This interview was not scheduled, but I'm very happy it happened! If you've been following along with the AI / Finetuning space, Jon's Airoboros dataset and set of models have been often mentioned, and cited, and Jon's latest work on the Bagel models took the lead on HuggingFace open LLM leaderboard So when I mentioned on X (as I often do) that I'm going to mention this on ThursdAI, Jon came up to the space and we had a great conversation, in which he shared a LOT of deep insights into finetuning, DPO (Direct Preference Optimizations) and merging. The series of Bagel dataset and models, was inspired by the Everything Everywhere All at Once movie (which is a great movie, watch it if you haven't!) and is alluding to, Jon trying to throw as many datasets together as he could, but not only datasets! There has been a lot of interest in merging models recently, specifically many folks are using MergeKit to merge models with other models (and often a model with itself) to create larger/better models, without additional training or GPU requirements. This is solely an engineering thing, some call it frankensteining, some frankenmerging. If you want to learn about Merging, Maxime Labonne (the author of Phixtral) has co-authored a great deep-dive on Huggingface blog, it's a great resource to quickly get up to speed So given the merging excitement, Jon has set out to create a model that can be an incredible merge base, many models are using different prompt techniques, and Jon has tried to cover as many as possible. Jon also released a few versions of Bagel models, DPO and non DPO, that and we had a brief conversation about why the DPO versions are more factual and better at math, but not great for Role Playing (which is unsurprisingly what many agents are using these models for) or creative writing. The answer is, as always, dataset mix! I learned a TON from this brief conversation with Jon, and if you're interested in the incredible range of techniques in the Open Source LLM world, DPO and Merging are definitely at the forefront of this space right now, and Jon is just at the cross-roads of them, so definitely worth a listen and I hope to get Jon to say more and learn more in future episodes so stay tuned! So I'm in San Francisco, again... As I've mentioned on the previous newsletter, I was invited to step in for a colleauge and fly to SF to help co-host a hack-a-thon with friends from TogetherCompute, Langchain, in AGI house in Hillsborough CA. The Hackathon was under the Finetune VS RAG theme, because, well, we don't know what works better, and for what purpose. The keynote speaker was Tri Dao, Chief Scientist @ Together and the creator of Flash Attention, who talked about SSM, State space models and Mamba. Harrison from Langchain gave a talk with a deepdive into 5 techniques for knowledge assistants, starting with basic RAG and going all the way to agents 👏 I also gave a talk, but, I couldn't record a cool gif like this for myself, but thanks to Lizzy I got a pic as well 🙂 Here is the link to my slides if interesting (SLIDES) More than 150 hackers got together to try and find this out, and it was quite a blast for me to participate and meet many of the folks hacking, hear what they worked on, what worked, what didn't, and how they used WandB, Together and Langchain to achieve some of the incredible results they hacked together in a very short time. The projects showcased a range of creative applications leveraging RAG, finetuning, and other large language models. Several projects like Magic RAG, CareerNavigator-AI, and CompetitionAI used RAG for document retrieval and knowledge enhancement. Others like rags2pizza and Naturalist DALL-E focused more on finetuning models for specific domains. Some projects compared finetuning and RAG, finding that combining both gives superior performance over using either alone but that result wasn't conclusive. My vote as a judge (which I did not expect to be) eventually went to the team that built the OptiMUS project, they had generated a systentic dataset, cleaned it up, finetuned a model on it, and showed that they want to optimize AI agents. They used WandB to track their work and I hope they take this project forward and keep making advancements in AI. Congrats for the win Ali and Shayan, hope you enjoy the WandB branded Airpods (even I don't have those) and the Meta Quest, well deserved! Thank you for tuning in! See you next week! Full Transcription : [00:00:00] Alex Volkov: Hi. Welcome back to Thursday. The Sunday special episode. This is Alex Volkov. And I'm recording this in. A gorgeous space. In San Francisco. Where I was. Invited to judge hackathon. And now I'm hanging out with a few friends from cerebral valley. So thank you. Valley folks. For letting me use this place for recording and Today, we have a special episode for you. As If you hear this on Sunday. Today's not a Thursday. We often times have special guests on the pod. Where conversations. Or deeper. [00:00:45] Alex Volkov: And usually I reserve that slot for a Sunday special release. So this is what you're hearing now. In today's episode, we actually have two conversations. Although I only planned on one. And the first part is the planned part that you hear from Joao Maura. He is a director of AI in Clearbit, and now acquired by HubSpot. And he's also the creator of Crew AI and the Gentek AI framework that can run. By orchestrating. [00:01:14] Alex Volkov: c [00:01:15] Alex Volkov: The digital AI agents and have them work together. [00:01:19] Alex Volkov: And I think you'll hear from, Joao why this peaked interest. For many folks. Specifically. Because as we caught up with. Wow. [00:01:29] Alex Volkov: Crew AI was trending on GitHub and getting number two on product hunt at the same time. And it's a really cool framework. And I think the underlying. Power of this is that it can use open source, local models. A lot of previous agent attempts used GPT4 For example, and the crew AI can use things like Mistral or Mixtral running in LM studio or Ollama on your Mac, which I think is super cool. [00:01:55] Alex Volkov: And I think on device AI, plus something like this framework is going to be very, very powerful. It was a great conversation was wow. And surprising to me, the second guest was not planned. However you may have heard from the previous Thursday that the. Bagel series of models from a. Self-proclaimed AI, tinker, John Durbin. Have taken over the leaderboards on hung and face. Including a bunch of mergers and we haven't. Done a deep dive into merges and merge good and Franklin state models. [00:02:32] Alex Volkov: But if you've been to Thursday for awhile, you probably heard about them. Merging is a technique to take a model or different models. And without any computation, great, bigger or different models using a dissection and some computing. Process of the layers of those models just based on weights without any training or continuing to fine tuning, which is incredibly interesting. [00:02:58] Alex Volkov: And John goes into this a little bit and he created. Bagel. Based on the inference of what I'll let you hear this at the end. And it's a very fascinating conversation. I took a lot from it and unfortunately we didn't have time for a long, deep dive, but I learned a lot from John and hopefully he'll come from the podcast and we'll be able to deep even dive even deeper and talk with John about. How to create data sets, why DPO is better than PPO and all of these great things. So we had two great guests. And I. Had a blast having them on the bud and I probably should do more of these deep dives. [00:03:37] Alex Volkov: So please let me know what you think. Don't forget to subscribe to the newsletter or I sent a summary and in the newsletter, you'll find my. Trip report, quote unquote for the hackathon. There was co-sponsor with together, AI. And Lang chain and Harrison was there and I gave a brief talk as well. And the, sorry, I that a bunch of pictures. [00:03:57] Alex Volkov: So if you're hearing this in your car, check out the newsletter afterwards on Thursday, either. [00:04:02] Alex Volkov: And with that, I give you our first guests as well. Maura. All right, everyone. Welcome back to ThurdsAI. And we have a great guest today. João Moura from I want to say clear a bit. If I'm not mistaken. Or Joao, could you please introduce yourself and what you do and then we're going to talk about the thing we're here to talk about. [00:04:36] Joao Moura: A hundred percent. Thank you for having me. First of all, you got my name right, it's hard to pronounce. I go by Joao, make it easier for everyone. I work at Clearbit, yes, but we just got acquired by HubSpot. I'm not sure. I'm Joao from Clearbit, from HubSpot, and from Crew AI. Everything at once. [00:04:54] Alex Volkov: Awesome. [00:04:58] Alex Volkov: Eye. I think it's your first time here on stage. Welcome. We've met In San Francisco, at the Ollama open source event, and I think like Teknium was there and a bunch of other folks, Ollama, and I met you and we had like a brief conversation, and you mentioned CREW. AI to me, and it sounds like super, super interesting, and then, this week and the previous week, there was like an explosion of interest in CREW. [00:05:17] Alex Volkov: AI, so I would love to hear from you how your like last few weeks have been going, definitely the time that we spent like together since then. A lot of stuff happened to Kurei. Could you just, without saying what Kurei is, could you just, like, recap on your experience for the past two weeks? [00:05:33] Joao Moura: A hundred percent, a hundred percent and first of all, that Oyama event the other day was so good. Had so much, so much fun on it. [00:05:41] Alex Volkov: It was [00:05:41] Joao Moura: last couple of weeks have been intense I gotta tell you, kind of like, the thing. Got, like, blown up out of proportion. Like, I have a lot of DMs, a lot of messages, a lot of issues, and not that many requests, I want to say, but but it has been a lot of fun. [00:05:59] Joao Moura: Kriyai just like, seems to have a lot of interest in from different people. I think this idea of building like AI agents is something that captivate most of the tinkerers out there, like how you can automate your life away. And it seems that have been resonating with a bunch of engineers out there. [00:06:16] Joao Moura: The last couple of weeks has been very intense in terms of Writing code, like at late nights having like to spare a few hours to insert DMs and help with the Discord community. And actually, I actually ended up recruiting my wife to help me with that. So if you see Bianca on Discord or over GitHub issues, that's my wife helped me out, make sure that I get it all covered. [00:06:41] Alex Volkov: Definitely shout out Bianca, thanks for helping. And uh, as well so, now trending on GitHub, I think number one , I think somebody submitted this to Product Hunt as well? [00:06:50] Joao Moura: That was a thing. So I have been working on this and like as an engineer working on an open source project, you don't, you don't think about this project as products necessarily from the get go, but as it starts to get more traction it got the interest of like this one guy that seems to be like a I don't know if it's a big deal or not, but it seems that he hunts a lot of products and product hunt. [00:07:14] Joao Moura: And for some reason he got like. The super fun thing is that I have been part of like, and I have seen other like product, product launches, and I know how much effort goes into preparing those and to be ready for it and have like a, like social media ready to post a lot of content about it. And I had none of that. [00:07:36] Joao Moura: I woke up in the morning and there was a message from a VC saying like, Hey, congratulations on your launch. And I was like, What is this guy talking about? I have no clue. It was very interesting because I, I opened like Product Hunt's website and I'm searching like, how do I cancel this? Like, I, I didn't want to launch this, at least not right now. [00:07:58] Joao Moura: And on Product Hunt's like documentation, they mentioned that you have two options, either, You send them a message like super urgent so that they can pull like the, the brakes on it, or you run with it. [00:08:13] Joao Moura: And at the end of the day, I was like, I'm just going to run with it. I'm going to see how it goes. And turns out we end up the day as [00:08:20] number two. [00:08:20] Joao Moura: And that was, that was something else. Thanks. [00:08:25] Alex Volkov: number one hunter. I think he hunted like most of the products on ProductHunt, so shout out Chris. And definitely, I saw this and what a surprise to wake up to and then get like the product number two. Definitely helped the stats probably. Right. So I think, I think with this excitement, let's talk about like why it's so exciting. [00:08:43] Alex Volkov: Could you give us like a brief primer on Crew AI? We've talked about agents before. We obviously talk about like auto GPT previously and GPT engineer from, from Anton Musica and like a bunch of other very interesting projects. Could you give us the brief primer on like a crew AI, what it is? [00:08:57] Alex Volkov: And then we're going to talk about like why you built it and the orchestration stuff. [00:09:02] Joao Moura: percent Crew I is a very thin framework. It's a Python framework. It's in the process of being converted to TypeScript as well, but it's a Python framework that allows you to build a group of AI agents. You can think about it as if it AutoGem and ChatBath had a child. [00:09:21] Joao Moura: That's the way that I usually describe it. So you're going to have a group of AI agents that are role playing in order to. perform a complex series of tasks. And you can do all sorts of automations on it and you can plug it to all sorts of different systems out there. I think that's the easiest way to describe it right now. [00:09:43] Alex Volkov: Awesome. And could you, you briefly mentioned this, GPT, could you talk about like the, the inspiration here? what made you start this as Clearbit was getting acquired and, or, or around this area, at least I think what made you work on this? There's a bunch of other , orchestration platforms out there, the bunch of agents what made you write your own instead of like taking something off the shelf on open source? [00:10:06] Joao Moura: So turns out that this is a fun story. There was so you're back into my wife again, always propping me up. I love her. She's so great. She was she was telling me, Hey, you have been doing all this amazing work at Clearbit. Because at Clearbit, we have been doing work with LLMs for the past one year. [00:10:22] Joao Moura: And at a scale that I believe not many have. And she was like, You should be sharing more about this. Like, you're leading these efforts and you're doing all these complex systems at scale. And this could definitely help and benefit other people. So she was telling me that I should do a better job at posting online in things like LinkedIn and Twitter. [00:10:41] Joao Moura: And Twitter, I, I think like I'm okay with, but LinkedIn was always hard to me. I feel like there is a, there is a harder threshold, like a higher threshold for how well your idea must be before you post it on LinkedIn. So I was considering like how, how I can do better LinkedIn posting. And because I was so excited about AI agents, I was like, can I build like a couple of agents that will actually help me out with this, where I can like shoveling my, like, like my, my draft and rough ideas. [00:11:11] Joao Moura: And it's going to come up with like some guidance and a better post that I can just edit and post. It turns out that I could and that's, that's how I started QueryAI. I looked into AutoGem and I was not a huge fan on how they, like, one, they didn't have the option to execute tasks sequentially. They also have a lot of assumptions on how this agent should work together. [00:11:34] Joao Moura: And I think The way that they work together should vary depending on the tasks that you're trying to accomplish. I was not a huge fan of it. Chat dev on the other side, I saw a lot of like good stuff on it, but it just didn't feel like a production system, right? Like it has like a game like UI, something that you would experiment with, but not something that you would deploy in production. [00:11:56] Joao Moura: So that's, that's how I came up with this idea of like, maybe I should do something myself so I can build this LinkedIn automation. And if that works, then I can build other sorts of automations. And that's how I started to create AI. I viewed it. Five agents from A social network researcher all the way to a chief content officer to help me create great ideas so that I can post them on LinkedIn. [00:12:23] Joao Moura: And it works great. I went from never posting on LinkedIn to post like three to four times every week. And I love what I post and it seems other people do as well. So from that point on. I decided that I want to create more automations and that's how CREATE. AI came to be. I just abstracted what I learned from that experience into this framework that I could then use to build other sorts of automations and things took off from there. [00:12:50] Alex Volkov: Wow, that's incredible. Incredible story. As a lot of the engineering stories happen when people create like cool things, laziness is somewhere there. Like I want to automate something that I don't want to do, but I definitely need done. I definitely have a bunch of those as well, at least for Thursday. The collection stuff and the other stuff that I would love to just like happen for me. [00:13:10] Alex Volkov: So definitely. Want to check out KuroAI for that and create like a Thursday collection thing. Could you, could you mention like the, like, like technical challenges here? You did mention that it's based on LengChain, if I'm not mistaken. You mentioned that there's not a lot of like, pull requests for people to help out with Could you talk about like the, the technical challenges you ran into? [00:13:30] Joao Moura: Yes so basically when I start to build this out, I realized pretty quickly that Agents are just as useful as how many tools you can connect them with. And when I was looking online, I realized that both YamaIndex and LinkChain already had all these amazing tools that you could, you could run with. [00:13:52] Joao Moura: So I wanted to make sure that I could, people could use those tools too. And build, like, Crews that use them. Because of that, I took the decision to build CREAI around LinkChain. So that if anyone wants to hook that up with their GitHub or their Gmail, there are already tools that were built out for that, and they're pretty easy to plug in and just work. [00:14:15] Joao Moura: And it seems Lemma Index tools also work. I'm putting together some experiments around that to share with more people. But basically that was some of the initial decision that that will lead to this design. I think some of the technical challenges that came from it is It's just realizing that as people start creating all these different curls for these different use cases, there's so many edge cases, right? [00:14:38] Joao Moura: You know that you can try to, like, steer LLMs your way, but especially if you're using, like, open source LLMs and smaller LLMs, they have a harder time just sticking with, like, a given. [00:14:54] Joao Moura: I started to add a bunch of guardrails in Cree AI that actually makes it way better than what you would get with any other agent framework out there, where if it's For example, one of them is if you're running out of iterations, like you're, like your, your agent is stuck on a cycle or taking too long to come up with an answer it's gonna force it to come up with an answer if it goes over a certain number of iterations that you could define. [00:15:21] Joao Moura: Another one is if it tries to use the same two in a row, it's going to prevent it to do that and guide there towards moving on. Another one is it has caching. So every two any agent uses is going to be cached so that if any other agent in the group decides to use the same two they don't need to actually execute it. [00:15:41] Joao Moura: So I think a lot of the challenges come from like how I can add all these guardrails to make sure that Independently of what the use case and what the person is building a group of agents for, that's still going to run smoothly. And that's, that's where a lot of the work has been, has been put, been putting on. [00:16:01] Joao Moura: So you mentioned local modals as well [00:16:04] Alex Volkov: we mentioned, we met in the OLAMA event, and OLAMA is a CLI, a shout out, OLAMA folks, is a CLI to be able to download and run open source models on your hardware, basically. Many of the previous agent attempts, Auto GPT like different ones, they use maybe GPT 4 or something. [00:16:20] Alex Volkov: We're getting to the tools and we heard previously in the space we heard from John Durbin that there are models now that are like better for specific tasks like function calling as well. Jao, could you speak a little bit about the difference that you see? Could Crue AI work with both, right? Open source and also like, API ones. [00:16:39] Alex Volkov: And could you [00:16:40] talk about a little, the difference that you see between like the open source models as we have them right now versus kind of the, the online models and which ones would you prefer for your tasks? [00:16:50] Joao Moura: Turns out that I think that the fact that crew AI supports local models is some like thing that, that. Make it take off because that's something that I wanted from the get go. Like these agents, especially if you're trying to automate complex tasks, they can become rather costly if you want to run them like 24 7. [00:17:09] Joao Moura: But with like the ability to use local models, you can basically just set and forget, and they're going to keep doing work for you. So I wanted to make sure to support local models because of that. Guru AI supports like any of the vendors that you're going to find support in link chain. So you can use any of the open source models out there, a drawback, GPT, you name it. [00:17:30] Joao Moura: And you can also use Zolyama, you can also use LM studio whatever is the best way that you have to run your models locally, you can use that. I. Specifically, like personally, love Olym. Olym is amazing. I love the guys that built it as well. And I think it's so easy to use that I ended up using that. And I have been using some of the smaller models. [00:17:51] Joao Moura: Shout out to Nose Research. I love that OpenARMS 2. 5 model. It's just amazing and so small. Like I can't believe how good it is. And that's one that I use a lot for like when I'm using I'm using OpenARMS 2. 5 just because of how well it works, but I also tried with Mistro, I also tried with Solar, I also tried with Nexus so many models out there, so good. [00:18:19] Joao Moura: One thing that I want to call out as well is that These local models, they definitely struggle a little bit more when compared to GPT 4 in terms of sticking with a given format. I'm also collecting all my executions data so that I can fine tune agentic models. Similar to how you have like instruct models and chat models, I want to make sure that we start to see more agentic models out there. [00:18:46] Joao Moura: I have seen some closed source ones that are not like, You're not able to touch on. So I'm building an open source data set that I can then use to fine tune those models. And then you basically are going to have these agents run on local models without a glitch. That would be at least the end goal. [00:19:05] Joao Moura: That's incredible, incredible specifically because [00:19:08] Alex Volkov: we've, we've had interviews with a bunch of folks who build agentic stuff. So one, one of the more successful episodes of last year for Thursday, I was in an interview with Killian Lucas from Open Interpreter and the open source community here definitely opened the thread with Killian specifically to say, Hey, when the users run a bunch of this stuff, we would love to have. [00:19:27] Alex Volkov: Users opt in maybe for some like telemetry or analytics to be able to build the data sets for the tasks that were completed or not completed. I don't know if you have this plan, but definitely this is a benefit to the community if you do have a way for folks to like, log their stuff. I also mentioned that like, I probably should reach out to you separately to see if like, these runs for these agents in crew could be logged in Weights Biases with the integration. [00:19:50] Alex Volkov: Would be definitely more than happy to like participate and see if we can like look at the execution stuff of your agent on Weights Biases. As well, I think before I let Umesh wanted to have like a bunch of questions for you as well. He's been running and he, he does agents of his own. I want to say [00:20:06] Alex Volkov: what's the next plans for crew? Where are you planning to take this? Many of these projects, suddenly people ask for a UI because maybe they don't want to do like, installing and, and doing like Python stuff. So you already mentioned TypeScript. Could you give us a little bit of a future sense of like, where are you planning to take this? [00:20:23] Joao Moura: think what we are getting now is a bunch of feature requests from most bunch of different sites. So there is some prioritization going on so that I can figure out what to focus next. One thing that seems to be a no brainer to me though is that we need to have a UI for this. [00:20:37] Joao Moura: I think this would be pretty cool and unlock a lot of use cases for people out there. I know there are other people that have been building UIs for their, like their businesses that are being built around this. I, I just think like an open source version would be better. So I'm definitely already working on the UI for this. [00:20:53] Joao Moura: We're going to be able to. Put your agents together, bring your, all your cartoons together, and then you can basically have these agents like run by yourselves. I, I might look into offering an option where you, like, we can even host it for you, and I'm still figuring out what that would look like. [00:21:10] Joao Moura: Maybe that's too far ahead. But but yeah, I think like the UI for it makes a lot of sense. Also another thing is that it seems a lot of the use cases kind of like go back into very similar tools over and over again. And even though you can hook them up with like link chain or lemma index tools, those might still require some like configuration. [00:21:30] Joao Moura: It might not be as straightforward for some people. So we might take an opinionated take. On a tool specific repository and package that you can basically use to bring, let's say, let's say they want to create an agent that does reg you might be able to do that with one line versus having to be like a custom. [00:21:51] Joao Moura: Like two cents for that. So that's another thing that we have been looking at as well. I think there's so many use cases. One thing that I'm trying to do more now is just kind of like chat with more people that are using this. Especially on the business side of things to understand like what other use cases we could support there. [00:22:08] Joao Moura: But yeah, a lot of interesting things cooking. [00:22:11] Alex Volkov: I'm looking forward to hear more about Kuru AI and upcoming things. I think Umesh Arkohos here has been doing Agents for a while and has a few questions as well. Umesh, go ahead. [00:22:23] Umesh Rajiani: Yeah. Hey, Joe thank you for, for coming in. We are almost 80, 80, 90 percent of our workflow now is agentic workflow. So we are employing the generative AI library of. I think that's pretty much it for the introduction of Google for Gemini and also a lot of work using Autogen. [00:22:41] Umesh Rajiani: And we got introduced to Crue AI, I think, four weeks ago through one of my engineers and found it pretty interesting. There are going to be a lot of pull requests coming in from us, actually, because we are thinking about a few things. I just wanted to ask you one particular question about the process part. [00:22:59] Umesh Rajiani: Your current library, as I understand, is is a linear process library and what we have is what we are employing with Autogen is, is also. Bit of a, a graph of actions as well as the dag approach as well. Dag approach, can be implemented using your process. But do you have a, a, a graph of actions, workflow in planning or something that is coming up? [00:23:24] Joao Moura: Yes, so this idea of processes, I want this to be like one of the cornerstones for our career AI. I, my understanding is that a lot, as I said earlier, like a lot of the different outcomes that you're going to get, a lot of the magic happens when you define true what processes these agents are going to work together, right? [00:23:43] Joao Moura: And there are so many options out there. Like you can have them work like sequentially, you can have them work like in a group, like if they're in a meeting, you can have like a consensus strategy where they can kind of like bet to see who is going to take on the task and even evaluate the results. [00:23:59] Joao Moura: So there's just a A lot of different processes that can be implemented there. And the idea is to implement all these processes so that people can have some work happen in parallel if they want to, or sequentially or whatnot. About a graph specific API, I I'm not sure how much I can tell about it, but we have been talking with link chain folks about it. [00:24:19] Joao Moura: And there's, there's some things that have been cooking there. [00:24:23] Umesh Rajiani: Enough said. This last question. So currently it is all Python but most of our implementations now because of the latency and everything and complexity of. The workflows that we are implementing, mostly our applications are enterprise applications. [00:24:36] Umesh Rajiani: We are employing a lot of Rust to, for, for a compiled workflow. So do you have any plans of porting it to Rust or you're looking for kind of a support in that area or something? [00:24:47] Joao Moura: Yeah. So we are, we are porting it to TypeScript right now, and there's some work being done in to build like an API where you might be able to just spin it off as like a service. [00:24:58] Joao Moura: And you can then like [00:25:00] add agents, create agents, outrun API. So you don't have to create one yourself. You just need to figure out how you want to host it. I haven't thought about porting in trust yet, but I would be open to that idea. For sure. If I can get enough people to help out, I create a repository and we can get things working for sure. [00:25:16] Umesh Rajiani: I'll, I'll reach out to you separately. Thanks Alex for, for allowing me to ask questions. Of course I have many questions, but I'll reach him out on his Discord. [00:25:23] Alex Volkov: Yeah, thank you Umesh, and João, I just want to like recap on the awesome success of Kuru AI. I agree with you. I think the fact that, like, we've had many frameworks like this, we've talked about many frameworks like this, the ability to run this completely on your machine, the ability to, like, not pay for somebody else the ability to like, use Olama. [00:25:43] Alex Volkov: I didn't know that you also support LM Studio. Shout out LM Studio, a friend of the Pada, hopefully we're, we're going to get on, on the next Thursday, I so I didn't know that I can, like, open up a local model on LM Studio and, and then the crew would use this API. Definitely. Definitely want to play with this now. [00:26:00] Alex Volkov: I want to say, I want to give you a few minutes to just like talk to the community. A lot of things are happening in this world. I find it very interesting where kind of the AI engineers, the kind of the traditional software engineer background folks, they're building the tools, they're building the rag systems, let's say they use the link chain. [00:26:17] Alex Volkov: From the other side, we have a bunch of machine learning folks who are Building the models, fine tuning the models, and working on that space, and reading the papers. And I do see a connection between, and obviously my role in Ways and Biases specifically is to connect these two worlds. I do want to see more people that train models also kind of like think about the agentic behaviors as well. [00:26:37] Alex Volkov: We heard John Durbin before talk about like, hey, there's specific data sets for RAG, there's specific data sets for execution and function. I think Eroboros has The, the data set has a bunch of like function calling as well. So definitely I want to see a connection here. João, please feel free to talk to the community in terms of like what you need to make crew the best crew ever. [00:26:57] Alex Volkov: Where can they find you, what you can get help with the floor is yours. Feel free to take over and ask everything. Community will provide. [00:27:06] Joao Moura: A hundred percent. And just to tap into what you said there, I agree. I think like there's something magical that happened like last year with like GPT taking the world by the storm is that it like it connected two groups of engineers that in the past didn't talk very much. [00:27:22] Joao Moura: And that was like AI and ML engineers with. regular software engineers. I have managed teams in both areas in the past, and I definitely have seen like that there isn't much interaction there, but right now it's, it's amazing to see all the amazing stuff that have been coming up from like those two groups to interacting more together. [00:27:40] Joao Moura: It has been a lot of fun. About, about CREATE. AI. Yes, I would say give me a follow on Twitter or X, I would say now, so give me a follow on X and I definitely will keep posting and share more about CRE AI and all the things related to LLMs, Agents, and everything else. You can know more about CRE AI by looking into its GitHub. [00:28:00] Joao Moura: So you can go into my profile slash Guru AI. I probably add the link to my ex account as well. From that, if you have follow up questions or if you want to like see what people have been cooking with it, I would say join the Discord community. We have around 500 people there and has been growing daily. [00:28:18] Joao Moura: So if you join that, you might be able to see other use cases and things like that. If you're curious about it, but you're just like, you're, you're not sure what you could build with it there's a bunch of examples in the README and even some videos that I recorded crews doing like, stock analysis or tree planners and all that. [00:28:38] Joao Moura: There is there's a lot of content there that you can consume in order to get your ideas. And if you do decide to give it a try, don't miss out on the custom GPT. It's also linked in the README and it can help you write the code. It can help you with ideas for the agents, ideas for the roles or for the tasks or anything around using QrooAI. [00:28:58] Joao Moura: If you're also curious at contributing to the project. GitHub has a bunch of issues. My wife, again, has been flagging and tagging all of them. So thank you so [00:29:07] Joao Moura: much. [00:29:07] Alex Volkov: out, Bianca. [00:29:08] Joao Moura: can find like all the ones that are tagged with help wanted or the ones that are related with questions And you can help answer them as well And we're gonna be writing new documentation from the scratch So this might be a great opportunity to help with like more simpler stuff as well if you're into that [00:29:24] Alex Volkov: Awesome and I think I saw something, I don't know if I have a link [00:29:28] Alex Volkov: to, to the generous documentation on the fly from, from just the, the code itself. And it looks super cool. I'll, I'll try to send this to you. Joao, thank you so much for joining Thursday. I, this is your first time here. Hopefully not the last. [00:29:40] Alex Volkov: Congrats on the success of Kru AI and it's been great meeting you and then having you on definitely thank you for coming and folks should definitely check out Kru AI, give Joao a follow and we will expect more. I can't wait to like run a few Kru myself to help me with Thursday night tasks, especially on local, local models. [00:29:58] Alex Volkov: It was super cool. Thank you for coming, man. [00:30:01] Joao Moura: I love it. Thank you so much for having me catch you folks online. [00:30:04] Alex Volkov: Awesome, and your audio quality was great by the way, thanks for testing out your mic. [00:30:07] [00:30:11] Bagel models the leaderboard from Jon Durbin [00:30:11] Alex Volkov: We're moving forward into the top open source on the LLM leaderboard and the creator. So if you guys open the open source LLM leaderboard, which we often talk about. On HuggingFace we, we've talked about kind of the, the difference between human evaluation and the automatic evaluations that OpenLLM leaderboard runs. [00:30:32] Alex Volkov: You will see a bunch of models. The top three ones are from CloudU and they're, they're like, I think merges of Yee34 and then the Mixtroll34b as well, but it's not based on Mixtroll. And then the rest of the is like a bunch of John Durbin Bagel examples. And, so all of those, there's like six models there that are based basically on the John's Bagel DPO versions. [00:31:00] Alex Volkov: And I just wanted to shout this out and shout out Durbin for, for working this hard and releasing these models. [00:31:06] Alex Volkov: Let's see if we can hear from the man himself. Hey, John. [00:31:09] Jon Durbin: Hey, how's it going? [00:31:10] Alex Volkov: Good. Thanks for joining us. I don't remember if you've ever been on stage. So feel free to briefly introduce yourself to the audience who doesn't know you. And definitely they should and they should follow you as well. [00:31:22] Jon Durbin: Yeah, I'm a software engineer. I'm an AI tinker. I've been doing synthetic stuff since I guess maybe April with Aragoros project. It's been tons of fun. Lately I've been mostly working on the bagel models. If you're wondering what the bagel name came from, it's from Everything, Everywhere, All at Once. [00:31:37] Jon Durbin: Great movie. Yeah, so that, that's the kind of the premise of the model is like all the prompt formats. Yeah. All the data sources, all the training techniques, there's Neptune, there's DPO yeah, just fun stuff there. As far as the leaderboard, that wasn't really my goal. If you look at the actual, like, token count per data set, I think the highest And then the last amount of tokens is actually probably the Cinematica dataset, which is movie scripts converted to roleplay format. [00:32:07] Jon Durbin: So it's, it's interesting that it does so well, but really I was targeting the model for general purpose as a merge base because I know that, MergeKit is so popular now. So I was trying to come up with a base model that has a little bit of everything and every prompt format so that anyone who wants to do this, alchemy with MergeKit. [00:32:28] Jon Durbin: Can use the Bagel series as a base, because I should, if you have an alpaca based model and a vicuña based model, they're not going to merge very well. It'll have, weird stray user tokens or whatever. The idea with Bagel is to be a good base. [00:32:42] Alex Volkov: I also saw quite a lot of work you're doing on new DPO data sets. Could you talk about those? [00:32:48] Jon Durbin: And then, yeah, I keep cranking out new DPO datasets to enhance the stuff that's lacking right now. [00:32:54] Jon Durbin: I think even the YI 34B. Might be a little bit overcooked. I used QLORA for both the supervised fine tuning stage and DPO. And it turns out DPO, you really need to use an incredibly low learning rate. I was even using, like, maybe 50x smaller learning rate for the DPO phase than the Then the supervised fine tuning phase, and even then [00:33:20] I stopped the run about halfway through and killed it because the eval started spiking all over the place. [00:33:26] Jon Durbin: Yeah, still, still lots of stuff to learn and I'd love to do a full weight fine tune of the E34B. I'm probably going to work on a Solar 10. 7B version of it next and maybe a DeepSeq 67B. I'm curious if the DeepSeq's, deeper network is actually going to improve things in any sort of way. But [00:33:46] Alex Volkov: awesome. John, thank you so much for joining and thank you so much for the deep dive. So I have two questions for you real quick. I did not expect you to join. So this is not a full blown interview, but I'm very happy that I have you. First of all, you mentioned that there's like two versions, DPO and non DPO, of Bagel. [00:34:01] Alex Volkov: And you mentioned the differences between them. You said like DPO version is more factual and truthful, but not great for RP. I wasn't sure what RP is. Roleplay? [00:34:10] Jon Durbin: Roleplay, [00:34:11] Alex Volkov: Yeah. And then creative writing. Could you give us like a little bit of a, of a sense of like, what's like DPO versus non DPO version? Is that just dataset based or is there something more going on behind the scenes that like makes the one model behave differently than the other? [00:34:27] Jon Durbin: Yeah, so really all of the Bagel series, you basically have two phases of training. There's the super, regular supervised, fine tuning stage where I just, you can look at the Bagel repository. Everything is completely open source and reproducible. But in the supervised fine tuning phase it's just a ton of data sets and and then I take that fine tuned model, fine tuned model, and then I apply DPO, direct preference optimization to it. [00:34:52] Jon Durbin: And I have quite a few DPO datasets in there, but really, the DPO landscape is sparse right now. You basically have DPO datasets from NVIDIA, the Helpsteer database, which is a human annotated one where they ran a bunch of gen a bunch of prompts against LLMs and then had humans rank them. [00:35:14] Jon Durbin: Then there's like the LIMSYS, 1, 000, 000, where you can find the exact same prompt sent to multiple models. And so you can take like the GPT 4 answers. Use that as the preferred answer, and then the Kunyu 33 or something as the rejected answer, because you're assuming the GPT 401 is better. [00:35:31] Jon Durbin: Same with there's Orca DPO pairs. I know Argya just did a new release of that, which is better. But we don't have a ton of DPO datasets that are specifically for creative writing tasks and stuff. I made one which is actually based on the Eroboros 2. 2 compared to the Eroboros 3 series where I actually rewrote most of the creative writing prompts with a different prompt and some other stuff. [00:35:59] Jon Durbin: I actually used the March version of GPT 4 which is better. So in that case you get Basically like three to four times the number of tokens in the output. So there's that DPO data set, which I make myself in the Bagel Code. But otherwise there's really no role play focused data in any of the DPO data sets. [00:36:21] Jon Durbin: So what happens is you take that supervised or, fine tuned model from the first phase. And you apply DPO to it, and it kind of experiences, forgetting of what it learned during the fine tuning of some of the stuff like creative writing and role play. Yeah same with code. So if you look at, my Twitter feed, you can see that I've released there's a Python DPO dataset that'll hopefully fix some of that stuff. [00:36:44] Jon Durbin: I just released another contextual question answering DPO dataset for better RAG performance after the DPO phase. I put out just a few minutes ago Gutenberg DPO, which is basically I parse maybe 14 or 15 books from Project Gutenberg that are public domain into chapters and then create prompts to actually write those chapters and then I create summaries so you have like the previous chapter summary inside the prompt and then I use that to prompt one of the local LLMs so I used Dolphin, eChat, and Lama 213b. To get the rejected values the outputs from these models are fine in some cases, but they're short and they, you'll notice with the LLM, like most of the LLMs, when you write a story, it's always a happy ending and it, and it ends with like, and they walked into the forest lived happily ever after. [00:37:37] Jon Durbin: It's boring and cliche. My hope with the Gutenberg stuff is that when you actually prompt it to write a chapter of a book, it's gonna be, from human writing that are popular books. They're a little bit old timey because they have to be to be public domain, but, [00:37:52] Alex Volkov: Yeah. [00:37:53] Jon Durbin: hopefully it will improve the writing and create creativity of the late whatever bagel models I do in the future with So I'm trying to kind of improve, improve that, but still a lot of stuff I need to do. I think the next thing I'll do before I actually make another bagel model is use something like the Goliath 120B to make a role play centric dataset for DPO. That way it doesn't completely forget how to do that as well. [00:38:15] Alex Volkov: Awesome. And I'm just looking at the number of data sets that, like you said, everything, everywhere, all at once. And this is why it's called Bagel, Everything Bagel. It's just like an insane amount of data sets. I'm just gonna run real quick. AI2, Arc, Error Bores Belly Belly, Blue Moon. [00:38:30] Alex Volkov: You have Capybara in there, Cinematica. Imo Bang, Gutenberg, LMsys chat, like, like tons, tons of stuff. It's incredible how well the model performs. John, one thing that I wanted to follow up on before we move on. You mentioned something that's better for RAG as well. You mentioned a DPO data set that's better for RAG. [00:38:45] Alex Volkov: Is that the contextual DPO that you released? [00:38:49] Jon Durbin: Yep. [00:38:50] Alex Volkov: What, what makes it better for, for RAG purposes? Could you, could you like maybe give two sentences about this? [00:38:56] Jon Durbin: And this is actually something you can reproduce with the AeroBoros tool as well if you wanted to generate your own data, but I have this instructor in there called Counterfactual Contextual, and what that does is it makes a bunch of fake facts, like it'll say, the Battle of Midway happened in the Civil War, something like that and it'll put that into context and then ask a question about it. [00:39:19] Jon Durbin: And then it'll have the real version of the fact as well, World War II, Battle of Midway and then the idea is that you want to train the model to always attend to the context and not try to base the answers on what it knows from the base pre training. For example, if you're doing I don't know, like a virtual, you have a different planet where the sky is purple. [00:39:41] Jon Durbin: And you ask the model, what color is sky, is the sky based on your lore book or whatever. You want to make sure that the model always obeys your context and, and answers accordingly, and not says the sky is blue, because I know the sky is blue. So the, the data set that I put in there has a bunch of those kinds of things. [00:39:59] Jon Durbin: You can't just put in the fake facts, because then the model will just You know, learn to answer incorrectly. So for every, for every fake version of the context, you have to put in a real version of the context as well. The other thing that makes it better for RAG is I actually stuff more than one piece of context into it because Like with RAG, the retrieval accuracy is the hardest part, so you want to retrieve more than one document. [00:40:23] Jon Durbin: So suppose you want to retrieve ten documents. If you want to stuff all ten of those into a single prompt and then you want to provide references to the user, you have to know which segment of the prompt it came from. This data set also includes, like, you can put metadata into the prompt for each section that you retrieve, and then when you ask for references in the output, it'll actually only reference that segment. [00:40:47] Jon Durbin: A bunch of stuff like that, yeah, I, I put in irrelevant context as well to make, try to confuse them all because retrieval is very noisy. All of that kind of stuff is in there. [00:40:57] Alex Volkov: First of all, I think from the whole community, thank you a lot for everything that you do and your work. And I really appreciate your time here on Thursday. You're more than welcome to always join us. And I didn't expect you to be here when I talked about. [00:41:09] Alex Volkov: The stuff that you just released, but it's really, really awesome when people from the community who work on the stuff that they do also come and have a chance to speak about them. So John, you're always welcome on Thursday. I would love to invite you again and talk deeper. [00:41:20] Alex Volkov: And as you release the next stuff that you're working on, I know you're working on a bunch of next stuff more than welcome to come here and, and, and discuss, or even like DM me before. So we'll know what to chat about. I will. Definitely mentioned the, the DPO datasets in the fine tuning hackathon that I'm going to this week. [00:41:35] Alex Volkov: And so thank you for that. That, that was why I wanted to do a little bit of a deep dive. [00:41:40] And also I want to shout out you as the, one of the most active users of Weights Biases. You posted your like recap that we sent and you have two reports there. And you're part of like the top 10 percent of most active users with 2, 500. [00:41:53] Alex Volkov: Hours trained in 23 and like 900 plus models. So that's, that's incredible. I just wanted to shout this out. [00:42:02] Jon Durbin: Yeah, I'm a little addicted. [00:42:03] Alex Volkov: Yeah, it's amazing. It's amazing. And I, I appreciate everything that you do and I think the community as well This is a public episode. If you'd like to discuss this with other subscribers or get access to bonus episodes, visit sub.thursdai.news/subscribe | |||
19 Jan 2024 | 📅 ThursdAI Jan 18 - Nous Mixtral, Deepmind AlphaGeometry, LMSys SGLang, Rabbit R1 + Perplexity, LLama 3 is training & more AI news this week | 01:10:40 | |
👋 Hey there, been quite a week, started slow and whoah, the last two days were jam-packed with news, I was able to barely keep up! But thankfully, the motto of ThursdAI is, we stay up to date so you don’t have to! We had a milestone, 1.1K listeners tuned into the live show recording, it’s quite the number, and I’m humbled to present the conversation and updates to that many people, if you’re reading this but never joined live, welcome! We’re going live every week on ThursdAI, 8:30AM pacific time. TL;DR of all topics covered: * Open Source LLMs * Nous Hermes Mixtral finetune (X, HF DPO version, HF SFT version) * NeuralBeagle14-7B - From Maxime Labonne (X, HF,) * It's the best-performing 7B parameter model on the Open LLM Leaderboard (when released, now 4th) * We had a full conversation with Maxime about merging that will release as a standalone episode on Sunday! * LMsys - SGLang - a 5x performance on inference (X, Blog, Github) * NeuralMagic applying #sparceGPT to famous models to compress them with 50% sparsity (X, Paper) * Big CO LLMs + APIs * 🔥 Google Deepmind solves geometry at Olympiad level with 100M synthetic data (Announcement, Blog) * Meta announces Llama3 is training, will have 350,000 H100 GPUs (X) * Open AI releases guidelines for upcoming elections and removes restrictions for war use (Blog) * Sam Altman (in Davos) doesn't think that AGI will change things as much as people think (X) * Samsung S24 has AI everywhere, including real time translation of calls (X) * Voice & Audio * Meta releases MAGNet (X, HF) * AI Art & Diffusion & 3D * Stable diffusion runs 100% in the browser with WebGPU, Diffusers.js (X thread) * DeciAI - Deci Diffusion - A text-to-image 732M-parameter model that’s 2.6x faster and 61% cheaper than Stable Diffusion 1.5 with on-par image quality * Tools & Hardware * Rabbit R1 announces a deal with Perplexity, giving a full year of perplexity pro to Rabbit R1 users and will be the default search engine on Rabbit (link) Open Source LLMs Nous Research releases their first Mixtral Finetune, in 2 versions DPO and SFT (X, DPO HF) This is the first Mixtral finetune from Teknium1 and Nous team, trained on the Hermes dataset and comes in two variants, the SFT and SFT+DPO versions, and is a really really capable model, they call it their flagship! This is the fist Mixtral finetune to beat Mixtral instruct, and is potentially the best open source model available right now! 👏 Already available at places like Together endpoints, GGUF versions by the Bloke and I’ve been running this model on my mac for the past few days. Quite remarkable considering where we are in only January and this is the best open chat model available for us. Make sure you use ample system prompting for it, as it was trained with system prompts in mind. LMsys new inference 5x with SGLang & RadixAttention (Blog) LMSys introduced SGLang, a new interface and runtime for improving the efficiency of large language model (LLM) inference. It claims to provide up to 5x faster inference speeds compared to existing systems like Guidance and vLLM. SGLang was designed to better support complex LLM programs through features like control flow, prompting techniques, and external interaction. It co-designs the frontend language and backend runtime. - On the backend, it proposes a new technique called RadixAttention to automatically handle various patterns of key-value cache reuse, improving performance. - Early users like LLaVa reported SGLang providing significantly faster inference speeds in their applications compared to other options. The LMSys team released code on GitHub for others to try it out. Big CO LLMs + APIs Meta AI announcements (link) These #BreakingNews came during our space, Mark Zuckerberg posted a video on Instagram saying that Llama3 is currently training, and will be open sourced! He also said that Meta will have 350K (that’s not a typo, 350,000) H100 GPUs by end of the year, and a total of ~600,000 H100 equivalent compute power (including other GPUs) which is… 🤯 (and this is the reason why I had to give him double GPU rich hats) Deepmind releases AlphaGeometry (blog) Solving geometry at the Olympiad gold-medalist level with 100M synthetic examples AlphaGeometry is an AI system developed by Google DeepMind that can solve complex geometry problems on par with human Olympiad gold medalists It uses a "neuro-symbolic" approach, combining a neural language model with a symbolic deduction engine to leverage the strengths of both The language model suggests useful geometric constructs to add to diagrams, guiding the deduction engine towards solutions It was trained on over 100 million synthetic geometry examples generated from 1 billion random diagrams On a benchmark of 30 official Olympiad problems, it solved 25 within time limits, similar to the average human medalist OpenAI releases guidelines for upcoming elections. (Blog) - OpenAI is taking steps to prevent their AI tools like DALL-E and ChatGPT from being abused or used to spread misinformation around elections - They are refining usage policies for ChatGPT and enforcing limits on political campaigning, impersonating candidates, and discouraging voting - OpenAI is working on technology to detect if images were generated by DALL-E and labeling AI-generated content for more transparency - They are partnering with organizations in the US and other countries to provide users with authoritative voting information through ChatGPT - OpenAI's goal is to balance the benefits of their AI while mitigating risks around election integrity and democratic processes Microsoft announces copilot PRO Microsoft announced new options for accessing Copilot, including Copilot Pro, a $20/month premium subscription that provides access to the latest AI models and enhanced image creation. Copilot for Microsoft 365 is now generally available for small businesses with no user minimum, and available for additional business plans. This weeks Buzz (What I learned with WandB this week) Did you know that ThursdAI is not the FIRST podcast at Weights & Biases? (Shocking, I know!) Lukas, our CEO, has been a long time host of the Gradient Dissent pod, and this week, we had two of the more prolific AI investors on as guests, Elad Gil and Sarah Guo. It’s definitely worth a listen, it’s more of a standard 1:1 or sometimes 1:2 interview, so after you finish with ThursdAI, and seeking for more of a deep dive, definitely recommended to extend your knowledge. AI Art & Diffusion Zero shot face adapted image gen - 3 different tech approaches What used to take ages, now takes seconds with 0 shot, there are quite a few approaches to generate images with real human faces, in 0 shot capacity, providing just a few faces. Gradio folks call it Zero-shot face-adapted image generation and there are 3 tools to generate those: 1⃣IPAdapter 2⃣PhotoMaker 3⃣InstantID Here’s a great summary thread from Gradio folks for this fast advancing field! Remember we had to finetune on faces for a long time? Dreambooth and then LORAs, and now we have this exciting development. Tools & Hardware Rabbit R1 partners with Perplexity The R1 device that was just announced, is about to sell through it’s first 50K in just a few days, which is remarkable. I definitely pre-ordered one, and can’t wait to get my hands on it. Jesse the founder has been all over X, getting incredible recognition, and after a few conversations with Aravind Srinivas, they agreed to make a deal right on X. Today they hopped on a space and announced that all the first 100K early buyers of Rabbit are going to get a full year PRO subscription of Perplexity (one of the best AI search engines out there) for free! I sure as heck didn’t expect it, but the email was sent just a few minutes after the X space, and now guess who uses perplexity pro? Here’s an example of a perplexity searching ThursdAI content (it doesn’t always get it right tho)! I guess that’s it for today, as I’m writing this, there are incredible other stuff getting released, Codium open sourced AlphaCodium (here’s a link to the founder talking about it) but I didn’t have a second to dive into this, hopefully will bring Imatar to ThursdAI next time and chat about it! Have a great weekend all 🫡 (please give us a good review on Apple Itunes, apparently it really helps discovery!) Full Transcription for convenience: [00:00:02] Alex Volkov: Hey everyone, happy Thursday. My name is Alex Volkov. I'm an AI evangelist with Weights Biases, and this is Thursday AI. [00:00:13] Alex Volkov: We had such a great show today, over 1100 of you tuned in to the live recording, which is incredible. [00:00:30] I also wanted to say that if you're not subscribed to thursdai.news newsletter, please go ahead and do because I send a full blog with the links to the show notes and to the speakers that we have on stage, and you should be able to follow up. [00:00:46] Alex Volkov: There's a bunch of multimedia, like videos, that are not coming through in the audio only podcast format. So please subscribe to ThursdayEye. News as well. This live recording, we also hosted Maxime Lebon, who's a senior machine learning scientist with J. [00:01:04] Alex Volkov: P. Morgan, and the author of several models, and Merged models, lately the Neural Beagle model that we've talked about. We had a great conversation with Maxime. And that full episode will be posted as a Sunday special evergreen content episode. So please stay tuned for that. [00:01:29] Alex Volkov: It's been an incredibly illuminating conversation in the world of merging and merge kit and everything else that Maxim does and it was a super cool conversation. So that's coming soon. [00:01:41] Alex Volkov: And, as I've been doing recently, the following is going to be a 7 minute segment, from the end of the live recording, summarizing everything we've talked about. [00:01:54] Alex Volkov: I hope you've been enjoying these TLDR intros. Please let me know in the comments if this is something that's helpful to you. [00:02:05] ThursdAI Jan18 TL;DR recap by Alex [00:02:05] Alex Volkov: Alright we started with talking today, Thursday I, January 18th. We was talking about n News imis, the Mixt mixture fine tune that came out from Teo and the folks at News. It, it was of the first fine noon of mixture, the mixture of experts model from a mistral that came from the news research folks. [00:02:35] Alex Volkov: And it released in two versions, the DPO only version SFT plus DPO version. Given different data sets they was trained on and actually different capabilities. It looks based on the community, the DPO version is like very well performing. I've been running this on my Macbook with LM studio and it really performs well. [00:02:53] Alex Volkov: So shout out and folks should try this. This is By far the best, looks like the best new Hermes model based on just benchmarks. They're trained on the best open source model that's currently Mixtro. Mixtro is number 7th in the world based on LMCS Arena, and that's an open source model that we all get to use. [00:03:10] Alex Volkov: Then we've covered the Neural Beagle 14. 7b from Maxim Le Bon. Maxim also joined us for a full interview that you can hear as part of the a podcast episode and Maxim released a Neural Beagle, which is a merge plus a DPO fine tune. And it's one of the top performing 7 billion parameters on the OpenLM leaderboard. [00:03:30] Alex Volkov: When released in a few days ago, now it's fourth. So the speed with which things change is quite incredible. We then covered the LMSYS. SGLang attempt is a 5x performance inference bunch of techniques together on the front end and the back end called Radix attention on the back end and the SGLang way to run through inference code on the front end that combines into almost a 5x performance on inference. [00:03:56] Alex Volkov: 5x is incredible Nistan mentioned that it does less than 5x on like longer sequences and then we had a conversation about Where it could improve significantly, which is agents and agents are sending short sequences. Alignment Labs told us that this could be significant improvement in that area. [00:04:13] Alex Volkov: So our agents are about to run way faster. A 5x improvement is just incredible. And we also mentioned that at the same day when this was released, another Optimization was shouted out by Tim Ditmers from the Qlora fame called Marlin that also improves by 4x some significant inference techniques. [00:04:34] Alex Volkov: And I wonder if those can be compiled together in some way. Quite impressive. We also covered neural magic doing spars, pacification and sparse. And we did in a deep dive into a short, deep dive. Thank you. Alignment and thank you Austin for what's spars, pacification means. And they do in this for like major models and they compress them with specification to around 50% sparsity. [00:04:55] Alex Volkov: It's zeroing. Out the weights that you don't actually use. And it makes the models like significantly smaller. We covered Desilang a little bit. We didn't actually get to the diffusion. I'll just read out those updates as well. Then we covered the OpenAI had new guidelines for upcoming elections, and they're trying to add techniques for folks to identify daily generated images. [00:05:18] Alex Volkov: And they're adding, restrictions to how their LLMs are used in the context of voter suppression, etc. We then talked about DeepMind and AlphaGeometry, where DeepMind released And open sourced looks like a model called Alpha Geometry that uses neuro symbolic approach with two models that solves geometry at almost a gold medal at the Olympiad level. [00:05:42] Alex Volkov: So Geometry Olympiads and quite impressive this release from from DeepMind and shout out. It was trained on a hundred million synthetic data set sources. A source from like more than one billion. Or so random examples and it's quite impressive. So shout out DeepMind as well. We also briefly mentioned Samsung that has a Samsung S24, the flagship phone that now Apple is needed to compete with, that has AI everywhere, uses the new Qualcomm chip and has AI in. [00:06:10] Alex Volkov: Pretty much summarization everywhere. There's like a button with the sparkles with AI. And one cool thing that we haven't mentioned, but I saw MKBHD on Twitter review is that they added real time translation of calls. So you can literally call some people with a different language and on device translation, after you download the model on device, we'll actually be able to translate this in real time. [00:06:30] Alex Volkov: So you can read what the other person said in different language, but also hear it. And that's like quite cool. Then we had a deep interview with Maxim Lebon, the author of many things. Recently, we've talked about Fixtral or Fixtral, the mixture of experts of the five models. We've talked about merges. [00:06:46] Alex Volkov: Maxim had a great explanation on, on, on his blog. And then on the Hug Face blog about what merges, what MergeKit does and how that. Plays into the whole ecosystem, the top LLM leaderboard now has been taken over by merges, specifically, likely because merging models does not require additional computer, additional training, and that's fairly easy to do with just the code merges takes and combines. [00:07:11] Alex Volkov: With different, using different algorithms like SLURP and other algorithms it combines different models and different weights from different models, including potentially building models of novel sizes. So we've seen 10 billion parameter models, like 120 billion parameters so you can use those techniques to Combine models or merge models into different ways. [00:07:31] Alex Volkov: There's also Frankenmerge that uses different models to combine into one. So we dove into that and what the inspiration for merging and what it actually does. Maxim also released like Lazy Merge Kit, which is a thin wrapper on top of the merge kit from Charles Goddard. So shout out to Charles. [00:07:47] Alex Volkov: So we had a very interesting interview about merging and thank you, Maxim, for joining us. Definitely worth a listen as well. And then we had breaking news from BigZuck and the meta team that talked about he gave an update about the number of GPUs that they have. And by the end of this year, they're talking about 350, and overall 600, 000 H100s or equivalents of compute which they're going to use for AI and Metaverse. [00:08:14] Alex Volkov: And Definitely a great update. They're training Lama 3 right now. The stuff that we didn't get to, but I wanted [00:08:20] to update, there's a, and I will add in show notes. There's a stable diffusion code that runs 100 percent in the browser with WebGPU and Diffusers. js, a thread from ClipDrop, the CEO Cyril Diagne. [00:08:32] Alex Volkov: And there's also, we've talked about DeciEye, the company that releases a bunch of models. They release DeciDiffusion, a text to image model with only 370, the 300. Sorry, 732 million parameters. It's twice as fast and 61 percent cheaper than Stable Diffusion with the same image quality, so that's getting improved. [00:08:51] Alex Volkov: But I think they're talking about Stable Diffusion 1. 4, so not SDXL or the new one. And Desi, I also released Desi Coder, and we also covered the Stable Diffusion Coder that is a coding model that runs closer on device, a 3 billion parameter model that beats Code Llama 7b. I think that's most of the stuff we talked about. [00:09:09] Alex Volkov: And then one of the major things that Umesh brought we've talked about corporate drama, maybe a new segment in Thursday Eye where Microsoft, Did some things that actually disrupted workflows and companies actual products built on top of Microsoft, which is considerably not great and led to a fight. [00:09:30] Alex Volkov: Hopefully not, but potentially a legal battle as well, and that's not something that should be done by a cloud provider such as Microsoft. Very ugly. In addition to this, we also talked about Microsoft announcing the CoPilot Pro that's now open for small businesses for 20 bucks a month with no minimum seats as well. [00:09:46] Alex Volkov: And I think that's most of the things that we've mentioned [00:09:49] Alex Volkov: Let's go. [00:09:51] Sounds: to all of you. [00:09:57] Alex Volkov: from, I guess [00:09:59] Sounds: all of you. Namaskaram to [00:10:07] Alex Volkov: 2024, we all need to get used to say 2024 at this point we have a bunch of AI news. My name is Alex Volkov, I'm an AI evangelist with Weights Biases, and I'm joined on stage here with dear friends, co hosts of Thursday AI. Podcast, newsletter, live X recording, community, I don't know, a bunch of other stuff as well. [00:10:29] Alex Volkov: Nishten does paper readings, is a semi part of this as well. Welcome everyone. Welcome. [00:10:33] Introduction to the Session's Structure [00:10:33] Alex Volkov: I will just say a few things before we get started. So first of all, for those of you who are new, who are listening to this for the first time first of all, welcome. [00:10:41] Alex Volkov: It's great that you have found us. Please DM me with like how you found us. I would love to know as I'm looking into the channels, et cetera. However, I will say that we've been here every week, pretty much at the same time. I don't think we've changed time since the summer. So 8. [00:10:55] Alex Volkov: 30 AM Pacific and we try to do this every Thursday. I think we missed one or two. I was sick once, apologies. But other than that, we're here to talk about the AI every week. And what happens often is as we as we talk about things, different breaking news happened and folks announced different stuff on Thursday., and we cover pretty much everything. A very broad spectrum in AI changes. So I know there's like spaces to talk about diffusion, specifically art spaces as well. So we cover diffusion to an extent, but we try to focus on I guess our main focus is open source LLMs. We love those. We have a bunch of folks here on stage. They're training and fine tuning the greatest kind of open source models and definitely follow up on the different how should I say, different techniques, like the merging stuff that we're going to talk to at length later, and we, we hopefully get to hear about them first before they take over hug and face which was the case, I think with some of the models and some of the techniques. [00:11:54] Alex Volkov: And I see two more folks joining us as well from different areas of the open source community. So I will say welcome LDJ and welcome alignment, LDJ. You've been missing in action. I was just saying, how are you, man? Welcome back. [00:12:08] Luigi Daniele: Yeah, I'm doing good. Glad to be [00:12:10] Alex Volkov: Yeah. And also we have Austin AKA Alignment Lab. What's up Austin? [00:12:16] Alignment Lab: Oh, dude, I'm doing great. I was actually just in a call with LDJ and he was like, oh, Thursday Eye is starting and I was like, let's go. [00:12:22] Alex Volkov: Yeah that's exactly what I like to hear that the calendar events is popping off and Thursday is starting. [00:12:27] Open Source AI: Nous Hermes Mixtral Finetune + DPO deep dive [00:12:27] Alex Volkov: So with that, I think it's time for the open source stuff. [00:12:44] Sounds: Open Source AI, let's get it started. [00:12:48] Alex Volkov: All right, so welcome to probably the biggest, the most fun, the most Contentful section of Thursday ai, where we talk about open source, LLMs and lms. I guess we should also start mentioning because a bunch of these models that we see are also multimodal, and I guess we'll start with. [00:13:08] Alex Volkov: , News Hermes Fine Tune on Mixtral we've been waiting for this, Mixtral was released I want to say a month or so ago, a month and a half ago, and now we're getting one of the top kind of data sets and fine tunes trained on Mixtral, and we're getting this in multiple formats. [00:13:25] Alex Volkov: Again, shout out Technium. If you guys don't follow Technium yet what are you even doing showing up on Thursday? I definitely give Technium a follow. But Mixtral fine tune is available and it comes in two variants and SFT and then DPO and SFT only. So SFT is a supervised fine tuning and DPO, direct preference optimization. [00:13:45] Alex Volkov: This is like a, not a new technique, but definitely has been around for a while. Many people are using DPOs at this point. We've talked about DPO multiple times. I think we also saw, Nistan, correct me if I'm wrong, the actual mixtural instruct is also DPO, right? We saw this in the paper. [00:14:00] Alex Volkov: So DPO is everywhere. And this is not the first time that the SFT and DPO pair is getting released separately. I think we've chatted with John Durbin who's, shoutout John, is in the audience. And that conversation is on the feed. So definitely check out the conversation with John. [00:14:16] Alex Volkov: And the Bagel models were also released separately with SFT and the DPO version as well. And I think John back then mentioned that each one has Different different things it's good at. And I also would love to figure out which one of the new, Neus Ermis Mixtural Fine Tunes is best at what. [00:14:33] Alex Volkov: Technium has a bunch of stuff in in, in the thread, so I'll link this below for examples. And I will say that the comparisons to Mixed Real Instruct. Technium posted a bunch of comparisons to Mixed Real Instruct. And it's interesting that not all of the benchmarks look like on improvements. [00:14:51] Alex Volkov: There's a few, I think on GPT4ALL and HelloSwag. The base model, at least the non DPO base model, still wins just by a little bit. But everything else, like ARX, AGI, EVAL, and MMLU are significant improvements. And we're gonna probably continue to see those improvements. Shoutout. If you have tried it, please let me know. [00:15:08] Alex Volkov: I will say this last thing, that finally, after setting up LM Studio again, shoutout to LM Studio we'll get to chat with LM Studio at one point. Hopefully soon, I am now, the first thing I do is download these models because it's super, super easy. Both of them, Studio and Allama, and there was a tiny, I think, quantization thing in the beginning, and now there isn't, and now it works great. [00:15:33] Alex Volkov: And these models, I've loaded them up on my Mac before, before a flight. And I was just able to chat with this AI with no internet connection or like poorly internet connection. It was really something. I know we've talked about this multiple times. Hey, put this on a a thumb drive and then have all of human knowledge, quote unquote. [00:15:51] Alex Volkov: I'm not really saying it's all human knowledge, but I've been actually able to do this before my flight and it was really cool. [00:15:57] Alex Volkov: And I think the last thing to mention here is that Technium suggests to make liberal use of system prompts. So all of Hermes models, which is, there's now a bunch of Hermes models flying around, definitely the most. At least the famous one is Hermes, I think, 7B, but also the YI version, and this seems to beat the YI version as far as our friend Wolfram Raven, Wolfram Loco Lama tested. [00:16:22] Alex Volkov: This is probably the best news model out of them all. So far, obviously it's based on the best. Open source model called Mixtro and definitely liberal use of system prompts. Yeah, roleplay is suggested setting expectations, specifications and everything else you can think of. Very easy to do with Elm Studio. [00:16:39] Alex Volkov: I haven't [00:16:40] dove into like actually how to steer these models for exactly the task that I do. Luigi, you said LDJ, you said that you want to Tell me how to use LM studio in regards on this. So I would love to hear from you. First of all, have you had a chance to try these models specifically? And second of all let's talk about system prompts in LM studio a little bit, because I think it's a part that people are definitely missing. [00:17:02] Luigi Daniele: Yeah. A lot of the latest models like Hermes and I think maybe Dolphin too, trained with system prompts. So if you really want to get the best use out of it definitely use that and it's just same thing with chat GPT really, where it's give instructions of how you maybe want to have it respond to you, or maybe add in a few threats of, of what you would do to the AI if it does not respond correctly, and so surprisingly that seems to actually sometimes. [00:17:28] Luigi Daniele: Give good results, I personally try to always say please and thank you, but yeah yeah. And there's also prefix and suffixes, which I think I talked to you about, Alex, [00:17:36] Alex Volkov: You briefly mentioned this, but maybe worth like a given a little bit of a heads up for folks. [00:17:41] Luigi Daniele: yeah I think it really is worth maybe just a sit down and just a video with me and you actually going through it, because, [00:17:47] Alex Volkov: Sure. [00:17:47] Luigi Daniele: it's a decent amount to go through, but, yeah on the model card of most models, if you just look at something called prefix or suffix that is usually described in the model card, then You apply that to the LM Studio settings on the right panel in the chat settings. [00:18:03] Luigi Daniele: And yeah, you just make sure you have those things right. If you don't, there's a good chance you're not actually using the model correctly. And it's not going to give you the best results. [00:18:10] Alex Volkov: And they differ from the base model as well. Like we've seen like different base models have different things that you want to you want to add there. And you may getting like the same performance, but getting under performed a little bit. I'll also say for folks who are using Mac the Silicon, Apple Silicon, there's a little hidden checkbox there that I don't know if it's like, it's by default already. [00:18:30] Alex Volkov: It's called use Apple Metal. And definitely make sure that's on for you. Significant improvement in performance and inference. All so I think NeuralRMS, anything else on folks here on stage that want to talk about this model and how it was trained and the difference in DPO? Folks, feel free to chime in. [00:18:45] Alignment Lab: There's the cool thing about DPO is It's so it's a reinforcement learning technique. I don't know if anyone else has had a chance to read the paper about it, but essentially what occurred was that some researchers found that the, that transformers already have a baked in optimal reward function. [00:19:03] Alignment Lab: And so what DPO is really doing is just training the model on that reward function, just biasing it towards the selected. Like good example when you give it a good and bad example pairs not directly unique to to the, to this model, but it is super interesting because it really opens up a whole bunch of possibilities for what you can do with the model now that you can give it negative examples and get more performance for it. [00:19:27] Alex Volkov: DPO is ranking different outputs in terms of like preference, . So can you talk about the pairs stuff? Everybody says DPO pairs, like what do they mean by pairs? Could you say this about this? [00:19:38] Alignment Lab: instead of training on like typically what you would do is you would build your data set. And that would be like your good data set. You'd have a weaker model that you, than the one that you use to synthesize the dataset or just bad examples of responses for every single example in the dataset. [00:19:54] Alignment Lab: So if you have one that's like, how do I make a cup of tea? And then instructions about how to make a cup of tea, then you'd also have that paired with a negative example of, a response to how do I make a cup of tea? And then, the response is something else, like how to build a Lego house or whatever. [00:20:08] Alignment Lab: And when you go to actually train it, you show it both at once, and you tell it which one is the positive and which one's the negative, and you just bias it towards the positive. It's quite similar, conceptually, to the way that OpenChat does the CRLFT training, although OpenChat actually has a specific token for the good and bad examples that it has weighted. [00:20:34] Alignment Lab: But functionally, it's, the idea is the same. You're just doing reinforcement learning which lets you take data where you may have bad examples in there, and rather than having to remove them and waste data, you can now make a good example and get more out of it than you would have been by just replacing it. [00:20:50] Alignment Lab: So it lets you recoup extra performance out of bad data. [00:20:54] Alex Volkov: Thanks for the explanation. And definitely we've seen at least in my game plays with the bigger model and the DPO version of noose. RMS mixture this feels like the DPO at least behaves a little bit. Actually don't know how to attribute this to the technique or to the datasets, but it's really good. [00:21:13] Alignment Lab: Yeah, we've noticed if we do a regular supervised fine tune first, like a just normal fine tuning, and then we DPO over that we, the models push just much further than either thing alone, too. I don't know if that's unilaterally true, because we do a fairly, specific kind of model when we make these big releases, but it seems, at least for the case of just general reasoning skill it helps a lot. [00:21:37] Alex Volkov: Yeah, it's super cool. And I guess the downside of this, not the downside, but the outcome of some of this is that folks now have, folks who want to just use a model and are trying to maybe tune in to Thursday Eye to know which model is good to use, or maybe they're reading the local Lama stuff. [00:21:53] Alex Volkov: There's now so many choices, including so many configurations. So maybe we should do Like a recap and also a simplification LDJ for like system messages and the prefixes alignment with DPO versus SFT. Just simplify and say, Hey folks, use this. Because right now there's so many, you can choose between quantization methods. [00:22:11] Alex Volkov: There's at least four or five different ones for you to choose from. And LM studio says in a few of them, use this is recommended, but it says recommended for five, five different ones. There's different quantization providers as well, right? So the bloke is obviously the most familiar one, [00:22:26] Alex Volkov: there's now a choice between DPO or SFT or DPO plus SFT, and We haven't even begun to talk about merges, which is coming as well. So there's a lot of choice and we need to simplify this for folks. So definitely just to simplify the Hermes models are usually very well behaved and great for role play as well. [00:22:43] Alex Volkov: Try them out. If you have the room to run Mixtrl for your stuff, Mixtrl is definitely by far the best open source models that we have. Go ahead, Levent. [00:22:52] Alignment Lab: Yeah, so Mixtrel is, that model is the architecture is very similar to a really old, comparatively old architecture that's been tried and true before. And so because of that, there's a lot of efficiencies that we just haven't integrated into the modern stack, but that will come. [00:23:09] Alignment Lab: And there's a bunch of new ones that people have been making. And between the new quantization methods that you can do with Mixtro, because since it's sparse MOE, it doesn't actually, need all of its weights as much as it, as as each other. So some of them are, like, less important. It lets you quantize those quite a lot without actually hurting the model's performance very much. [00:23:27] Alignment Lab: And you can also offload these layers when they're not being used. And then you can do like expert pre caching, where you predict some experts ahead of time, which lets you get faster inference speed. And at the end of the day, if the sort of quick sharp, which is like 2 bit quantization method continues to prove out that it's as performant as it claims, We could end up running Mixtro on 4 gigs of VRAM, like on a laptop. [00:23:58] Alex Volkov: And [00:23:59] Nisten Tahiraj: We will. [00:24:00] Alex Volkov: we will. [00:24:00] Nisten Tahiraj: it to perform a bit better. [00:24:02] Alex Volkov: So I guess this takes us to the next, I'll go ahead and stand, and it's going to take us to the next optimization stuff. [00:24:09] Nisten Tahiraj: We could definitely have it run on on 4 gigs. I've had it a little above 4. However, but the point is to have it run well. The quantization, it still makes it a little bit unfit for anything other than very short conversations. And we'll get it there. [00:24:30] Alex Volkov: All right. So in this, in, in this [00:24:32] Nisten Tahiraj: we'll have Mixtro under 4 gigs very soon and it'll be good. [00:24:37] Nisten Tahiraj: Yes. [00:24:37] Alex Volkov: And that's a promise. That's a promise. [00:24:39] LMsys SGlang - increased inference by 5X [00:24:39] Alex Volkov: So what happens is once you go and put those bigger models on slower hardware, which is possible you then wait painfully a long time for inference to actually happen. But this takes us to the next thing from the folks from LMSys. They released a fast and expressive LLM inference with Radix attention and SG Lang. [00:24:59] Alex Volkov: So folks from [00:25:00] LMSys, if you guys remember from Models like Vicuna that took Lama and trained it on additional datasets. and NMSIS Arena and all these places like we definitely trust them at least with some of the evaluation stuff. I think, is MMLU also in NMSIS's area? Or at least they test on MMLU. They released a inference optimization kind of collection of techniques. [00:25:24] Alex Volkov: I don't think it's one specific technique because there's like Radix attention. Yeah, go ahead. [00:25:28] Alignment Lab: It's where all this was going in the first place between all these sort of different prompting programming frameworks and inference engines. What they've done is they built out the back end with the end goal of having an extremely controllable, steerable compiling system for programming outputs from a, from like an AI in the way, like a Pydantic or in the way that you would typically use sort of structured grammars and sampling techniques. [00:25:58] Alignment Lab: And way more. It's hard to explain in, in summary in a way that's very easily grokkable without getting too technical but it's a combination of many things that we've been doing individually, which were always gonna be one big thing, they just saw it first and did it first, and now, when you're looking at it, it seems very obvious that this is probably how things should look going forward [00:26:17] Alex Volkov: so let's actually talk about [00:26:18] Bluetooth: overall, just a [00:26:19] Alex Volkov: they have. Yeah, they propose like different co designing the backend runtime and the frontend language, which is like Alain said, a structured domain specific language embedded in Python to control the inference generation process. It's called domain specific language, DSLs. [00:26:35] Alex Volkov: I, I think many folks have been using some of this. I think DS p Ys as well from is being like mentioned in the same breath. And then this language like executed in the interpreter code or in compiler code. And on the backend they have this radix attention technique for automatic and efficient KV cache reuse. [00:26:53] Alex Volkov: I don't know if that's like instance like MOE specific or not yet, but definitely. The combination of those two plus the code that they've released shows just incredible results. Like folks, we live in an age, and we've talked about multiple of those techniques. We live in the age where somebody like this can come up and say, Hey here's an example of a set of techniques that if you use them, you get. [00:27:12] Alex Volkov: 5x improvement on inference. In the same breath that we're saying, Hey, we're going to take Mixtrel and put it in 4GB, and we've seen this obviously with Stable Diffusion, which we're going to mention that runs fully in the browser, we're now seeing releases like this from a very reputable place. A collection of techniques that have been used to some extent by some folks, and now all under one roof, under one like GitHub. [00:27:35] Alex Volkov: Thing that actually improves the inference by 5x on all of the major evaluations, at least that they've tested, that we always talk about. So 5x on MMLU and HelloSwag is significantly more performant, all these things. Quite impressive. One thing that I would definitely want to shout out is that the maintainer of Lava the LMM, the kind of the visual Lama, is definitely also replied and said that the execution of Lama is actually, of Lava, is actually written in the report itself. [00:28:07] Alex Volkov: And it improves lava execution by 5x as well. And by execution, I mean like inference speed, basically. So without going like too much into Radix attention, because honestly, it's way too heavy for the space. It's quite incredible that we get, do we get stuff like this from like places like LMCS, specifically in the area of running smaller models, sorry, running bigger models with smaller hardware. [00:28:33] Alex Volkov: Go ahead, Nissan. [00:28:36] Nisten Tahiraj: I'll say something. So it does automate a lot of the tricks that people have been pulling, and it works great for large amounts of smaller prompts. Once you go to longer prompts, the benefit is not that much compared to VLLM. I think it felt like five or ten percent faster when it came to VLLM. So again, I haven't taken a very deep dive into it. [00:29:01] Nisten Tahiraj: Just want to just make people aware that it's fantastic for smaller prompts and stuff. But for longer ones, you don't necessarily need to switch your whole stack to it. VLLM still works fine. Yeah, I think for if you're doing like what you would normally be doing with VLLM, which is like processing like large amounts of data or serving for just general purposes. [00:29:24] Nisten Tahiraj: Probably, there's no need to switch your stack. I think for, specifically what it feels optimized for is Asian frameworks, in which you have many models communicating short strings back to each other. One model wearing many hats. And the optimizations just while we're on the topic, is crazy right now. [00:29:43] Nisten Tahiraj: There's still three papers with major inference optimizations for MixedRole alone, as well as for VLLM, and that seem to compose everything pretty well. Having an alternative to VLM that's similarly. Performance is huge because VLM is a big bottleneck on a lot of stacks because of the way that it handles attention off on the CPU. [00:30:00] Nisten Tahiraj: It feels a lot like when llama CPP got like offloading the same week that speculative decoding came out with hugging face transformers and. Everything just got a hundred times faster, like a half a year ago or so. [00:30:12] Alex Volkov: Yeah, I would also it definitely felt like that day when LMS released the SG Lang optimization that we just now talking about I don't have a link for this, but also LES released from IST Austria. Released Marlin, which is a 4 bit, I think the way I know it's cool is that, Tim Dittmers from QLOR retweeted this and said this is a huge step forward. [00:30:33] Alex Volkov: And Tim Dittmers is the guy who in KUDO mode, the codes, KUDO kernels, within like a night or something, planning for 3 months and then finishing. So I know that Tim Dittmers, when he says something is a huge deal, he probably Probably knows what's up. So Marlin released the same day that like the SGLang released and it's a linear kernel for LLM entrants with near ideal. [00:30:53] Alex Volkov: 4x speedup up to batch sizes of 16 to 32 tokens. And they came out pretty much the same day yesterday on January 17th. So I'm going to add this in the show notes. So Marlin is also like an exciting optimization. And Nostia, I fully agree with you where we see these breakthroughs or collections of method that suddenly are finally collected in the same way. [00:31:11] Alex Volkov: A bunch of papers that haven't, released code as well or haven't played with different things. And it's very exciting to see them Keep coming out, we're only at the beginning of this year. And I think to the second point that you just mentioned, with agent frameworks Specifically, RAG, Retrieval Augmented Generation this benefit is significant like you said, because the short strings back and forth, these agents communicate with each other. [00:31:34] Alex Volkov: Last week we've talked with one such author from Cru AI, Cru specifically is an orchestration of different agents that do different tasks and coordinate and talk to each other and improving inference there. Many of them run on GPT 4 and I haven't fully gotten into how to do this yet, but SGLang also say that they're like LLM programming can actually work with various backends. [00:31:55] Alex Volkov: So OpenAI as well and Tropic and Gemini and local models. That's very interesting if they actually improve OpenAI inference in Python. But DSPY RAG, so RAG on DSPYs from Omar Khattab is definitely mentioned in the SGLANG report. I know I'm throwing like a lot of a lot of acronyms at you guys. [00:32:14] Alex Volkov: So SGLANG is the stuff we talk about as the That's the new language from LMCS org that speeds up some stuff. DSPY I haven't talked about yet, so we'll cover but one of the tasks on, on, on DSPY's RAG, so retrieval is mentioned that it gets like a significant boost. Like Nissen and Austin said, not necessarily for longer context prompts. [00:32:35] Alex Volkov: 30, 000 tokens for summarization, maybe this technique that caches a bunch of. Stuff between calls is not going to be super helpful, but for fast execution of multiple things is definitely significant 5x. And like I think Lyman said, it's only the beginning of optimization cycles that we see, and it's quite exciting to to see them come out. [00:32:56] Alex Volkov: I think we've covered two optimization techniques, SGLang, and then Marlin as well. I'll put a link to the show notes as well. [00:33:03] NeuralMagic, compressing models with sparcification [00:33:03] Alex Volkov: And I think now it's time to move to Yeah, one, one, one thing that we're going to chat about is neuromagic and I definitely focus on stage. Feel free to talk about neuromagic because I saw [00:33:20] somebody told me it's cool, but I have no idea how to even simplify this. [00:33:23] Alex Volkov: So if you want us and you want to take a lead on this one, definitely feel free. [00:33:28] Alignment Lab: Okay Neural Magic. This is actually the first conversation I think that me and LDJ both geeked out really hard on we were talking, because we were both the only people the other person knew who even knew about this company. Neuromagic has been making miracles in the corner for years. [00:33:44] Alignment Lab: I first got interested in them because they had made a BERT model that was initially, it was nearly like I think a gig on your computer to run and, it spoke English perfectly well and all this other stuff. And they had compressed it to the point that the full model completely On your computer was like 15 megabytes and it, and what blew my mind was like, how does that even know English? [00:34:06] Alignment Lab: And it's it was at like 96 percent the original accuracy, despite all of that. They specialize in these like optimization and compression techniques. And so what they do typically is they have a stack, which they wrote a paper about a while ago, which I'll post in the comments here. [00:34:22] Alignment Lab: It's called Overt Surgeon, which is basically a process in which they have a teacher model. In a student model, in the student model they use distillation in the the more traditional sense than I think it's more commonly used now, where you're just training on a model's output, and they use the actual logits during they basically load both models in during the training run, and train the smaller model to behave like the larger model, and while they're doing that, they're also pruning it, which is, Essentially, you reduce the weights that are not getting used during training to zero, which lets your computer not have to calculate them, so it moves much faster. [00:34:58] Alignment Lab: And then they also quantize, which is where you reduce the accuracy. Basically, without getting too technical, you're literally summarizing the parameters of the model, such that it's literally a smaller file. And they do this all at once, which takes the larger model, And compresses it into the student model that's starting out smaller, and then they're quantizing the student model and pruning it, so it's both running faster and literally getting smaller, and they can, as far as I'm aware, there's nobody who's even coming close as far as being able to compress a model so much and recently I think about two months ago we first saw that they're integrating transformers with Sparsify Alpha, which is now just out and it's called Sparsify on the GitHub. [00:35:43] Alignment Lab: Totally check it out. You can make a tiny llama and do all that stuff to it and make it microscopic. It's amazing. And [00:35:49] Alex Volkov: here, Austin, just real quick. So we've been talking about quantization for folks who are like not following the space look super closely. Let's say there's different quantization techniques in, and some of them create like small files, but the performance or like the accuracy, is getting lowered. [00:36:03] Alex Volkov: How is Sparsification different from quantization, at least on the basic level. Are they compatible? Will they be used could you use both of them on the same file? What is this thing, sparsification? [00:36:15] Alignment Lab: so in reality, probably if it were like more accessible of a tool, we would all likely just be doing both every single training run. But since there's always new quantization techniques, it doesn't make sense to. But with sparsification, the specific difference is rather than taking the same model and reducing the accuracy of its, the calculations, but making it smaller, the model's staying the same size physically on your drive, but you're reducing the weights that aren't getting used to to a zero value. [00:36:50] Alignment Lab: And what that does is just means your, your GPU just has to do less calculations for the model to do inference and it makes it just much faster. [00:36:59] Alex Volkov: All [00:36:59] Nisten Tahiraj: Also, we for the next Baklava version, Neural Magic did make a A clip model for us. So shout out to them. They were able to cut down the size by from about four times smaller. [00:37:14] Nisten Tahiraj: So we'll we'll have that out soon. And yeah, also for anybody else that. wants to learn about sparsity, just look up Nir Shavit on on YouTube. N I R S H A V I T. He's the OG MIT professor that pioneered sparsity and has a lot of videos out, and Neuromagic is his company. And yeah, it's looking really promising in the future because they can optimize at a deep level for CPU inference. [00:37:45] Nisten Tahiraj: And it's not necessarily just quantization, it's also They are reducing the amount of unused weights. So yeah, expect to see a lot more stuff about sparsity from the GPU poor side of the spectrum, , because that's where the benefits are yet to be read. [00:38:02] Nisten Tahiraj: Anyway, shout out to Neural magic as well. [00:38:04] Alex Volkov: shout out to Neer Shovit and Neural Magic, it looks cool, and they just got into sparsifying fine tuned models as well, I think they sparsified some new models, and I don't know if they got to open chat yet, but I think some folks are waiting for PHY sparsification, definitely. The area of smaller models running on smaller hardware is advancing super, super fast. [00:38:26] Star Coder from Stability AI - 3B coding model bearing CodeLLama [00:38:26] Alex Volkov: Let's move on, folks, because we've been in the open source area for quite a while, and then we also need to get to our to the end of our conversations here and start doing deep dives. So StarCoder was released from Stability. A brief review here is a 3 billion parameter language model. [00:38:41] Alex Volkov: From Stability AI it does code completion and obviously it runs offline cause it's a small model and you can run it. They claim it can run on MacBook Airs as well. And they say something like without GPU. Interesting. Accurate completion across 18 languages at level comparable to models twice their size. [00:38:57] Alex Volkov: This is a Code Llama. Interesting comparison to Code Llama at this point, because we've seen a bunch of other models already beat, I think, Code Llama on different metrics. But people still compare themselves to the big dog. And it's very interesting. They use the multi stage process, pre training in natural language. [00:39:15] Alex Volkov: fine tuning on code datasets to improve programming language performance. And it supports fill in the middle and expanded contact sizes compared to previous versions of stable coder. And I think, oh yeah the stable diffusion now has like a commercial membership plan because everybody's thinking about, okay how was. [00:39:33] Alex Volkov: Table going to make money. So they have this membership where you can use their models. So it's not like fully open source. I think you can use this models commercially if you participate in this membership, otherwise you can use them for research. So stable quarter, check it out. I think it's new on, on hug and face. [00:39:48] Alex Volkov: I think from today I believe, [00:39:50] Discussion on Neural Beagle 7B & Model merging [00:39:50] Alex Volkov: And I think the last thing that I want to chat about in open source just briefly is Neural Beagle 7B from Maxim who's in the audience and is going to come up hopefully in the interview in a few. [00:39:59] Alex Volkov: Minutes, I want to say maybe 20 minutes, Maxim. Neural Beagle back when I added this to my notes, was the top performing 7 billion parameter fine tune in, in, in open source LLM leaderboard. It's no longer the top performing, it was definitely number 4, at least. [00:40:14] Alex Volkov: And it's a merge plus a DPO, that's what I saw from Maxim, a merge of Actually interesting what it's a merge of, so let's go into the model card and check this out. [00:40:24] Alex Volkov: But Maxim looks like have a bunch of models and Neural Beagle, the, this Neural Beagle 14, 7 billion parameters has an average of 60 on the, all the scores, 46 on AGI eval. And yeah, it's one of the top performing models and it's a merge of different things. And it already has a demo space that I'll link in the show notes as well. [00:40:43] Insights on Lazy Merge Kit [00:40:43] Alex Volkov: Yeah, it uses Lazy Merge Kit, which is a collab that Maxim also we're going to chat about and figure out what this means, what this merging thing means but definitely, I think that this model triggered one of the Nathan's in AI that says, Hey, I wanted to ignore this merge business for a while, but I guess I can't anymore because, merges is not to be ignored at this point. [00:41:04] Alex Volkov: And this is a merge of the Wunna And distilled Markoro. Slurp. So which is also a merge. So if you guys hear me and you're like confused, like what are all these things mean? Hopefully we'll be able to clarify this one. Maxim. Maxim also had a tweet where there's now a collab where you can take a model like this and basically map out the genealogy of these models. [00:41:25] Alex Volkov: What is based on what? And it's quite cool to see. And what else should I say about this model? I think that's pretty much it. It's very performative. I actually haven't had the chance to use this, but it's right up there and it's a merge model. There is, there's the [00:41:40] checkbox, like we said, in the open LLM leaderboards. [00:41:42] Alex Volkov: If you don't want for some reason to see the merge models and we'll see like more trained models, you will uncheck that. But definitely the merge models are competing for the top of the LLM leaderboards right now. Haven't seen a lot of them on the LMCs arena, so it's going to be interesting to see how they treat the merge models. [00:42:02] Alex Volkov: And I think that's most on open source, and we've given this corner almost 40 minutes, so I think it's time to move on a little bit here, folks. So I'll, yeah, I don't have breaking news here, so I'll just do this, a small transition so I can take a breath, haha. [00:42:17] Sounds: Namaskaram to all of [00:42:22] Deep mind to Alpha Geometry [00:42:22] Alex Volkov: LMs and APIs, and I think the biggest player in this whole, Aparigraha, Niyama, Shaucha, Satya, Ashtanga, Yama, Ashtanga, Niyama Ashtanga, Ashtanga, Ashtanga, Ashtanga, Ashtanga, Ashtanga, Ashtanga, Ashtanga, Ashtanga, Ashtanga, Ashtanga, Ashtanga, Ashtanga, Ashtanga, Ashtanga, Ashtanga, Ashtanga, Ashtanga, Ashtanga, Ashtanga, Ashtanga, Ashtanga, is deep mind, deep mind released, A Nature article, which they always do, they always publish in Nature, this time the link to Nature article didn't really work but hopefully they fix it by now, and they released Alpha Geometry, so they released like a bunch of stuff, Alpha Fold, if you remember, Alpha Go Alpha Zero, they had a model that, that self trains to play anything, not only chess, or, not only Go, and now they've released Alpha Geometry, that solves geometry, almost a gold medal Level at the at the Olympiad level, so they have this this how should I say, this nice chart that says the previous state of the art on this Olympia Gold Medallist Standard gotten to ten problem solved there's like time limits. I'm not sure what the time limits are actually are. I don't have it in my notes. But you have to solve these like very like difficult geometry levels. Folks compete for the gold medals in this Olympiad. And alpha geometry now comes very close to the gold medalist standard. [00:43:29] Alex Volkov: So the gold medalist is answers 25.9 problems solved, and alpha geometry now answers 25, and they claim that the previous state of the art answered 10, just 10. So they more than doubled and they're getting close to the Olympiad. I think I saw like a tweet from Nat Friedman or somebody. That says they would offer a 1, 000, 000 prize for somebody who solves the Geometry Olympiad at the Golden Medal, and now we're getting there. [00:43:53] Alex Volkov: They use the newer symbolic approach and they combine all of them with a symbolic deduction engine to leverage the strength of both. Which some folks compare to thinking fast and slow, where you have system 1, system 2 thinking, or at least the outline system 1, system 2 thinking. [00:44:09] Alex Volkov: In this case, this does actually help. They have the neuro symbolic approach. I think they use this, the neuro symbolic approach. I don't think I've seen this before. And I think the most interesting parts It was trained on over a hundred million synthetic geometry examples generated from one billion random diagrams. [00:44:27] Alex Volkov: Completely, solely synthetic geometry examples. This whole data set for training of this model that beats Humans at Geometry, which was previously very difficult, is fully synthetic. And I think that's super cool. We only began this year, but definitely this is going to be the year where full synthetic datasets are going to rule. [00:44:49] Alex Volkov: And Yeah. Opinions, folks here on stage. Have you read about this? What's interesting to you? I would love to hear folks kind of chime in on this as well, because I think it's like super cool and kudos for them to releasing this. Also, I saw somebody said, I think Bindu said that they released this open source, but I haven't seen anything. [00:45:06] Alex Volkov: Definitely Luigi Go and then Nistan. [00:45:09] Luigi Daniele: Yeah it's funny that you brought up Nat Friedman having that bet up. Because I remember that too, and now I'm thinking, I wonder if he'd be willing to give up like the million dollars or whatever the money is to DeepMind. Ha [00:45:20] Luigi Daniele: was done by Google DeepMind, so that'd be funny. [00:45:25] Nisten Tahiraj: How has Google not discovered AGI yet and fallen so behind? [00:45:30] Nisten Tahiraj: This almost feels like an internal illness or something. Something's going on. Because yeah. [00:45:40] Alignment Lab: I don't think that Google needs to compete is the thing. I just don't think they're incentivized to release anything into the space because they don't have to. There's really not anything here except money to lose for them. [00:45:51] Alignment Lab: They already have all the data and stuff. Yeah, and back to the geometry problems, I can't wait to test this, if they release it, as to how it does when given really random, very long numbers. If it still solves the problem, then that, that will be extremely impressive. And yeah, I've done those Math Olympias with geometry questions and they're not easy at all. [00:46:18] Alignment Lab: You have to picture stuff in 3D. 4D and whatever in your head. They're very tricky problems. So yeah this is pretty huge. That's all. Yeah. [00:46:26] Alex Volkov: Quite, quite huge and kudos on them. Umesh, I think you actually found the source, right? I just [00:46:32] Umesh Rajiani: Yeah so there is GitHub repo on Google DeepMind. So if you go to Google DeepMind on GitHub and then alpha geometry, you can find the code repo for that. So Nistan, if you want to test it out, it's there for you. So I'm taking your [00:46:47] Alex Volkov: hark on this just like for a little bit. Did Google release code for us finally? Did Google like open source something? Welcome back, Google. [00:46:54] Umesh Rajiani: yeah, so this is like first release kind of thing, coming out of Google. So it's going to be, yeah, it is quite quite interesting. [00:47:01] Alex Volkov: Definitely moves us towards like more generalist [00:47:04] Bluetooth: I'll have it up in a sec. [00:47:05] Alex Volkov: Yeah, listen, please put this and we'll add this to the show notes as well. Definitely the question, how have they not solved AGI yet? Solving math at the Olympiad level seems like moving us forward, definitely. This neuro symbolic approach where they combine language models with a symbolic deduction engine, which I have no idea what symbolic deduction means in this case. [00:47:24] Alex Volkov: But leveraging strength of both, this seems like going towards the right path. We've seen, I think Similar things with vision as well, where you combine kind of vision heads into one model they can understand. I don't think this model was multi modal at all. Doesn't look like, but maybe I'm wrong here. [00:47:42] Alex Volkov: And I think Yeah, the solutions for this thing is verifiable by machines. I saw this one tweet that will go down in history. Somebody said, computers has always been good for calculations. So I don't understand the big deal there, here. And I think I think it's really funny to like, keep this tweet behind the scenes. [00:48:04] Alex Volkov: Alright, so shout out to DeepMind for this fairly incredible release. Hopefully some of the techniques they used will be then used by folks in other areas as well to get us AIs that are significantly better at the geometry and different things. Oh yeah, Umesh, just before, before we continue, you want to talk about this NeuroSymbolic thing? Cause we've talked about this. I think Daniel Jeffries talked about this last time we've talked about Rabbit. [00:48:27] Alex Volkov: If you guys remember, this was at the end of the last space and we've talked about Rabbit LAM, Large Action Model. And Umesh, you just mentioned something that they also use NeuroSymbolic to an extent, right? [00:48:39] Umesh Rajiani: Yeah, so the LAM Large Action Model, basically based on Neuro Symbolic Programming for when, specifically when they are talking about training the model from the actions that you're going to perform is basically they are encoding Neuro Symbolic Programming to train the model or capture the actions, basically. [00:48:55] Umesh Rajiani: So that's what we're trying to do. Namaste. In theory, they are saying we have to see what comes out in practice. [00:48:59] Alex Volkov: Yeah, and based at least on their examples, it looks like very compelling and potentially like being able to solve a bunch of stuff or like to remember based on your actions. So neuro symbolic not a new approach. I apologize. I will edit this. Definitely Rabbit said this, you're right and hopefully we're going to get to see this lamb thing. [00:49:19] Alex Volkov: So back to OpenAI as elections are happening right now and everybody was fearing like, Hey, what's going to happen with deepfakes, et cetera. OpenAI released their guidelines toward election, as they prepare for elections, obviously, they're aware that they're happening. And I think the few interesting things there that they're taking steps to prevent their tools like Dalai and Shajipati from being abused. [00:49:38] Alex Volkov: I don't know. We have open source, so I don't know if folks will go to the GPT 4 to generate let's say, propaganda. But DALI, for example, starts to integrate some cryptography to their images, which is very interesting. Cryptography solutions, which, again, In case you download the actual file and then send it, could be a thing. [00:49:58] Alex Volkov: But I don't know if [00:50:00] somebody takes a screenshot of a Dalit generation, if that will apply at all. There are definitely like usage policies for like stuff like Chajapati enforcing limits on political campaigning and impersonating candidates and discouraging voting. And then they want to run ahead of what happened with Facebook and Cambridge Analytica, and like all these things they want to get ahead of us which, it makes sense. [00:50:18] Alex Volkov: So the technology they use to detect images were generated by DALI I haven't seen any release on them that says, Hey, we'll build a tool for you to actually identify if those are generated images or not. It's going to be interesting because like with LLM writing all of these tools that you use to like dump AI text in there, they're all can be obscured with another LLM. [00:50:38] Alex Volkov: I don't know if it's a futile attempt, but definitely a worthwhile one. And at least in the basic UI, I think blocking some attempts of destabilizing democracy, I think it's a good idea. And I think that's mostly it. I think there's one different mention that somehow silently they removed where the terms and conditions thing where their outputs is not to be used for war or weapon developing. [00:51:04] Alex Volkov: And I think they removed that and I think they're also like signed something with Department of Defense, but I think that's all for OpenAI. [00:51:11] Microsoft announces CoPilot pro [00:51:11] Alex Volkov: And then I wanted to mention about Microsoft and Umesh, definitely feel free to chime in here as well, because the underlines the benefit for open source, but quickly Microsoft announced Copilot, we've talked about Copilot, the kind of previously BingChat, Copilot everywhere. [00:51:25] Alex Volkov: So they've announced like different paid plans for Copilot Pro, 20 bucks a month premium, and then it does. Enhanced image creation, where we don't even get We don't even get in, in, in Dali like by default, and it's now generally available for small businesses with no user minimum. So if you guys remember, we've talked about Copilot before when Microsoft announced it for large enterprises it integrates into Microsoft 365 everywhere. [00:51:49] Alex Volkov: And now the Copilots are also open for smaller businesses. And soon there's going to be like this Copilot Studio to build custom GPTs. Very cool for small businesses. We'll see how much actually folks will use this. And there's also some Microsoft Saga that they've changed some stuff in their pipeline. [00:52:04] Corporate Drama - Microsoft Azure changing moderation flows and breaking products [00:52:04] Alex Volkov: So Umesh, you mentioned this in the beginning. We'd love to hear from you what's been going on as you guys are big Azure users through Microsoft. [00:52:11] Umesh Rajiani: Ooh happened [00:52:15] Umesh Rajiani: day before yesterday. Actually, we got a call from one of our clients, which is one of the, one of a very big financial institution. And we have a deterministic pipeline, which was constructed using Azure studio, in fact. And we work together with very core Microsoft team actually to make sure that it is right. [00:52:36] Umesh Rajiani: properly deterministic because there are some legal implications and everything. And and then the tool started failing and because we had some function calling, which would actually go into the knowledge base of the company. And that function calling was was getting extracted, getting triggered using what you call the deterministic intent from user's prompts, basically. [00:52:56] Umesh Rajiani: And and that entire function calling was failing. Now, we carried out all types of work and everything it was very frantic because it was a front end tool and it started having some impact. And it was, remember, it was working for six months. So it's it worked without any problems for six months and suddenly it just stops working. [00:53:14] Umesh Rajiani: And the reason was that there were two words that were in the definition of The tool, so that definition of tool was actually informing the pipeline what the tool is all about and that's how the tool was getting invoked and those two words basically were getting flagged into The OpenAI API. [00:53:32] Umesh Rajiani: So we're basically Azure OpenAI API, not OpenAI's direct API. We are routing it through Azure and it's a separate separate instance of of GPT 4 and there are separate guidelines. They mimic some of the guidelines that are there in OpenAI, but Microsoft has its own guidelines and they change the guidelines without actually informing the clients. That basically triggered. Yeah. So we literally we literally had legal people and literally had fight. It was an open fight, literally, with Microsoft. If you were in that room, you would have you would have seen. It was really bad. And and then eventually there were talks about cases and stuff like that. [00:54:08] Umesh Rajiani: And eventually, basically actually this company is actually modifying the contract with Microsoft. Where Microsoft will be liable to inform the company before they change any kind of guidelines. And you know what happened after that is, is the beauty because in the beginning of my startup, like beginning of the year, we implemented some solutions where we have a direct contract with Microsoft And we have implemented solution on the backing of those contracts. [00:54:34] Umesh Rajiani: So in last two days, actually, I've gone back to those clients with whom we have implemented solutions so that they have a direct contract with Microsoft, because we don't want to be a party involved as far as the SLAs are concerned, because this is very dangerous if you're developing solutions for. [00:54:49] Umesh Rajiani: For people and and if the core solution through which you are driving the entire application pipeline is getting changed without any kind of data contract backing, so to say. Yeah, this is a great learning for us and I've been always a proponent of. Open source solutions, and I think this has given one more kind of a booster to us because now we can go back to the new clients and say, Hey, guys if possible, if we give you the kind of solution that you're looking for, then let's go to open source solution rather than going for a closed source solution. [00:55:20] Umesh Rajiani: So [00:55:20] Alex Volkov: And this is like a huge, yeah, a huge like reason why, right? Getting, it's very interesting, like in this area we mentioned, definitely feel free to chime in on this a little bit more. The outputs of LLMs are usually non deterministic. And so this has to be built into understanding when you build tools on top of this. [00:55:36] Alex Volkov: But this is not that. This is them adding not like a different model or something like a different that you can switch. They're adding something in between or some like policy thing without announcing this to the customers. And supposedly if you go to Azure instead of OpenAI, for example, you would go for the most stability as underlined by the fact that when OpenAI had downtime after Dev Day, Microsoft Azure, GPT for like endpoints, they were all fine. [00:56:02] Alex Volkov: They were all green, right? So supposedly you would go for the stability and kind of the kind of the corporate backing. There's also like different ISO things and HIPAA compliances, like all these things that Microsoft Azure like proposes on top of OpenAI. But here we have a case where like underlines how. [00:56:17] Alex Volkov: How important open models that you host yourself are, even if you host them, like maybe on Azure as well, because then nobody can change the moderation endpoints for you and suddenly decide that a few words in your prompt are not, to be used anymore. [00:56:32] Umesh Rajiani: Yeah, but Alex this had nothing to do with the prompt, actually. It was actually the definition of the function that was there. And the key is like I would draw an analogy to what you call the data contracts. I don't know how many people are aware of data contracts, but when you have. [00:56:47] Umesh Rajiani: Ownership of data within a very large organization, let's say 20, 000, 30, 000 people up you have data contracts where the data originates from a particular source and some other division is using that data. So you have a contract between those two and that data contract details the data definitions which are there and the contract sign, the signatory of the contract is responsible to ensure that if they change any kind of data structure or data definition. [00:57:14] Umesh Rajiani: Then the receiver of the data or the client of the data contract is supposed to be informed. That is a part of your data contract. And that's how these large organizations function. And what we need is that kind of a framework where you have a data contract with the service provider. [00:57:30] Umesh Rajiani: So even if you're going with an open source solution, and if your open source solution is hosted by someone, Then you need to have that kind of a contract in place. So it's not just that open source solution is a solution for everything. It's about the person who is providing the inference. So if you are controlling the inference, then you are secure because you are not going to make the changes without, understanding the repercussions of those changes. [00:57:52] Umesh Rajiani: But if you are let's say hosting open source model on Amazon Bedrock, for example. And if they have a system prompt that lies in front of your prompt that goes to the the model, then you have to make sure that Amazon adheres to their responsibility in terms of giving you the required inference. [00:58:12] Alex Volkov: Absolutely. Thanks for giving us the, first of all, like it's, it sucks that it happens and hopefully now Microsoft, like you said, they [00:58:20] changed their their approach here. Aniston, go ahead if you want to follow up. [00:58:26] Nisten Tahiraj: Yeah. So for us, this has been amazing. I already have clients lining up to pay for the Baclav API. So I'll just say that first before it's even out. However It is extremely unfortunate for those that built, let's say, apps in a hospital or for a therapist because now those kinds of applications just had a moderation engine added, and they added apparently for their safety, and now whoever was relying on these applications, now they just stop working. [00:59:02] Nisten Tahiraj: Out of nowhere, and this is an extremely immature thing to do this is something you expect from like a random startup with kids, not from freaking Microsoft, and it is pretty worrisome that this safety hysteria has gotten to the point where You're literally just breaking medical applications in production without modifying, without notifying people. [00:59:27] Nisten Tahiraj: That's just, you lost people's trust now. You're not going to gain that back for a couple of years. And I hope they realize and don't do this again. Don't break production and make changes. To people in Prad that are relying on this for like SOC 2 or as in the case of UMass that have signed service level agreements. [00:59:49] Nisten Tahiraj: Because now those people lose all their money if they don't, if they don't provide the service. And it's really bad. That's all I have to say. It's pretty bad. [00:59:58] Alex Volkov: Yep. Very bad look from Microsoft. Even I think I remember like not entirely OpenAI, when they talked about Sunsetting some models and there was like a developer outcry that said, Hey, like we use those, we haven't had time to change how we work with different prompts, et cetera, for the newer models. [01:00:15] Alex Volkov: And so OpenAI actually went back and said, Hey, we heard you and we're going to release we're going to deprecate deprecation is going to be pre announced in advance. It's going to be way longer Omesh let's yeah, let's go ahead. [01:00:27] Umesh Rajiani: Yeah, very quickly I think you have raised a very valid point, Alex, that I think all the models that they actually put out of service, they actually should make them open source. I think that's the best solution. [01:00:39] Alex Volkov: Nah, I wish this was the case. We're still waiting for potentially like open source GPT 2. 5. We haven't seen any open sources from OpenAI for a while. Besides like some GitHub code, I agree with you. There should be a way for folks to keep doing this, the same exact thing they're doing. [01:00:52] Alex Volkov: I don't know, in my example, I use Whisper, no matter like what their API really says, what it's like, what they deem inappropriate to translate, the Whisper that I use is hosted and it will be the same version until I decide basically and test everything. All right, folks, we're moving forward, I think, just quickly. [01:01:10] Alex Volkov: There's not a lot of stuff in the vision area. I will mention briefly we've been here for more than an hour. So I'll definitely like recap the space a little bit. If you're joining, let me just play the music and then I'll recap and then we'll get into the interview. So with with Hour 15, you're listening to Thursday Eye. Those of you who just joined us, welcome. If you haven't been here before, this is a weekly space all about AI, open source, as our friend of the pod, Jan, just tweeted out, everybody and everybody in LLM space and open source is in here, and very great to see. [01:01:45] Alex Volkov: We've covered open source stuff, we've covered corporate drama right now, and then we're moving on to an interview. Thank you. [01:01:53] This weeks Buzz from Weights & Biases [01:01:53] Alex Volkov: And then we're going to talk about AI, art, and diffusion, if we're going to have time at the end of this. There's a brief mention that I want to say, but basically, let me just reintroduce myself. [01:02:01] Alex Volkov: My name is Alex Volkov. I'm the AI Evangelist with Weights Biases. And we have a small segment here for Weights Biases that I want to choose to just bring. I just came back a few days ago from San Francisco Hackathon, the WeHub sponsor with TogetherAI and LengChain. It was a pretty cool hackathon. [01:02:20] Alex Volkov: It was very brief, like a few hours with AGI House. But basically the theme was RAG versus FineTune. And I think the theme was versus, and I just promised I'll bring some learnings from this. So there's a bunch of projects that did different things. They used Together's endpoint for FineTune. [01:02:35] Alex Volkov: So if you can FineTune. On your models and your GPUs that's one thing, but for many of the AI engineers, that's very difficult to do. So there's a bunch of startups together as one that they offer like very simple fine tuning. I'll definitely add my my Link to the show notes, to the presentation I gave there, which talks about how easy it is to fine tune using their endpoints. [01:02:56] Alex Volkov: And the folks that won the hackathon, some folks won different prizes, basically used both Reg and FineTune. And it looks like also there was a paper released afterwards from some folks trying to identify what's better. Is it just doing RAG on top of Hindu models or just doing basic RAG? [01:03:13] Alex Volkov: And I don't think we have a clear answer yet. Definitely this hackathon wasn't the end all of all answers. However it does look like doing RAG on top of a fine tuned model improves just a little bit on top of just basic RAG. And it looks like RAG wins on top of just a regular fine tuned for information retrieval tasks as well. [01:03:30] Alex Volkov: So definitely do not skip RAG. And I think from the open source perspective, which we love here on Thursday Eye getting more RAG kind of Related models is definitely going to happen. I think we saw some from John Durbin. I think I saw Technium. You mentioned something about like function calling. [01:03:47] Alex Volkov: Datasets are coming to, to, from news as well. So definitely that area is still to be explored. But it looks like the combination of FineTune and RAG wins just a little bit on top of just basic RAG. I think this is the outcome of that hackathon. Next week in this corner of 1B is going to be an interview with Jason. [01:04:06] Alex Volkov: Stay tuned for that. [01:04:07] BREAKING NEWS - Meta announces LLama 3 is training and will be pen source [01:04:07] Alex Volkov: I think now we have, and many folks have been DMing me because right now we have breaking news. Breaking news actually happening right now. [01:04:17] Sounds: AI breaking news coming at you only on Thursday ice. [01:04:27] Alex Volkov: You know I love to use this sound. You know I love to use this sound, everyone. We have some updates from BigZuck. you guys see this because it's over on threads. And I don't know how many of us are on threads. I definitely know that I barely go there. We have some updates from BigZuck specifically around Training Lama 3. [01:04:43] Alex Volkov: There's like key updates about the long term vision. I think the summary there is They have an insane amount of GPUs this year. So like literally he says at the end of this year, we'll have three, around 350, 000 NVIDIA H100s. I'm going to repeat this slowly for the people in the back. 350, 000 NVIDIA H100s and overall 600, 000 H100s or equivalents of compute if you include other GPUs. [01:05:13] Alex Volkov: You remember those hats that people wear, like GPU poor, GPU rich hats? I think Zack can stack the GPU rich hats, like one on top of the other and it still won't be enough because 600, 000 H100 compute is just like ridiculous. And he talks about. Two major parts of their vision, AI and Metaverse are connected. [01:05:32] Alex Volkov: I love how like it was Metaverse, and then suddenly AI started being a thing and now oh, they're connected. I definitely am expecting AI to exist in some form of virtual slash world, et cetera. But definitely he talks about Lama 3. And Lama 3 is coming. They're currently training it per BigZakh. [01:05:48] Alex Volkov: We know that's coming or like we at least expected this, but I think now is like more of a confirmation. And I'm very excited about Lama 3. I will just mention that it's not been a year since Lama 1 yet. So we're in January Lama was released in like around February 12th, 13th or so. [01:06:06] Alex Volkov: And it's not half, like it hasn't been a year yet. And here we are like training the third model on top of Lama. We've had just an incredible amount of like innovation on top of it. So definitely expecting and we're obviously going to cover this as much as possible. So this is I think most of it. [01:06:23] Alex Volkov: Oh and this last thing that he added, Zak has added and I think it's Adding to Thursday as well where we have to start talk about hardware is that he says I think lots of people will talk to A. I. s frequently through the day using smart glasses like what we're building with Ray Ban Meta. [01:06:38] Alex Volkov: And I think we've [01:06:40] talked about their smart glasses that they're like multi modal glasses. They have a camera built in them. You can press a button and actually pass the image into the LLM. They're making improvements in speed as well. I will say just like an additional one thing we've talked how Meta is adding a bunch of AI into every chat and nobody like necessarily used them. [01:06:58] Alex Volkov: Recently, a friend of mine, maybe because, I'm an AI evangelist, so he felt free to do this in our chats. He just added an AI bot to our chat. Literally, just like my DM with a friend who has no, nothing about AI, like it's not part of his world. He does something else. Recently, he's Hey, let me add this thing. [01:07:14] Alex Volkov: So Meta is definitely letting folks experiment with AI more than some other places. And he just added in the AI to our chat. It was super cool. So here's an update from Zack BigZack. Allama3 is training and then they have a lot of GPUs. They're like super GPU rich and, hopefully we'll get the benefit. [01:07:30] Alex Volkov: Go ahead, Nissan. Yeah, [01:07:36] Nisten Tahiraj: H100s? Yeah, they're going to need that if they're going to have visual stuff from people's glasses. But it's an insane amount. That's all. Yeah, I just ran some quick calculations. I got roughly similar numbers to what Nishtan just said. And if I'm doing my math I'm running just some numbers based off the alleged GPT 4 leaks of the amount of GPU hours that it might take, let's say if they used all those meta GPUs. [01:08:08] Nisten Tahiraj: It's do a GPT 4 level model. I'm getting numbers it would take less than a week pretty much to train, yeah, this is an insane amount of GPUs for people that, don't have good references for this. Yeah. [01:08:18] Alex Volkov: I think it's insane enough to maybe open a new category like on top of GPU rich. It's just quite incredible and like hopefully they're committed to the open source of this in Lemma 3. Omesh, you had a comment as well? [01:08:32] Umesh Rajiani: Yeah, what if Lama 3 is going to be multi modal? Then they will need those GPUs. [01:08:37] Alex Volkov: I'm really hoping it will. Like they're training the models, like multimodality is something they talked about. It's time. To move towards the LMM world and multimodality, and they will need all those GPUs to crank out. The vision part of this hopefully multimodal in other areas reminder meta has released like bull a bunch of attempts at multimodality in other areas, not only image. [01:08:59] Alex Volkov: IMU motion units and they've talked about F-F-M-R-I signals they've talked about, like incredible stuff. But definitely modality, other modality like sounds like audio. Live video would be super cool, like I think this year is the year of live video, so not only, hopefully not only vision, and if it's vision, then hopefully it's like a live video. [01:09:18] Alex Volkov: Alright folks, we're coming up on two hours, [01:09:20] Alex Volkov: and with that, I think this is the summary of today's Thursday Eye. Thank you everyone for joining. If you haven't subscribed yet, definitely feel free to subscribe at ThursdayEye. News. I appreciate everyone's time and attention here. Thank you so much for the Co hosts and guests for today's pod and shallow with everyone. [01:09:36] Alex Volkov: And I have to end this on the very happy note of the alchemy thing, because the one thing that came out from the conversation with with Maxim, who merges and Nistan and everything is that a lot of this is alchemy and a lot of this is like trying to see how things work when you combine and not continue to train models, they still perform better. [01:09:55] Alex Volkov: So I have to end on this very happy tune, which will represent the alchemy that we're all doing. And we love it. Thank you everyone for joining this Thursday. I will see you next week. Cheers. And we'll add this banger to the show notes as well. Bye everyone. This is a public episode. If you'd like to discuss this with other subscribers or get access to bonus episodes, visit sub.thursdai.news/subscribe | |||
28 Jan 2024 | 📅 ThursdAI - Sunday special on Merging with Maxime LaBonne | 00:35:30 | |
Hey everyone, we have an exciting interview today with Maxime Labonne. Maxime is a senior Machine Learning Scientist at JPMorgan, the author of Hands on GNNs book and his own ML Blog, creator of LazyMergeKit (which we cover on the pod) and holds a PHD in Artificial Intelligence from the Institut Polytechnique de Paris. Maxime has been mentioned on ThursdAI a couple of times before, as he released the first Phi mixture-of-experts, and has previously finetuned OpenHermes using DPO techniques which resulted in NeuralChat7B For the past couple of months, following AI on X, it was hard not to see Maxime's efforts show up on the timeline, and one of the main reasons I invited Maxime to chat was the release of NeuralBeagle7B, which at the time of writing was the top performing 7B model on the LLM leaderboard, and was specifically a merge of a few models. Model merging Model merging has been around for a while but recently has been heating up, and Maxime has a lot to do with that, as he recently checked, and his wrapper on top of MergeKit by Charles Goddard (which is the library that put model merging into the mainstream) called LazyMergeKit was in charge of >50% of the merged models on HuggingFace hub leaderboard. Maxime also authored a model merging blogpost on Hugging Face and wrote quite a few articles and shared code that helped others to put merged models out. Modern day Alchemy This blogpost is a great resource on what model merging actually does, so I won't go into depth of what the algorithms are, please refer to that if you want a deep dive, but in a nutshell, model merging is a technique to apply algorithms to the weights of a few models, even a few instances of the same model (like Mistral7B) and create a new model, that often performs better than the previous ones, without additional training! Since this is algorithmic, it doesn't require beefy GPUs burning power to keep training or finetuning, and since the barrier of entry is very low, we get some cool and crazy results as you'll see below. Yeah, quite crazy as it sounds, this method can also create models of non standard sizes, like 10B or 120B models, since it's slicing pieces of other models and stitching them together in new ways. If you recall, we had a deep dive with Jon Durbin who released Bagel, and Jon specifically mentioned that he created Bagel (based on everything everywhere all at once) as a good base for merges, that will include all the prompt formats, you can read and listen to that episode here This merge frenzy, made HuggingFace change the leaderboard, and add a checkbox that hides model merges, because they are flooding the leaderboard, and often, and require much smaller effort than actually pre-training or even finetuning a model And quite often the top of the leaderboard was overrun with model merges like in this example of Bagel and it's merges by CloudYu (which are not the top ones but still in the top 10 as I write this) ThursdAI - Recaps of the most high signal AI weekly spaces is a reader-supported publication. To receive new posts and support my work, consider becoming a free or paid subscriber. On why it works? Nisten summarized this pretty well in this now famous copypasta tweet and I've confirmed with Maxime that this is his current understanding as well, it's quite unclear why this seems to perform so well, but it of course doesn't stop the "folks who look for AI Waifus" to keep merging. Following folks like Nathan Lambert from interconnects.ai to start paying attention even though he didn't want to! (Still waiting on your writeup Nathan!) UPDATE: As of today Monday Jan 29th, Nathan Lambert just released a super comprehensive deep dive into merges, which you can read here 👇👏 YALL + Automated LLM Evaluation Maxime as also worked on so many models of his own, that he built a convenient little tracking leaderboard to track their performance, which he called YALL, Yet Another LLM Leaderboard and it's on HuggingFace. You can see that NeuralBeagle is the top dog (sorry, I literally could not resist) It uses the Nous evaluations, and Maxime has created an automation called LLM AutoEval that makes it really simple to run evaluations, which you can run in a Colab super easily. LLM AutoEval is on Github. Merge-aology! Since chatting, Maxime has released a Colab and later a HuggingFace space that takes models names, and shows the genealogy, nay, Merge-aology of the models, which models it was merged from and it's pretty crazy how deep this rabbit hole goes, and crazier even still that these models perform very well after all of these lobotomies! Try it out here: https://huggingface.co/spaces/mlabonne/model-family-tree I really hope you enjoy this special deep dive, I definitely learned a BUNCH from this conversation with Maxime, and I'm very happy that he came on! This is a public episode. If you'd like to discuss this with other subscribers or get access to bonus episodes, visit sub.thursdai.news/subscribe | |||
26 Jan 2024 | 📅 ThursdAI - Jan 24 - ⌛Diffusion Transformers,🧠 fMRI multimodality, Fuyu and Moondream1 VLMs, Google video generation & more AI news | 01:40:45 | |
What A SHOW folks, I almost don't want to write anything in the newsletter to MAKE you listen haha but I will I know many of you don't like listening to be babble. But if you chose one episode to listen to instead of just skimming the show-notes, make it this one. We've had 2 deep dives, one into the exciting world of multi-modalilty, we chatted with the creator of Moondream1, Vik and the co-founders of Prophetic, Wes and Eric about their EEG/fMRI multimodal transformer (that's right!) and then we had a DEEP dive into the new Hourglass Diffusion Transformers with Tanishq from MedArc/Stability. More than 1300 tuned in to the live show 🔥 and I've got some incredible feedback on the fly, which I cherish so if you have friends who don't already know about ThursdAI, why not share this with them as well? TL;DR of all topics covered: * Open Source LLMs * Stability AI releases StableLM 1.6B params (X, Blog, HF) * InternLM2-Math - SOTA on math LLMs (90% GPT4 perf.) (X, Demo, Github) * MedArc analysis for best open source use for medical research finds Qwen-72 the best open source doctor (X) * Big CO LLMs + APIs * Google teases LUMIERE - incredibly powerful video generation (TTV and ITV) (X, Blog, ArXiv) * 🤗 HuggingFace announces Google partnership (Announcement) * OpenAi 2 new embeddings models, tweaks turbo models and cuts costs (My analysis, Announcement) * Google to add 3 new AI features to Chrome (X, Blog) * Vision & Video * Adept Fuyu Heavy - Third in the world MultiModal while being 20x smaller than GPT4V, Gemini Ultra (X, Blog) * FireLLaVa - First LLaVa model with commercial permissive license from fireworks (X, Blog, HF, DEMO) * Vikhyatk releases Moondream1 - tiny 1.6B VLM trained on Phi 1 (X, Demo, HF) * This weeks's buzz 🐝🪄 - What I learned in WandB this week * New course announcement from Jason Liu & WandB - LLM Engineering: Structured Outputs (Course link) * Voice & Audio * Meta W2V-BERT - Speech encoder for low resource languages (announcement) * 11 labs has dubbing studio (my dubbing test) * AI Art & Diffusion & 3D * Instant ID - zero shot face transfer diffusion model (Demo) * 🔥 Hourglass Diffusion (HDiT) paper - High Resolution Image synthesis - (X, Blog, Paper, Github) * Tools & Others * Prophetic announces MORPHEUS-1, their EEG/fMRI multimodal ultrasonic transformer for Lucid Dream induction (Announcement) * NSF announces NAIRR with partnership from all major government agencies & labs including, OAI, WandB (Blog) * Runway adds multiple motion brushes for added creativity (X, How to) Open Source LLMs Stability releases StableLM 1.6B tiny LLM Super super fast tiny model, I was able to run this in LMStudio that just released an update supporting it, punches above it's weight specifically on other languages like German/Spanish/French/Italian (beats Phi) Has a very surprisingly decent MT-Bench score as well License is not commercial per se, but a specific Stability AI membership I was able to get above 120tok/sec with this model with LM-Studio and it was quite reasonable and honestly, it’s quite ridiculous how fast we’ve gotten to a point where we have an AI model that can weight less that 1GB and has this level of performance 🤯 Vision & Video & Multimodality Tiny VLM Moonbeam1 (1.6B) performs really well (Demo) New friend of the pod Vik Hyatk trained Moonbeam1, a tiny multimodal VLM with LLaVa on top of Phi 1 (not 2 cause.. issues) and while it's not commercially viable, it's really impressive in how fast and how quite good it is. Here's an example featuring two of my dear friends talking about startups, and you can see how impressive this TINY vision enabled model can understand this scene. This is not cherry picked, this is literally the first image I tried with and my first result. The image features two men sitting in chairs, engaged in a conversation. One man is sitting on the left side of the image, while the other is on the right side. They are both looking at a laptop placed on a table in front of them. The laptop is open and displaying a presentation, possibly related to their discussion. In the background, there is a TV mounted on the wall, and a cup can be seen placed on a surface nearby. The scene suggests a casual and collaborative environment where the two men are sharing ideas or discussing a topic. Vik joined us on the pod to talk about why he didn't go with Phi-2, he also mentioned that Phi-1.5 was retroactively also MIT'd, it's license literally says MIT now on HF 👏 Great conversation, tune in for that at around 00:31:35 Adept is teasing FuYu Large - their CHONKY VLM Adept previously released Persimmon, and then Fuyu VLM (which is a type of persimmon we see you adept) and now tease the release for Fuyu Heavy, a much bigger model that can compete or come close to GPT4V and GeminiUltra on MMMU and MMLU (text) while being 20x smaller approx. While we don't yet get to play with this, they show some great promise in the benchmarks ⭐️ Performance: Excels at multimodal reasoning and matches/exceeds text-based benchmarks.❗️ Challenges Faced: Dealt with issues related to image data, model stability, and pre-training data scarcity.✅ Evaluations: Outperforms Gemini Pro on MMLU and MMMU benchmarks.AI Summary by Arc Browser (haha see how I cheated here? I sometimes do shortcut summaries using Arc Max, it's dope, try it) https://t.co/BZi6EKhS5R Fireworks AI releases FireLLaVa - with a commercially available license FireLLaVA is the first commercially permissive open-source LLaVA model, a type of multi-modality model called a Vision-Language Model (VLM) that can understand both visual and textual inputs. * The original LLaVA model was limited for commercial use as it was trained on data generated by GPT-4, which has non-commercial licenses. * Fireworks.ai recreated the LLaVA training data using an open-source language model, CodeLlama 34B Instruct, to make a commercially viable version.- * FireLLaVA performs comparably to the original LLaVA model on benchmarks, showing open-source models can generate high-quality data for VLM training. * FireLLaVA is available via HuggingFace and through Fireworks.ai's prediction API, enabling new visual capabilities for applications. Vik and I chatted about this, and while Fireworks didn't release datasets, they did release an example of how to start collecting them, and it's clear that everyone is clamoring after great vision / image datasets 👏 Really hoping that many great dataset for multimodal AIs will come out in 2024 giving us increasingly better multi modal LMMs 👏 Big CO LLMs + APIs (Blog) GOOGLE announces LUMIERE video generation model that shows incredible push in consistency Supports multiple tasks like image to video, text to video, video inpainting, Video stylezation and more, looks incredible. It seemed that they have cracked both spatial and temporal consistency, something that's severly lacking in previous video generation attempts, and makes character consistency quite remarkable. Of course, as with other google incredible papers, we never know if we'll ever see this model or be able to play with it, here's hoping 🤞 Google will add 3 new AI features to chrome * Chrome is introducing 3 new experimental AI features to make browsing more efficient: * Tab Organizer: Chrome will automatically group similar tabs to help with multitasking * Custom themes: Users can generate unique browser themes using text prompts and AI image generation * Writing help: Chrome will offer suggestions to help users draft messages and posts on websites - They are currently only available to US users who opt-in on the Experimental Features page I think this development is super super important because making AI accessible via the incredible Chrome platform to billions of people, is going to put Gemini in front of grandmas, students, everyone. Qutie impressive and the compute needed to pull something like this off is also quite mindboggling! 👏 Of course, they are not the first browser to add AI, I love the Arc Browser and it has AI previews that I use quite often! This weeks Buzz (What I learned with Weights & Biases this week) Have you like many of us have trouble getting structure output (JSON, other stuctures) from LLMS? Jason also had this problem, that's why he authored the Instructor Library, which makes it easy to guide the LLM to give structured output using Pydantic. Jason has presented at Ai Engineer conference, and recently collaborated with Weights & Biases to launch a free course in how to guide your LLM to give structured outputs! Jason is also an independent consultant working with companies on their AI implementations and has many battle tested examples from implementations across the board, which he shared with us on the pod. Give this short course a try if you haven't yet, it's really high quality content, in addition to tons of other stuff we have there, for free 👏 Voice & Audio 11Labs has a new overdub studio and it's really working well Check out this short segment of myself, speaking in dubbed Russian! It’s really sounds like me, sent to my mom to see if she falls for it 😆 She didn’t AI Art & Diffusion Hourglass Diffusion Transformers New high resolution diffusion architecture from K-diffusion and RoPE team (X, Blog, Paper, Github) Paper presents a new method called HDiT ( HourGlass Diffusion Transformers) that shows promise in training models with high resolution images without incurring the significant hardware costs that go with scaling image sizes, replaces the latent diffusion models enabling O(n) complexity and scaling well. Utilizing tricks and best practices for transformers architectures, like RoPe (that we've covered on ThursdAI before) cosine similarity self-attention, RMSNorm, GeGLU, etc. and using something called local self attention, this paper shows incredible promise for high resolution architectures for image creation tools. We had the pleasure to host Tanishq Abraham, one of the co-authors (and CEO of MedArc, Director of research with Stability + PHD at 19) to walk us through the paper, explain the problem and the solution. Additionally, friend of the pod Enrico Shippole is co-author as well 👏 and Alex Birch joined us silently from the audience 👂while giving commentary in the group chat. P.S - All of these co-authors attribute the bulk of the work to Katherine Crowson from k-diffusion 👏 Tools & Others Prophetic introduces Morpheus-1 - multimodal foundational model trained on fMRI and EEG signals In a breaking news fashion, the folks behind Prophetic, a new startup that just announced MORPHEUS-1 as we were hopping into the space, came to chat with us. They are working on a new multimodal ultrasound transformer! That's right, multimodaliy is not only about images/text folks, we've covered this before but these chads are actually trying this out, they have trained a transformer architecture to take EEG and fMRI signals and output directions for the ultrasound to activate areas of the brain to induce Lucid dreaming. And they are asking for beta testers! It's all quite futuristic, and if you're in NY, reach out to them (and then let us know if you had Lucid dreams!) Definitely worth a listen on the pod and check out their video announcement for mode details, was really quite an incredible conversation with Wes and Eric. National Science Foundation launches NAIRR pilot (Blog) Partnering with 10 other federal agencies as well as 25 private sector, nonprofit and philanthropic organizations, the NAIRR pilot will provide access to advanced computing, datasets, models, software, training and user support to U.S.-based researchers and educators Basically, this is a huge governmental endeavor to provide resources about AI, make sure companies collaborate and keep AI accessible across the board and tons of government agencies as well as private sector companies have joined hands in this. Just look at this list, it's a veritable who & who of AI in US (notably, Tesla/X is missing) And that’s all folks, that’s all she wrote (or I guess, I wrote) today! What an incredible show, really thankful for folks who came out, guests and co-hosts and see you next week! If you scrolled all the way to here and want to show me that you did, your emoji of the week is 🍊 (only cause persimmons don’t have emojis) so DM or reply with this and share this pod with 1 friend or tag us on social media! Full Transcription below: transcript [00:00:00] Alex Volkov: right, folks, it's time for the sound. Let's get it started today. [00:00:11] Alex Volkov: Welcome, everyone. Welcome to [00:00:13] Alex Volkov: this live recording of ThursdAI, the Twitter space, podcast, and newsletter that brings you. everything that happened. the AI world, every Thursday, literally almost every Thursday. My name is Alex Volkov, an AI evangelist with Weights Biases, and [00:00:33] Alex Volkov: this is ThursdAI [00:00:37] Recap & TL;DR [00:00:37] Alex Volkov: Alright, recap, here we go. Taking a deep breath. We've talked about incredible amount of stuff here on Thursday AI for January 24th. We've talked about the areas of open source LLMs was very interesting. We've talked about stability AI, releasing a stable LLM, tiny version, 1. 6 billion parameters. That's really good at different languages, the European languages as well. [00:00:58] Alex Volkov: And it's not commercially viable. For open source, but it is under the stability membership. So if you have that's a great model for you. We've talked about Intern LM2 for a state of the art on math LLMs. We briefly mentioned this, but it's getting 90 percent of GPT 4 performance on math, which is, was quite incredible. [00:01:16] Alex Volkov: We also had the pleasure of Tanishq, Abraham to join us from MedArk for the analysis of open source models as it relates to the medical field. And it turns out that the model called Quen72 from Alibaba, Quen72 is the best open source doctor that we have achieving like incredible and beating even MedPalm1, which was back then by Google trained as one of the best medical LLMs. [00:01:42] Alex Volkov: We also. were a very multi modal heavy space today like a lot we had the like we had the folks from Prometheus lab join us and talk about their multi modality which is not Trans, which is transformer based, but not LLM based so their multimodality is EEG signals and fMRI signals as they work on hyper focused ultrasound to induce a lucid dream state in your brain. [00:02:11] Alex Volkov: Their multimodal model is basically taking inputs from EEG and outputs in, in the directions or where to focus this ultrasound is super cool. And I definitely advise you to listen to them. It wasn't planned. I just saw the post. I just commented, Hey, we're going to talk about this. They jumped on Prometheus looks like a cool multimodal attempt, nothing to do with vision, but also we talked about vision multimodality as well. [00:02:34] Alex Volkov: So we've covered Adept the company who was founded by a few folks from the original Transformers paper and they have previously released. Per semen models. And then EU eight B was a multimodel that did not use a vision encoder like a different architecture. They released an announcement. They didn't release any code or weights or the way for us to try this yet, but they released something called Fool You Heavy, or they announced something called FU You Heavy, which is an extension of the previously released fool you eight B. [00:03:00] Alex Volkov: Significantly more trained. And they talked about how difficult it is to train multimodal models and they claim to have a third. Place in the world after GPT 4 and Gemini Ultra on a bunch of the multi modal metrics and evaluations like MMU and MMLU. They also talked about the process, how difficult it is to train these models at scale. [00:03:20] Alex Volkov: So cool from Adept and we're waiting for some ways to test this. We also talked about fire lava, which is, if you remember, we've talked about lava before multiple times. Lava is a Open source way to train models in multimodal and like Baklava from Focus on Stage here, Nissen and Farrell, and Obsidian from LDJ who's also on here and also Moondream. [00:03:39] Alex Volkov: Like all of the things we've talked about are based on Lava. Lava was not commercially permissive licensed because of the data set. Fire Lava decided or released the first Lava model with commercial permissive license from Fireworks AI. And we also had it. Quite an interesting chat with Vic, who is the author of Moondream 1, which is a tiny 1. [00:03:59] Alex Volkov: 6 billion parameter vision language model, also on top of Lava, that has Phi 1 as 1. 6 billion. The foundational kind of brain, the LLM brain in it the conversation with Wick was very interesting. So shout out Wick. Thanks for coming up. Specifically because he also mentioned that Phi 1 Microsoft, if you guys remember Phi 2 was MIT licensed back in December. [00:04:20] Alex Volkov: It was a surprise to all of us. And apparently they went back and also changed the the License on Phi 1, which is super cool, and Vic told us that he saw this. So Moondream is a very capable, very tiny vision model that works quite well. Definitely worth listening to this conversation with Vic. [00:04:36] Alex Volkov: We also announced in the This Week's Buzz category of ours, or segment of ours, about Everything Weights Biases, we announced a new course in our academy from Jason Liu, the author of the Instructor Library. And he has a course now that was released today called LLM Engineering Structural Outputs. [00:04:54] Alex Volkov: And as Nissen , pointed out a bunch of the folks in open source are learning from these free YouTube videos and definitely worth checking out Weights Biases Academy because there's a bunch of knowledge there. And it's all for free and just join and just register. It's super, super cool. And then we had an incredible honor again of having one of the authors of this paper. [00:05:12] Alex Volkov: As always, I love when we discuss stuff and the authors of the stuff come to chat with us. So we had Tanishq Abraham. But also we had Alex Birch in the audience listening to us while he was working and sending us DMs from the new paper called Hourglass Diffusion High Resolution Image Synthesis. [00:05:30] Alex Volkov: And this paper will be in the show notes and Dinesh went through the kind of the in depth of the problem he tries to solve. And they. They talked about integrating transformers and diffusion models previously to separate areas and they haven't came up with the first one, but they definitely used a bunch of the techniques to optimize transformers into the diffusion world and create a pixel space, high resolution image synthesis, which is, shows great promise going forward. [00:05:59] Alex Volkov: Incredibly insightful conversation from Tanishq, definitely worth a listen. We also covered in this area, we covered Instant ID, which is a one, one shot or zero shot face transition into diffusion models. So you can upload one picture of yourself and get quite incredible results in image diffusion. [00:06:17] Alex Volkov: Or like generative images with your faces or your kid's faces, which is super cool. I haven't tried my cat. I don't know if it like works on cat's faces. I'll try it out. We covered a new, a state of the art. Automatic speech recognition system that beats Whisper or at least runs 30 times faster than Whisper on different tasks. [00:06:36] Alex Volkov: We're going to add this to the show notes as well. And a little bit about deepfake audio with 11 labs have a dubbing studio released. And some conversation about whether or not or how it already affects politics. And then the last thing we've covered is the National Science Foundation, NSF, announces a new partnership from all major labs and government agencies around AI, and includes DOD and DOA, and includes OpenAI and Tropic, includes open source folks like Hug and Face, and MetaAI is also participating in this. [00:07:11] Alex Volkov: And also Ways and Biases is part of that huge partnership, governmental partnership. So I think this is all the stuff that we've covered in this space. [00:07:19] Show starts with house keeping and structure breakdown [00:07:19] Alex Volkov: We have quite the show for you today, and as always there's no boring weeks in AI, is there? And some weeks start slow and then pick up, some weeks start Crazy from the get go. If you remember, there's one week where one Friday had a bunch of releases, and this week we had a very full week, full of very cool innovations, but also exciting stuff. [00:07:47] Alex Volkov: And then we have some authors of those stuff here with us today, and we're gonna talk about a bunch of multimodality, which we've been talking about for a while. Obviously the space started with the multimodal GPT 4 and then we just kicked it into high gear. I think that it's time to get started with our default segment. So for those who are new to Thursday AI, we usually segment this to five or six segments, the biggest one being open source LLMs. And then we have big companies LLMs and API. So we usually cover the Google stuff and OpenAI stuff. [00:08:18] Alex Volkov: Mistral has been here and there, been [00:08:20] in the open source, now is the big company as well. So depends on what they release, that's where Mistral stuff falls. And then we talk about vision and video, which is Basically, we'll recover the multimodality stuff and that section is going to be the, I think, the main one today. [00:08:36] Alex Volkov: There's so much stuff. It's crazy. We also have tthis com this corner I call This Week's Buzz. I feel like I have to explain this. Maybe people don't get this dad joke that I put in there. Buzz, as in bees, right? So bees, Buzz. And Weights and Biases, the shorthand for Weights and Biases is WandB. [00:08:54] Alex Volkov: Weights and Biases, W and B. And for a very funny reason, there's a mascot of ours that's a bee that's holding a wand, because it's WandB. And like this little joke has been Prevalent like in many places. I think I haven't explained it yet. And so this week's buzz is actually the corner about everything that I've learned with Weights Biases every week. [00:09:13] Alex Volkov: And so this corner we're going to chat with Jason and announce some cool stuff. The next corner we have is voice and audio, which we usually have a bunch of stuff. We have VB from Hug Face usually join us. He's like the AI audio person over there. There's not a lot of voice and audio stuff. [00:09:29] Alex Volkov: So I actually don't have anything voice and audio related in my notes. However if you guys know like very cool things that happened. This week with voice and audio, please let me know, we're going to talk about them. We're going to move to AI art and diffusion in the next segment. We're going to talk about some cool things there. [00:09:45] Alex Volkov: And then the last segment is like a free for all, it's tools and others. So I usually put agents in there. I usually put like super cool things. So I have two, two, two exciting things to talk about there. So this is usually the structure. [00:09:58] Nisten Tahiraj: I do have, is one more thing there, and it's the W2V, the BERT speech encoder. think it's for meta, and it's about, it's supposed to be like 30 times faster than than Whisper. So yeah, it's another very efficient automatic recognition ASR model. So I'll I'll post it in the links [00:10:20] Alex Volkov: And I think also we had 11Labs announce like a yeah, I had a tweet about actually ThursdAI Content, that I spoke in English, obviously, and then I asked it to translate to Russian. We'll cover this, 11Labs has a dubbing studio. [00:10:33] Alex Volkov: . [00:10:33] Open Source LLMS [00:10:33] Alex Volkov: And then, let's go to open source, folks. I think let's go to open source. [00:10:55] Alex Volkov: All right, let's start with our open source segment here. And I think the first thing we should probably quickly mention is our dear friends at Stability AI, folks who've Made a dent on the industry with Stable Diffusion, obviously but they're training a bunch of other stuff. We've talked about multiple stuff they did. [00:11:12] Stable LM 1.3B [00:11:12] Alex Volkov: We've talked about Stable Video Diffusion and like how open source lags behind closed source, but not by that much. And Stability released a new LLM, which they had the Stable LLM before, I think, Nistan, have you used Stability stuff before? For the LLM stuff? [00:11:31] Nisten Tahiraj: I have Months ago, so I'm not up to date on [00:11:35] Alex Volkov: Yeah, so [00:11:36] Nisten Tahiraj: used it on Google collabs and [00:11:37] Alex Volkov: Yeah, so they're not like, they haven't changed the industry in the LLM world as much as they have in the image diffusion world, for sure. However, there's a big however, they're working on multiple fronts. And it looks like, I had a chance to actually chat with Imad for almost 20 minutes. [00:11:52] Alex Volkov: Imad is this like very incredible person who knows a lot about a lot. And it's like the conversation there is like basically a stream of consciousness conversation, which I had. No trouble in following up because we talk about everything here on ThursdAI. But the folks who were with me and talking to Imad, they looked at me and was like, How do you know all this? [00:12:11] Alex Volkov: And I'm looking at Imad and was like, How does Imad know all this? That's what happens when you're on stability. So they released they're training a bunch of different models. This week they gave us Stable LLM, which is a tiny model, 1. 6 billion parameters model. It's really we've been saying this previously. [00:12:24] Alex Volkov: It's really funny to say small LLM, right? If you expand the LLM abbreviations, like a small large language model. But this one is tiny. It runs super fast on, on multiple devices. I think their point is to actually like edge device running. So obviously we've covered multiple small. LLMs before, we've covered PHY, if you remember PHY 1, we're gonna talk about PHY with Vik in a second. [00:12:47] Alex Volkov: We also talked about like PHY 2, I think there's like a few others StabilityRelease, there's It's pretty good. It's pretty good. I was itching to play with this, they released a GGUF. Apparently I dunno if you knew this name, but apparently stability has their own CPP and their like GGF file, which is like a, for those who are not following all the AT acronyms. [00:13:11] Alex Volkov: GGF is a quantized version of models. So apparently stability has, like stability. CPP is incompatible with Lama cpp . And so apparently Elm Studio had to add a specific support for this and they did. And so if you wanna play with stability, AI. Stable LM, now you can , with LM Studio, and LM Studio at least in my experience, gave me ridiculous performance. [00:13:34] Alex Volkov: I got, on, on this Macbook M3, M3 Max I got more than 130 tokens per second, which was like ridiculously fast. And the model was fairly capable for a small model. I was very impressed. So if you want to play with a small model, you want to do some stuff with this, stability is definitely an interesting one. [00:13:53] Alex Volkov: Support in Elm Studio. Yeah, go ahead. [00:13:56] Nisten Tahiraj: yeah, it's a 1. 6B. So in that means it's 1. 6 gigs to run at eight bit without losing much accuracy. However, the, that means that it has a lot more applications for tiny stuff, because then you can get that down to 800 megs. And so on. So this is people did find some issues. Again, it's a tiny model, but they found issues with it being able to continue the conversation. [00:14:24] Nisten Tahiraj: However, for one shot answers, it was extremely capable. So just keep that in mind when using it. It is probably right now the best model for that size. Just keep in mind if you're going to do something with it. Don't expect much in terms of follow up stuff. Just if you can do it in one shot, great. [00:14:48] Nisten Tahiraj: Use that. And yeah that's about all I have to say. [00:14:51] Alex Volkov: Yeah. And additional things that it punches above its weight on other languages. So if you folks remember when we talked about Mistral, for example, getting compared to open the eye on Tropic, et cetera Mixtral medium, the model is like specifically for the German, the European language, the German, Spanish, French, Italian, all those it's significantly better. [00:15:11] Alex Volkov: Stability is also playing in that market looks like for the smaller size. And so this. Out this tiny model beats the five versions of three billion parameters. So it beats models twice its size, even some seven billion parameters, specifically for , European languages, [00:15:25] Alex Volkov: and if you remember, we've talked about MPT from Mosaic, was that? Yeah. So this model beats the Mosaic MPT 7B, which was probably back in May was like the coolest like open source model. So that was 7 billion. This beats that on empty bench and everything. [00:15:40] Alex Volkov: It's quite incredible. It beats Falcon 40B. It's really, the speed, the reason why we bring you these models is not only Hey, use this one. Because Nissen said this one may not be exactly good for your commercial stuff. Also, it's not really commercially viable. There's a specific stability license that you have. [00:15:58] Alex Volkov: Stability membership, they call it. They have to apply for stability AI membership. And then based on the size of your business you're able to use, they have to make money somehow. But we bring this to you also to show that how fast we're moving from a 30 billion parameter model to a 77 billion parameter model, and now to a 1. [00:16:13] Alex Volkov: 6 billion parameter model, that compresses like incredible amounts of trillions of like words from the human knowledge into just, listen, do we say like this can go down to like less than a gig, right? If we look super quick, [00:16:28] Nisten Tahiraj: Yep. At 4 bit, it should be 800 So we're getting to the point where they'll just fit in a Raspberry Pi Zero with 512 megs and they'll be conversational [00:16:40] and useful and even multi modal. So we're almost there. [00:16:43] Alex Volkov: Yeah, it's quite incredible. And then, okay, so this is stability stuff. Meanwhile, I'll say hi to a new guest of ours that I just saw on my timeline. [00:16:51] Prophetic announces MORPHEUS-1 an EEG/fMRI multimodal to induce lucid dreams via hyperfocused ultrasound [00:16:51] Alex Volkov: What's up Wes, how are you? [00:16:53] Wes Louis: Hey [00:16:54] Wes Louis: guys, how are you? [00:16:55] Alex Volkov: Hey. Hey welcome. Folks maybe saw my tweet, maybe didn't as that I love planning for Thursday, but I also love breaking news. As I was planning, I was going through my feed, and thankfully my Twitter feed is back at his own, like giving me the best AI stuff. And Wess and I think your co-founder is also here. [00:17:10] Alex Volkov: Eric, yeah. Let me add you real [00:17:12] Alex Volkov: quick. I didn't plan on this folks. I just literally just like tagged and they came. The video that you guys posted came through my timeline and I would love to go and give you a stage for a minute or two to explain what prophetic is because the transformer stuff that you discussed with the EEG and fMRI signals, I really dig. [00:17:30] Alex Volkov: Could you summarize that video for us for a brief, like two sentences? That would be super cool, I think. [00:17:38] Wes Louis: So [00:17:38] Wes Louis: this has been something we've been working on for a while. [00:17:40] Wes Louis: It's really a, essentially, [00:17:42] Wes Louis: a multimodal transformer model that is designed entirely for neural data. And so basically, what we've done is, we built a data set of EEG and fMRI and, what we're designing is a neural simulation device to basically induce lucid dreams. [00:17:59] Wes Louis: And so we build the data set on heightened prefrontal cortex activity. This is, the neural correlate of lucid dreaming. And we basically built a model where you prompt it with your current brain state. We have a set of sensors on the device, and then we output targets for the neurostimulation. [00:18:17] Alex Volkov: That's quite incredible. So for folks in the audience, we talk about multimodality often and oftentimes we just mean VLMs, like we mean like vision and text, which we're going to cover like a bunch today. But today I think the highlight of today's Thursday is multimodality applies to many things. So you guys are, your multimodality is not even there's no text in there at all, right? [00:18:36] Alex Volkov: This is just EEG signals and fMRI signals. Is that correct? [00:18:41] Wes Louis: Yeah, it's purely prompted with EEG. And one thing I'll say is, everyone talks about multimodal. And, so you're using, let's say, an LLM, and you're prompting it with a photo, for example. This is similar in many ways because neural imaging data, particularly EEG, is you can nicely get, you can get it into, it's a neural image you can get it into an image format. [00:19:02] Wes Louis: And then prompt the model that way, but then on the generation side of things that's entirely, we use a pretty unique fMRI embedding process that we've come up with ourselves and ultimately the idea there is that you take this heightened neural activity, And those are candidates for targets for nerve simulation. [00:19:20] Wes Louis: And, we [00:19:21] Alex Volkov: What do you, sorry, what do you mean, what do you mean by targets for folks who have no idea what this means? [00:19:26] Wes Louis: Yeah. We're using this is the other big technology that makes all this work is FocusUltraSound. FocusUltraSound, for those that don't know, is this Really, cutting edge neurosimulation technique that can get, quite deep into the brain, other techniques, people who may be familiar with, direct current, alternating current, really get soaring to the surface. [00:19:47] Wes Louis: Of the brain, whereas focus ultrasound can get quite deep, but there's also this ability to steer the beam and also create acoustic holograms. And so when we think of heightened neural activity it really takes the form of these 3D figures. And the idea being that we can create these outputs of fMRI targets and then translate those over to the focus ultrasound. [00:20:12] Alex Volkov: This multi modal transformer takes on the input EEG signals, and on the output it prints out those targets. Those are targets for this technology to then stimulate the brain to go into a specific state. [00:20:31] Wes Louis: Yes, and all of this is closed loop so in that, once you create the simulation, the model is prompted again with the current brain state and this is continuous. Process of learning and figuring out what sets of tokens lead to this heightened state and that heightened state is really identified as gamma frequencies and that's really the fastest band of activity. [00:20:53] Wes Louis: So it's this continuous process until someone gets to a lucid state. [00:20:58] Alex Volkov: That's quite incredible. So you guys announced the LLM today, but it's not like you're not releasing the open source. This is just an announcement of your efforts, correct? Anything else you want to add here? And I think you started talking about folks can join the beta if they want to. [00:21:12] Nisten Tahiraj: Yeah, that's what I [00:21:12] Wes Louis: would point out is that we have a beta program that, that this is really the purpose of this announcement is we're looking for people to sign up. We've had 200 or so in the last, Two hours. And so this spring we'll have it working. And if you're a New York based or you're willing to come out to New York we'd be, more than happy to have you test out the product. [00:21:31] Alex Volkov: That's awesome. Congrats folks. Actually, you want to add anything? [00:21:33] Eric Wollberg: Alex. Hey, how's it going? This is Eric. I'm a [00:21:36] Alex Volkov: Oh, Eric, yeah. [00:21:37] Eric Wollberg: with West. Yeah. Hi thanks for doing this. Yeah, one thing that's just I think, the sequence of how we've released these things, we showcased in October our prototype that we designed with Card79 notably did, Neuralink for Elon, and then we, Also worked with Max Hodak at Science. [00:21:52] Eric Wollberg: Max Hodak used to run Neuralink for Elon and then spun out Science. So really top consumer VCI kind of design folks. And so then now we have this model, right? This ultrasonic transformer where now we're going to be migrating that on to, the technically working prototype and beginning neuromodulation. [00:22:08] Eric Wollberg: So that's what the beta user program is all about. We've got, yeah, like 225 people signing up in the first two hours we're really looking for we're excited to have people on board and begin to do this you have an opportunity if you're, especially if you're early up on that list to be the first person to achieve an ultrasonically induced lucid dream, which is You know, I think it's going to be a pretty watershed moment. [00:22:28] Alex Volkov: That's super cool. I've tried to, to lucid dream a lot of times in my life and I never actually got to a stable one. So I'm excited to follow you guys, but also excited from the technology application of this, because we talk about transformers and a lot of this is going to LLMs. [00:22:42] Alex Volkov: Now we're going to, this week we're going to talk about Transformers as applied to the fusion models as well. And here you are like doing like full multimodality out, out of the left field. So I love it. And hopefully you guys will do some cool things and keep us up to date and welcome to, to join on Thursday. [00:22:55] Alex Volkov: I, to talk about this. [00:22:57] Nisten Tahiraj: Awesome. Thanks, Alex. Thank you, Alex. [00:22:58] Alex Volkov: Thanks for hopping on, folks. And as folks, as I love breaking news here on Thursday. This is like a tiny breaking news. Thank you, Wes. Thank you, Eric, for joining folks. If you want to try, the future, sign up for the beta, because why not? [00:23:09] Alex Volkov: And I think it's it feels like non invasive, right? You put this headset on, and then hopefully you go to sleep, and hopefully you're able to control your dreams, which is like what Vision Pro will do for outside world, but this is like inside your dream, it's super cool. All right, let's move on to, I think we're moving on to the big, no, actually we're moving on to the big category for multimodality as we're already here. [00:23:33] Alex Volkov: Vision and video and multimodal, or at least VLM multimodal. [00:23:38] Adept teases Fuyu Heavy, their flagship multimodal catching up to Gemini Ultra and GPT4V [00:23:38] Alex Volkov: I'm gonna start with the big dog here, ADEPT. If you guys remember ADEPT Labs were co founded by a few folks from the original Transformer paper. I don't think they're no longer there, but I have to, I feel like I have to add this. [00:23:52] Alex Volkov: Prefix every time we talk about adept, adapt released a few models for us. If you guys remember, Persson was a seven B model or eight B, eight B it was weird, but they released an 8 billion parameter model. It was like very interesting back then. They also then on top of this released fio, which is persson is the type of fruit, F is the type of tree that persimmon grows on. [00:24:10] Alex Volkov: So we see you adept, we see your jokes here. Also. I love the LLM naming and then they raised Fuo back then. And FIO was. Interesting from the perspective of it didn't use a vision encoder, it did something else. It was very interesting that their approach to vision models allowed them to use Non standard image sizes, because they didn't train it on such a thing. [00:24:31] Alex Volkov: So back then, that was what was interesting. And now, they've announced, they haven't released anything. They haven't said, hey, here, use this. I wasn't even able to use this. But they announced Fuyu Heavy. Fuyu Heavy, according to them. And so far, Adept have been trustworthy enough for us to trust. [00:24:48] Alex Volkov: What they say this is the third in the world multi modal or I guess VLM. So not multi modal like, like Wes and Eric just told us, but a multi modal in the sense of like images plus text together. This is the [00:25:00] third in the world model behind GPT 4 Vision and Gemini Ultra. Which Gemini Ultra we haven't yet tried, obviously, we don't have access. [00:25:08] Alex Volkov: If you have access in the audience for Gemini Ultra, and you want to help me, help a brother out, let me try and play with this, please let me know. But so they're announcing, AdeptFuyu is announcing that Fuyu Heavy, their model, is 20 sizes smaller than GPT 4 Vision. I have no idea how they even know what size GPT 4 Vision is. [00:25:28] Alex Volkov: They say that around 20 to 30 sizes smaller. And comes very close in the multimodality stuff. And they talk about the challenges of creating like large multimodal image based model. The challenges are stemming from there's not a lot of assets properly to test. There's not a lot of the tooling instrumentation stuff are really hard for images as well. [00:25:47] Alex Volkov: And so they announced this they showed some very incredible performance. And I will remind folks that Adept specifically started with tools to make you run your computer. So their models are specifically tuned on UX, UI and web stuff. And expecting to hear more from them and finally getting to play with this. [00:26:06] Alex Volkov: Go ahead, Faro. [00:26:09] Far El: I just [00:26:09] Far El: want to say that, [00:26:10] Far El: Demos are easy. I'm going to take it with a [00:26:14] Far El: grain of salt until I actually see the model or are able to test it. The thing is that there is no indication of actual like speed of the inference or whether these examples were cherry picked or not, right? There's a lot of question marks about this, especially when you just come out and, make a marketing announcement without actual access to the model. [00:26:37] Far El: Yeah, it looks cool, but I'm not, I'm not hyped just because it's not like it's not verified or validated [00:26:43] Nisten Tahiraj: in any way. [00:26:44] Alex Volkov: Yeah, I'm with you, I'm with you. Specifically I will say though, about Adept specifically, we've seen stuff from them, we've seen papers from them before, and they did, folks started asking like, Hey, where's the weights? Where's the weights? And they did say that, stuff is coming, but they want to like, keep a competitive edge. [00:27:00] Alex Volkov: But we see, we've seen like at least a new architecture from them, if you remember with Fuyu. And so we know [00:27:05] Nisten Tahiraj: Oh, of course. [00:27:06] Alex Volkov: yeah, the Fuyu architecture is legit, like they literally was able to. create a multi modal without an image encoder thing back then. We're definitely going to listen to this. But based on the metric that they released, if this actually performs as well on MMMU, which is the kind of the equivalent of MMLU. [00:27:25] Alex Volkov: For multi modal stuff it's going to be very exciting their heavy model, definitely. [00:27:29] Fireworks releases FireLLaVa with a fully commercially viable license [00:27:29] Alex Volkov: Moving on, actually, Pharrell we'd love to hear what you think about this. And actually, Vic, this is wrapping you up to the next conversation. Fireworks AI that I haven't actually used, but they released the first Lava model with commercial permissive license from Fireworks. [00:27:43] Alex Volkov: So Lava was released. Lava, we've talked about Lava is the architecture. That allows many of these models to be trained in a multi modal fashion, correct? Lava was released, it was not with a commercial license because it was trained on a bunch of I want to say that wasn't marked for commercial and open source licensing. [00:28:01] Alex Volkov: So a lot of these models that we get, we cannot actually use in production. And FireLava announced that like their first Lava model was commercially permissive licensing. And I think that's super cool because finally folks will be able to build this. And as a reminder, Lama, the LLM was released without commercial license. [00:28:19] Alex Volkov: And then Lama 2 released with commercial license and then incredible amount of stuff started happening because companies who wanted to use this in production actually started like looking into this and using Lama 2. And so hopefully the same will start happening with FireLava. I actually am not sure if they released the weights. [00:28:36] Alex Volkov: I think they did. Yes, they released the weights on Fireworks AI, FireLava 13B on HugInFace. And yeah, listen, go ahead. You guys trained stuff on top of Lava. So please, first of all, introduce the stuff that you've trained on and then also like comment on the ability to use this now in production. [00:28:56] Nisten Tahiraj: Yeah, I just want to say that The entire vision open source vision field, and non open source, it is extremely competitive right now. For example, here, we've released Baklava, which is bak lava. Again with the naming. So that that was three months ago. Also LDJ here made the obsidian, which is like the three B one, and then they made A seven B as well. [00:29:22] Nisten Tahiraj: We also have the dev lead of Quinn. He was in the audience as well, so they made the Quin 14 b vl. And this part is, oh, and we have Vic as well, who also made a very fast. And a small model recently. And Valkylava was being used as a benchmark, which was pretty interesting, actually. Yeah, the Vision LLMs are extremely competitive right now, and I think it's one part where open source can really surpass what you get from from any from any API, because it's something you can run local on the device and you have full control over. [00:30:01] Nisten Tahiraj: So the interesting thing yeah, as for Fireworks 13b, that's still Lama 13b base, as far as I saw, and I tried to use their inference on their site, but it wasn't working, and I can't complain too much about it, because ours is not working either. That's why I wasn't using WSGULAG yeah, also to comment a little bit on Fuyu, because I do like their trying a completely new approach. They don't use stuff that's similar to clip image models, which is what everybody else uses. They do something where they take, I think, groups of pixels or stuff. They serialize it, so the image is just being represented as just another string of text or a string of tokens. So they can scale. [00:30:48] Nisten Tahiraj: To 8k, 16k, whatever you have, they don't have, they don't have that limitation that others have in, in terms of architecture. So it is good to see that approach is working overall, whether it will be competitive we'll see. So yeah, I wanted to comment on that. But yeah, I haven't actually tried the Fireworks model itself, but I did see, again, the architecture is similar to also Lava 13b. Yeah, that's about all the comments I have on that. [00:31:22] Alex Volkov: And like you said, interestingly, it's still based on Lama, right? And it's time for, it's time for new things. And I think this takes us to the next topic of conversation. And again, Vic, I want to introduce you properly this time, or at least let you introduce yourself. [00:31:35] Moondream1 from Vik Hyatk - 1.8B VLM [00:31:35] Alex Volkov: But the next kind of iteration or of our conversation about multimodality, like we said, today is a multimodal space is the existence of like very tiny vision models, vision, large language models, or a large multimodal model, it's really hard to like, name these things. Vic, welcome to the space, this is your first time, please introduce yourself and then let's talk about Moondream a little bit. [00:31:57] Vik Hyatk: Hey folks hey Alex, thanks for having me. Super excited. My name is Vik. I'm pretty new to the AI space, I think. Like a lot of people, I got into it when that big stable diffusion moment happened. And I was like, this is what I need to spend my life working on. So I went out, bought a workstation with 3090 and started playing around with stuff. [00:32:15] Alex Volkov: You and me both brother, you and me both. And, okay. So the reason why you're here and the reason why I'm , calling on you in the vision and video area is because of Moon Dream one. You, can you introduce Moon Dream one a little bit to the audience? [00:32:29] Vik Hyatk: Yeah so it's a small language model. It's about 1. 6 billion parameters. It's built on top of Siglip from Google or DeepMind. I forget which one of the two. Trimil, because that's the vision encoder and it uses 5. 1. 5 as the text model, and then it's trained using the standard lava. So super thankful for the folks that worked on these projects amazing models they've put together. [00:32:52] Vik Hyatk: It works. I'm tooting my own horn a little bit here, but it's surprising. I see people post screenshots of them asking questions and it still blows my mind that it works that well. [00:33:03] Alex Volkov: I let me talk the horn a little bit because I definitely tried out. Thank you for the hugging face. How can I say, space that you put up like super quick, and the next follow up is going to be about how to actually use this, but this is based on Lava, so the same non commercial license, correct? [00:33:19] Vik Hyatk: [00:33:20] Correct. The top piece of feedback I've gotten from people is that they want to see this with a commercially permissive license. I'm working with, working on that. The FireLava folks didn't release the dataset, but thankfully they did talk about their process to create the the non encumbered version of the dataset. [00:33:37] Vik Hyatk: So I'm working on it. I'll have that out in a couple of days, the dataset at least, and then we can start training models that aren't encumbered like that. [00:33:44] Alex Volkov: Incredible. And so the next thing that I wanted to talk to you about is PHY 1. So PHY is from Microsoft. PHY 1 was not released with a commercial license. We remember it was trained on synthetic data in tiny stories, like a tiny 1. 6 model. So we saw a few releases since then. So obviously we talked just now about StableLM. [00:34:01] Alex Volkov: Semi commercial, if you're a part of their membership, and also Phi2 was MIT license. It's a little bit bigger. It's three, I think, billion parameters. Have you tried with Phi2 and could you speak about that experience? [00:34:14] Vik Hyatk: Yeah, I I did actually. So I was initially working on training Moondream 1 with PHY 2 once it came out. There are some issues with fine tuning it when you have flash attention on I believe. And so it just takes a lot longer to train. So I went back and looked at PHY 1. 5 and I saw that they updated the license for 1. [00:34:32] Vik Hyatk: 5 to MIT as well. [00:34:33] Alex Volkov: Oh, really? [00:34:35] Vik Hyatk: stick with what works. Yeah. [00:34:37] Alex Volkov: Wow. I did not know this. So it actually updated the license backwards. [00:34:42] Vik Hyatk: Yeah, on the Hugging Face page, at least it says MIT now. [00:34:45] Alex Volkov: I love it. Like it would make sense, right? But folks, I don't think we've talked about this. So like breaking news here. Thanks, Vic. Phi 1 is also, we'll check this. We'll double check, [00:34:55] Nisten Tahiraj: Also three. They're both MIT licensed now. So whatever pressure we put on Microsoft's Azure side, it worked. [00:35:03] Alex Volkov: nice. That's incredible. All so now, so this part of your stack of Moonbeam is now MIT licensed. So Lava is the only thing that's holding this back from being used in [00:35:14] Vik Hyatk: Just the [00:35:14] Unkown: data set, yeah. [00:35:16] Alex Volkov: The dataset. Okay. Okay. So definitely there's work being done there. I will just pay send folks attention to the nest, to the top of the space where I had my tests. [00:35:25] Alex Volkov: I literally just pasted an image. And again, thank you for the demo, Vic. Folks will get the demo in show notes as well. I pasted an image of two of my friends just sitting and talking across like a TV with some things. Literally the model said, image features two men sitting in chairs engaging in conversation. [00:35:42] Alex Volkov: One man sitting on left side, one other on the right side. That's obvious, but still cool. They're both looking at a laptop placed on the table in front of them. The laptop is open and displaying a presentation. Possibly related to their discussion. So this feels like hallucination a little bit because the model does not know what it displays, but fine. [00:35:57] Alex Volkov: And so in the background, there's a TV mounted on the wall, a cup that can be placed on the surface nearby. The scene suggests a casual collaborative environment. This is ridiculous. This is like a super tiny model and it outputs this scene almost perfectly. And. I've tested like the same image in different, like a bigger, GPT 4, it pretty much gives me the same information. [00:36:17] Alex Volkov: So I was really impressed. So Turing the Horn, for sure, because the tinier the model is, the better the utilization. And we've talked about different vision enabled hardwares that are possible or not possible based on whether or not they're going to be able to run stuff on like Raspberry Pi. And, the smaller these models, the smarter they are, the better we'd be able to use them in cheaper hardware. [00:36:40] Alex Volkov: Really impressive. What are you planning to do with this? Like, how has the community accepted this? What type of conversations did you get into? And what are you planning to do next here? Besides training the [00:36:51] Vik Hyatk: I was blown away by the reception to this. I've, when I put it up, I thought like maybe it might get like a hundred likes or something and then I'd move on to my next project. But I've seen a bunch of super cool demos. Come out of this, I think the fact that it is small and it runs inference so fast makes a lot of use cases that were previously not possible, a lot more viable, like captioning a video in real time or recaptioning a billion images and whatnot. [00:37:15] Vik Hyatk: There's a couple of things I'm working on. Obviously the top thing is like getting it to a permissive license. I also, I could use some help on a couple of fronts. So I do want to make it easier to run, get gguf, olama integration and whatnot. [00:37:30] Alex Volkov: Definitely LM Studio integration. I would love To play around with this with Elm Studio, just to see how fast this is, this runs on my software. MLX would be a cool suggestion as well the community is very excited about MLX, I don't know if you saw. But Elm Studio is a friend of the pod, definitely it's connected to YouTube. [00:37:46] Alex Volkov: I think it's super easy to just add it there. Right? Listen it's not difficult. [00:37:51] Nisten Tahiraj: You just gotta add a Jason file to, to, to your model and that's it. Or just message him 'cause he's very responsive to this stuff. And might even write the Jason for you. And then it will be immediately available for everyone running LM Studio. [00:38:06] Vik Hyatk: Amazing. Another thing we have going on, by the way, is we're building an agent version of this with Open Interpreter in mind. [00:38:13] Vik Hyatk: A version of this that's excellent at identifying UI elements because we want Open Interpreter to have the ability to operate purely off of a local model. Open Interpreter, by the way super cool project. Check it out, folks, if you haven't already, is is a way to have the LLM use your computer. [00:38:31] Vik Hyatk: So you can do stuff like. Just tell LLM here I want to turn dark mode on and it'll figure out what buttons to click to enable dark mode for [00:38:40] Alex Volkov: for folks who follow ThursdAI closely, they remember Kilian came on the pod like a week after Open Interpreter was released, and this was, I think, in 2023, our most famous or received episode back then. It was a super cool conversation, so shout out Kilian Lukas, and definitely Open Interpreter since then has been very huge community of people building very cool things. [00:39:00] Alex Volkov: Recently released the kind of the browsing area, where it can Controls the computer for you. And it definitely needs eyes for that. And so I think it used GPT 4 vision and now you're saying that Open Interpreter will get open source eyes. Is that what I'm hearing? [00:39:15] Vik Hyatk: Exactly. That's a goal. CogAgent is super promising in this space. They didn't release their datasets, so we're working on replicating that. CogAgent is just too big for most people to run on their computers. It's I forget, 17 billion parameters or something. [00:39:29] Alex Volkov: Is that CogAgent and CogVLM, right? I think we, yeah, I think we talked about this. Yeah. It's really good [00:39:35] Vik Hyatk: but yeah, that's another place where if folks want to get involved the link in my bio as a Discord would love to collaborate with folks on getting that dataset together and training that version of the model. [00:39:44] Alex Volkov: So I think the kind of the thing I'm hearing from Fuyu, from you as well, the data set for vision stuff are the bottleneck to create like incredible things, right? Like data sets for images, data sets for how people use different UIs, for example, like all these data sets are the kind of the bottleneck for us to get to the next hurdle of getting these models even smaller, even faster performing. [00:40:04] Alex Volkov: So what are we doing folks? Let's start building multimodal data sets. [00:40:09] Nisten Tahiraj: Yeah, and at first for Baklava, we were going to have the dataset also open source because we are, the code for us is also open source as well. So it's not just open wave. It is fully open. However, the data we couldn't because of So that's not available and yeah, it's pretty hard to make datasets for vision because with text is very, it's very easy now to manipulate, modify, do whatever you want to, to the data and you can do that at large scale with images, just aren't that many tools, that many ready to go datasets and the open source models just started getting good at them. [00:40:52] Nisten Tahiraj: So yeah, that's going to remain. A challenge for the time being, but again if anybody here is like a grad student or you're at a company or something in academia, the biggest contribution you can make probably is in the data sets, because the models will get replaced. You'll always have better models coming and going, but the data sets are forever. [00:41:15] Nisten Tahiraj: If you want to make an impact in this field, get your professor, university, whatever to, to put some money for datasets. We need datasets. For images. With images. Yeah. [00:41:27] Alex Volkov: And we need them like bigger and bigger ever increasingly bigger scale. All right, Vic, so thank you so much for joining us. Thank you for talking, taking us through how you created Moonbeam. And thanks for telling us like what's next, how [00:41:40] the community can help besides, besides just, data sets provided and testing. [00:41:45] Alex Volkov: What else would you need? [00:41:48] Nisten Tahiraj: I I have a [00:41:49] Vik Hyatk: list of issues on GitHub where I'm looking for help with various But besides that, Compute always helps. I'm currently I'm limited on how many things I can do because my 4090s can only do so many matrix multiplications at a given time. So if anyone has Compute that they can give me access to run these, that would be super appreciated. [00:42:09] Alex Volkov: Yes, I I've seen this time and time again on ThursdAI on stage, folks ask for sponsorship for compute. I'm actually getting I'm actually getting like DMs from different companies like, Hey Alex, the space is super cool. Can we sponsor someone? Can we? And I'm like, no, I already work with Let's Ambassadors, I don't need sponsorship. [00:42:25] Alex Volkov: I would want to connect guys that work on super cool things. We need compute to keep going with different companies around like compute specifically. So I'll definitely keep you in mind. And go ahead, Nissan. You had a thing you want to say? [00:42:38] Nisten Tahiraj: Yeah, just really quickly, this is a very effective way to make projects that are impactful. For example, with Balclava, Pharrell here, and Suntex, they just put out a readme, and tweeted something out, and we got compute. And we got it from Together Computer. So they, they sponsored that, that project and they ended up being a very impactful project that a lot of people use. [00:43:05] Nisten Tahiraj: That, that works pretty well. I just say be careful with conditional stuff. If they're gonna start talking about an NDA, just Ignore them because that's not really, then you're doing an exchange, you're basically doing work for that person, so that's just a job contract, that's not a sponsor, if someone's sponsoring an open source model [00:43:27] Alex Volkov: Better be. [00:43:28] Nisten Tahiraj: not be like an NDA, that's not, that's no longer a [00:43:32] Alex Volkov: Better be open source after that. Yes, absolutely. So Vic, I'll keep you in mind when people reach out to me. Folks in the audience, if you work at a company that wants to be featured forever in the, in the open source community, definitely reach out to Vic and we want more of this. [00:43:47] Alex Volkov: We want more of like tiny models that perform incredibly well. We want them to be built into different Tools that we can all use without relying or paying by just using our machines. So definitely we'll keep in mind. Vic, welcome and welcome to the community of ThursdAI. More than welcome to keep joining and participating in this. [00:44:06] Alex Volkov: I think it's time for us to move on, folks. It's been around 40 minutes. I think we're actually good on time. I think it's time for us to move on to this week's buzz. I wish I had a I really want to do like a music transition here for the, with this week's buzz, with like bees buzzing, etc. [00:44:20] Alex Volkov: But maybe for next week. Let me just play the regular music and we'll transition and talk with Jason a little bit. [00:44:24] This weeks buzz - Jason Liu launches a new course with Weights & Biases for free [00:44:24] Alex Volkov: All right, welcome to this week's buzz, where I talk about some cool things that happened or I learned in Weights Biases. Weights Biases is, ooh, that was an abrupt music stop. Weights Biases is the system of records for all your LM needs. So pretty much like most of the folks up on stage who use who train models use Weights Biases. [00:44:52] Alex Volkov: It's incredible. The ubiquity , where bias pretty much prevented everywhere. I just saw a stable Kwan, one of our friends of the pod just train something and just post like words and biases, like a snapshot of his last curve going down and literally just asked Hey, do you mind putting a link to the dashboard? [00:45:08] Alex Volkov: And he did. So you wanna check out how his model is going? I think he's training. I don't think I saw, he's training something like super cool, like a Oh, he's training a mixture. Four 400 million parameters. So he's training like a tiny MOE of mixed role. StableKwan is, he just posted like a chart with the train loss from Weights Biases and I just asked, Hey. Can we follow along with the training? And he posted a link to the Weights Biases dashboard, which is super cool. [00:45:34] Alex Volkov: Which got a reaction from Weights Biases CEO. . And so I love seeing this in the wild. So folks, if you're training models, please put those dashboards up so people can follow along. It's super it's really nice. But on the other news from Weights Biases this week I want to say hi to Jason Liu. [00:45:47] Jason Liu: Yeah, Jason Liu. [00:45:48] Alex Volkov: Jason Liu. Welcome, Jason. I've seen you around. I've seen you, I think at AI engineer event from SWIX. I don't know if we like ran into each other there, but you had a talk there as well. Yeah. [00:45:58] Jason Liu: Yeah, it was Paidandic is all you need. It did pretty well on YouTube, so I'm pretty [00:46:02] Alex Volkov: It did great. I also talked with a bunch of people. I think I was interviewing folks, outside of the stage while we were giving the talk, but then it was very well received. And this is on the same similar topic that we're going to talk about now. So please feel free to introduce yourself briefly. [00:46:15] Alex Volkov: And then we're going to talk about the stuff that we did together. [00:46:19] Jason Liu: Great. Yeah. So I'm Jason. In the past year and a half, I've been mostly doing a lot of applied AI consulting. Before that, I spent the past like eight years just doing like machine learning. So I did the big data wave, the machine learning wave, the neural networks and deep learning wave. [00:46:32] Jason Liu: And now we get generative AI. So it's been a lot of fun. And in my spare time I work on a library called Instructor. So now. We have Instructor in, I think, JavaScript, Python, and Elixir. And the general idea is that we want to bring just functions and structs into LLMs and make LLMs feel a lot more backwards compatible with existing code rather than creating new abstractions to handle some of these things. [00:46:55] Jason Liu: And I think that's been pretty well received in the community. [00:46:57] Alex Volkov: Absolutely. So Instructor is definitely where I know you from. And today we have an announcement together. So feel free to. Feel free to announce the cool thing that we did and that you worked on really hard. [00:47:09] Jason Liu: Yeah, so we're starting a new series around, the idea of using like schemas and structures to prompt language models. And I think. At the day or end of this week, we're going to release the first part of a LLM engineering series. And the first part really is just an introduction on how we can use things like structure to prompt LLMs a lot better, right? [00:47:30] Jason Liu: In the past, we just like beg for the language model to give us JSON. Now we have things like JSON mode and function calling and tools, which gives us the ability to get more structure. But we still need a lot more tools and ways of thinking about how we can reason about these structures. And so part one is going to be around justifying and motivating why we wanna, why we might want to do this. [00:47:54] Jason Liu: And then I think in February or March we'll start working on part two that uses a lot of the new ways and biases, observability tools to look at how I've solved a lot of LLM problems in production with a lot of my consulting clients. [00:48:07] Alex Volkov: So just to highlight for folks, Weissenbeisser has a free courses area, Weissenbeisser Academy. And some like very prominent folks in the industry have collaborated with Weissenbeisser to like just basically teach. So we teach you for free how to do these things. So we have courses from like training, LLM from scratch, fine tuning, et cetera. [00:48:24] Alex Volkov: And then Jason is announcing a new course today that he wrote and and recorded and we helped edit a little bit and publish and also obviously talk and promote this a little bit about how to actually ask your model to give you what you need as a developer, as a AI developer in the structured output, which uses the instructor library. [00:48:42] Alex Volkov: Correct, Jason? [00:48:43] Jason Liu: Yeah, these ideas can be used in other libraries as well, right? So for the Python community, we're really using a library called Bydantic, and so this is supported in things like Langchain and Marvin. And so even if you don't use a library like Instructor, learning how to think about prompt infrastructure is still something that's going to be really applicable and valuable for everyone listening. [00:49:05] Alex Volkov: And you mentioned before, there's like a bunch of stuff that open the icons up with, like JSON mode, in example, etc. There is functions back in June. But also The other LLMs, they don't necessarily follow the same kind of new abstractions that OpenAI releases. I think Anthropic just recently announced that they're moving to function system messages or moving to just messages, things. [00:49:27] Function calling in OpenSource LLMS [00:49:27] Alex Volkov: And also we have open source, which is like all over the place. So I guess my question is, with these libraries, with these Paidantic approach and Instructor, would that apply to other LLMs? Does this apply to open source, which we talk a lot about? [00:49:40] Jason Liu: Yeah, so right now there's only a few open source models that support function calling. So if you've looked at some of the work from the functionary team, they have been training I think mixed role now with function calling, same with the guys that like news research with Technium. There's been a lot of progress in the open source world and getting things like function calling. [00:49:58] Jason Liu: If you want more structured outputs [00:50:00] too, there's a great library called outlines. That can use something like the Hugging Face Transformers library to also do structure extraction. And again, they also support things like Pytantic. And the goal of the course really is to show you how to think about and how to model these problems in a particular way. [00:50:15] Alex Volkov: Absolutely. And I think John Durbin in the audience I think Ouroboros was trained on function calling as well, if I'm not mistaken, John. So folks who haven't heard our conversation with John, definitely go and check out where the deep dive with John about Bagel, which now includes the Ouroboros dataset, which now includes function calling as well. [00:50:33] Alex Volkov: So that's awesome. The open source also moves there. Go ahead, Nissan. [00:50:37] Nisten Tahiraj: Also really quick the news vision model ended up being good at at function calling, although it had other drawbacks. It was good at function calling because of the Arrow Boro's like thousand something functions dataset. And as far as I saw the newer bagel models, so Bagel seven B are also good at at that, at at function calling. [00:50:57] Alex Volkov: So big old model series from Maxim Le Bon. Again, shout out Maxim Lebon, who came on the pod last week, and then the full deep dive with him will be released this Sunday, so make sure you're subscribed. We talk about, we don't talk about FunctionCall, we talk about NeuroBeagle. NeuroBeagle is like one of the top performing 7 billion parameters, it's a merge, it's a cool conversation about merging. [00:51:16] Alex Volkov: But let me back, let me get back to Jason just real quick. Jason, you're also like doing independent consulting, you said, in multiple places, and you're like helping them build. I got to like tap into your experience from like actually like hands on AI building and companies. Could you give us like a little bit of what do companies struggle with? [00:51:32] Alex Volkov: Like with the first obvious thing that comes to mind that people like AI builders probably like already solved in their minds. What do you have to go through to not only build to them, but also educate them on as you join the company, it starts like helping them out with AI stuff. [00:51:47] Jason Liu: Yeah. So one of the biggest things I noticed is that when we look at something like a RAG application, really what it looks like is a recommendation system. If you went on Netflix, for example, and you watch a bunch of movies and the recommendations don't get better, it would be a really terrible experience and you probably lose a lot of customers. [00:52:03] Jason Liu: But for a lot of companies these days that are using things like agents or retrieval, We are in a situation where, you know, no matter how many users you get, if you don't improve your language model, if you don't improve your embeddings, the product doesn't really get any better. And so one of the big things I'm focusing on this year is helping these companies build a better feedback loop and a data flywheel. [00:52:22] Jason Liu: And so we can know for sure that as we get more users, there's these network effects that improve the models that we want to train. And so I think step one is, being able to fine tune your own embedding models and your re rankers and go from there and then, see what comes up in the future. [00:52:39] Alex Volkov: Awesome. So definitely folks, give Jason a follow. The course, I think we're releasing it today, but I haven't seen any social mentions, but it's really worth watching. I watched a few of this and we'll follow as well. And this is a course series now. So we're going to start with this, and then we're going to continue with the monitoring tools that Waze Ambassadors have. [00:52:56] Alex Volkov: Correct? [00:52:58] Nisten Tahiraj: Yeah, the first course is like 30 minutes. It's super quick. The real goal is to show you what's possible and get you thinking about some new ideas. And then the next course will be deeply integrated with the more visibility tools from Wisdom Biases and specifically around the experiences I've gotten from consulting production clients. [00:53:13] Alex Volkov: Incredible. Thank you, Jason. Thank you for joining us. And thank you folks who worked on the course together with you. I'm excited to see this. And again, the reminder, there's a bunch of free stuff there. There's a bunch of like knowledge just drops here. And hopefully I will be able to tap into this community and also build more things. [00:53:29] Alex Volkov: Go ahead, Nistan, and then we'll move on. [00:53:31] Nisten Tahiraj: Yeah, I just want to say that a lot of us here that got good at machine learning were from just a random YouTube series. So the Karpathy series on Building one from scratch. The full stack is just pronounced like that. Their LLM one from way back in April and March. So I'm really looking forward to this one because doing YouTube tutorials is actually extremely efficient. [00:53:53] Breaking News - HuggingFace announces a collaboration with Google [00:53:53] Nisten Tahiraj: But on that note, we have breaking news. [00:53:56] Alex Volkov: Wait, we have breaking news. Hold up. You know what this means. [00:54:11] Alex Volkov: Yes, Nistan, go ahead now. [00:54:14] Nisten Tahiraj: Phil Schmidt, who is a friend of the pod and has been here. [00:54:18] Alex Volkov: Here, yes. [00:54:18] Nisten Tahiraj: definitely. Yeah, Devleet at, At Hugging Face, he's also the one that did the integrations, if I might be wrong, but the integrations for with AWS Bedrock and also with CloudFlare workers. Yeah, so now it looks like he's been working on doing an integration. [00:54:35] Nisten Tahiraj: with Google, where you'll be able to just take whatever models or fine tunes and stuff you have on HuggingFace and then use Google's infrastructure, use both their TPUs and NVIDIA H100s, they're advertising this, that Google owns, to continue training, fine tuning, serving, deploying stuff via HuggingFace. [00:54:55] Nisten Tahiraj: Google. Is this is a very interesting move. Google's jumping in more on the open source side there. I don't know what this means, but this is a very interesting development. [00:55:06] Alex Volkov: I know what this means. This means that, if Hug Face becomes public ever, buy their stock. That's what this means. Hug Face like literally embedded into the, like the infrastructure of AI and definitely worth following. And the more integrations they have, the better, like it is for the open source community as well. [00:55:25] Alex Volkov: All right, folks. Thanks Nissan [00:55:26] Nisten Tahiraj: This is not financial. By the [00:55:28] Alex Volkov: financial advice, but they're also not public yet. Look, I don't think this move. Yeah, I don't think this moves the needle for, in terms of Google investing, [00:55:36] Hourglass Diffusion Transformers deep dive with Tanishq Abraham [00:55:36] Alex Volkov: Alright folks, we're moving forward and the way, where we're moving forward is also like into kind of like diffusion mode, and I'm very excited to introduce Tanishq. [00:55:45] Alex Volkov: Tanishq, have you been here before? Remind me, please. I don't think you've been here on stage before. [00:55:50] Tanishq Abraham: I, I don't think I've been on stage [00:55:52] Alex Volkov: No. All right. So I'm very excited to have you here. Thanks. Thank you for joining us. So folks, one of the coolest things that came out in at least the research area from this week was this paper from. [00:56:03] Alex Volkov: From multiple authors, some of them friends of the pod, like Enrico, if you remember the chat with Enrico we did with rope scaling is on the paper as well. Katherine Crowson who we should mention, I don't think she's been here or, but we've talked about some stuff that she did. Stefan Baumann, Alex Birch, Tanishq, you're on there, Daniel Kaplan, and then Enrico, a friend of our Nico. [00:56:23] Alex Volkov: Tanishq has been the friend of the pod behind the scenes, you guys didn't know this, but we've met in NeurIps so we've met before. Tanishq, do you mind introducing yourself just briefly for the audience who haven't met you or followed you so far? [00:56:34] Tanishq Abraham: Yeah, sure. My name is Tanish. I am a research director at Stability ai and also CEO of MedAR, which is a medical AI research organization. I've also been involved with fast ai, been working on, diffusion models for [00:56:48] Tanishq Abraham: I guess past year and a half or so. Yeah, so I do all kinds of stuff. [00:56:53] Tanishq Abraham: Generative ai, [00:56:53] Tanishq Abraham: medical ai. Yeah. [00:56:55] Alex Volkov: You also just like a briefly skipped over the fact that you got your PhD at 19, right? Is that correct? [00:57:01] Tanishq Abraham: Yes, that's correct. I got [00:57:02] Tanishq Abraham: it. That was last year. Yes, [00:57:03] Alex Volkov: So if folks in the audience don't know what this means that there's not many like 19 year old PhDs and Tanishq is one of them. And also we met once. I think a year and a half ago. And then the next time we met in Europe, I just remember every detail of our conversation. But that's beside the point. [00:57:17] Tanishq Abraham: yes. [00:57:19] Alex Volkov: Thanks [00:57:19] Tanishq Abraham: met at the Stability AI [00:57:21] Alex Volkov: Lunch party. That was super cool. And since then, many things have changed. And I really want to talk to you in that area, right? So this paper, shout out to all the authors because I'm looking at this. I've seen like multiple folks share this paper. Paper is talking about high resolution image synthesis. [00:57:39] Alex Volkov: With something called Hourglass Diffusion Transformers. And I will pin your great thread about this here on top of the space, and it will be in the show notes. Could you briefly tell us the problem this tries to solve? And then we're going to go into actually how this kind of approaches how to solve this. [00:57:57] Tanishq Abraham: Yeah, definitely. [00:57:58] Nisten Tahiraj: Yeah. So first of all, of course preface this by saying it's mostly, of course [00:58:01] Tanishq Abraham: Kat's genius work here. And we were just lucky to be able to help her on this project. But yeah, just to get her started. [00:58:06] Alex Volkov: just one tiny second because it's worth a shout out. So Kat, by Kat you refer to Katherine Carlson, right? And if folks ever used Stable Diffusion before, either in Automatic 1. 1. 1 or whatever, and you [00:58:20] choose anything with K dash that's, this is the Katherine, right? [00:58:24] Alex Volkov: This is, K Diffusion is like her area. Very incredibly prolific person in this area I don't know many facts about her, but like everybody who I talked to from this paper, including Enrico, everybody's like referring to Kat, that's her work. So maybe a huge shout out to Kat and yeah, go ahead, please. [00:58:40] Tanishq Abraham: Yeah yeah, she's like a, she was like one of the original AIR people, so yeah, I had, she helped start the field in a way, anyway, [00:58:46] Tanishq Abraham: To To provide some context of [00:58:48] Tanishq Abraham: what this paper is about the idea is that, if you want to do like high resolution generation, so think like 1024 by 1024 the typical approaches these days utilize some sort of multi stage approach, like the most common one, like stable diffusion, is this sort of latent diffusion where you have to encode it in with some sort of auto encoder into some latent space and you're doing diffusion on the latent space and you're not actually doing it on the actual pixels. [00:59:15] Tanishq Abraham: And so that comes with some disadvantages. For example, if I don't know if people who are like doing things like image editing with stable diffusion, you realize you don't have a whole lot of fine grained level of control in terms of the actual, at the pixel level. [00:59:30] Tanishq Abraham: It's difficult to do that because it's happening in the latent space rather than at the pixel space. So there are various different things where like it has its own challenges. Of course, like latent diffusion has a lot of different advantages too, but you know for some applications it may not be ideal. [00:59:44] Tanishq Abraham: And then on top of that the other aspect that, we wanted to like, look into basically was the fact that we're seeing People move towards towards transformer models for diffusion as well. And of course, in the past, most of the diffusion models have been with, a U net architecture, a convolutional U net. [01:00:02] Tanishq Abraham: Also stable diffusion uses a convolutional U net. But, there have been a lot of papers examining the use of transformers. And, of course, the nice thing about transformers is, people know how to train them, they're quite scalable, so people would rather use transformers for diffusion over over something like a U net. [01:00:18] Tanishq Abraham: But again, the problem is that So far, it's mostly only been applied to the Latent Diffusion Scenario, mainly because it would be very hard to do this at pixel scale because of the quadratic complexity of attention. So if you wanted to scale up to higher resolution, you know that, it would be, at the number of pixels, you're going to have quadratic scaling with that. [01:00:40] Tanishq Abraham: So it would be very difficult to train this with, I guess enough resources or whatever. So that's the problem that we're trying to solve is what can we do to resolve the quadratic complexity of the transformer architecture that allows us to then train a diffusion transformer in pixel space. [01:00:58] Tanishq Abraham: So that's what the hourglass diffusion transformer tries to address. [01:01:02] Alex Volkov: Thank you for the brief introduction. For I will try to recap as a way I understand this. So folks who are not machine learning scientists in the audience would be able to follow along. But basically Gen AI, this whole wave of Gen AI has two, two big infrastructures so far, right? [01:01:15] Alex Volkov: The diffusion, the stability AI and of the image models and video models. They're based on like diffusion or you said latent diffusion, correct? And then there's the LLM area with basically based on transformers. And we've seen a bunch of stuff going back and forth in tech, like in techniques between them, right? [01:01:31] Alex Volkov: So Laura, I think is a thing that like many people in the diffusion area, like trained Laura's on different concepts. And then obviously like fine tuning with Laura's then became a thing and back and forth. We've seen like back and forth different approaches. I think you said like The open source area in LLMs in Transformers specifically has a bunch of like super cool tricks and optimization techniques and flash attention different things, right? [01:01:54] Alex Volkov: There's a bunch of stuff that people developed in one area that wasn't necessarily applyable to to, to diffusion models. And so you guys set out to try and unify those two, or at least use some of the tricks and looks [01:02:09] Alex Volkov: succeeded to an extent. Yeah. Go ahead please. [01:02:12] Tanishq Abraham: yeah, I think it's, yeah, about Now that we have this transformer architecture, we can try to apply some of the tricks that people have been using, things like, rope embeddings there are other tricks like RMS norm, these are the sorts of tricks, for example, that are used in the Lama architecture these sort of similar architectural decisions and you could take those sorts of best practices and try to see if they help with diffusion now. [01:02:33] Tanishq Abraham: So yeah, I think that's the idea. And like people were exploring yeah, that's like another interesting thing about our papers. Like people were exploring diffusion transformers, but they were using very kind of old architectures for diffusion transformers. And here we're trying to also apply all these tricks that we see. [01:02:47] Tanishq Abraham: People are applying the LLM space and trying to apply that to to, to diffusion. Yeah, that was also an important part of our paper as well. [01:02:54] Alex Volkov: And of course, you mentioned Rope, and I want to shout out a friend of the pod, Enrico, from News Research, Enrico. Wait, I don't actually remember if Anuka is part of News Research. Maybe, so he and News Research worked on the Rope paper together. And for folks who are interested in hearing about Rope, we had a deep dive during the summer, one of the coolest episodes. [01:03:12] Alex Volkov: Most of it back then went above my head, but it was super cool going back there and saying, Hey, oh, I learned this. Rope is basically a way to extend context windows and do a bunch of other things for Transformer based large language models. And I wonder how does Ropen play here? And Enrico is part of the authors here on, on the paper. [01:03:29] Alex Volkov: So he contributed at least part of that work, I assume. Enrico? [01:03:34] Tanishq Abraham: Yeah. I think the rope stuff is like something that We even, we haven't like fully explored the full potential there, I think. But at least for what we were doing, we saw improvements in, in performance, just using rope over other sorts of, these sorts of position embeddings. [01:03:50] Tanishq Abraham: But yeah, I think there's definitely potential for allowing the model to handle larger resolutions or do things like this because of the rope embeddings that we have in the model. Yeah it's, I think, also meant for future work. [01:04:02] Alex Volkov: Incredible. You guys use all these techniques. You introduce, or I guess start formally announcing this concept of the fusion transformers, which is the mixture of these two things. And what are some of the results that you get? You've trained a few models to test. [01:04:15] Alex Volkov: How do you even, measure that you're getting performance or you're just looking at algorithms or you're actually generating images. Can you talk us through the process of validating this like theories and papers? [01:04:26] Tanishq Abraham: Yeah, but I just want to yeah, I guess to take a step back to clarify we didn't necessarily invent the concept of diffusion transformers. That is something that people have already developed but the idea that we focus here is the problem is in the past, diffusion, Transformers were done with the latent space because of this quadratic complexity. [01:04:45] Tanishq Abraham: So we basically have a different type of transformer architecture, which is this hourglass transformer that enables for Like O of N scaling, so like a linear complexity. So it, it will scale with the number of pixels much better than it won't blow up like, like you, you have with with the attention quadratic complexity. [01:05:07] Tanishq Abraham: So that was the main trick that we're using. So we have some tricks in there. That allow it to have that property. And that's what enables us to do it on the pixel space, as opposed to the latent space that the previous diffusion transformers were doing. And then on top of that, we are adding all these additional transformer tricks, which no one had tried out before with diffusion transformers. [01:05:27] Tanishq Abraham: So those are the main sort of contributions of this paper in terms of in terms of, and yeah, I guess one thing, the, yeah, the other thing worth mentioning is that the way that this architecture is able to do this is partly because it's, it the architecture is a very hierarchical architecture. [01:05:45] Tanishq Abraham: So it's actually able to process at different image resolutions. And for example at the high resolutions, we use a sort of the, this sort of local attention, which is what is. Having this linear scaling, but then at the low resolutions, we were able to do the regular attention. [01:06:01] Tanishq Abraham: Yeah, there's also this hierarchical processing of the image resolution. That's also, I think, an important point, which enables also for higher fidelity as for generation. And yeah, in terms of testing the [01:06:13] Alex Volkov: Yeah. And so the next question is how do you actually like test the architecture? How do you validate these like approaches that you tried actually better than what the field has previously been at? [01:06:26] Tanishq Abraham: Yeah. We looked at two datasets. One, we did ImageNet generation. So can conditional, class conditional ImageNet generation. So that is, passing in an ImageNet class, you generate images of that class. So if you pass in a zebra [01:06:40] class, you're generating zebras, or you're in some sort of dog class, you generate the dogs. [01:06:43] Tanishq Abraham: That's, we train a model for that. We train it at a resolution of 256 by 256 and that, that's one of the experiments where we compare to other architectures. And so we we're, the interesting thing is that, of course, we're comparing to other architectures that are using, for example Latent Diffusion, that they're, using the latent space there the architecture is functioning on the latent space and not on the pixel space, but we have our architecture that's functioning on the pixel space and using this hourglass transformer and it's getting better results than with the with the latent space. [01:07:19] Tanishq Abraham: We're beating, for example, the previous Diffusion Transformer model which was using the latent space. And then another interesting data set that we used was the FFHQ. Data set which is this sort of data set of high yeah like high resolution faces and so this is at this is at a 1024 by 1024 resolution and so this is like you know very difficult to be able to train especially in a pixel space you know at Scale of 1024 by 1024. [01:07:47] Tanishq Abraham: And actually there are not many other diffusion models that are trained on this model. There are a bunch of GAN models, for example, but not really many diffusion models. There's like only one or two that we actually found in the literature because it is, it can be a bit difficult because of this, because of the. [01:08:01] Tanishq Abraham: The pixel scale or the, the resolution of the images, but yeah we were managed to train a model with our architecture. It can, it trains quite fast. And yeah we are able to we're basically like, I guess at this point now we would be the best diffusion model for that for that data set. [01:08:18] Tanishq Abraham: And we are measuring with FID. But of course, like FID, as a metric also has its problems it does have some bias towards like towards GANs and so GANs tend to have a lower FID kind of in terms of the bias of the FID. So like when we look at it qualitatively, honestly, we think like it's quite comparable to the GANs, might be better than the GANs, honestly. [01:08:41] Tanishq Abraham: So we may do more evaluations and study that further. But honestly, this may be like. One of the state of the art models for this FFHQ dataset but it's a bit hard when you're using as a metric, but that's of course the problem with, everyone's using that metric in the literature, but yes, but yeah, I think that, again, that's another really interesting result that we observed. [01:09:01] Tanishq Abraham: And then, of course, we do [01:09:02] Alex Volkov: I want to follow up with a question here real quick. For folks like, hard for them to follow like much of this, but they've used something like Stable [01:09:09] Tanishq Abraham: oh, sorry. [01:09:10] Alex Volkov: No, that's all great. This is all recorded. Folks can like pause and go to, and go research and come back and listen to you. [01:09:15] Alex Volkov: This is great. Like you did the deep dive. I really appreciate it. I just want to bring this back a little bit upwards towards like [01:09:21] Unkown: Sure. [01:09:22] Effects on the industry from Hourglass Diffusion Transformers [01:09:22] Alex Volkov: affect the industry, given that we have stuff like Stable Diffusion out, and that keeps getting better, Mid Journey is getting like reality adjacent to the point where like it's really hard to distinguish, there's like different upscalers that take the outputs and then run some upscaling how does this affect the industry to, in your mind? [01:09:40] Alex Volkov: Will this Accelerate some stuff. Will this be applied to different areas that like the fusion models have not been traditionally in? What is the kind of the, let's say this is a building block that you've created. How does this affect us in three, six months? [01:09:54] Tanishq Abraham: Yeah, I think this is just a kind of a new unique direction to explore. Of course, I think latent diffusion is still a very interesting, invaluable direction, but this is just it's always good to have different directions to explore. And I think And honestly, like this architecture can be applied to latent diffusion as well, and maybe we get even better results, for example, we can do maybe like, multi megapixel level synthesis by combining, this method with latent diffusion or something like this as well. [01:10:23] Tanishq Abraham: So it's not even like it's. Limited to just the pixel space. That's what we're showing that, that's something that is interesting about this. But again, it can also be applied to agent diffusion and can even, of course, these models could be scaled up further. There's a whole lot of future work to explore here, I think. [01:10:39] Tanishq Abraham: And yeah, I think and of course it's computationally efficient. And yeah, I think the nice thing is yeah, moving towards the transformer architecture when, it's, people understand the transformer architecture at this point. I think, there's people understand how to scale it and different tricks. [01:10:55] Tanishq Abraham: And it's, I think this is a good, by introducing this architecture, this is a good way for. As to try to bring some of those advances in transformers into the diffusion model field as well. So I think that's the other interesting aspect of this. [01:11:12] Alex Volkov: for me reading this is not a machine learning scientist. Reading this was like the highlight of interesting things were like The open source community moves in, in, in different areas, but also like bringing over some of the learnings, bringing over some of the talent the tooling around, like making things available. [01:11:28] Alex Volkov: And I think that's like very exciting. We also have Alex Birch, is that correct? The name also in the audience. So shout out Alex. And then what else do we not cover this stage? What is the last thing that you want to say? Or maybe shout out some of the co authors feel free, the stage is yours. [01:11:44] Tanishq Abraham: Yeah, I'm just looking at some comments that I, Alex also has some comments that he said. So he thinks, for example, that with this model, that there's potential to. Achieve more realistic textures than even mid journey. So I think, we have observed, like with the model, like the, because that's the thing about using, when you're using a latent diffusion where, it's not, you're not doing, when you're not doing it at the pixel level, it's a bit. [01:12:07] Tanishq Abraham: Difficult to get those get those, textures accurately, but if you're doing it the pixel level I think you're able to get those textures yeah, it can do that much better. And we've observed that with the models that we've been training. And yeah, I definitely agree with Alex there. [01:12:22] Tanishq Abraham: Yeah, I think also like it may have potential to achieve like really realistic textures and that, that's something. That I guess we could look forward to hopefully. Yeah. [01:12:31] Alex Volkov: that's incredible cause I think the realism comes from the imperfections, especially like textures and skin, et cetera. And like diffusion models have, at least for many folks are easier identifiable by the kind of the smoothness of edges and different things. So definitely like more more textures are there for humans in real pictures. [01:12:50] Alex Volkov: And then we're looking forward to more of that in diffusion models. That's incredible. So definitely, thank you for breaking this down for us, Dinesh. Thank you and Catherine and Alex and everybody else in Rico who worked on this. I think we have some questions from folks on stage here. Vic, go ahead, please. [01:13:05] Vik Hyatk: Yeah, another question. [01:13:06] Vik Hyatk: I just wanted to see I played around with the repository a bit. It's a great way for anyone interested in getting into diffusion models to get started. It's not your typical research code base. It's super clean. [01:13:19] Vik Hyatk: You're not going to run into a bunch of dependency issues and whatnot. [01:13:22] Vik Hyatk: So that [01:13:23] Vik Hyatk: was amazing. It's also super compute efficient, so you don't need a ton of compute. To start to see good results. I'd strongly recommend checking it out if anyone was feeling intimidated [01:13:32] Vik Hyatk: before, [01:13:32] Vik Hyatk: don't be. [01:13:34] Alex Volkov: Incredible. [01:13:35] Tanishq Abraham: Yeah. That, that, that comes down to Kat's again, Kat's genius. I think this is a code base that she's been working on for quite some time and I also really enjoy working with it. [01:13:42] Tanishq Abraham: It's like one of my favorite diffusion model code bases. So I definitely agree that anyone who's interested in playing around with diffusion models should check it out. [01:13:49] Alex Volkov: So that, that's on Cat's GitHub. We're going to add this in shell notes called KDiffusion, correct? It's now [01:13:55] Alex Volkov: part of that existing code base, but now like this, the Hourglass Diffusion Transformer. Get used to say Diffusion Transformers from now on, folks. Hourglass Diffusion Transformers, HDITs, are now a thing. [01:14:06] Alex Volkov: And Tanish, thank you so much. And Alex for joining in from the comment area. And thank you for working on this work. Hopefully this will get the recognition it deserves and definitely as a foundational block to get us Higher performance, lower, hardware requirement models that look way better. [01:14:22] Alex Volkov: Incredible. [01:14:23] Open source models in medical fields [01:14:23] Alex Volkov: Tanishq I wanted to follow up with you, because MedArk is something that you're now CEO of medical things, and then you had a tweet today that I really wanted to talk to you about specifically because Quyen was involved, and we have like folks from Quyen, usually like friends of the path as well, they join us could you, [01:14:37] Alex Volkov: let's talk through this please, let's talk through How open source is catching up to medical space. [01:14:42] Alex Volkov: Could you briefly summarize what we've talked, recent work from you guys? [01:14:46] Nisten Tahiraj: Yeah. Sure. Yeah. I've been [01:14:48] Tanishq Abraham: quite busy with all kinds of different research projects. So that was another. Ongoing research project that we're working on at MedArc and that I'm shared some progress of that today morning. So basically, at MedArc, we're of course interested in [01:15:00] developing open source medical language models. [01:15:03] Tanishq Abraham: So that, that's something that we're heavily interested in. And of course, in order to be able to do we wanted to understand what The current capabilities of these language models look like the open source language models and no one had done like a very proper analysis of this as far as I could tell and yeah, basically we, what we did is we added this suite of tasks known as the Multimed QA. [01:15:24] Tanishq Abraham: Sweet of tasks. So this is a kind of a bunch of tasks, a total of nine tasks that were they came from different other papers and stuff, but Google put them together as this is their sort of evaluation bench, this is the evaluation benchmark that This is what Google was using to evaluate their MedPAL models and, whatever models they had. [01:15:44] Tanishq Abraham: And then, the community, the medical AI community been using that. It's been used to evaluate GPT 4 [01:15:49] Unkown: and all kinds of [01:15:50] Tanishq Abraham: other models as well. And yeah, I, we, at MedArf, we added it to the LM eval harness. So that's like the common sort of for open source language models. [01:15:59] Tanishq Abraham: Everyone I think uses LM eval harness to evaluate the models on various tasks. So now it's in there. So people can easily also evaluate their, whatever the models they have on these medical tasks. And so once we added it into LM eval harness, we just wanted to just. Do a comprehensive like analysis of a whole bunch of models in the open source space, just to see like these sorts of generalist models. [01:16:21] Tanishq Abraham: Like they're not necessarily particularly trained on medical data. Of course they've probably seen some in, in, in their pre training or whatever, but that's not their main purpose and that's not their main focus in their pre training. And I'm, I was just curious what their performance would look like and, how it compares to other models like GPT 4. [01:16:36] Tanishq Abraham: GPT 4 is also a generalist. It's a generalist language model as well. It's not also necessarily trained on medical, but, it's really good at that. In fact Prompt Engineer GPT 4 is state of the art on this benchmark, actually. [01:16:48] Alex Volkov: I remember this. I remember where Google came up with a specific medical device and then GPT 4 just like basically with prompt engineering on that benchmark became the top one, right? This was quite incredible that the most generic [01:17:00] Alex Volkov: model we have. Yeah, [01:17:02] Tanishq Abraham: that's the it's called MedPrompt. That's the state of the art, this prompt engineering, prompt engineered GPT 4, it's called MedPrompt. And so they do a whole bunch of tricks like, dynamic few shot and GPT 4 written chain of thought and all kinds of tricks that they throw at GPT 4 and they got state of the art. [01:17:18] Tanishq Abraham: And then of course they use the same tricks to then, later claimed that GPT 4 is better than Gemini as well. It's not just for medicine that you can use it. They use it for just general prompt engineering as well. But yeah, anyway so yeah, this is, so overall the point is I wanted to evaluate how these models do in the how the open source models do in this, on this benchmark. [01:17:38] Tanishq Abraham: And so I evaluated a whole bunch of models. I evaluated Lama, Mistral, Mixtral. I evaluated the Yi series of models. I evaluated Quinn. Yeah, so I evaluated a whole bunch of models here and basically what I found out is first of all, Lama 2 is not that great compared to all these other models, actually, and it's, It's interesting because in the literature people are still fine tuning Lama 2 for medical purposes but, it actually doesn't have a very good base capability of for medical knowledge. [01:18:09] Tanishq Abraham: So Lama 2 is not very good at medical stuff, but the models that are quite good are basically the Yi series of models, so Yi 34b is really good, as well as the Quen series of models. So Quen 72b is The state of the art open source model and it's, and this is not like doing any sort of prompt engineering or anything like this. [01:18:28] Tanishq Abraham: This is just like five shot prompting and it's beating MedPalm version 1. So MedPalm version 1 was released in November of 2022 and that was like the first sort of yeah, that was Google's model that they had. And this Quenz72B is beating MedPom1 without any sort of prompt engineering or any of these tricks. [01:18:50] Tanishq Abraham: And yeah, I think that's really, honestly, quite impressive because [01:18:54] Alex Volkov: Yes. [01:18:55] Alex Volkov: I want to shout out Jun Yang or Justin Lin a friend of the pod, the head of technical, working on Quen for such like incredible achievement. And thank you for testing this. Because we and Nistan, like you worked on AI in medicine as well. Like we've waiting, this is going to happen. [01:19:11] Alex Volkov: Want it or not, there's like several doomers that say, Hey, never trust an AI doctor, but, many people already go to JGPT to, maybe get a second opinion. And Google has obviously been working on this MetPalm and MetPalm2. [01:19:22] Alex Volkov: I think for many people it's going to be easier to digest this idea if the model that talks to them is like fully runs on their computer, open source, no internet, like no data sharing. [01:19:33] Alex Volkov: I think that's a very important piece of this as well. And it's great to see that, we're now getting like some cool comparison, but definitely open source is coming strong on this one. [01:19:42] Unkown: Yeah. [01:19:43] Nisten Tahiraj: Yeah. I had the same thing as, Astonish with the Lama models, you can train them on good medical data, but they don't have a, they don't perform great at the base. I'll tell you, it's still, GPT 4 is king when it comes to it. And the product I worked on last year in March, it's still going, Dr. [01:20:04] Nisten Tahiraj: Gupta. ai is, it is still going. It's just a very well prompted, engineered product. Doctor with with a good RAG system too, that was one of the first, but I will say the thing about the main concern now, and why I think open source will basically completely dominate medical AI, is that their main concern is If they're dependent on some kind of API endpoint that makes the hospital and people's medical data really vulnerable to malware and foreign intelligence groups, which have been wrecking havoc with with medical data and ransomware. [01:20:42] Nisten Tahiraj: So that's their main concern. And the only way we're going to solve that is by having models that they run locally. So I'm really glad Tanishq actually took the task on. Benchmarking some of these, because you have the entire medical safety field and all the funding and all the people and I have yet to meet an AI safety person that even knows how to rename a file in Linux, let alone actually write some kind of benchmark. [01:21:07] Nisten Tahiraj: So I'm glad someone's actually taken on the challenge of making open medical yeah, medical LM benchmarks. [01:21:19] Tanishq Abraham: Yeah, I completely agree in terms of yeah, I definitely think open source is definitely the feature for medical AI and medical LLMs. And I think hospitals and doctors will be more comfortable when they know they have access to the model and this is the model that they're using rather than when it's behind some API also where not only like in the case of like malware or things like this, but open eye. [01:21:40] Tanishq Abraham: AI will just change the model or something like this too, or, these are all concerns that we see this already happening with the models that OpenAI has. And, these are all like concerns that, there needs to be complete transparency when working with with these kind of more crucial applications. [01:21:55] Tanishq Abraham: And, by doing all this open source I think that that provides that transparency that doctors and hospitals and healthcare systems will be comfortable with that. That's why I'm really excited about working in this area. And I think there's really a lot of potential here. [01:22:09] Alex Volkov: incredible. Thank you for this work, Dinesh. Thank you for bringing us kind of the idea of which of the models. Surprisingly, Quen. Like I wouldn't assume if you gave me all the models that we've talked about I wouldn't assume that Quen was the most performing, but hey, we'll take what we can get. [01:22:22] Alex Volkov: Quen72b, the best open source doctor, folks. You hear, you heard it here based on this research. [01:22:30] Tanishq Abraham: Yeah. Thank you for letting me share all this work. [01:22:32] Alex Volkov: That's incredible. And as a friend behind the scenes, but now friend of the path, you're always welcome. Thank you for the deep dive on the Hourglass Diffusion Transformers. Thank you for the authors as well. Alex, like still, I think is in the audience and Catherine and Rico and some other folks, and definitely for MedArk, keep us up to date. [01:22:48] Alex Volkov: We'll keep reporting and the stage is yours whenever you want it. I think folks we're moving forward. I think Nissan, unless you have, or sorry, Tanish, you have the one last thing you want to [01:22:57] Tanishq Abraham: I would just say please follow first of all, follow all of our Hourglass Diffusion authors. They all deserve your support and also please follow MedArk as well. [01:23:06] Alex Volkov: 100 percent worth following and definitely will be in the show notes for folks who are listening to this while driving and cannot like click that follow button. I think we're moving to as we're in the hour and a half into the space, let me reset [01:23:20] this a little bit for folks. If you just recently joined us, you're listening to ThursdAI where we talk about everything. [01:23:26] Alex Volkov: And everything incredible and interesting in the world of AI and open source, LLMs, big companies we cover. And we also had a deep dive today about vision video. My name is Alex Volkov. I'm with Weights Biases. I'm an AI evangelist. And yeah, we're here every week and we keep up to date. So you don't have to, so if you were out of Twitter or if you don't even participate in Twitter and you're just listening to this on the podcast, we got you we're going to cover everything that's most important and then send you this, so definitely check out. [01:23:52] Alex Volkov: There's the i. news for that. And I think we're moving towards the big companies area, which we haven't touched. We briefly covered in the breaking news where Hug Face just announced a partnership with Google. So you'd be able to very easily run the models from Hug Face on TPUs and the Thingisneyosa GPUs, which is incredible because Google has those, but they don't even give them away. [01:24:15] Alex Volkov: I think they're all reserved for collab or something. But also. Everything that I have today in the big company LLMs and APIs and everything is from Google. [01:24:25] Google teases LUMIERE, SOTA video generation models [01:24:25] Alex Volkov: So the next thing that we're going to talk about is Lumiere. And I don't know if you guys saw the video, but I definitely saw the video. I think Pharrell, you sent this in our group chat first, but by that time there was already spreading around. [01:24:37] Alex Volkov: . So there's obviously the whole area that we've talked about. Sable Diffusion Video releases like very short videos image to video and text to video. And then there's the front runners in the closed source, which is Runway and Pika. And there's like another one Firework. Oh, Leonardo is doing some incredible things. [01:24:54] Alex Volkov: All of them have very short videos and the consistency between the frames is not like incredible. And Lumiere. Has shown a video and this, like for all, sorry, you're saying this could be like very cherry picked, et cetera. But it feels like this is like another step in this direction that's significant. [01:25:13] Alex Volkov: And for folks who are not like watch the video yet, definitely worth watching. I'm going to add this it's already on the top of the space, but basically you see they announced a bunch of stuff that Lumiere can do besides just generation. So video in painting is one that they've announced. [01:25:28] Alex Volkov: They announced like a text to video text to video, image to video in painting. And they have something like they say, realistic, diverse, and coherent motion specifically around the motion of kind of the characters, which has been lacking in all these like video synthesis. I will say it's. [01:25:44] Alex Volkov: It's pretty remarkable to even discuss that oh, this vision text to video image is not as good as that one. It's really incredible that we're, like, at this point where we can say, a highbrow, Oh, yeah, I prefer this output. We're, like, we're typing text and getting a video back. [01:25:59] Alex Volkov: It's ridiculous on the surface of even saying this to us. Like a year and a half ago that this would even be possible. But with that said, we're moving forward. We're like hedonistic adaptation is a thing. We're getting used to these tools and we're getting them like day to day. And then we're like, okay, yeah, this tool is better. [01:26:15] Alex Volkov: They said the existing video malware synthesized distant keyframes, followed by temporal super resolution and then that's probably it makes it temporal consistency difficult to achieve. Temporal consistency basically says where like characters throughout the video, what they do. [01:26:30] Alex Volkov: And so you've all seen these videos where like the face changes from frame to frame, et cetera. And this. This series of videos from New Year looks very consistent, like spatially and temporally. Like definitely where the characters are in the video, but also like throughout time. And they Attribute this to different methods that they've used I will not go into this, but I think the tasks are very interesting. [01:26:53] Alex Volkov: They have video editing applications image to video and painting and stylized generation. Something I also liked. You'd be able to take like an image and then generate videos based on that style, not necessarily that image. So very impressive from folks from Google, as always from Google. [01:27:08] Alex Volkov: I haven't played with this. I don't think there's a way for us to play with this yet. So there's a paper maybe some of the ideas in the paper could be reproduced in open source. But it's like a model show in the paper from folks quite a lot of folks, Omar Bartal, Hila, Omar, Charles Herman, and there's like a bunch of folks there on the paper. [01:27:25] Alex Volkov: Very like visually appealing demo as well. So definitely we'll add this video in the show notes. And I think we have. One more thing here in Diffusion stuff. Yes, the one last thing that I wanted to talk about is Instant ID. Where so we moved off from Lumiere, Lumiere is like super, super cool, but we haven't seen this work. [01:27:43] Alex Volkov: Hopefully the releases as Google has a back, they have an example of like when they released stuff, like Dreambooth was released and everybody was using this. And. I think that's pretty much it in the big companies and open source. [01:27:55] InstandID - 0 Shot face transfer diffusion models [01:27:55] Alex Volkov: The other thing that I wanted to mention is instant ID. We've mentioned this briefly before, but it's been pretty much everywhere on my timeline. If you haven't played with this, I very strongly encourage you to play with this. Because instant ID is a technique to transfer to create diffusion models with your face. [01:28:11] Alex Volkov: And we've all probably tried this at once. With, like I said, like a dream booth from. Nathaniel Ruiz, who's a dear friend of the pod, who's been here a couple of times. There's like other techniques also to transfer your face into a latent diffusion model. And they all used to take multiple images of your face and some amount of training. [01:28:32] Alex Volkov: And Instant ID is basically a technique that you can try right now, super quick. With zero shot, one image. You can generate images with your face, or with your kid's face, or whatever. And literally I just want to highlight how impressively fast we're moving towards these type of tools. This used to take fine tuning. [01:28:52] Alex Volkov: This used to take GPU and knowledge, and there's, like Kokhya, and like this used to take Loras and before Loras, Dreambooths. So actually there's a couple of companies that I know that built on top of providing the fine tuning experience around this, where you upload images, you get like this huge, like four gigabit, like stable diffusion file specifically trained on you as a concept. [01:29:13] Alex Volkov: And now there's like a zero shot transfer thing called Instant ID. Where a hug and face demo is included here. I will attach this now soon. Where you just upload one image of yourself. Literally for me and Nishtha and Tanishq, for the non on, Umesh, for the non anons here on stage, we'd be able to use our profile picture here and just generate us with a cowboy hat in, in noir style and it will look like us. [01:29:36] Alex Volkov: For most of the time. I've tested this Instant ID on my kids. And, I'm not going to post this because of privacy. But my kid loved it incredibly so much. He was a superman. It looked like him. It's unbelievable. That it was, like, able to transfer this with one image. It's quite incredible how fast we moved here. [01:29:52] Alex Volkov: Definitely, if you haven't tried Instant ID but you have tried avatars before, Try Instant ID, you'll be blown away. It runs on your Mac as well, not that great, but it runs through Pinocchio computer. Definitely worth noticing how fast we're moving in this generation. And shout out to whoever built this. [01:30:08] Alex Volkov: And there's quite a few technologies like this now. Highlighting how fast we're moving, and I think that's pretty much it. [01:30:15] Voice and Audio - New tech challenges Whisper [01:30:15] Alex Volkov: So we've covered our diffusion. We've covered yeah, let's move to voice and audio Nistan, you brought us this new, so I definitely want you to pull up the tweet and let's talk about the faster encoder ASR. [01:30:25] Alex Volkov: And then we can also, while maybe you pull this up, I will say that this week I've 11Labs announced like a big funding rise, but 11Labs also released their dubbing studio. And if you followed Twitter at all, not even the I Twitter for the past like week and a half, two weeks, you maybe have seen the dubbed video of the Argentinian prime minister, or I don't know if he's a prime minister or president, probably president, right? [01:30:55] Alex Volkov: Yes, president. Millet something he went to the World Economic Forum and gave a speech in Spanish. And then there was a dubbed version, as like these meetings of global summits of leaders, et cetera, they have. Instant translation in their ear to any language, and that's a human that knows both languages. [01:31:14] Alex Volkov: And then, somebody said, hey, okay, this is one example, and they posted a Heijan. If you remember Heijan, we've talked about Heijan, quite incredibly translation, dubbing, and leap modulation service, where you can upload yourself and get an instant avatar. Somebody used Heijan on the whole speech. [01:31:29] Alex Volkov: And that went ridiculously viral. I think there was like 50 million views on it, on X. And that was like mostly a combination of [01:31:40] Millet being like very viral in his opinions, being like, stoking some controversy. But also because you literally hear the person. Speak in English with a Spanish accent where this didn't happen, like literally he spoke in Spanish. [01:31:52] Alex Volkov: Quite incredible technology and people have been shocked and said, Oh my God, this is coming for all of us in DeepFakes. Fine, we've talked about this multiple times. So Eleven Labs now has a, like a alternative to this, called Eleven Labs Dubbing Studio. And I've actually used this on a piece of Like on a trailer for ThursdAI, of me speaking in English, and they asked to dub me in Russian, the language that I do speak, and my mother tongue from Ukraine, and it sounded ridiculously cool. [01:32:18] Alex Volkov: Here's a quick snippet of me from a Thursday I show with you three weeks ago that I dubbed into Russian for your entertainment. [01:32:28] Gadget for children, for parents who have children who do not want to buy iPhones. Because then Instagram will destroy their brains. This is the perfect device for this. [01:32:36] It looks like a language. In fact, you can talk to a rabbit, it is very cute, there is one simple interface, this is a voice. [01:32:43] Alex Volkov: It sounded like, so far, How should I say, these models that emulate voice did not work on me. Specifically, my accent is not that great, but because my accent is probably Russian, the Russian version of me sounded really close to me. [01:32:54] Alex Volkov: For the first time, I was like, Oh, okay. All right. And Eleven Labzner released this dubbing studio and hopefully these models are now coming to open source. [01:33:04] AI deepfake of Biden caused controversy on mass media about AI [01:33:04] Alex Volkov: Because there's also a thing where I think there's a recording of Biden saying something like stay home going around and everybody in the media making the big fuss about, Oh my God, AI is coming for all of us. [01:33:15] Alex Volkov: And there's a big cry for folks to say, we should build tools to detect against this, et cetera. And my stance remains the same. Listen, I think we've talked about this multiple times. The only way through these woods is for everybody to know that their voice is very easily be fakable with three seconds or 10 seconds of their voice. [01:33:31] Alex Volkov: It's time for the it's time for humanity to adapt to the situation where there's no panacea here. You should just know that just trusting voice blindly without knowing the source just don't do that because it might as well be fake. I don't know if you want to add anything. [01:33:44] Alex Volkov: Yeah, go ahead. [01:33:45] Nisten Tahiraj: really quick, I want to say, we already have laws to deal with this. More law is not necessarily going to fix the issue because, fraud is illegal in a free market. And if you want. Or at least people that are more in politics and stuff. If you want to solve the issue, do the job you already have. [01:34:05] Nisten Tahiraj: You already have a list of spam callers, which you have been identified without an AI. And can you shut them down? So People love to imagine problems and love to think of doom or whatever in the future and then they completely ignore the stuff in front of them. All of us do this, but yeah, again, fraud is illegal. [01:34:27] Nisten Tahiraj: Can you shut it down as a job, as a government? You don't need a new law, you don't need to be make speeches about AI. You need, just need to shut down fraud when it's identified. Otherwise, all of these tools and conferences and stuff are pointless. [01:34:42] Alex Volkov: As predicted. [01:34:43] Nisten Tahiraj: that's what I'm gonna [01:34:44] Alex Volkov: Yeah, no, that's great. As predicted, the first. Election related deepfake type thing. The media was all over this and the doomers were like, here we go. And people were like it came sooner than we thought. And no, we've literally been talking about this for the past year. [01:34:57] Alex Volkov: That like elections are coming. These things are going to happen. The technology was there even before. Now it's just like a little bit more accessible. The laws are in place, make it more difficult for grandmas to get spam calls, not make it difficult for the open source stuff. So hopefully like the more prevalent these technologies are, this is my stance, the better the chance that, people will just get used to this being everywhere. [01:35:19] Alex Volkov: And definitely for folks of us who have our audio out there, we're doomed, right? So come up, like my usual suggestion here is come up with your loved ones with a key phrase that only you to know like. The Terminator scene with the dog come up with this and make sure that if you get a call in 3 a. [01:35:34] Alex Volkov: m. at night, it sounds like a bad quality version of you, of your relative from somewhere, from an unknown phone. Make sure it's them by asking like, Hey, remember we went to Hawaii and you never went to Hawaii? And they say, Oh yeah, of course. But also you can probably most of those will be LLMs, so you can probably like. [01:35:53] Alex Volkov: Don't prompt trick them, the spammy LLM calls that sound like you're a relative. [01:35:57] W2V BERT ASR gets whisper quality with significantly less parameters [01:35:57] Alex Volkov: Alright, moving for unless, listen, you want to add some stuff about this W2V BERT speech encoder? I've added it to the top of the space. [01:36:07] Nisten Tahiraj: Yeah, just really quickly, I'm gonna do the paper reading on it 'cause [01:36:10] Alex Volkov: Oh, hell yeah! [01:36:11] Nisten Tahiraj: It's a pretty nice paper, so stay tuned from that at some point when we announce it and it's from MITs and and Google and some people from Google. So it's a, another really nice encoder only it has potentially seems to be up to 30 times faster. [01:36:29] Nisten Tahiraj: So this could [01:36:30] Alex Volkov: then whisper, [01:36:31] Nisten Tahiraj: quite useful. It could be quite useful for those making assistance that run on local devices or on low resource devices. But also, For stuff on the web. Now it is officially supported by the Transformers library. We'll wait on Zenova to I think probably it's going to be available via WebGPU and stuff, I'm guessing. [01:36:55] Nisten Tahiraj: Yeah it's very, it's nice to see that that field also going forward. Because we already have excellent speech recognition. We know it works really well. We just needed to work on more low power devices and mobile and [01:37:08] Alex Volkov: Absolutely. And looks like some stats here. A bunch of languages are more than the Stan Whisperer, 143 languages. And you can like fine tune this on specific languages as well to make it like better. And VB benchmarked it on Mongolian, and beat Whisperer in less than 1200 steps. So smaller model, like fine tunable, super, super cool, and the best part of it is MIT license. [01:37:29] Alex Volkov: So there have been other ASRs. They're not in this license. And now we're getting like a state of the art tiny model in this license. I think that's most of the stuff that I wanted to cover. [01:37:39] NSF announces a new initiative called NAIRR [01:37:39] Alex Volkov: No, I wanted to cover one last thing. One last thing. National Artificial Intelligence Research Resource. N A I R R. [01:37:47] Alex Volkov: Which is coming to us from National Science Foundation, United States National Science Foundation collaborating with agencies and different so All of these incredible three letter agencies are collaborations in this foundation now. NSF is the science foundation, both DARPA and NASA, and NIST, which is the Institute of Standards and Technology, and DOD and DOE, and, like, all these things. [01:38:11] Alex Volkov: But also, the private sector is joining this companies like Entropic and OpenAI. And Palantir, and Google, and Luther, and HugInFace, and Weights Biases. Obviously, I saw this oh, that's cool. We're, like, Weights Biases are participating in this incredible effort. Are all joining together in this initiative to, to promote, support AI research and advancing like safe and secure and trustworthy AI. [01:38:33] Alex Volkov: And it's also great to see like folks like Hug Face here and Meta as well is represented folks who push open source as well, because, these government affiliations, government organizations, they have to have folks who promote open source as well. And they've organized them to. Four focus areas open enable AI research to access into diverse AI resources via the NAIRR pilot portal. [01:38:56] Alex Volkov: So definitely expect there to be government grants for GPUs for different things, I don't know how easily those will be obtainable, but we had some folks in Canada from Canada before talked about you could ask for grants. to train or fine tune like the stuff that Tanish was talking about research which open source is better medical in QA could be happening through the government they also focus on security and And I think something called NARR classroom, which I have no idea. [01:39:22] Alex Volkov: Oh, which new communities for education, training and user support. Like very government like approached. However, this is definitely like good to see the companies that participate in this. It's not only government, it's also open, like a private sector as well. NVIDIA is there, AMD is there, Eleuther, like we said, open source folks are represented as well. [01:39:43] Alex Volkov: A huge kind of chunk of companies, it's good to see that the government is like actually moving towards some standardization which may be needed hopefully less regulation, more standardization. And I think with that, we are pretty much all over the news that we had for [01:40:00] this week. Which was great. [01:40:01] Alex Volkov: I want to say thank you. A huge thank you again for, first of all, the listeners who come here and listen, and the folks on stage who help me from week to bring you the latest and greatest in the iNews. [01:40:11] Alex Volkov: Thank you so much, and we'll let you go on this Thursday, and we'll see you next week. [01:40:14] Alex Volkov: Take care, everyone. Bye bye. This is a public episode. If you'd like to discuss this with other subscribers or get access to bonus episodes, visit sub.thursdai.news/subscribe | |||
02 Feb 2024 | ThursdAI - Feb 1, 2024- Code LLama, Bard is now 2nd best LLM?!, new LLaVa is great at OCR, Hermes DB is public + 2 new Embed models + Apple AI is coming 👀 | 01:22:35 | |
TL;DR of all topics covered + Show notes * Open Source LLMs * Meta releases Code-LLama 70B - 67.8% HumanEval (Announcement, HF instruct version, HuggingChat, Perplexity) * Together added function calling + JSON mode to Mixtral, Mistral and CodeLLama * RWKV (non transformer based) Eagle-7B - (Announcement, Demo, Yam's Thread) * Someone leaks Miqu, Mistral confirms it's an old version of their model * Olmo from Allen Institute - fully open source 7B model (Data, Weights, Checkpoints, Training code) - Announcement * Datasets & Embeddings * Teknium open sources Hermes dataset (Announcement, Dataset, Lilac) * Lilac announces Garden - LLM powered clustering cloud for datasets (Announcement) * BAAI releases BGE-M3 - Multi-lingual (100+ languages), 8K context, multi functional embeddings (Announcement, Github, technical report) * Nomic AI releases Nomic Embed - fully open source embeddings (Announcement, Tech Report) * Big CO LLMs + APIs * Bard with Gemini Pro becomes 2nd LLM in the world per LMsys beating 2 out of 3 GPT4 (Thread) * OpenAI launches GPT mention feature, it's powerful! (Thread) * Vision & Video * 🔥 LLaVa 1.6 - 34B achieves SOTA vision model for open source models (X, Announcement, Demo) * Voice & Audio * Argmax releases WhisperKit - super optimized (and on device) whisper for IOS/Macs (X, Blogpost, Github) * Tools * Infinite Craft - Addicting concept combining game using LLama 2 (neal.fun/infinite-craft/) Haaaapy first of the second month of 2024 folks, how was your Jan? Not too bad I hope? We definitely got quite a show today, the live recording turned into a proceeding of breaking news, authors who came up, deeper interview and of course... news. This podcast episode is focusing only on the news, but you should know, that we had deeper chats with Eugene (PicoCreator) from RWKV, and a deeper dive into dataset curation and segmentation tool called Lilac, with founders Nikhil & Daniel, and also, we got a breaking news segment and (from ) joined us to talk about the latest open source from AI2 👏 Besides that, oof what a week, started out with the news that the new Bard API (apparently with Gemini Pro + internet access) is now the 2nd best LLM in the world (According to LMSYS at least), then there was the whole thing with Miqu, which turned out to be, yes, a leak from an earlier version of a Mistral model, that leaked, and they acknowledged it, and finally the main release of LLaVa 1.6 to become the SOTA of vision models in the open source was very interesting! Open Source LLMs Meta releases CodeLLama 70B Benches 67% on MMLU (without fine-tuninig) and already available on HuggingChat, Perplexity, TogetherAI, Quantized for MLX on Apple Silicon and has several finetunes, including SQLCoder which beats GPT-4 on SQL Has 16K context window, and is one of the top open models for code Eagle-7B RWKV based model I was honestly disappointed a bit for the multilingual compared to 1.8B stable LM , but the folks on stage told me to not compare this in a transitional sense to a transformer model ,rather look at the potential here. So we had Eugene, from the RWKV team join on stage and talk through the architecture, the fact that RWKV is the first AI model in the linux foundation and will always be open source, and that they are working on bigger models! That interview will be released soon Olmo from AI2 - new fully open source 7B model (announcement) This announcement came as Breaking News, I got a tiny ping just before Nathan dropped a magnet link on X, and then they followed up with the Olmo release and announcement. A fully open source 7B model, including checkpoints, weights, Weights & Biases logs (coming soon), dataset (Dolma) and just... everything that you can ask, they said they will tell you about this model. Incredible to see how open this effort is, and kudos to the team for such transparency. They also release a 1B version of Olmo, and you can read the technical report here Big CO LLMs + APIs Mistral handles the leak rumors This week the AI twitter sphere went ablaze again, this time with an incredibly dubious (quantized only) version of a model that performed incredible on benchmarks, that nobody expected, called MIQU, and i'm not linking to it on purpose, and it started a set of rumors that maybe this was a leaked version of Mistral Medium. Remember, Mistral Medium was the 4th best LLM in the world per LMSYS, it was rumored to be a Mixture of Experts, just larger than the 8x7B of Mistral. So things didn't add up, and they kept not adding up, as folks speculated that this is a LLama 70B vocab model etc', and eventually this drama came to an end, when Arthur Mensch, the CEO of Mistral, did the thing Mistral is known for, and just acknowleged that the leak was indeed an early version of a model, they trained once they got access to their cluster, super quick and that it indeed was based on LLama 70B, which they since stopped using. Leaks like this suck, especially for a company that ... gives us the 7th best LLM in the world, completely apache 2 licensed and it's really showing that they dealt with this leak with honor! Arthur also proceeded to do a very Mistral thing and opened a pull request to the Miqu HuggingFace readme with an attribution that looks like this, with the comment "Might consider attribution" 🫳🎤 Bard (with Gemini Pro) beats all but the best GPT4 on lmsys (and I'm still not impressed, help) This makes no sense, and yet, here we are. Definitely a new version of Bard (with gemini pro) as they call it, from January 25 on the arena, now is better than most other models, and it's could potentially be because it has internet access? But so does perplexity and it's no where close, which is weird, and it was a weird result that got me and the rest of the team in the ThursdAI green room chat talking for hours! Including getting folks who usually don't reply, to reply 😆 It's been a great conversation, where we finally left off is, Gemini Pro is decent, but I personally don't think it beats GPT4, however most users don't care about which models serves what, rather which of the 2 choices LMSYS has shown them answered what they asked. And if that question has a google search power behind it, it's likely one of the reasons people prefer it. To be honest, when I tried the LMSYS version of Bard, it showed me a 502 response (which I don't think they include in the ELO score 🤔) but when I tried the updated Bard for a regular task, it performed worse (in my case) than a 1.6B parameter model running locally. Folks from google replied and said that it's not that they model is bad, it's that I used a person's name, and the model just.. refused to answer. 😵💫 When I removed a last name it did perform ok, no where near close to GPT 4 though. In other news, they updated Bard once again today, with the ability to draw images, and again, and I'm sorry if this turns to be a negative review but, again, google what's going on? The quality in this image generation is subpar, at least to mea and other folks, I'll let you judge which image was created with IMAGEN (and trust me, I cherry picked) and which one was DALLE for the same exact prompt This weeks Buzz (What I learned with WandB this week) Folks, the growth ML team in WandB (aka the team I'm on, the best WandB team duh) is going live! That's right, we're going live on Monday, 2:30 PM pacific, on all our socials (X, LinkedIn, Youtube) as I'm hosting my team, and we do a recap of a very special week in December, a week where we paused other work, and built LLM powered projects for the company! I really wanted to highlight the incredible projects, struggles, challenges and learnings of what it takes to take an AI idea, and integrated it, even for a company our size that works with AI often, and I think it's going to turn out super cool, so you all are invited to check out the live stream! Btw, this whole endeavor is an initiative by yours truly, not like some boring corporate thing I was forced to do, so if you like the content here, join the live and let us know how it went! OpenAI releases a powerful new feature, @mentions for GPTs This is honestly so great, it went under the radar for many folks, so I had to record a video to expalin why this is awesome, you can now @mention GPTs from the store, and they will get the context of your current conversation, no longer you need to switch between GPT windows. This opens the door for powerful combinations, and I show some in the video below: Apple is coming to AI Not the Apple Vision Pro, that's coming tomorrow and I will definitely tell you how it is! (I am getting one and am very excited, it better be good) No, today on the Apple earnings call, Tim Cook finally said the word AI, and said that they are incredibly excited about this tech, and that we'll get to see something from them this year. Which makes sense, given the MLX stuff, the Neural Engine, the Ml-Ferret and the tons of other stuff we've seen from them this year, Apple is definitely going to step in a big way! Vision & Video LLaVa 1.6 - SOTA in open source VLM models! (demo) Wow, what a present we got for Haotian Liu and the folks at LLaVa, they upgraded the LlaVa architecture and released a few more models, raging from 7B to 34B, and created the best open source state of the art vision models! It's significantly better at OCR (really, give it a go, it's really impressive) and they exchanged the LLM backbone with Mistral and Hermes Yi-34B. * Better OCR and higher res * Uses several bases like Mistral and NousHermes 34B * Uses lmsys SGlang for faster responses (which we covered a few weeks ago) * SoTA Performance! LLaVA-1.6 achieves the best performance compared with open-source LMMs such as CogVLM or Yi-VL. Compared with commercial ones, it catches up to Gemini Pro and outperforms Qwen-VL-Plus on selected benchmarks. * Low Training Cost. LLaVA-1.6 is trained with 32 GPUs for ~1 day, with 1.3M data samples in total. The compute / training data cost is 100-1000 times smaller than others. Honestly it's quite stunningly good, however, it does take a lot more GPU due to the resolution changes they made. Give it a try in this online DEMO and tell me what you think. Tools This isn't a tool, but an LLM based little game that's so addicting, I honestly didn't have time to keep playing it, and it's super simple. I especially love this, as it's uses LLama and I don't see how something like this could have been scaled without AI before, and the ui interactions are so ... tasty 😍 All-right folks, I can go on and on, but truly, listen to the whole episode, it really was a great one, and stay tuned for the special sunday deep dive episode with the folks from Lilac and featuring our conversation with about RWKV. If you scrolled all the way until here, send me the 🗝️ emoji somewhere in DM so I'll know that there's at least one person who read this through, leave a comment and tell 1 friend about ThursdAI! This is a public episode. If you'd like to discuss this with other subscribers or get access to bonus episodes, visit sub.thursdai.news/subscribe | |||
05 Feb 2024 | 📖 ThursdAI - Sunday special on datasets classification & alternative transformer architectures | 00:50:37 | |
Hello hello everyone, welcome to another special episode (some podcasts call them just.. episodes I guess, but here you get AI news every ThurdsdAI, and on Sunday you get the deeper dives) BTW, I'm writing these words, looking at a 300 inch monitor that's hovering above my usual workstation in the Apple Vision Pro, and while this is an AI newsletter, and I've yet to find a connecting link (there's like 3 AI apps in there right now, one fairly boring chatbot, and Siri... don't get me started on Siri), I'll definitely be covering my experience in the next ThursdAI, because well, I love everything new and technological, AI is a huge part of it, but not the ONLY part! 📖 It's all about the (big) Datasets Ok back to the matter at hand, if you've used, finetuned, trained or heard about an AI model, you may or may not realize how important the dataset the model was trained with is. We often talk of this model, that model, and often the only different is, additional data that folks (who I sometimes refer to as alchemists) have collected, curated and structured, and creating/curating/editing those datasets is an art and a science. For example, three friends of the pod, namely LDJ with Capybara, Austin with OpenChat and Teknium with Hermes, have been consistently taking of the shelves open source models and making them smarter, more instruction tuned, better for specific purposes. These datasets are paired with different techniques as well, for example, lately the so-called DPO (Direct preference optimization) is a technique that showed promise, since it not only shows a model which answer is the correct for a specific query, it shows an incorrect answer as well, and trains the model to prefer one over the other. (see the recent Capybara DPO improvement by Argilla, which improved model metrics across every evaluation) These datasets can range from super high quality 16K rows, to millions of rows (Teknium's recently released Hermes, one of the higher quality datasets comes in at just a tad over exactly 1 million rows) and often times it's an amalgamation of different other datasets into 1. In the case of Hermes, Teknium has compiled this 1 million chats from at least 15 different datasets, some his own, some by folks like Jon Durbin, Garage bAInd, and shareGPT, from LMsys.org, which was complied by scraping the very popular sharegpt.com website, from folks who used the shareGPT extension to share they GPT4 conversations. It's quite remarkable how much of these datasets are just, conversations that users had with GPT-4! Lilac brings Garden With that backdrop of information, today on the pod we've got the co-founders of Lilac, Nikhil Thorat and Daniel Smilkov, who came on to chat about the new thing they just released called Lilac Garden. Lilac is an open source tool (you can find it RIGHT HERE) which is built to help make dataset creation, curation and classification, more science than art, and help visualize the data, cluster it and make it easily available. In the case of Hermes, that could be more than millions of rows of data. On the pod, I talk with Nikhil and Daniel about the origin of what they both did at Google, working on Tensorflow.js and then something called "know your data" and how eventually they realized that in this era of LLMs, open sourcing a tool that can understand huge datasets, run LLM based classifiers on top of them, or even train specific ones, is important and needed! To strengthen the point, two friends of the pod (Teknium was in the crowd sending us 👍), LDJ and Austin (aka Alignment Lab) were on stage with us and basically said that "It was pretty much the dark ages before Lilac", since something like OpenOrca dataset is a whopping 4M rows of text. Visualizations in the Garden. So what does lilac actually look like? Here's a quick visualization of the top categories of texts from OpenOrca's 4 million rows, grouped by category title and showing each cluster. So you can see here, Translation requests have 66% (around 200K rows) of the translation category, and you can scroll on and on and add filters and really dissect this whole thing up and down. The categorization is created by running Lilac on your dataset, which uses embedding algorithms and other neat tricks to quickly chunk and put labels on the categories (AKA classifying them). Btw, you can see this view and play around with it yourself, here But running this on your own local machine can be a drag, and take hours if not days for bigger datasets, including sometimes hanging and not even working 100%, so the Lilac folks created Lilac Garden, which is a hosted solution by them to provide a dataset, and do classify something like 4M in 4-5 hours or so. Which is definitely not possible on local machines. If you're into that kind of thing, again, Lilac is open source ,so you don't have to sign up or pay them, but if speed and this view matters to you, definitely check Lilac out! RWKV with Eugene (Pico Creator) On the news segment of ThursdAI we mentioned Eagle, which is the 5th version of RWKV, an attention free, potential alternative to Transformers, that's being developed fully in the open source. Later in the show we had the honor to have PicoCreator, one of the front running folks in the RWKV effort, which is an attempt to see if Transformers can be beat with a new type of architecture (RNN) that doesn't require specific attention mechanisms, that add the problem of Quadratic Attention scaling, making LLMs hard and expensive to run the more context is provided. Eugene had some technical issues so joined in the middle of the pod, so we didn't have a full deep-dive, however, I figured it's important to bring this info to you guys, as these efforts may yield AI that runs 10-100x cheaper and potentially faster on devices, using almost infinite context lengths. RWKV and other attempts like StripedHyena (Together AI) and Mamba (from Tri Dao) are attempts that are worth watching as they may supersede or join with Transformers to create the next jump in LLM capabilities. That's all for this Sunday, needless to say, with the Vision Pro releasing on a Friday, it's been a full weekend of future exploration, which is the main driver in my personal life! P.S - if you read through to here, you get a gift! A teaser, I have done something different on the pod, recorded a human interest podcast x AI, for the first time. I mostly bring the news and sometimes deep dives like this one, but this story I couldn't ignore, so stay tuned if you're into dating x AI, and how technology disrupts our lives and wether this is all moral or not, as I recorded an Episode with Sasha Jadan and his new Fiancee Karina, which his AI bot picked out for him, after swiping and matching with over 5200 girls on Tinder. The AI also... suggested he'd propose which he did. It was a very interesting conversation that I plan to upload soon! That's it from me this week, see you all on ThursdAI and don't forget, if you liked this, do me a solid, listen to the pod and then leave a review or a 5 star (at least a 4?) on Apple podcasts 🙏 This is a public episode. If you'd like to discuss this with other subscribers or get access to bonus episodes, visit sub.thursdai.news/subscribe | |||
09 Feb 2024 | 📅 ThursdAI - Feb 8 - Google Gemini Ultra is here, Qwen 1.5 with Junyang and deep dive into ColBERT, RAGatouille and DSPy with Connor Shorten and Benjamin Clavie | 01:53:51 | |
Hihi, this is Alex, from Weights & Biases, coming to you live, from Yosemite! Well, actually I’m writing these words from a fake virtual yosemite that appears above my kitchen counter as I’m not a Vision Pro user and I will force myself to work inside this thing and tell you if it’s worth it. I will also be on the lookout on anything AI related in this new spatial computing paradigm, like THIS for example! But back to rfeality for a second, we had quite the show today! We had the awesome time to have Junyang Justin Lin, a dev lead in Alibaba, join us and talk about Qwen 1.5 and QwenVL and then we had a deep dive into quite a few Acronyms I’ve been seeing on my timeline lately, namely DSPy, ColBERT and (the funniest one) RAGatouille and we had a chat with Connor from Weaviate and Benjamin the author of RAGatouille about what it all means! Really really cool show today, hope you don’t only read the newsletter but listen on Spotify, Apple or right here on Substack. TL;DR of all topics covered: * Open Source LLMs * Alibaba releases a BUNCH of new QWEN 1.5 models including a tiny .5B one (X announcement) * Abacus fine-tunes Smaug, top of HF leaderboard based Qwen 72B (X) * LMsys adds more open source models, sponsored by Together (X) * Jina Embeddings fine tune for code * Big CO LLMs + APIs * Google rebranding Bard to Gemini and launching Gemini Ultra (Gemini) * OpenAI adds image metadata (Announcement) * OpenAI keys are now restricted per key (Announcement) * Vision & Video * Bria - RMBG 1.4 - Open Source BG removal that runs in your browser (X, DEMO) * Voice & Audio * Meta voice, a new apache2 licensed TTS - (Announcement) * AI Art & Diffusion & 3D * Microsoft added DALL-E editing with "designer" (X thread) * Stability AI releases update to SVD - video 1.1 launches with a webUI, much nicer videos * Deep Dive with Benjamin Clavie and Connor Shorten show notes: * Benjamin's announcement of RAGatouille (X) * Connor chat with Omar Khattab (author of DSPy and ColBERT) - Weaviate Podcast * Very helpful intro to ColBert + RAGatouille - Notion Open Source LLMs Alibaba releases Qwen 1.5 - ranges from .5 to 72B (DEMO) With 6 sizes, including 2 new novel ones, from as little as .5B parameter models to an interesting 4B, to all the way to a whopping 72B, Alibaba open sources additional QWEN checkpoints. We've had the honor to have friend of the pod Junyang Justin Lin again, and he talked to us about how these sizes were selected, that even thought this model beats Mistral Medium on some benchmarks, it remains to be seen how well this performs on human evaluations, and shared a bunch of details about open sourcing this. The models were released with all the latest and greatest quantizations, significantly improved context length (32K) and support for both Ollama and Lm Studio (which I helped make happen and am very happy for the way ThursdAI community is growing and connecting!) We also had a chat about QwenVL Plus and QwebVL Max, their API only examples for the best open source vision enabled models and had the awesome Piotr Skalski from Roborflow on stage to chat with Junyang about those models! To me a success of ThursdAI, is when the authors of things we talk about are coming to the show, and this is Junyang second appearance, which he joined at midnight at the start of the chinese new year, so greately appreciated and def. give him a listen! Abacus Smaug climbs to top of the hugging face leaderboard Junyang also mentioned that Smaug is now at the top of the leaderboards, coming from Abacus, this is a finetune of the previous Qwen-72B, not even this new one. First model to achieve an average score of 80, this is an impressive appearance from Abacus, though they haven't released any new data, they said they are planning to! They also said that they are planning to finetune Miqu, which we covered last time, the leak from Mistral that was acknowledged by Arthur Mensch the CEO of Mistral. The techniques that Abacus used to finetune Smaug will be released an upcoming paper! Big CO LLMs + APIs Welcome Gemini Ultra (bye bye Bard) Bard is no longer, get ready to meet Gemini. it's really funny because we keep getting cofusing naming from huge companies like Google and Microsoft. Just a week ago, Bard with Gemini Pro shot up to the LMSYS charts, after regular gemini pro API were not as close. and now we are suppose to forget that Bard even existed? 🤔 Anyhow, here we are, big G answer to GPT4, exactly 10 months 3 weeks 4 days 8 hours, but who's counting? So what do we actually get? a $20/m advanced tier for Gemini Advanced (which will have Ultra 1.0) the naming confusion continues. We get a longer context (how much?) + IOS and android apps (though I couldn't find it in IOS, maybe it wasn't yet rolled out) Gemini now also replaces google assistant for those with androids who opt in (MKBHD was somewhat impressed but not super impressed) but google is leaning into their advantage including home support! * Looks like Gemini is ONLY optimized for English as well We had quite the conversation on stage from folks who upgraded and started using, including noticing that Gemini is a better role player, and less bland, but also that they don't yet support uploading documents besides images, and that the context window is very limited, some said 8K and some 32K but definitely on the lower side. Also from Google : a llama.cpp wrapper called localllm (Blog) OpenAI watermarks DALL-E images and adds per key API limits (finally) (Blog) OpenAI's using something calledC2PA for pictures made by DALL-E 3, whether you're chatting with ChatGPT or using their API. It's a way to show that DALL-E 3 actually created those images. But it's just for images right now, not for text or voice stuff. Adding this info can make the files up to 32% bigger, but it doesn't mess with the quality. The tags tell you if the source was DALL-E 3, ChatGPT, or the API by including special signatures and stuff. Just a heads up, though, this C2PA thing isn't perfect. The metadata could get wiped either on purpose or by mistake. They also released an update to the developer experience that allows you to track usage but also restrict usage per API key! Very very needed and helpful! This weeks Buzz (What I learned with WandB this week) First part of the live series with the Growth ML team was live and AWESOME! Vision BRIA - Open-Source background removal (non commercial) 📷 Introducing Open-Source Background Removal by @BriaAI 📷 Now live on @huggingface, RMBG v1.4 excels in separating foreground from background across diverse categories, surpassing current open models. See demo [https://t.co/DDwncjkYqi] #BriaAI #OpenSource #AI @briaai https://t.co/BlhjMMNWxa Voice MetaVoice (hub) 1.2B parameter model.Trained on 100K hours of data.Supports zero-shot voice cloning.Short & long-form synthesis.Emotional speech.Best part: Apache 2.0 licensed. 🔥 Powered by a simple yet robust architecture: > Encodec (Multi-Band Diffusion) and GPT + Encoder Transformer LM. > DeepFilterNet to clear up MBD artefacts. That's it for us this week, this time I bring you both the news segment AND the deepdive in one conversation, hope it's not super long, see you here next ThursdAI! 👏 Full Transcript: [00:00:00] Intro and housekeeping [00:00:00] [00:00:00] Alex Volkov: You're on ThursdAI, and I think it's time for us to get started with the recording and the introduction. [00:00:26] Alex Volkov: Happy, happy Thursday everyone! Today is February 8th, 2024. I don't know, This is the second calendar year the Thursday is happening in, so I don't know if I need to mention the year or not but we're well on our way into 2024 and you're here on Thursday, I, the Thursday I is the space, the newsletter, and the podcast to keep you up to date with all of the very interesting things that are happening in the very fast moving world of ai. [00:00:58] Alex Volkov: Hopefully by now, all of you already have ThursdAI in your podcast, wherever you get a podcast, Spotify, recently YouTube as well, which is weird. But with this introduction, I will just say, hello myself, basically. Hey everyone. My name is Alex Volkov. I'm an AI evangelist with Weights & Biases. [00:01:15] Alex Volkov: Weights & Biases is the reason why this comes to life to you. And there's going to be a little segment about Weights & Biases in the middle here as well, and I'm joined on stage. Often, and pretty much every week by great friends, experts in their fields. As we talk about everything AI related this week, especially we're going to have some interesting things. [00:01:34] Alex Volkov: Those of you who come back week after week. Thank you, and we love that you're part of the community, and it's great to see how many people just return, and those of you who are new, we're here every week and The community doesn't stop after we finish the space. There's a bunch of spaces. I think our friend AlignmentLab had the space that went on for the full week, I think. [00:01:55] Alex Volkov: I don't know if he ever slept. That's maybe why he's not here on stage. But we're here every week for the two hours to give you updates for the first hour and definitely some very interesting deep dives that has been happening, that have been happening for the past few Weeks, I want to say, so I just want to shout out some friends of ours that recently we were featured in the deep dives. [00:02:16] Alex Volkov: We've talked with Maxime Lubon, who trained the Beagle series and then also gave a deep dive with us about model merging. That was really fun. And on the last deep dive, we talked with the Lilac folks and they're building an open source tool. That lets you peer into huge data sets, like imagine millions of rows, data sets, and they chunk and cluster this. And we've talked about the importance of data sets in creation of LLMs or large language models. [00:02:46] Alex Volkov: And they've taken the huge data sets of the folks to usually come up on ThursdAI. Technium from Nous Research just released their Hermes dataset, for example. And the folks in Lilac talked to us about how that would be visualized and how you can see which parts of it is comprised of. [00:03:03] Alex Volkov: It's quite an interesting conversation about how to approach the training and fine tuning area. And we haven't often talked about dataset curation and creation, so that conversation was a very nice one. So we have deep dives. I will say that last weekend, I also interviewed, and that's probably going to come up as a separate episode. [00:03:24] Alex Volkov: I interviewed Sasha Zhadan from Moscow, and this was a first for me. And I just want to like, highlight where this weird thing takes me, because that's not ThursdAI, and that's not about the news. That was just literally about AI stuff. So this guy from Moscow, and this will be dropping on ThursdAI podcast soon. [00:03:42] Alex Volkov: This guy from Moscow built a bot that auto swipes for him on Tinder. And that bot started using gpt instruct, and then moved to gpt chat, gpt etc, and then moved to gpt 4. And he talks about how this bot kept improving with the improvement of AI. And then he autoswiped a wife, basically. And then this was, this took over the Russian ex. [00:04:08] Alex Volkov: I don't know if you guys are on the Russian side of ex, but I definitely noticed that everybody, that's all they could talk about. This guy Previously also did some shenanigans with OpenAI stuff. And so it was a very interesting conversation, unlike anything that I did previously on ThursdAI. [00:04:21] Alex Volkov: And definitely that's coming more as a human interest story than anything else. But it's very interesting. And also his fiance also joined and we talked about the morality of this as well. And it was really fun. So if that kind of new type of content also interests you definitely check out. [00:04:37] Alex Volkov: That's probably not going to end up on X. [00:04:40] Alex Volkov: And I think with this, it's time to get started. , The usual way we get started here is I just run through everything that we have. Just so you know what we're going to talk about. [00:04:52] Alex Volkov: And then we're going to start with segment by segment. So that's [00:04:54] TL;DR and recap of the conversation [00:04:54] Alex Volkov: Hey everyone, this is a recap of everything we talked about on ThursdAI for February 8th. 2024 and we had a bunch of breaking new stuff today, specifically around the fact that Google finally gave us something. But I'm gonna do this recap properly based on the categories. So let's go. So in the category of open source lms, we've talked about Alibaba releases a bunch of new Qwen models, specifically under the numbering 1.5. [00:05:33] Alex Volkov: And we had the great pleasure again to talk with Justin J. Yang Lin. from Qwen team the guy who's a tech lead there and pushes for open source. And he came up and talked about why this is a 1. 5 model, not a 2 model. He also talked about the fact that they released a tiny 0. [00:05:51] Alex Volkov: 5 billion one. This is like a very tiny. Large language model. I think it's really funny to say a tiny large language model, but this is the case. And he talked about multiple releases for Qwen. We also had, friend of the pod, Piotr Skalski from Roboflow, who's like a vision expert who comes up from time to time, and the author of I forget the name of the library. [00:06:12] Alex Volkov: I will remember this and put this in the show notes as well. He came up and he had a bunch of plays with the visions part of the Qwen. ecosystem, and we've talked about QNVL plus and QNVL max with Justin as well, and we've talked about their potential for open sourcing these models. They also released a 72 billion parameter model that's now part of the top of the Hug Face leaderboard, which is super cool. [00:06:34] Alex Volkov: So definitely a great conversation. And I love it when the authors of the things that we talk about come out and talk about the, in ThursdAI. We then smooth, smoothly move to the next topic where Abacus, the company Abacus AI, there is Finetune that's now top of the Hug Face leaderboard, and that's based on QN72B, and not even the new one, the previous one, so 1. [00:06:54] Alex Volkov: 0, and that's now the top model on Hug Face leaderboard, and that has an average score of over 80. And I think it's the first open source model to do and they haven't fully released the process of what they what they used in order to make this much better in different leaderboards. But they have mentioned that they're going to train this model on top of the Mikulik over Mixtral. [00:07:17] Alex Volkov: And it's very interesting. And they also They're building some other stuff in Abacus as well. Very interesting. And then we moved to talk about LMSYS Arena. LMSYS Arena is the place that we send you to see which models users prefer better versus just the benchmarks and evaluations hung in phase. [00:07:35] Alex Volkov: LMSYS Arena added a bunch of open source models, so shout out OpenChat again. They added another Hermes the Finetune that Technium did for Hermes on top of Mixtral, and they also added a bunch of Qwen versions as well. LMSYS adds open source, so you continuously can see which models are better and don't have to judge for yourself, because sometimes it's not very easy. [00:07:55] Alex Volkov: We also covered JINA embeddings that are fine tuned for code. JINA from the company JINA AI and the representative Bo Wang who came, and he's a friend of the pod. We talked about their embeddings for code. Bo didn't show up this time, but maybe next time as well. Then we moved to big companies, LLMs and API, and definitely the conversation turned interesting, where multiple folks here on stage paid the new 20 tax, let's say from AI [00:08:20] for for the rebranded Bard now called Gemini and the launch of Gemini Ultra. [00:08:25] Alex Volkov: And we've talked about how long we've waited for Google to actually give us something like this. And now we're getting Gemini Ultra and Bard is no more, Bard is Essentially dead as a brand, and now we're getting the Gemini brand. So if you used to go to BART, now you go to Gemini, but also the brain behind this also improved. [00:08:41] Alex Volkov: So you get Gemini Pro by default for free, I think, and Gemini Ultra is going to cost you 20 bucks a month. It's free for the next two months, so you can sign up for a trial, and then you'll get Gemini Ultra. And you'll get it not only in the web interface, you also get it in iOS and Android apps. And if you're on Android, it also integrates with the Android Assistant. [00:09:00] Alex Volkov: That's pretty cool. It has a context length of not very much, I think we said 8 or 16 or so and some folks contested this in the comments, so we're still figuring out the context length, and it looks like the context length for that is Restricted with the UI, less on the API side, and Gemini Ultra did not release an API yet. [00:09:17] Alex Volkov: So we've talked about Gemini Ultra and different things there. We also covered that OpenAI adds image metadata to all DALI generations, whether through the UI or through the API, this image metadata can be stripped. So it's not a watermark per se, but it's definitely helpful. And there also the OpenAI gives us a little bit of a developer experience thing where you can restrict. [00:09:36] Alex Volkov: Per key on API keys different possibilities. So if one key gets stolen, you can lock only that one, or you can restrict it to only like a specific use as well. In the vision video category, we've talked about the new model for background removal called RMBG from Bria AI. It's not a fully commercial license, but you can play with this now. [00:09:57] Alex Volkov: There's a demo I'm going to add to the show notes. And also it runs fully on your client via the efforts of friends of the pod Zenova from Transformers. js. And it's pretty cool to have a model that removes background super like with two clicks with no back with no servers. And in the voice and audio category, we talked about MetaVoice, a new. [00:10:14] Alex Volkov: licensed Apache 2 licensed text to speech model, not from Meta, even though it's called MetaVoice, and it's funny it's pretty decent and has zero shot voice cloning which means that you can provide a piece of your voice and fairly quickly get a your voice speaking back to you generated. And we also talked about breaking news from NVIDIA AI, something called Nemo Canary 1B, which is a ASR model, Automatic Speech Recognition model, that's now top of the leaderboards on Hug Face, and it beats Whisper on everything, including specifically for four languages. [00:10:48] Alex Volkov: It's trained on 8, 500 hours 85, 000 hours of annotated audio, and it's very fast conformer encoder as well. We barely covered this, but Microsoft added DALI editing with the designer. So if you remember, Microsoft also did a rebrand. It used to be called Bing Chat, and now it's called Copilot. [00:11:07] Alex Volkov: And that Copilot now adds capabilities that don't exist in other places, like GPT, ChatGPT with DALI. So Microsoft's DALI now is involving the designer thing, and they have cool things where you can edit images. On the fly, you can click on different segmented objects from your generated image and say, Hey, redo this in a different style. [00:11:27] Alex Volkov: The video for this is super cool. I'm going to add this in the show notes. And it's very interesting to see that Mali Microsoft with their co pilots is moving away from where the capabilities is for ChatGPT exist. We also barely, briefly mentioned and glanced through this, but Stability AI released an update to stable video diffusion, including a web UI that you can use now, and it's not only a model, it's a web UI as well, and that web UI is pretty cool, if you didn't get an access to it, I'll link to the show notes, I think it's now possible to register, much nicer videos, and obviously it's in the open source. [00:11:59] Alex Volkov: as much as possible. So super cool. But the web UI shows you other people's video attempts. You can actually use their prompts to create videos of your own. They have some controls. It's very nice. Then I think we talked a little bit at the end there about Vision Pro and my experience with this as it comes to AI. [00:12:15] Alex Volkov: We didn't dive in into Vision Pro, even though this is my new, this is my new toy in life. And I'm very happy to participate in the renaissance of spatial computing. And we covered like the intersection of AI and spatial computing. And I think the very interesting part of today's ThursdAI was thanks to two new guests, Benjamin Clavy and Connor from Weaviate, and we've talked about DSPy and Colbert, or Colbert, and Ragatouille, which is a library to use Colbert embeddings. [00:12:43] Alex Volkov: And we talked about what they mean, and this was a great learning kind of experience for me. And if you see these concepts on your timeline and you have no idea what we talked about, I basically played the role of, hey, I'm the village dummy, let's say. I'm gonna re ask the question about what this means, why should we use this as well. [00:13:01] Alex Volkov: And I think this is our show today, folks. This is the quick summary. If I missed anything super big and important, please let me know. [00:13:08] Open source LLMs and AI news [00:13:08] Alex Volkov: But otherwise, I think we'll start with open source. All right, welcome to the open source corner. And I guess because the tradition of ThursdAI is Something releases, I go in the comments and say, Hey, I'm going to talk about this on ThursdAI. Do you want to join? And sometimes people say yes. And this is how we met Justin or Junyang here on stage. Junyang is the dev lead for the Qwen team and welcome Junyang. [00:13:50] Alex Volkov: It's very late where you are. So I really appreciate your time here. Please feel free to unmute and introduce yourself again. Some folks already know you, but if in case some new folks are listening to us, feel free to introduce yourself. And then let's talk about the stuff that you released. [00:14:06] New Qwen models 1.4 from Alibaba [00:14:06] Junyang Lin: Yeah. Thanks Alex. Nice to be at Thursday. ai it's a very great program for us to talk about ai. I am j Young and you can call me Justin. I'm working in the team for the LM and LMM. And we are now working for the new LLM, Qwen 1. 5, and we are also upgrading our vision language model, QwenBL, to QwenBL Plus and Max. [00:14:33] Junyang Lin: Plus and Max are not open sourced yet, but we have demos, so you can try in our HuggingFace organization, and you can find our demos, and you can try with Plus and Max. And the max is the best one, and I am very confident with the max demo. And about our language model today actually this week we are open sourcing QWAM 1. [00:14:58] Junyang Lin: 5. Maybe you previously you have noticed the QWAM 2 code inside Hugging Face target based transformers. Yeah, we are moving to new codes for you to use our QUANT models because in the past few months I have been interviewing our users and they found some problems with using our code, the original QUANT code, so I'm moving a step forward. [00:15:23] Junyang Lin: So this is why we had the QUANT 2 model, but for the model themselves actually we are still we in our judgment, we are still at the 1. 5 not 2 yet. We're still training the real Qwen 2, so this time we have Qwen 1. 5. For Qwen 1. 5 we are actually fixing a lot of problems because there are some models like 7 billion and 14 billion, there are a lot of people using these models, but they are actually quite old. [00:15:50] Junyang Lin: They were released months ago. They have some problems for Qwen 14 billion It is actually only supporting around 2 to 4K context length, which is far from enough for a lot of users. So for this time, we have upgraded all models to supporting 32, 000 tokens. And for the sizes, we have released more sizes. [00:16:15] Junyang Lin: Previously, we had 1. 8, which is the smallest one. But this time, we have 0. 5. only 0. 5. I used to think this one is just for experimental usage but there are some users in Twitter they found still 0. 5 can used to be do something so if you have any comments on [00:16:40] 0. 5 you can share the comments to me. And we also have 4 billion which is between 1. [00:16:46] Junyang Lin: 8 and 7 billion. The reason why we have 4 billion is that actually when we first released 1. 8 billion it is actually popular because they would like to deploy the small model to some devices like cell phones. but they found just 1. 8 is not good enough for them to for the applications. [00:17:07] Junyang Lin: So they want something just smaller than 7 billion, but much better than 0. 8. So we have 4 billion. Yeah. We have a wide range of sizes. These are for you to choose. And, [00:17:19] Alex Volkov: six, six models overall Junaid? [00:17:22] Junyang Lin: Yeah. Six [00:17:23] Alex Volkov: Six sizes overall, but definitely more models than this, because you also released, I think for the first time, you released quantized versions as well, correct? [00:17:32] Junyang Lin: No, but previously we have released GPDQ, [00:17:35] Alex Volkov: Oh yeah. [00:17:35] Junyang Lin: our convention, but this time I also have AWQ and also GGUF maybe GGUF is the new one admittedly, previously I don't know too much about AWQ and GGUF. This time I tried and everything is okay. So I just released the AWQ and GGUF. [00:17:52] Junyang Lin: And GGUF is the new thing for me. But it is quite popular in the community. Like Elm Studio, like you introduced. To me and I found a lot of people using gguf they use in Olama. So I collaborated with Olama. So you can now just run one line of code, like Olama run QWAM. So you can use the QWAM models with Olama and you can also use it in Elm Studio. [00:18:15] Alex Volkov: I just wanna [00:18:16] Junyang Lin: No [00:18:16] Alex Volkov: just a tiny pause here because I think first of all, to highlight the importance of this community, you guys are releasing a bunch of great models in open source, and first of all, just a Great. At testament to the community because you're listening to what folks have been saying, how they're reacting to your models and part of the Thursday aid, I was able to just introduce you to, to LM Studio and you guys work together. [00:18:37] Alex Volkov: And now the second year model drops, not only you guys already pro providing us quantized versions in four and GGF stuff. It's also very easy to start using and I think, just a shout out to you guys for thinking about this because a lot of models when they release they just release a waste file and then it's up in the community to figure out how to run them, when to run them, what's the problems. [00:18:57] Alex Volkov: And this was the issue with Gwen before. It was like harder to use and maybe only on hug and face demos. And now you guys released it with support for the most popular open source runners out there. So Ollama, if folks haven't used Ollama by now, definitely there's a CLI, just like Ollama installed this. [00:19:14] Alex Volkov: And LM Studio, which we've talked about a bunch, so shout out LM Studio. Shout out JAGS. And I'm, I was very happy to introduce both of you. So it's been great. And I've used the small model, the baby model as well. How was the reception from the community? What have you seen people do? Have there been any fine tunes already that you're excited about? [00:19:33] Junyang Lin: yeah this is a very great comment for helping us to improve. Yeah, previously like us, a lot of people just drop open source models and they just let the community to use it. But this is maybe, this may be not right, because we can do more to the community, maybe we can do things. more easily than the community users. [00:19:56] Junyang Lin: So this is why we are changing our style. We try to modify our code, try to adapt to the usages to make our models more popular. And recently I found them just gradually fine tuned our models. Previously fine tuned users are inside mainland China because they have chances to talk to us, so they will know more about our models so they, they can finally fine tune it. [00:20:24] Junyang Lin: But with the support of Lama X Tree and especially Alto wing Winland helped me a lot. Technium just introduced wing land to me, and I found some people are using X lotto to do it. I dunno if Chen I don't know if I pronounced his name he's one of the users of Qwen and he he previously got the usage of our models and then he quickly fine tuned a lot of model its name is Q U Y [00:20:54] Alex Volkov: Oh, Stable Quan. Yeah, I think I know what the guys are talking about. Stable Quan from also Nous Research [00:20:59] Junyang Lin: yeah, stableQwen I'm quite familiar with him, I just talked to him very much, and he just directly used our models, very quickly finding a series of models, and I find them, the quality are quite good. [00:21:12] Junyang Lin: So this is quite encouraging for me, because you can find people are interested in your models, they can find you in it, very fast speed, and I recently found Smog by Abacus AI, but I got no chance to talk to them because I don't know who actually built the model, but I found a small 72 billion is built on Qwen 72 billion [00:21:37] Alex Volkov: Oh, really? [00:21:39] Junyang Lin: Open open leaderboard. [00:21:40] Alex Volkov: Smog is the next thing we're going to talk about, so you're taking us exactly there. I think, Nisten, you have a question just before, and then we're going to move to talk about smog. Just on the community part just the names you mentioned. You mentioned Stablequan, definitely friend of the pod. [00:21:52] Alex Volkov: You mentioned Technium introduced you to Winglian, the guy from Axolotl. All of this happens in the ThursdAI community, and I love it. I'll just say that I see Robert in the audience here. Smog is from Abacus AI, and I think Robert has some connection to Bindu, so Robert, if you can introduce Junyang to Bindu, that would be great, and then we'll figure out, like, how they use the 72B model. [00:22:12] Alex Volkov: 72B model that you guys released is one of the more performant ones. I think it's even outperforming Mistral Medium, is that correct? [00:22:21] Junyang Lin: Yeah it's now this version QEM 1. 5 SIMD2 BDN is for the chat model for the base model, it is actually quite similar some users have found that I admit that, and, but for the chat models, we have some improvements because this time we are not only Actually, we not only SFD the model, but we also use DBO. [00:22:40] Junyang Lin: We have some progress in DBO. So we've reached like 8. 67 in MTBench. This is a relatively high score and we just did simple DBO and just improved the model. And we also sent our model to Chatbot Arena in Elimsys. supported by Together AI, because we have some friends in Together AI. They just built API for us, and we have been in chatbot arena, so you can try it in chatbot arena to see how it really performs. [00:23:18] Junyang Lin: Is it really perform just like the score of MTBench? I'm not quite sure, because I'm also dependent on the users feedback. [00:23:27] Alex Volkov: it depends on human preference. I so first of all, Justin, you're taking over my job now because you're also reporting on the stuff that I wanted to mention, but definitely a shout out for getting added to LMSYS. That's not super easy. Not every model out there on the Hagenfest leaderboard gets added there. [00:23:41] Alex Volkov: So definitely super cool. Yeah, please go ahead. If you have anything else to [00:23:46] Junyang Lin: for as you have mentioned Mistral Medium, I'm not sure which one is better Mistral Medium or Qwen 72 Billion from some reviews they might be similar for the Qwen 1. 5 72 Billion similar to MiQ some of my friends like Blade just tested In EqBench, the scores are very similar, but I need some more reviews to let me really know that how the 72 billion model really perform, that how is it better or is it worse than MeeQ? [00:24:20] Junyang Lin: They're all okay for me. I just want real reviews for me. Yeah, [00:24:23] Alex Volkov: Yeah, [00:24:24] Junyang Lin: it. [00:24:25] Discussion about Qwen VL with Nisten and Piotr [00:24:25] Alex Volkov: awesome. Junaid, thank you for joining us. And Nisten, go ahead. You have a few questions, I think, about the interesting things about VL. [00:24:34] Nisten Tahiraj: Yeah, so one thing is that the 0.5 Bs and the small models, I know Denova in the audience was specifically looking for one around that size or like a 0.3 to run on web GBU, because then even at 32 bit, which older browsers will still support it, it will still only take two gigs. So that, that would run anywhere. [00:24:58] Nisten Tahiraj: But my question. [00:25:00] So shout out to Feliz de Nova for all that. I know he's going to do something with it but my question for you was more about the Macs and the the larger Qwen QwenVL chats are those also based off of the 72B and did you find more improvements in going with a larger LLM, and I also wanted to know your opinion on Lava. [00:25:27] Nisten Tahiraj: The Lava 1. 6 method where they mosaic together four clip models on top to get a larger image, even though it slows down inference because now it's got a output like 2000 embeddings. So yeah, what do you think of Lava and is there more stuff to share about the Clang, [00:25:47] Junyang Lin: VL, Max. Yeah for Plus and Max it may be, sorry for me not ready to open source it. [00:25:57] Junyang Lin: I cannot decide these things. Yeah actually it's built on larger language models much larger than the Plus, and you can guess whether it is 72 billion. It is not that important, and we have found that The scaling of the language model is really important for the understanding of the VR models. [00:26:18] Junyang Lin: We have tested it on the MMMU benchmark and we have found that the Max model is highly more com competitive and performs much better than the Quin bi plus. Although previously many people have thought that Quin Bi Plus is strong enough, but we found that the max had. Much better reasoning capabilities, just understand some, something like some reasoning games like poker or these things like that, some complex things that people can understand through the vision information they can somehow understand it. [00:26:52] Junyang Lin: I think the performance might be a bit slower. Approaching Gemini, Ultra, or GPE4B for the QEMDR MAX. We were just gathering some reviews. I'm not quite sure, but [00:27:05] Alex Volkov: From the review perspective, I want to say hi to Petr, our friend here on stage, from Roboflow. Petr is one of the vision experts here on stage. Petr, welcome. Feel free to introduce yourself briefly, but I definitely know that you got excited about some of the GwenVL Plus stuff, so definitely feel free to share some of your insights here. [00:27:30] Piotr Skalski: Okay. Yeah. And first of all, awesome to meet somebody from Qwentin. Yeah. [00:27:36] Piotr Skalski: So yeah I'm from Roboflow, like you said and I'm responsible there for computer vision and growth. So it's like in between of being ML engineer and marketing something like this. [00:27:49] Piotr Skalski: And yeah, I was experimenting with Qwen, Plas and Max last week. Super impressed in my opinion. I know that you tried to be humble, maybe, but. In my opinion it's, at least on things that [00:28:04] Junyang Lin: I test, it performs like the best compared [00:28:08] Piotr Skalski: to other [00:28:09] Junyang Lin: models. Thank you very much. Thanks for the appreciation. [00:28:14] Piotr Skalski: Yeah. And especially the fact, so the biggest game changer for me, and I know that there were models that were capable of that before, is the fact that you can ground those predictions and you can, for example, point to a specific element on the image. So it's not only that you can ask questions and get answers and do OCR, but you can straight up do zero shot detection if you would like. [00:28:40] Piotr Skalski: Yeah. Which is which is awesome. And that's something that none of the. Other popular models can do to that extent, at least on the [00:28:51] Piotr Skalski: things [00:28:51] Piotr Skalski: that I [00:28:51] Piotr Skalski: tested. My question is, [00:28:55] Piotr Skalski: do you plan to open source it? Because it's awesome that you can try it out for the API and I highly appreciate the fact that you created the, HF space and you can go there and try it. [00:29:07] Piotr Skalski: But is there a chance that you will open source it even with the meeting? License are not necessary. [00:29:16] Junyang Lin: Yeah personally, I would like to open source some but I cannot decide these things, but I think there's a chance I'm still promoting these things inside the core, but I cannot say too many things about these stuff, but we will try because we have found out that we ourselves can also build very good LMM. [00:29:37] Junyang Lin: I think the gap Just between the big corp between us and the big corp. In LMM, it's very small. And we have found that our techniques or our training is quite effective. So maybe one day we'll share to the community, but for now it is still APIs and demos and I would try to think about these things. [00:29:59] Junyang Lin: And also question about. The comparison with us and Lava, and I have just tried Lava 1. 6 not quite freQwently. I just tried it. I think it's a very good model and it it has very good performance in the benchmark results but I think the limitations of these other open source models may be that It still lacks sufficient pre training for them Skullscape just said we can do Qwen can do OCR and you can find that Qwen's reasoning capability is quite strong because we have done a lot of pre training work on it. [00:30:39] Junyang Lin: We have done a lot of data engineering on pre training because we have capabilities of handling different resolutions and different aspect ratios so that we can use the curated, the OCR data and put them in the pre training. And when the vision length model can understand a lot of textual like linguistic information inside the images, they may do something like like we said, reasoning, and you will find that really powerful, very impressive, or things like that. [00:31:13] Junyang Lin: Yeah I think the gap between other models and us, or also Gemini Ultra and GPT 4b, maybe still the lack of large scale data. for training. Yeah, this is my opinion. [00:31:27] Alex Volkov: we're waiting for more data, but we're also waiting for you guys too. I just want to thank you for being the champion for open source from within the organization, and really appreciate all your releases as well. I think Piotr and Nisten, like everybody here on stage, definitely. It feels that, and thank you for coming and talking about this. [00:31:45] Alex Volkov: Justin, feel free to stick around because the next thing we're gonna talk about, you already mentioned, which is Smog 72 B which is the top of the leaderboard. And I just read through the thread from Bindu, ready from Abacus ai and it looks like they didn't even use 1.5. I think they used 70 the previous Quinn [00:32:02] Junyang Lin: yeah, they used the previous QUANT72B. If they are really based on the base language model there might not be a lot of differences. Because 1. 5 for the base language model 72B is actually slightly better than the original 72B for the base language model. Yeah. [00:32:22] Alex Volkov: for the base ones. And very interesting what they [00:32:24] Junyang Lin: the base one. [00:32:25] Alex Volkov: So they, they don't share any techniques, but they promised to open source their techniques. They're saying like, our next goal will be to publish these techniques as a research paper and apply them to some of the best Mistral models, including Miku. [00:32:37] Alex Volkov: So I got confused. I thought that they already fine tuned Miku, but no, they just fine tuned on top of Qwen. And now the top Hug Face leaderboard model is based, is a fine tune of Qwen, which is like also super cool. [00:32:50] Junyang Lin: Yeah, I'm very proud of it. [00:32:52] Alex Volkov: Yeah, congrats. [00:32:53] Junyang Lin: They are using our model to be the top of the model. I'm also really expecting their technical report to see how they reach the top of the benchmark. But I think it is not that It is not that kind of difficult because you have a lot of ways to improve your performance in the benchmark, so we'll still see how it really performs in the real scenarios, especially for their chat models, yeah. [00:33:18] Alex Volkov: Yeah, that's true, [00:33:20] that's often the case. But I just want to shout out that the world is changing like super fast. We're definitely watching and monitoring the Hagenface leaderboard. And performing better than Mistral Medium is impressive. And this looks at least on the MMLU, this is 77. I think they said they broke The average score of 80, this is the first model that broke the average score of 80 on the open source leaderboard on hang and face, which is super cool based on Quinn as well, and definitely worth it. [00:33:46] Alex Volkov: I'm gonna add this link to the show notes and hopefully we'll find a way to connect you guys with the Bindu team there at Abacus to see how else this can be improved even for, and whether or not these techniques can be put on smaller models as well. I think in the open source, the last thing. [00:34:00] Junyang Lin: expecting the chat. Yeah, I'm really expecting to chat with them. Yeah, continue, [00:34:05] Alex Volkov: So definitely hoping that some of our friends can connect between these awesome teams and learn from each other, which I think is the benefit of speaking in the public and putting things in open source. Now, moving on, the last thing that you definitely mentioned is the update from LMSys, which is quite a few of our friends of the pod are now also part of the chatbot arena. [00:34:24] Alex Volkov: They just announced this yesterday. They've added Three of your versions, right? They added 1.572 B, 1.57 B, 1.5, four B, and they also added open chat. So shout out the folks from Open Chat Alai and the Alignment Lab and some other friends of ours who like release open chats latest release and they also added news imis fine tune. [00:34:47] Alex Volkov: So if you guys remember we've talked about news fine tuning on mixed mixture and that improved on the mixture of expert model from. From Mistral a little bit based on DPO data sets. So now that's also in the LMCS arena and it's now powered by Together Compute. Which I have no affiliation with besides the fact that they're awesome. [00:35:04] Alex Volkov: They're sponsoring a bunch of stuff. And we did a hackathon together together is great. Like you can easily fine tune stuff on their platform, but now they're also sponsoring the arena, at least to some extent, which is great because we get more models and arena keeps going. And if you guys remember, or you probably use it, LMC's arena is this another great way for us to feel what human preference is in models. [00:35:27] Alex Volkov: And for many of these models. That's what's more important than actual performance on evaluations, on leaderboards, et cetera. So definitely great update from LMCs as well. And I think that, I'm gonna ask my folks here on stage, but Nisten, Far El, if this is like anything else in open source that's super interesting this week, I think that's mostly it. [00:35:44] Alex Volkov: We can talk about Gemini. [00:35:48] Nisten Tahiraj: There was a data set, which I think is pretty huge of HackerNoon that they released. And oh, there was one more thing HuggingFace made a GPT store. [00:35:58] Alex Volkov: Oh, [00:35:59] Nisten Tahiraj: they made their own GPT store. Yes. I think that's a big, [00:36:03] Alex Volkov: I want to hear about this, for sure. I haven't used it yet, but I invite the Hug Face folks that are listening to this to come and tell us about this, because I haven't used it yet, so I don't actually have many opinions. But yeah, they released their own open source GPT store, which is super cool, and we're going to add this maybe in the show notes, but I don't have a lot to say about this. [00:36:24] Alex Volkov: And I think, in the spirit of Yeah, go ahead. [00:36:27] Nisten Tahiraj: Oh, sorry. Sorry. I'll quickly say that the HackerNoon data set of tech articles, those are some Because they have a lot of guest developers I remember over the years, they had the best ones. Those articles, that data set, is extremely great for any kind of coding or website or whatever work you're doing. [00:36:50] Nisten Tahiraj: That's because it's step by step instructions on how to build something and all the code for it, it's pretty awesome and it's at the very beginning on the Jumbotron if you guys see it from Daniel VanStream. And yeah, and it's MIT licensed and it's 6. 9 million articles and you can do whatever you want with it. [00:37:07] Nisten Tahiraj: That, shout out to them. [00:37:09] Alex Volkov: We'll add this again to the show notes. And as you said something about articles and code, I remembered another thing that definitely Also worth mentioning Junaid Embeddings, if you guys remember, we had a chat with Bo Wang from Juna deep dive into embeddings a while ago, and Junaid Embeddings released a fine tune for code. [00:37:25] Alex Volkov: So just a quick shout out that embeddings can be fine tuned, embedding models can be fine tuned for specific purposes, and definitely embeddings for co and you guys re if those of us who follow from week to week, we talk about embeddings a lot. We've talked about NumX Embeddings last week, the open source full, including the training datasets. [00:37:42] Alex Volkov: We've talked about. OpenAI changing embeddings and giving us new ones and cheaper ones. And Junaid, we had a deep dive and I definitely welcome you to go and check out that special episode with Bo Wang from Junaid and they trained their own BERT model as the backbone, the LLM backbone that decides about embeddings and they just released an update to their embeddings fine tuned for code retrieval specifically. [00:38:03] Alex Volkov: And I think for many folks are building rack system. That's something that they should be aware of that embedding models can be also fine tuned for specific purposes like Q& A and obviously code as well. So if you haven't tried that yet and you're doing a bunch of material on top of code, for example, using some of the data sets that Nisten just mentioned, that probably there's code in there definitely check this out. [00:38:25] Alex Volkov: I think we're moving on to the big company thing, and I don't have a big company transition, I do have this one though. [00:38:43] Google finally lanuches Gemini Ultra [00:38:43] Alex Volkov: Just in, as we started the space, maybe an hour before, our friends from the big G, Google finally answered the question that we've been asking since 10 months and three weeks ago, where is Google? So GPT 4 was released to us after ChaiGPT released in, I want to say December, maybe December 1st, November 31st of 2020. [00:39:06] Alex Volkov: Then GPT 4 was released in March of 2023. And throughout this time, there was this famous video of Satya Nadella asking where is Google and where's this like 600 pound gorilla in the room of search? And we're going to make them dance. And they definitely make them dance. And we've been waiting. [00:39:25] Alex Volkov: Where's Google? Where's Google? And Google has released. Quite a few stuff for us since then. Just for context, I think everybody knows this already. Google is the place of the birth of the transformer paper. So like most of this, the recent Gen AI explosion is, can be attributed to transformers architecture that came out from Google. [00:39:43] Alex Volkov: Google had trained multiple models, including like Palm, and we've talked about Palm and Palm 2, and I don't even remember all the names of the models that they've released for us throughout the years. Google then also. At some point gave us BARD, which is their interface, the chat interface that people used in order to play with their models, and I think some of this was Bye. [00:40:04] Alex Volkov: Bye. Palm, something else as well. And recently, and I think around December, they said, Hey, you know what? We're here and we have this thing called Gemini after the unification of Google Brain and DeepMind under one org. And we're going to give you Gemini Pro right now, but we'll tell you that Gemini Ultra, that was back in December. [00:40:23] Alex Volkov: The Gemini, I guess December will tell you the Gemini Ultra is coming and it's going to be better than GPT 4 and you're going to get it soon. And we've been like saying when? And today is the day is the answer for those questions. So today we're celebrating, congrats folks at Google who finally released and upgrade to their LLM capabilities. [00:40:41] Alex Volkov: Not only an upgrade, so much an upgrade that they've killed the Bard brand completely. No more Bard. That's what I'm understanding. No more BARD, even though that's very confusing. If you guys remember a few weeks ago, we've talked about LMSYS changes were barred with Gemini, I think, something like confusing like this, shot up to the top of the charts and just was trailing GPT 4. [00:41:05] Alex Volkov: So like second best model in LMSYS arena was barred with GPT 4, or sorry, barred with Gemini. See how confusing this is? And now there's no more barred. But there is an LNCS. Anyway, this is like the whole naming is confusing thing, but Google, including a blog post from Sundar and everything, Google comes out with a new update and says, Hey, Bard is no more. [00:41:25] Alex Volkov: It's now Gemini and the models are also Gemini. So that's confusing. And the models are Gemini Ultra. We finally get access to Google's answer to GPT 4 today, which is incredible. That answer is Ultra 1. 0. [00:41:40] And we can get this. As part of something like a paid premium tier that's called GMA Advanced on Google. [00:41:46] Alex Volkov: So you can actually go right now, you can sign up, it's 20 bucks a month, and it starts 20 bucks or 30 bucks? I think it's 20 [00:41:52] Nisten Tahiraj: It's two months free [00:41:54] Alex Volkov: Yeah, and you get two months, two months trial because they have to prove themselves to you because many people will decide whether or not they're going to go with Google or with JGPT. [00:42:03] Alex Volkov: And we're going to talk about which one folks will prefer. I haven't tried it yet. Literally as I woke up, I had to prepare my notes for the space. I just want to say. Google, welcome to the party, we've been waiting for you, and I counted, it's been exactly 10 months and 3 weeks and 4 days since GPT 4 released that you came with the same level of, at least, based on benchmarks. [00:42:24] Alex Volkov: And now we're gonna talk with some folks who actually tried it, Nisten, you tried it, I think Ray, you also tried it let's talk about your first impressions from BART, oh, or, sorry, Gemini. [00:42:35] Nisten Tahiraj: One, it's heavily moderated. No one's surprised by that. It does answer and reason nicely, or at least the way it communicates, it's a lot more eloQwent, I would say. It feels nicer in the way it reasons stuff out. However, compared to Mistral Medium, or Mixtral, it doesn't quite obey you. I tried my standard question, which is just like Climb out a schedule of building a city on Mars and write the code in C and JavaScript. [00:43:10] Nisten Tahiraj: And that's a pretty complex question for, that only the best models get. And I needed to re prompt it in order for it to give the answer. And even then, it only wrote some JavaScript. But it was really good JavaScript. However, it didn't do the rest of the task. Okay, it's not bad. It is worth using. Again, very heavily moderated. [00:43:33] Nisten Tahiraj: As for the vision side of it, it's extremely heavily moderated. I was even telling it to count out, I had an old gaming PC on the floor with two GPUs on the side, and I told it to make me a JSON of all the parts that it sees in the picture. It won't answer questions like, that have humans in them, or even if they're like Star Wars characters or whatever. [00:43:58] Nisten Tahiraj: But This, I thought, would be something pretty simple, and it, even this one it refused to answer. Yes is good, I think. On the, as far as the vision side goes, the model, the open source models might have it already beat, or will soon. [00:44:19] Ray Fernando: Yeah, I wanted to add, Ankesh from Google DeepMind actually wrote because I've been posting some of this stuff, and he says, To preempt any confusion, multimodal queries don't go through Pro slash Ultra yet, but that is coming soon too. [00:44:33] Ray Fernando: Which makes sense a little bit of why you're seeing some of that stuff. I've been seeing similar things when I've been doing some image analysis or even trying to generate images that have people. One of my examples I've just been posting on my my Twitter feed is like having to analyze a meme. [00:44:48] Ray Fernando: So it's the hot girls meme or the hot ones meme and I was like, hey, this is very popular. Can you tell me what this meme is? And it's I'm sorry I can't because there's images of people. And then I had to do some other meme analysis with Elon Musk and it's the same type of queries. But to add to what Nisten was saying, I've been doing a lot of creative writing tasks, and the writing output has been actually really nice. [00:45:10] Ray Fernando: And it doesn't have all that extra fluff that you normally would get from ChatGPT 4. And what I find with OpenAI's ChatGPT 4 is that they freQwently say, Hey, don't use purple prose, which is all that extra fluffy stuff you read that make people sound smart. It's I just want a regular sounding piece. [00:45:27] Ray Fernando: And usually ChatGPT would do that and then revert back to its normal state but I find that Gemini Advanced just keeps going through it and, continues with the writing pieces of things. And for coding stuff, it's really strange. You actually cannot upload any CSV or any text files. [00:45:43] Ray Fernando: They only let you upload images right now. So you can only have a picture of a microphone and a picture of the little icon to upload an image. Because I wanted to just do a simple analysis on my tweets with a CSV file. And it's there's no place that I see to actually upload that. And I could probably upload so many lines, but there's also a character cutoff, too, that doesn't allow me to upload a lot of code for, [00:46:03] Ray Fernando: A code base. [00:46:04] Alex Volkov: What's the, I was about to say this next thing. Do we know the context length? Anybody have an idea of where Gemini Ultra is at around? 'cause we know that GT four is 1 28 K and I think they recently opened this up on the UI as well. I've been noticing less restrictions. I've been able to pace like a lot more code. [00:46:21] Alex Volkov: My, my test is, you guys know my test is the transcription of the Thursday I conversation that I past and Claude with the a hundred K context definitely takes all of it. GBT. For the pro kind of level used to refuse and now recently it's okay. Yeah, let me summarize this for you Have you guys been able to sense the context length of Gemini Ultra? [00:46:41] Alex Volkov: Is it any close? Actually, go ahead Welcome to the stage, buddy [00:46:46] Akshay Gautam: Hello, I just wanted to bring up that their official document mentions that it's 2k context length. [00:46:53] Alex Volkov: Actually, we don't get greetings of the day [00:46:57] Akshay Gautam: I see. Yeah. Yeah. Greetings of the day everybody. My name is Akshay Kumar Gautam and I'm an applied AI engineer. I was a data scientist before, but now I work with, modeling and stuff. And yeah I was literally waiting for, I tried, came out, I paid for it because why not? And and a lot of stuff. [00:47:14] Akshay Gautam: First of all, it's really good at coding. By the way, the context length is 32K at least that's what they say. Yeah, 32K. And and the model is not good at keeping context, like that is what I was here to talk about. It will lose sense for example, if you ask it to do multiple things in a single prompt, it will not. [00:47:33] Akshay Gautam: Unlike chatGPT, but like with coding, it's better than chatGPT in my humble opinion. [00:47:41] Alex Volkov: so I want to talk about some advantages that Google has, the big dog definitely, because an additional thing that they released, which Chantipiti doesn't have, is ChairGPT has this, but they released an iOS and Android app, but Android also has integration with the Google Assistant, right? [00:47:56] Alex Volkov: So you can now join this advanced or ultra tier and use this from your Android device. Now, I'm not an Android user, but I definitely understand that the ecosystem is vast and many people just use this assistant and we're waiting for Apple. We don't have anything to say about Apple specifically today, besides the fact that, they released the, maybe the next era of computing. [00:48:16] Alex Volkov: But. There's nothing AI series, still the same series from like 2019 with some examples, but Google has now moved everybody who wants to, who pays the 20 bucks a month and has an Android device basically towards this level of intelligence, basically a GPT 4 level of intelligence. And I saw that Marques Brownlee, MKBHD on YouTube, like one of the best tech reviewers out there. [00:48:38] Alex Volkov: He has been playing with the Android stuff, and he said that even the integration Google Assistant even uses your home stuff. So you can actually ask this level of intelligence to turn on some lights, whatever, and probably better context. Actually, you have any comments on this? Have you played with the Assistant version? [00:48:54] Akshay Gautam: Two things first of all, Bing chat was already available on Android devices, right? The Copilot, now it's called. Copilot uses GPT 4, so it's already really good. And you can actually use a lot of voice stuff with Copilot as well, which was surprising. The Google Assistant to be honest, in terms of assistants among Siri and I have a Samsung device, so it has Bixby and, among all the AI systems, Google Assistant was the best one by far, in terms of how much you can, use it, and hoping to get access because I have paid for the Ultra, but I still don't have, access to everything. [00:49:29] Akshay Gautam: Also, there's no API for Ultra, so you cannot actually test anything as well. [00:49:34] Alex Volkov: we haven't gotten an API developers Sundar Pichai said the developers announcements are going to come next week. IOS hasn't updated yet. Yeah, go ahead Nisten. [00:49:44] Nisten Tahiraj: I just really quickly tested it with the entire Lama. cpp file. I am down to 15, 000 tokens I cut it down to and it's still too long. We know it's under 16, 000 that you can paste in. I will know [00:50:00] exactly in a few minutes, [00:50:03] Alex Volkov: So not super, super impressive in terms of like long context. I will also [00:50:06] Nisten Tahiraj: at least not for the UI, [00:50:08] Alex Volkov: for the UI. Usually, yeah, usually for some reason they restrict the UI or they forget to update this. And then the model itself is like way longer context, but for now not extremely impressive comparatively. [00:50:18] Alex Volkov: And again, we're comparing the two like main flagship models OpenAI GPT 4 and now Google's Gemini Ultra. And I also want to say one thing, Gemini seems to be optimized only for English as well, even though it will answer like most of the questions other languages, but it looks like the optimization was focused on English as well. [00:50:36] Alex Volkov: including some of the apps as well, which is, understandable, but we have to, as we're trying to compare apples to apples GPT 4 is incredibly versatile in multi language operations as well. LDJ, you have some comments? Welcome, buddy, to the stage and give us some Have you played with Ultra so far? [00:50:55] LDJ: Yes I was actually wondering, does anybody know of plans for them to integrate this with Google Home? Because I just asked my Google Home right now are you Gemini? And it said, I'm a Virgo. And then I asked it, what AI model are you running right now? It said, sorry, I don't understand. So I don't think it's, at least mine, I don't think is running Gemini right now. [00:51:16] LDJ: But [00:51:17] Alex Volkov: No, so I think the announcement was [00:51:18] Junyang Lin: to put it. [00:51:19] Alex Volkov: The integration into Google Home will come from the Google Assistant. So if you have an Android device, you'll have Google Assistant there. That you can switch on like a smarter brain, and that you can ask it to integrate like with your home through the device. So you can ask it to do stuff in your home. [00:51:34] Alex Volkov: But the Google Home itself, like the Google Home devices that you have, they're not talked about upgrading them, but maybe at some point, because why not? But I haven't seen anything on this yet. Anything else here? [00:51:46] Junyang Lin: I think that'd be the perfect. Sorry. Yeah, go on. [00:51:48] Alex Volkov: Yeah, no, that would be great. I agree with you. Being able to walk around your house and just talk with GPT 4 level intelligence to do operations, I definitely agree. [00:51:55] Alex Volkov: That would be great. I gotta wonder anything else here on Ultra? We've talked about its code performance. We've talked about its inability to talk about people. Anything else interesting that we want to cover so far? And again, folks, it's been two hours and we're already giving you like a bunch of info, but we'll play with this going forward. [00:52:12] Nisten Tahiraj: It's about 8, 000 the context length that you [00:52:14] Alex Volkov: Are you serious? Wow, that's not a lot at [00:52:17] Nisten Tahiraj: that's as much I was able to paste it like 7, 500. [00:52:20] Alex Volkov: So yeah, folks, you heard it here first. You'll get more context than you previously got probably, but it's not a lot comparatively. Even though it can probably, it's probably a consideration of compute for Google, right? How much context to give you the model probably gets more. And it's also a vision enabled model. [00:52:36] Alex Volkov: But I think that we've covered this enough. Gemini Ultra. It's here, it's very impressive from Google, and yet, I want to say personally, maybe a little bit underwhelming because, they need to convince us to move, and it's going to be the same price, and I don't know, let me just ask this before we move on. [00:52:55] Alex Volkov: Anybody here on stage who has access to both plans to pay for this and not GPT? [00:53:03] Nisten Tahiraj: I haven't paid for anything since September But I'm [00:53:08] Junyang Lin: not the right person for this question. My company pays for like my character description. So I might keep both [00:53:15] Alex Volkov: Interesting. [00:53:16] Junyang Lin: paying for mine's out of pocket. I'm just going to keep both. I like the OpenAI app because it's just the multimodal picture on my phone. [00:53:23] Junyang Lin: I'm on the go. For Google, I'm just curious because it's two months free. That just means that, they have me hooked. We'll see. [00:53:30] Alex Volkov: Yeah, it's two months free. And then let's check in back in two months, and see how many of us kept paying. All right. I so Google also releases. a Llama CPP wrapper called Local LLM. I don't know if you guys saw this. It's pretty cool. It's an open source tool from Google that actually helps you run LLMs locally on CPUs and then also on the Google Cloud with a super easy integration. [00:53:51] Alex Volkov: Very interesting choice. They also call out the bloke that you can download models from the bloke with their tool. And I think it's very funny that if you go on. The description of the blog of local LLM, they call this. Now, the tool, they told you in the code snippets, they say, Hey, install OpenAI. [00:54:10] Alex Volkov: So I found it really funny. But yeah, they have a wrapper there that integrates with Google Cloud as well. [00:54:15] OpenAI adds DALL-E watermarking and per API key restrictions [00:54:15] Alex Volkov: Running through the big companies areas like super quick. OpenAI added watermarks to Dali images. They use this new metadata thing called C two P embeds and it embeds in the metadata. [00:54:27] Alex Volkov: And so basically what this means for us is not that much, but when you download images from Dali generated, I assume that the same will come to Microsoft copilot. They will now have in the metadata, where like the location is and everything else. They will now have the fact that they have been generated with. [00:54:43] Alex Volkov: They have been generated with DALI this information will sit in the metadata. Now it's only images, not text or voice or anything else from OpenAI. This happens over the API or from the ChatGPT interface as well. This increases the file size a little bit because of some of the stuff, but it's not super interesting. [00:55:00] Alex Volkov: This can be stripped. So it doesn't mean that if the lack of presence of this thing does not mean that it's not generated with DALI. It just, if there is, it's definitely generated with DALI. And so this is an interesting attempt from OpenAI to say, Hey, we're doing as much as we can. [00:55:15] Alex Volkov: It's not foolproof, but an interesting attempt. And also, I just want to mention that if, for those of us who develop with OpenAI, The API keys, they keep upgrading the developer experience there and the API keys part. And now you can restrict per API key. You can restrict its usage, which many people have been waiting for a long time. [00:55:33] Alex Volkov: And that's really like many people has been wanting this. You can create one API key for OpenAI for a specific purpose and restrict it to only DALI, for example. And you can, I don't know if you can restrict. based on credits, I don't think so, but you can definitely restrict in, in the usage related stuff. [00:55:49] Alex Volkov: That's, I think, all the updates from the big companies and the LLMs and APIs, [00:55:53] Alex Volkov: This week's buzz is the corner and I stopped the music too prematurely. This week's buzz is the corner where I talk about the stuff that I learned in Weights & Biases this week. And I don't know how many of you were, had a chance to join our live segments, but we definitely had a build week. And I think I mentioned this before, but actually we had a live show on Monday. [00:56:19] Alex Volkov: We're going to have another one this probably tomorrow. Yeah, tomorrow. I think it's Noon Pacific, where I interview my team, the GrowthML team in Weights & Biases, about the build with projects that we've built, uh, last December to try and see what's the latest and greatest in this world. So as we build tools for you in this world, we also wanna Build internal tools to see what are the latest techniques and stuff like we just talked about. [00:56:46] Alex Volkov: For example, it gives us a chance to play around with them. It's like an internal hackathon. And what happened was is we build those tools and we present them to the company and then this was basically it. And I said, Hey, hold on a second. I learned the best publicly. I learned the best about, the way I just learned from Connor and Benjamin. [00:57:02] Alex Volkov: I learned from Nisten and Far El and all the folks in the audience. And Luigi and I had a whole section where he taught me weights and biases before. I learned the best by being public and talking about what I'm learning as I'm learning this. And so I did the same thing with our folks from the GrowthML team. [00:57:15] Alex Volkov: We just literally folks came up on stage and I asked them about what they built and what they learned. And we're going to summarize those learnings in the live show. And that live show, if you're interested, is all over our social, so on Weights & Biases YouTube and LinkedIn. Yes, LinkedIn, I now need to also participate in that whole thing. [00:57:33] Alex Volkov: So if you have tips about LinkedIn, let me know. But it's live on LinkedIn, live on YouTube. I think we did X as well and nobody came. We're probably try to send you to the live YouTube flow. But basically the second part of this is coming up tomorrow. We're interviewing three more folks and you get to meet the team that I'm, the incredible team that that I'm part of. [00:57:53] Alex Volkov: Very smart folks. like Kaggle Masters, and some of them came to Kano's show as well, which is super cool. And I find the first conversation super interesting and insightful for me. Definitely recommend if you're into Understanding how to build projects that actually work within companies was the process. [00:58:11] Alex Volkov: We have folks who build something from scratch, we have somebody who runs a actual bot with retrieval and re ranking and evaluations and like all these things and [00:58:20] have been running them for a year basically on the production. So you can actually try our bot in Discord right now and in Slack and on GPTs. [00:58:28] Alex Volkov: If you want to hear about the difference between a mature, rag based But that's in production for a professional AI company, but also the difference between that and something that somebody can like quickly build in a week. We've talked about those differences as well. So definitely worth checking out that live. [00:58:46] Alex Volkov: Moving on from this week's buzz, and I learned a lot. Okay, so back from the this week's buzz, we're moving into vision. [00:58:52] [00:58:57] Alex Volkov: And Bria AI like super quick, they released a new Background Segmentation Model, or Background Removal Model, that's live on Hug Face, is called RMBG V1. 4, and I think the cool thing about this is that it now runs completely in the browser, thanks to the efforts of our friend Zinova, who is no longer in the audience, I think, from Hug Face and Transformers. [00:59:19] Alex Volkov: js, and it's super cool. You can like, remove backgrounds completely without sending any images to anywhere, and just straight from your browser. That model is called, again, RMBG, and it's not Commercially viable. So you cannot use this for professional stuff, but it's open for you to try and play with in the voice category, the voice and audio category. [00:59:39] Alex Volkov: We don't have a lot of audio stuff lately, so I think the main audio stuff that we've talked about was. I want to say Suno is like the latest and greatest, but we're still waiting for some cool music creation stuff from different labs. And definitely I know some of them are coming but in the voice category and you know that we've been talking about, my position in this and Nisten and I share this position. [01:00:01] Alex Volkov: I think personally, The faster models will come out that can clone your voice and the faster they're going to come out in open source, the better it is generally for society. I know it's a hot take, I know, but I know also, I cannot reveal the source, I know that voice cloning tech is going to be at open source like super, super quick. [01:00:21] Alex Volkov: And I think it's like one of those. Break the dam type things that the first kind of major lab will release a voice cloning and then everybody will see that nothing happened in the world, everybody else will release theirs, and we know everybody has one. We know for a long time that Microsoft has, I want to say Valley, was that Valley? [01:00:38] Alex Volkov: That clones your voice in under three seconds. There's papers on this from every company in the world. We know that OpenAI has one. They collaborated with Spotify and they cloned Lex Fridman's voice and it sounds exactly like Lex Fridman. We know that companies like Heygen, for example, I think they use 11labs. [01:00:54] Alex Volkov: 11labs has voice cloning as well. None of this is open source, everything is proprietary. So we're still waiting for the voice cloning area from open source from a big company. But for now, we got something called MetaVoice from a smaller company. Not from Meta, it's just called MetaVoice, it's confusing. [01:01:08] Alex Volkov: It's just like a tiny model, 1. 2 billion parameters model. It's trained on 100k hours of data, which is quite significant, but not millions of hours. And it supports zero shot voice cloning. So basically under a few samples, like a basic sample of your voice, and then you're going to get a clone of your voice or somebody else's, which is what scares many people in this area. [01:01:30] Alex Volkov: It has like long form synthesis as well. It's super cool. And it has emotional speech. If you guys remember, we've talked about. How important emotion is in voice cloning, because again, for those of you who follow ThursdAI for a while, you may remember myself voice cloned in kind of Russian, and I'm doing this with a lot of excitement, when the regular voice cloning thing for Alex speaks in a monotone voice, that's Very clearly not the same kind of person. [01:01:56] Alex Volkov: So emotional speech is very important. And some of this is with prompt engineering and some of this happens in voice casting or voice acting. And the best part about this MetaVoice thing is Apache 2 license and it sounds pretty good. And so we've talked about multiple TTS models, and now this model is definitely out there. [01:02:14] Alex Volkov: So if you're building anything and you want a TTS model for you with voice cloning, I think this is now the best. the best shot you have. It's called MetaVoice. I'm going to be adding this to the show notes as well. And I think we have a breaking news from a friend, VB with another model called Nemo. [01:02:30] Alex Volkov: So let's take a look. Yeah, definitely a new model from NVIDIA. It's called Nemo. Let me actually use this. I want to use the sound as much as possible. [01:02:50] Alex Volkov: So I'm gonna go and try and find this tweet for you, but basically we have a breaking news, literally Rich VB, which is the guy friend of the Padawars, who's in charge of, like, all the cool voice related and TTS related tech and Hug Face, he mentioned that NVIDIA AI released Nemo Canary. [01:03:07] Alex Volkov: Nemo Canary is the top of open a SR leaderboard. VB is also part of the folks who are running the leaderboard for us, a SR stands for automatic speech Recognition. No, I think I'm confusing this. Yes, automatic speech recognition. Cool. Thank you, Nisten. So basically, if you guys remember Whisper, we talked about Whisper a lot. [01:03:25] Alex Volkov: This is the leaderboard, and Whisper has been on top of this leaderboard for a while. Recently, NVIDIA has done some stuff with stuff like Parakit. And now we have a new contender in the ASR leaderboard called Nemo Canary 1B. 1B is not that much. Whisper The highest Whisper large, I think it's 2. 5 B or something. [01:03:44] Alex Volkov: This is now the top SR leaderboard. It beats Whisper and it beats Seamless from Meta as well. And I don't know about License here. It supports four languages. Whisper obviously supports a hundred, which is, uh, which is, we know the best for many kind of low resource languages as well. Trained on not that much hours of annotated audio, only 85 1000 hours or so, and it's super fast as well. [01:04:10] Alex Volkov: It's very interesting that NVIDIA does multiple things in this area. We had Parakit, now we have Canary as well. What else should we look at? I think Bits, Whisper, and a considerable margin, again, on these specific languages. Folks, we've been, I think, we've been on this trend for a while, and I think it's clear. [01:04:28] Alex Volkov: Incredible automatic speech recognition comes on device very soon. Like this trend is very obvious and clear. I will add my kind of thoughts on this from somebody who used Whisper in production for a while. The faster it comes on device, the better. And specifically, I think this will help me talk about the next topic. [01:04:47] Alex Volkov: Let's see what else I have to cover. Yeah, I think it's pretty much it. The next topic [01:04:51] Nisten Tahiraj: I'm trying it right now, by the way. And it's pretty good. [01:04:55] Alex Volkov: Are you transcribing me in real time or what are you doing? [01:04:58] Nisten Tahiraj: yeah, I was transcribing your voice through the phone to my laptop but weirdly enough it doesn't output numbers, it only outputs words however [01:05:06] Nisten Tahiraj: It seems pretty good, huh? I don't know, it seems good to [01:05:09] Nisten Tahiraj: me, LGTM looks good to me. [01:05:11] Alex Volkov: Yeah, it was good to me. Absolutely. The word error rate, the word error rate for Whisper is around 8%, I think, on, on average for these languages and for Canary is less than it's 5. I think, if I remember correctly, VB told us that word error rate is like how many mistakes per 100 words it does, and this does, Five Mistakes Versus Eight, I think on the general data sets. [01:05:36] Alex Volkov: Quite incredible. This is coming and I think I'll use this to jump to the next thing [01:05:39] Alex finds a way to plug Vision Pro in spaces about AI [01:05:39] Alex Volkov: . The next thing, and briefly we'll cover this, is that I haven't used it for the show, but for the past, since last Friday, basically, I've been existing in reality and in augmented virtual spatial reality from Apple. [01:05:52] Alex Volkov: And the reason I finally have a chance to connect these two things is because. I use a lot of the hand gestures within the Vision Pro from Apple, which was released on Friday and a lot of voice as well. And obviously we've talked about Siri, we've talked about finally Google stepping up with their assistant. [01:06:08] Alex Volkov: Siri voice recognition and also typing is not that great. And I know because I used Whisper in production for a bunch. I also use Super Whisper, shout out Neil on my Mac to actually dictate a bunch. And all those tools, all the new tools, Whisper and now Canary and like all these things, they understand me and my accent very well. [01:06:26] Alex Volkov: Whereas Siri is like on device. So Siri actually has two automatic speech recognition. They have the fast one on device and they actually do send your voice on onto the cloud and they return something. So you would [01:06:40] actually see a wrong transcription and then the right one replaced the wrong one. And the right one is actually generally okay, even though with my accent doesn't get me as much, but the wrong one is very bad. [01:06:50] Alex Volkov: It's it's like they stopped. Thinking about ASR, Automatic Spatial Recognition in Apple, back in 2019, and that's what they shipped. However, there were quite a few papers from Apple on this topic, and I know for a fact that we're getting on device. And the reason I'm excited about this in the spatial context as well is because you can talk instead of using Hands on keyboard and that's very cool I think that's all I had to connect with the spatial computing in addition to I've tried all the AI tools and games and everything And we're still not there. [01:07:19] Alex Volkov: There has been one thing that I want to connect if you guys know from the diffusion model area There is a way to generate images in 360 around you and I thought this was super cool because this is essentially a holodeck moment where you can stand in full virtual embedded reality and just say, Hey, I want this thing to appear. [01:07:39] Alex Volkov: And we have now models of text to 3d that are coming like super soon. We obviously have virtual friends that embedding them in real space needs a robot. But now if you have this like spatial computing thing, you can actually put an AI friend in the corner. You will always talk to you. So there's a few like attempts at this in the Apple thing. [01:07:57] Alex Volkov: but not a lot. And also I will ping back to this like last thing where Apple is coming. We've talked about this. Apple is coming on Friday of release of Vision Pro, which was the day after last Thursday. Apple had their uh, shareholder meeting. And in there, Tim Cook said, Hey, we launched spatial computing. [01:08:15] Alex Volkov: We're really happy. This is the next iteration of spatial stuff, blah, blah, blah. I definitely agree about all this. If you watch my feed for the past week, that's pretty much all I can talk about besides AI. However, going back to the AI, Tim Cook finally mentioned the word AI in the call, and he's not the only one. [01:08:30] Alex Volkov: It's very clear where the thing is going. Every earnings call for every major company mentioned AI. Tim Cook specifically mentioned AI finally and said, Hey. We're very excited about this technology and we're going to show you something like soon. So I expect that this WWDC is going to be Spatial and AI related and I definitely think that Apple are thinking about both just because the way Siri looks in Spatial is just incredibly like nice. [01:08:55] Alex Volkov: And I can see how embodying AI in your physical world, where you have spatial awareness, you can put something in the corner, it will sound like it's coming in the corner. And I'm waiting for the, for the point where that has a bot, like a Tesla Optimus bot with AI. [01:09:11] Alex Volkov: But before that, we'll definitely get there with spatial computing. So I'm going to have embodied AI agents around me and I'm going to ask questions. For some reason, the ChatGPT interface within the headset is horrible. And specifically because we all know that the iPhone app you can talk to, but Vision Pro only has access to iPad apps, and you can install the ChatGPT iPad app, but you cannot talk to it, which is a miss, I think, on OpenAI's part. [01:09:35] Alex Volkov: This isn't in my segment about the Vision Pro. I tried as much as possible to connect these things to AI to bring this to you. But, separately from this my full review of Vision Pro is, holy s**t, this device is the new category of computing, and I can talk about this in a different space if you're interested. [01:09:50] Space reset [01:09:50] Alex Volkov: and I think it's time for a reset the space, as we've gone up for an hour here, folks. A little bit more than an hour. I'm just gonna play some music, reset the space, and then we're gonna have a conversation with some folks here on stage. [01:10:12] Deep dive into DSPy, COLbert and RAGatouille with Ben Clavie and Connor Shorten [01:10:12] Alex Volkov: Welcome, everyone, to the second hour of ThursdAI. Where we usually, we have a bunch of stuff to cover still from the news angle, like the Bria stuff and the MetaVoice stuff and the Arts in the Fusion. But, and also maybe you want to have some time to talk about Vision Pro, but for now, we have two guests here on stage that I want to welcome and introduce. [01:10:31] Alex Volkov: And we're going to talk about very interesting things that maybe some of you who follow the Twitter, XAI, Ecosphere have been seeing around and I really want to say I want to say thank you and welcome to Conor and Benjamin for joining us. Maybe let's unmute Conor first and then Benjamin and just introduce yourself. [01:10:49] Alex Volkov: Benjamin, I know you're going through some stuff, buddy. And as much as you can Benjamin feel free to, to talk to us, but we'll try to cover as much as possible. Conor, go ahead and then Benjamin. [01:10:58] Nisten Tahiraj: Hey Alex, are you able to hear me first [01:11:00] Alex Volkov: Yes, we can hear you loud and clear. [01:11:03] Connor Shorten: Awesome, cool. I think I've been like refreshing the Twitter page and all that, but awesome. So I'm Connor. I'm a research scientist at Weavier. I also host the Weavier podcast. And yeah, I've just been so excited about DSPI and I'm, really excited to be diving [01:11:15] Connor Shorten: into it further. [01:11:16] Alex Volkov: That's awesome. And I think that WayVid podcast was the first podcast that I came on as a little bit of a guest from NeurIPS. So we had a great conversation outside of NeurIPS sign. If you guys want to check this out, but also WayVid podcast, the folks from Weights & Biases had a great chat with you. [01:11:29] Alex Volkov: That's where I know you from. Actually researched my position and my team based on the conversation you had with them. Very knowledgeable. And thank you for that content. It's really great. And folks definitely should check it out. And I want to also say hi to Benjamin Clavy. Welcome, Benjamin. [01:11:44] Benjamin Clavie: Hey, [01:11:45] Benjamin Clavie: thank you for having me. Can you hear me? [01:11:47] Alex Volkov: Yes, you're coming through loud and clear. [01:11:50] Benjamin Clavie: Yeah. Thank you. Yeah, I've made Tato, which you might have seen if you're interested in T at all, which is [01:12:02] Benjamin Clavie: physically here, but not present in, but [01:12:05] Alex Volkov: Do, what's in terms of background? Could you give us a little bit of background? Like how you came up to build these things? What's your background? Is this AI? Give us maybe a few brief sentences there. [01:12:15] Benjamin Clavie: I'll say. My background [01:12:16] Benjamin Clavie: here is basically ai. I've done the stereotypical thing of dropping out of uni and immediately gone walking into NLP and I've been doing retrieval on NLP for 6 7 years now. [01:12:25] Benjamin Clavie: Very standard background. [01:12:27] Alex Volkov: So definitely related background. Okay. So we're here to talk about multiple multiple things, interesting things. And Conor, I think maybe let's just start with. I think the guy behind some of this work Omar Khattab is not with us, right? But definitely some of the work that we're going to talk about is attributed to him. [01:12:45] Alex Volkov: So maybe, can you, Conor, can you start us with an introduction to maybe DSPy and then Colbert, and then we're going to talk about Colbert and Ragatouille, and then just a brief one, then we're going to dive into what this means for retrieval stuff, definitely as it relates to you guys in Wave V8 rags are everywhere and like better rack systems and better. [01:13:03] Alex Volkov: Options to prompt these LLMs to better retrieve is, everybody's looking for those. So let's start maybe there. [01:13:12] Connor Shorten: Okay, so I'll try to keep the story going from intro to DSPy and then taking it into retrieval. So I think the first thing about DSPy that will like capture your interest is the programming model. It has this way of Writing initial prompts in a really succinct way, and then you can chain together or compose these graphs of several large language model calls with tool use in the middle, and we can come into retrieve a little bit there as well, but you start off with a really coarse description of what you want it to do, re rank these documents, and then it will optimize the, the whole description of the task as well as giving you a few shot examples to put in the prompt. [01:13:50] Connor Shorten: So that's the first thing that is just super interesting I'm sure everyone listening has done this like manual tweaking of the prompt to try to, get it to do your task and how irritating that can be. And so that's probably the quickest value add is it automatically will come up with the prompts. [01:14:03] Connor Shorten: And then when you want to switch your language model you've been over there saying please output JSON, four exclamation marks performing better than one. And now you switch from GPT 4 to Gemini Ultra, or say, you want to see if Quinn can be view shot prompted to do this. [01:14:17] Connor Shorten: You can now recompile the prompt by using DSPy, and you can switch your language model without having to then redo the prompt tuning. [01:14:24] Alex Volkov: So I have to pause right here, Connor, because I'm coming to this as clean as possible with not a lot of understanding of these things . You said recompile the prompt. [01:14:33] Alex Volkov: I'm definitely one of the folks who've tweaked prompts, tried again, saw, okay, it works for a GPT 4. I'm definitely one of those folks. What do you mean compile the prompt, recompile the prompt? Let's talk about the compilation part of this. [01:14:44] Connor Shorten: I even, when I met Omar, I said, compile it. It's overloaded. I think this kind of analogy started with calling LLMs the new operating system for LLMs and So I think that's the line of thinking to be calling it a compiler. Really we mean automated prompt [01:15:00] tuning. [01:15:00] Connor Shorten: But the reason compiling, I think is the right way to think about it, is, let's say you have eight large language model programs eight parts of it that's what I think is the really exciting that's what I think makes LangChain so popular is people see this gallery of examples of chains where you first analyze some chunks of blog posts, extract the topics, then, You later on aggregate the topics into a description of the topic and then you maybe pass it to an editor prompt, and then you maybe have a council of reviewers, like there's this chain, and so with each component of the chain, or I think graph is now the more common abstraction. [01:15:35] Connor Shorten: You have a prompt there. So let's say you have eight language, or however many, I imagine that as this, continues to evolve, we're going to see like super deep LLM the programs that will have so many LLMs in the middle of it. And so you have a prompt for each of those components. [01:15:49] Connor Shorten: And so that's why compiling, I think the analogy is great because you're compiling the prompts for all of these prompts and yeah, so that's why I'll defend the compiling. [01:16:01] Alex Volkov: So I'll just say like from a perspective of a tinkerer. That's something that maybe triggers me a little bit to say, Oh, I need to compile stuff. No, I just write Python code, but you're saying developers do not fret. Compiling is not that like crazy. It's specifically very helpful and useful for larger applications and very, is very helpful for when you want to replace the brain behind the stuff that you're doing or you want to do this in a structured way. [01:16:24] Alex Volkov: Is that me understanding correctly of what we're talking about? [01:16:28] Connor Shorten: Yeah, I agree completely with that. [01:16:29] Alex Volkov: Awesome. So that's DSPy and Omer Hatab Latent Interactions, or Latest Interactions I think the nickname is. We're definitely going to add him to show notes as well. He's the author of this. DSPy has been around for a while. I definitely know that he has been posting about this quite, quite a lot, but recently has been on the pickup as well. [01:16:46] Alex Volkov: And maybe Colbert is one of the reasons. Let's maybe, can you introduce Colbert as well, Conor? Or do we have some stuff about DSPi still to cover in the introduction phase? [01:16:56] Connor Shorten: Okay, I can transition to Colbert. [01:16:58] Alex Volkov: Colbert? Colbert? How do we, how do you even pronounce this thing? [01:17:02] Connor Shorten: I was surprised when Omar pronounced it Colbert because it, it's Bert and then there's Stephen Colbert. I'd heard him on the podcast with I think Christopher Manning from Stanford who had, asked him about that. [01:17:14] Alex Volkov: So if Omar, the creator of this pronounced Colbert, unfortunately, even though it's BERT models, I think Colbert is what we're talking about. But yeah, from Stephen Colbert. What is Colbert? Why is there excitement on my feed around this? And let's give us an introduction, Carmen. [01:17:31] Connor Shorten: So the, probably the right way to start thinking about it is in search, you typically have retrieval and then re ranking and retrieval is where you have like encodings of the documents. Like you put each of the documents into an embedding model and you get a vector embedding, and then you're doing just, dot product distances between the query vector and these document vectors. [01:17:51] Connor Shorten: So there's no interaction between the query and the documents. The representations are encoded completely separately in retrieval. And then you'll typically pass that into a re ranker. And so there are three kinds of re rankers. There's point wise re rankers that take as input the query in the document and then output a relevance score, doing the interaction between just this query and this, the query in this one document. [01:18:12] Connor Shorten: Then there's pair wise where you take two documents in the query and have a tournament of two at a time. And then there's the list wise re rankers where you're taking all the documents as input at once. So the re rankers are pretty effective, you have this massive latency overhead by doing it like that. [01:18:28] Connor Shorten: So what Colbert introduces is this late interaction. So the benefit of having this interaction between the query and the document most similar to the point-wise cross and coer reran, where you keep the vectors for the the documents and you have this kind of interaction between the inner token vectors. [01:18:47] Connor Shorten: So let me, it's right now what we're doing mostly with vector search is, and this is why the BERT thing is actually really important, is because we're using these encoder only models that output that like a vector for each of the token. But then we pool all those vectors to represent the object with one vector. [01:19:02] Connor Shorten: But Colbert, you keep all the vectors for the query and the document. And then you have this inner, it's maybe a little hard to just talk you through the math behind this, but you have this. The maximum similarity of each of those query vectors with all the document vectors. So say you have 100, document vectors and you're at index 0 of the query vector as you do the maximum similarity with those 100. [01:19:22] Connor Shorten: Then you're at the first vector of the query, second, third, so on. And then you'll average that out. So you now have this late interaction of the vectors between the query and the document. I hope that maybe Benjamin can take the mic from here. I hope that gets the gist of it. [01:19:37] Benjamin Clavie: Yeah, that was pretty good. So just to clarify, like max similarity is like when you're using normal vectors or like batch representation, you do have a single vector for the whole document. [01:19:48] Benjamin Clavie: When you're using Colbert, like Connor said, you've got one vector per token, and at retrieval time, what you do is you compare every single one of your query tokens, so generally not a lot, like maybe 32, and you compare that with every single token in every single document, and you make, you only keep the highest similarity, and then you sum that up, and so you compare every token to every token, you get this really fine grained comparison, instead of trying to slot everything into one massive vector, which would probably lose information. [01:20:17] Benjamin Clavie: Because you're doing it at the token level, it's very clear, I call this like a bag of embeddings because it's like quite close to what we do with TF IDF but with embeddings instead of like just a word count. [01:20:29] Alex Volkov: Wow. Okay. So let me try. So Connor said a bunch of stuff. Then Lindgren, you simplified. Let me try to simplify from my understanding. Okay. Regular rack system, regular basic, not without even the re ranking step. Connor? Like the basic stuff that people do in the wavy examples, for example or whatever local embeddings you have, let's say a vector store of a bunch of information. [01:20:49] Alex Volkov: You have a user asking a question, you want to augment LLM's information. tree because of the knowledge cutoff. And then you embed the user's query in some sort of embedding. We've talked about embeddings multiple times here on ThursdAI. You get some number back and like Benjamin said, you get one embedding for the whole document or the whole query. [01:21:08] Alex Volkov: You get like just one, not per token. You get one embedding and then you use that. And to compare, and the usual similarity score is the ways to compare this. Then if we, you wanna go to advanced stuff, then you maybe do some re ranking. Re ranking is basically showing you like another LLM step, basically, right Conor? [01:21:28] Alex Volkov: Or some maybe model that does re ranking for you, that chooses, you retrieve multiple examples, and you choose which one like fits better. And you can do this based on several things. The downside of this is, the bigger documents you embed, the kind of, um, The last concepts maybe in this whole embedding are similar to your query. [01:21:47] Alex Volkov: And we've all like talked about this kind of similarity is very interesting because embedding definitely has dimensions, but it's hard to figure out if a huge document like embeds into one is how should I say, averages with everything that happens in there. And the benefit here of cold bear. [01:22:06] Alex Volkov: Finally, I'm pronouncing this correctly. Colbert is that instead of embedding one time, it embeds per token. And am I getting this correctly? That sounds to me like a lot of compute. Is that correct? Embedding per token sounds okay, now we can compare each token from the query to each token of the document. [01:22:24] Alex Volkov: But is it significantly overhead in terms of compilation time compute? What's the downside? It sounds better on the surface. [01:22:32] Benjamin Clavie: So yeah, [01:22:33] Alex Volkov: Go ahead, Benjamin, please. Yeah. [01:22:35] Benjamin Clavie: clarification was quite clear in that, yeah, it's very clear, the problem with single vector representation is You've got a long document, and you're essentially asking the model to be like, I'm going to squeeze in every single thing that could be to know about this document into 500 floats or something, which is not a lot of space. [01:22:54] Benjamin Clavie: But, Colbert takes more storage space, to answer your question, like you will need to store more tokens even though there are compression techniques, and we'll get into that later. But compute wise, it's essentially the same, because when you're using any sort of transformer model, you'll be attending to every token anyway. [01:23:09] Benjamin Clavie: The only difference is Colbert actually stores those, instead of just averaging them at the end. [01:23:15] Alex Volkov: Oh, so the, on the output of something like Colbert, you actually get all of the [01:23:20] embeddings per token and not just one embedding per the whole document. And then you can, it's like the storage is higher, but you can actually use those for more, better, higher quality comparisons. That's what we're talking about here. [01:23:33] Alex Volkov: Is that correct? [01:23:35] Benjamin Clavie: That's the gist of it, yeah. And then after Colbert You've got Colbert V2 and PLED, which is essentially Omar and Tim found out that, yeah, that does take a lot of space, but can we compress the embeddings? So most of the time when you see Colbert using production, it actually compresses every single token vector to just one or two bits. [01:23:56] Benjamin Clavie: So don't take that much space [01:23:58] Alex Volkov: Oh, so Colbert v2 is, what, 10x size or something comparison, right? Something like this. Conor, can you speak about this? Cause obviously you're in the vector dataset space. The more folks host, the better it is, for you guys. Cause you get a pet token. Can you just speak about the size of this and like the improvement as well? [01:24:20] Connor Shorten: There's a couple ways you can do this quantization. The most common is just to have k means on the segments. You divide vectors and every two contiguous values you would then cluster that and then reduce the precision to like, eight bits, so when you quantize the token vectors, you can take down the storage overhead a lot. But yeah, I think Benjamin already said it all. [01:24:43] Alex Volkov: Okay, so now let me take this into the practical realm because Colbert, the original paper came out in 2020 and I don't remember this off the top of my head, but the way I'm reading, I have some mental documentation here that I'm using to ask you guys the proper questions. And then Colbert V2 came out and a significant compression of the data because they quantize the actual individual embeddings and performance is essentially the same, I assume. [01:25:06] Alex Volkov: And then. It also came out a while ago, and then, Benjamin, I think you're in charge, single handedly, for the resurrection, or like the renewed interest, because all of what we're saying doesn't not, doesn't sound to me super easy, as somebody who just okay, it's super easy for me to use a vector database, like wavy, other competitors, local vector stores, they all have very simple tutorials for me to just embed the query, go do a regular the nearest neighbor can then search whatever, and then just do this for the user. [01:25:34] Alex Volkov: Now, all of what we're talking about, embedding per token, like comparison, like all of these things sound complex to me, and then that's where Ragatouille comes in, correct? So can you talk about, you see all this happening, and then what's your library doing why is it in charge of the resurrection of this whole concept? [01:25:53] Benjamin Clavie: Yeah, I don't know if I'll go as far as resurrection, but yeah, Colbert is basically used by everyone who is quite aware of search, like pretty much every search startup, people at Google, etc. are using Colbert, but they don't got that big outside the poor user area, and the reason I think it's something that Omar mentioned the other day is I wouldn't say Colbert itself isn't usable, but it's not approachable. [01:26:16] Benjamin Clavie: If you go look at the repo, it's scary. There's a lot of things. How do I store those vectors, et cetera. And the point of Rege2 is trying to bridge that gap because we are now at the point, I think, where AI has users that aren't like traditional AI for users, especially in IR. Vectors are complicated. [01:26:33] Benjamin Clavie: Embeddings are complicated. And the point of Rege2 was basically like, yeah, but what if you could use Colbert and just like 4 lines of code, and I tried to build that, and it turned out to be quite easy to build, so that's how it came to be. [01:26:46] Alex Volkov: So you built it, it's quite easy for you. What is it? Just this is like a library wrapper on top of, The knowledge of how to run Colbert in production. What is the library like? Is this the lang chain for Colbert? Tell us like what folks are to expect when they open up and they say, okay, I need to use something like this. [01:27:03] Alex Volkov: This is super interesting. This is higher quality retrieval. How do I start? [01:27:09] Benjamin Clavie: Yeah, so I think there's two things here, that's where I would like it to be, and where it currently is. Where I would like it to be is to keep adding more stuff and basically bridge the gap between what's popular in IR research or retrieval, which is probably a few years ahead of what's actually popular in the mainstream because it's quite obscure. [01:27:26] Benjamin Clavie: And then what it is right now, like when you open like a tool, it's basically there's two main classes, one that you can use to fine tune and train Colbert models and hopefully more late interaction models, but right now it's just Colbert. And tries to abstract away all the hard stuff there's a thing called hard negatives, when you're training for retrieval, and you need to mime for hard negatives, and that's they're done in the background. [01:27:48] Benjamin Clavie: And then you've got the main one, which you can use to use Colbert to re rank her, or use Colbert to uncode documents in memory, or use Colbert to create an optimized Colbert index, which does the compression, etc. So it's basically, yeah, give it your documents, it will process them, and then you end up with something you can play. [01:28:04] Alex Volkov: Just from a perspective of nobody that used this model so far . Let's say I already have a vector database existing. I need to reed everything in there to start using called Bay and with regulatory. And that's what you mean by fine tune or is there like an additional thing that's called fine tune? [01:28:20] Alex Volkov: 'cause this is not like the LLM fine tune that we've talked about here on Thursday and multiple times. This is a different fine tune. What are we fine tuning? How long does it take? Does it need GPUs? If you don't mind, walk us through this. If how easy this is for the user to do. [01:28:36] Benjamin Clavie: Yeah, that's a good question. So it's actually quite similar to LLM fine tunes, just on a much smaller scale, because you would actually be fine tuning the model itself. There's another paper by Omar and team, Omar is everywhere in this link, regardless. There's another paper by Omar and team called UDA PBR, which is actually a combination of choosing DSP, so the proto DSP Y. [01:28:59] Benjamin Clavie: With Colbert to make the fine tune Colbert to any unknown domain. So any new domain, you could technically get a much better retrieval model using that. Right now there's only one implementation. That's something we would like to have in Regentoo. But yeah, the other question is, can you use joint distinct vectors with this? [01:29:17] Benjamin Clavie: The answer is no, and that's quite annoying. And when fine tune, I also mean like you can fine tune the model, but you can also just choose Colbert of the shells and use that to embed your documents and create a new index. Beef. If I have to speak of the cons, I would say there's no VectorDB except Vespa, which I don't think qualifies as a modern VectorDB we probably mean here that can use call back embeddings out of the box. [01:29:41] Benjamin Clavie: I know there's interest, maybe Connor, you guys will support it at [01:29:44] Connor Shorten: some point soon. Yeah, we're definitely working on it. I think we, I think, I do think that you've maybe understated the contribution of Ragatouille before you did this, it wasn't, it was not easy to train your own Colbert model, and it definitely wasn't something that we saw as freQwently. [01:30:03] Connor Shorten: It was like, Yeah, I think that you've definitely evangelized it. I don't necessarily agree with the most people doing search were doing it this way. Maybe I've just opened a thing, but I think most people have been doing the kind of pooled vectors thing and this is very new, but and yeah, we are working on adding it. [01:30:22] Alex Volkov: I, from my perspective, just judging by the social feeds, I agree, Benjamin, without working through it I don't think I've been even been interested. But I want to maybe ask Connor here as a follow up. So you, Ragatori, you see it blowing up, like what piques your interest in how approachable this is? [01:30:36] Alex Volkov: What's fine tuning a Colbert model mean for retrieval? You guys are like researching every retrieval technology out there as much as possible in order to bring this obviously to your users as well. Quality of retrieval is very high of a very high importance as well, but storing these like vectors in different vector databases. [01:30:54] Alex Volkov: What do you see in Ragatori like exploding and how does this translate into people are using rags better, sorry, rags better. [01:31:05] Connor Shorten: Yeah, I guess it yeah it definitely is just I think what I opened with this kind of retrieved and re rank it, collapsing it into the one thing. And I think it's really just explained it really well. I agree with you, Alex. I don't think I would have understood Colbert as well as I do now if it wasn't for Benjamin and Ragatouille. [01:31:21] Connor Shorten: So that's what I think, but under the hood, it's I think it's still like this re ranking thing where we can still use, we still use the pooled vector and like an HNSW search to surface the candidates and then we'll now bring the, the other token vectors with it. [01:31:35] Connor Shorten: And then, for Weaviate that just means opening up, like having a more generic type [01:31:40] for how we store vectors to, instead of just one vector now we have this, like an open interface. To, to let you still use the, because the pooled vector embedding search is still very popular as well. [01:31:51] Connor Shorten: The OpenAI embedding. I think the Matryoshka thing, maybe we could talk about that as well. I think that has some flavors of this. I'm not sure if it still has the same kind of hierarchy to it. But I think there's also, maybe I'm going off topic, but there's also a paper from DeepMind about semantic IDs. [01:32:06] Connor Shorten: And so semantic IDs, they're like this like hierarchical, discrete quantized things where it'd be like you Like at the, say you have three, three IDs and they're each eight bits and the first one would be like whether it's about sports or news or something like that. So there's definitely a, yeah, this is definitely like a newer thing, I would say. [01:32:25] Connor Shorten: And I hope I answered the question. I think I just did like a circle around. [01:32:28] Alex Volkov: No, with this article, definitely. I just want to touch about a concept that may be not familiar for folks here on the ThursdAI stage. Matryoshka embeddings came to my, on my radar just recently after OpenAI released their new embedding models. And one of the things they've added in their new embedding models is the ability to reduce dimensions like via API call. [01:32:45] Alex Volkov: And people were starting thinking like, Hey, how did they do this? What usually, like when you get an embedding model, you get And then some folks started saying there was this paper called Matryoshka embeddings that Matryoshka, if you guys are not visualizing what this is like the Russian dolls thing where one fits into another. [01:33:00] Alex Volkov: And there's this paper, and I think the author of Matryoshka embeddings is on my Reddit as well. Maybe we'll get him on ThursdAI that actually allows for significantly smaller, correct me if I'm wrong, way to do this. And I think. Folks from Junaid definitely talked about trying to train Matryoshka with some other stuff. [01:33:17] Alex Volkov: So this is like a new concept we haven't touched upon yet, but could potentially be an additional competitor here. I want to scroll back real quick. We have Benjamin back. Benjamin let's talk about the speed of this for like larger documents. Definitely what I Learned about Regato definitely, but also about Colbert is for larger documents. [01:33:36] Alex Volkov: I saw something, I think from Omar about just like millions of rows or something significantly faster. Could you speak about like the speed of this whole thing? Are we getting like an improvement significantly for speed? Like why would a person who already has a setup consider switching to something like this? [01:33:51] Alex Volkov: And let's talk about the seconds it takes to run through like a bunch of documents. to find similarities. [01:33:59] Benjamin Clavie: Okay, so that's, so I did miss a few things, so it might have been said already, but there's a trade off here in that creating a Colbert index as in an optimized one using quantization, like Connor said, is quite slow, like pretty slow, because it has to run k means on all your embeddings, etc., but the con, like the flip side of that is that once your documents are in an optimized index, Query is pretty much in constant time, like it doesn't matter if you've got 100 million documents or billions, it will take about 50 60 milliseconds, and that's because the indexing optimization step, I think, creates A bunch of centroids that you can use to, you can use as a gateway to documents, like to simplify things. [01:34:40] Benjamin Clavie: So query is pretty much constant, and that's a big pro of optimized Colbert indexes. I think that's what counts, because it also means that adding and deleting from a Colbert index is very slow, because you need to recompute that. And I think that's space here for some sort of hybrid approach. Also using NHSW for like smaller collections, because you don't need that sort of optimization if you've got like 10, 000 documents or something. [01:35:04] Alex Volkov: Interesting. It's just for my understanding brain this is very similar to pre compilation of some stuff versus like runtime executions or some stuff you're saying if basically you can offload. The compilation part, and your users will not basically suffer from this, right? [01:35:20] Alex Volkov: You don't have to go and call different APIs for this. If you're able to do this, and then you precompile everything, and the benefit here is larger indices, like larger, like significant larger document stores. You're talking about like millions or a hundred millions or so. But then retrieval is almost near time, like instant, under like milliseconds. [01:35:41] Alex Volkov: That's, I think, a crazy benefit for folks, especially in enterprises and different places where Yeah, I think it's like a significant improvement towards regular like search and vector comparison. Conor, would you say so as well? Because you guys are in the business of vector comparison and bringing people. [01:36:00] Alex Volkov: Are you seeing like a significant improvement from a retrieval speed here. [01:36:08] Connor Shorten: Yeah, I think the latency probably isn't too bad because you, the way that I understand Colbert is that you still, or Colbert, sorry, I would agree on Colbert, but, is that you still have the the top 100 search with HNSW and, that latency is, Pretty slow. It's gonna be like five milliseconds at a million scan. [01:36:25] Connor Shorten: That's like the most hand wavy thing ever, but and then you just bring these quantized vectors into memory to then re it's way faster than, the cross encoder approach where you're going to take those top 100 results and then append them with the query and send them to a, an inference container to get back the scores and sort them. [01:36:39] Connor Shorten: So it's way faster than that. I think maybe one thing out of what you just said that I'd want to parse is I don't think it's the same analogy as compile it or compose it at runtime. It's maybe more so like an asynchronous kind of thing where you can query the index that you currently have and then in the background, the index can start doing that k means quantization. [01:37:00] Connor Shorten: That's probably the slowest thing of as Benjamin just mentioned. Like that quantizing the token vectors and now we're, let's say we're I'm actually not familiar with the detail of exactly how many token vectors you're keeping for document, but let's say it's 512, right? [01:37:14] Connor Shorten: And now you're going to be running k means over, each of those and in parallel and then you also are, trying to multi thread the per segment codebook. So I think feeding that, fitting that codebook is going to be your challenge. And so that's probably, and then keeping that fresh because these codebooks, if that's the way you're doing it, I don't The thing about Matryoshka and it's like maybe, and it's like maybe you can get the quantized vectors out of the box with one of the embedding models, but it's the quantization schemes are pretty dependent, like dependent on your data, particularly, like you can't it's not like the embedding models that you get from the common APIs that they come with the code books. [01:37:53] Connor Shorten: You have to fit these code books to your data. So I think the way to think about it would be that we can fit these code books like asynchronously in the background and you can query what you currently have and then, the updating and having the refresh indexing that can happen with a cycle kind of way. [01:38:10] Alex Volkov: All right. I wanna maybe move towards, okay. Let's say folks are interested to trying this. Benjamin, could you could you speak about how to like. Is Regatoid the right start? Do they have to? I think you mentioned this briefly. I just want to return to this. Is this only like significantly better for a large set of documents? [01:38:28] Alex Volkov: What are the steps to getting started here and what people should know? And then I guess we'll ask about if where to find you guys and how to keep up to date with as these developments around this area happen. [01:38:43] Benjamin Clavie: So if you want to get started, I think Regato is probably definitely the easiest way to try Colbert. We've got a few example notebooks on the GitHub repository. If you want to contribute more, please do. That's the big thing. I need more documentation, more notebooks. But you can try re ranking or indexing in memory or building your index. [01:39:01] Benjamin Clavie: So I've got Finetuning pretty much out of the box. So I'd say start there. In terms of retrieval performance, like Colbert is always a really strong competitor. Performer in the existing IR literature, and we do have a re ranker, so you can just try it out, just use it to re rank before you commit to indexing your whole documents, just to see how it would perform for you. [01:39:21] Benjamin Clavie: So that could be an easy way to slot in any existing pipeline, basically, just retrieve documents, re rank them. and see what the rerun code does for you. [01:39:29] Alex Volkov: And that in that case, I think integration with existing libraries also exists for folks who use like ClangChain or LAMI index. I saw that they also integrate at least some parts of this, correct? [01:39:40] Benjamin Clavie: Yeah, and I do want to thank them for that because they basically did this within 24 hours of me reusing ReGaTu. On Lama Index you can use Colbert Indexes and on LangChain you can use Colbert Indexes and you can use like Colbert's rerun code as well. So if you already use LangChain you can add like an extra Colbert step using [01:40:00] ReGaTu in three more lines of code, I think. [01:40:02] Alex Volkov: Incredible. So folks definitely definitely who are interested in trying out what the big dogs use for search re ranking is a very easy, like without committing re ranking is a fairly easy way to get started with this and see if you get a significant performance. And Connor, we barely touched on DSPies. [01:40:19] Alex Volkov: I do want to have a conversation about because that's also all over my feed and basically Omar is all over my feed. And could you Let's say, how, does this all connect somehow with DSPies or no, and because DSPies is for the prompts area. This is more for the retrieval area. Where's the connection point that I'm missing besides Omar being everywhere? [01:40:39] Connor Shorten: I think that, oh, I think Omar being everywhere is maybe the biggest connection I, because to me it's kinda like D SPY is like optimizing the LLM program prompt part. And then I think to have the optimi optimization loop connect between that and the retrieval model, you definitely, there's works like propagator in pairs. [01:40:59] Connor Shorten: Omar has, I think, UDAPDR, something like that, where you use the LM to generate synthetic queries, then you fine tune the embedding model with that. So that's that would be where the connection would be, DSPy is like a synthetic data framework, you tell it what you want it to do, and it will use the LLMs to generate successful executions of the task, and then you use that to distill it to either small models, or to tune the prompts, or you could fine tune an embedding model. [01:41:25] Connor Shorten: I don't think it's quite, I think that would be pretty advantageous. Benjamin can take the mic from here. [01:41:32] Benjamin Clavie: Yeah, I wouldn't say DSPy and Colbert are directly related. They exist in the same space, but definitely very different tools. Like Connor mentioned, UDA PDR, which is a paper, the paper I mentioned, actually, where you use DSP and hopefully soon DSPy to fine tune a Colbert to any domain. [01:41:50] Benjamin Clavie: It's not exposed. It's never been exposed to before and get it to a state of the art result only domain. That's a really good application of DSPy to Colbert. And likewise, you can use Colbert as a retriever on your DSPI pipeline, but it's just a component, it's not quite the DSPI thing. [01:42:08] Connor Shorten: I do have something, though, that is very related to retrieval generally. [01:42:12] Connor Shorten: Is we saw all these amazing LLM query router things. I want to give Llama Index credit for evangelizing most of this stuff. But, so one example is, say you have the LLM pick a metadata filter to put on the vector search. Like you want to, search only where you're searching through, let's say you have an index of podcast clips and you want to say only where the speaker is Omar Khattab, and you have an LLM predict that filter, and then that would be in the retrieval engine. [01:42:38] Connor Shorten: And so you have this you have a prompt behind that same with text to SQL. There's a prompt behind how you we'll put these things around retrieval. And so DSPy can optimize the prompts or optimize the models that do that to get the maximum performance out. And not, I, not to, I don't mean to say anything negative about the existing frameworks, but you're right now, locking into the prompts that they have built in to the framework it do these things, whereas DSPy opens it up to optimize it for your thing. [01:43:06] Alex Volkov: Interesting. Yeah, I don't think it's negative necessarily. I think people after using some of these frameworks they understand that and we've seen this from multiple folks. This, they could potentially start with something like a Lama index or LinkedIn and then quickly figure out that some more. [01:43:20] Alex Volkov: Freedom is needed and de SPI saying is a potential kind of way to do that. Okay. Connor, anything else? Very interesting. So first of all, you have a bunch of great content on this. You recently did. I think it's been to the top of the tweet. I'll definitely add this to the show notes as well. [01:43:32] Alex Volkov: You did a deep dive into de SSPs on your, was that on the podcast or was just a video? Definitely we'll send folks there. Anything else you want to add of like, how to find you, where to find your content and definitely folks should follow you. First of all, we'll add your things. [01:43:48] Connor Shorten: Thanks, Alex. Yes, I have two podcasts right now with Omar, of course, and then I have Carol Duserlink, who's created this. Infer, Retrieve, Rank, Program. It's one of the coolest examples of DSPi. And yeah, and then I have one video out so far explaining the whole thing. Quickly, I wanted to point people to the update to DSPi Assertions. [01:44:05] Connor Shorten: Because I think this is the most important thing with these prompting frameworks. And I think it's important. to also understand Instructor from Jason Liu, which is where you use Pydantic to define the schema of the outputs that you want from the language model, and then you validate the outputs to make sure that it, outputted JSON with the keys that you wanted. [01:44:23] Connor Shorten: And so DSPi Assertions is in this similar category, and this is like the most common discussion I'm seeing in the DSPi Discord is people looking to add Instructor to DSPi and jointly looking to do this thing of like structured outputs and have this retry mechanism. There's a new work from Arnav Signal Sig, oh, sorry, Arnav Singh V. [01:44:43] Connor Shorten: We haven't met yet, but, and know more about DSPi assertions. And I'm going to link it in the description of this chat. Cause I highly recommend people check it out. [01:44:50] Alex Volkov: Awesome. Nisten, just before I give you a question I will shout out that Jason Liu from the instructor library came to the Weights & Biases courses, and there's a course that he builds with us as well that's for free. You can just go 1db. ai courses. I'll definitely add this in the link below about structured output and how to force these LLMs to give us better structure output. [01:45:09] Alex Volkov: It's funny that a person named Jason is building, you tools to get LLMs to output JSONs. But that's all I have. Just super quick. Nisten, go ahead. You had a question here. [01:45:19] Nisten Tahiraj: I just want to say it's pretty amazing that the people we bring here are from the industry. We actually use, like from last week, I started using Lilac, I might actually start running Ragatouille on that on that Hacker Neon dataset. And so I wanted to know and mainly since some people ask in the comments, what have I used, I forced myself to only use open source models. [01:45:45] Nisten Tahiraj: And cause I feel like that's the only way they're going to start getting better if we restrict themselves to them. I don't recommend you do it just yet, just wait another. Maybe a week or two but I want, I wanted to ask uh, we see some limitations with retrieval augmentation systems, like in GPT 4 when people use it. [01:46:07] Nisten Tahiraj: It only gives three points from the document, doesn't really summarize it and stuff. What are the benefits of going with the Colbert? I'm sorry. Is it because it's much faster? Can you feed it many more documents? I'm talking from a practical point of view, not necessarily even from a tech person's point of view, like as a business who has a lot of customer data why should they use this versus just putting it on pgVector and doing function calling? [01:46:41] Nisten Tahiraj: Is this faster that way? And what limitations does using again, RegA2 with Colbert [01:46:47] Benjamin Clavie: have? That is a good and open question. So limitations we have a lot right now, like the lack of Cloud hosting offering is a big one. There's not really somewhere you can host this except doing it yourself, which is a big problem. [01:47:05] Benjamin Clavie: And the main reason to use it, I would say, is generalization because the thing when you use any of the shared submitting models is they look good on benchmarks, and they tend to work quite well, but they've been optimized for those benchmarks. Whereas Colbert, for instance, like Colbert V2, has never been trained on the MTEB benchmark for retrieval, etc. [01:47:24] Benjamin Clavie: The reason it generalizes well is because working at the token level makes it a lot easier for your model to encode information. Whereas, like, when you're trying to squeeze everything into a single vector, it might not very well, not work very well, say, for your custom domain. Whereas with Colbert, you can always assume it's going to be okay in every domain, but if it's not the best, you will need to fine tune it later. [01:47:45] Benjamin Clavie: It's probably the biggest draw, I'd say. [01:47:51] Alex Volkov: Awesome. So I definitely wanna thank you guys for coming up and explaining these concepts that have been floating around in very simple language. And I appreciate your patience with me re asking this in the way that I understand, because I know definitely that this is my way to understand, but also some folks in the audience. [01:48:06] Alex Volkov: That's how we do here on ThursdAI, so more than welcome to rejoin. For I now consider both of you friends of the pod, so I agree with Nisten. It's really cool to see the authors of the libraries and the tools that we use. Come here to ThursdAI to talk about them, [01:48:20] and obviously, upcoming features as well. [01:48:22] Alex Volkov: Definitely welcome. Benjamin, thank you for doing a bunch of open source stuff, and evangelizing the whole con birth call birth thing to make it simpler for folks. Definitely, thank you. And any anything you want to add here that I haven't touched yet? Please go ahead, Benjamin. [01:48:36] Benjamin Clavie: I do have a few shoutouts, shall we say. One of them is that LungChain and DSPy are not mutually exclusive, and I shared that in the chat. There is now LungChain x DSPy integration, where you can define your chains in LungChain and still use DSPy to optimize things, which is pretty cool. [01:48:53] Benjamin Clavie: And in the embedding world, so you mentioned Matrioshka embedding, and we talked about Colbert, and the people at JIN are actually training a Colbert model right now using Matrioshka embedding for compression, as like some sort of let's try this out, see how it works. And the final one is, you might have brought this up already, but the people at BAI train, like really, BGM3, as a really cool embedding model that in a single pass outputs. [01:49:19] Benjamin Clavie: Dan's Vector, Burst, or Colbert Style Multivector Implantation, and the Splate Style Sparse Implantation. I won't go into too much detail about that, [01:49:26] Alex Volkov: I'm sorry. I don't think I covered that. Who was that? Sorry. Could you repeat? [01:49:31] Benjamin Clavie: The people at BAAI, the people who do the BGE [01:49:34] Alex Volkov: Oh yeah, but yeah. We've talked about their model recently. They, [01:49:37] Benjamin Clavie: ABI, yeah, [01:49:38] Alex Volkov: Oh, I did not know. [01:49:39] Alex Volkov: So they're now have a thing where outputs a regular embedding and also called burst style embedding. [01:49:46] Benjamin Clavie: Yeah, the big thing last week was M3, which has a Colbert Style Embedding, Splate Style Embedding, which is a Sparse Implantation method, and Dan's Embedding, which is just a single model, a total of three. [01:49:57] Alex Volkov: Oh, that's incredible. Okay. So we're adding some knowledge here. Thank you for, let me just repeat just the way that I hear this, we've talked about the BAAI BGE M3. M3 basically stands for multiple things. One of them is multilinguality. So they upgraded their embeddings to use not only English, but also I think a hundred languages as well. [01:50:14] Alex Volkov: So now Benjamin, you're saying they're also implementing for us this step, the output, the dense embedding, but also the. The call Burr embedding, correct? [01:50:25] Benjamin Clavie: yeah, yeah, one of the meanings of M, I think, is [01:50:27] Alex Volkov: Multicomposability or some con yeah. Multifunctionality. Yes, exactly. [01:50:33] Benjamin Clavie: can use it to generate different kinds of embedding. And I think that the first Non Colbert, actually like strong multi vector model. There's issues as in the vectors are too big, etc. [01:50:45] Benjamin Clavie: But it's a very nice thing to see happen. Definitely, like [01:50:49] Alex Volkov: Oh, definitely shout out then we need to get the folks from BA AI here to speak about this. So if you folks know them, definitely connect them to me. I would love to hear about from the authors of BG. Yeah, definitely shouts out Junaid. I think Bo Wang, we've mentioned he's a friend of the pod. [01:51:03] Alex Volkov: He came when Junaid released embeddings and he often comes here and gives us like insights about how embeddings work. Shout out Bo and the team with Junaid as well. Connor your stage, if you want to add everywhere else where folks. You can follow or shout out your stage. And then we're going to continue with some more news. [01:51:21] Connor Shorten: It's been so cool to be a part of the podcast. And I love how it's integrated into X because this is actually my favorite place to manage communication. So if you want to reach out, here would be great. [01:51:31] Alex Volkov: Yeah. So definitely give a Connor a follow and a Wave8 podcast is incredible. We've been, by we, Wits and Biases. We had a mutual video together and Connor hosted our folks. And there was a, I learned a bunch of it before I joined Wits and Biases as well. A great source of information from both of you. [01:51:45] Alex Volkov: Thank you guys so much for coming up, explaining these complex. on the surface concept to us, maybe complex also implementation wise, but making them simpler as well. I think it's very important talking about them. I think it's very important and you are now considered friends of ThursdAI community and hopefully this will get more folks to learn about this, contribute, etc. [01:52:05] Alex Volkov: And I think with that, we're like, a bit over the top, like two hours since I started the recording. We had a great show today. Thank you everybody for listening and coming. I just wanna summarize this in a few notes that that I really enjoy my time here every week. And I really enjoy learning from folks. I think Nisten, you mentioned today that it's so cool to have the authors of the things we talked about. [01:52:25] Alex Volkov: So today we also had this benefit. We had Benjamin here and we had Connor who covered this. And we also had Justin again from the Qwen team to talk about the Qwen stuff that they released. And it's really cool that the community now connects different people. [01:52:36] Alex Volkov: So I was able to connect Justin and the Qwen team with the LM studio folks and Olama folk. No, I think only LM studio. And they were able to work together that they release is now supported in LM studio. the second they release something. So I love how this community comes together. I encourage everybody who listens to this to also participate in this. [01:52:55] Alex Volkov: Either follow everybody who's on stage here interact with our posts and boost the signal a little bit. Tell your friends if you're working with friends and they don't listen to ThursdAI. And there's alpha in listening to ThursdAI like today definitely tell your friends where this alpha can be found. [01:53:10] Alex Volkov: And with that, I want to thank you all and have a nice Thursday. Bye bye, everyone. This is a public episode. If you'd like to discuss this with other subscribers or get access to bonus episodes, visit sub.thursdai.news/subscribe | |||
16 Feb 2024 | 🔥 ThursdAI - Feb 15, 2024 - OpenAI changes the Video Game, Google changes the Context game, and other AI news from past week | 01:57:37 | |
Holy SH*T, These two words have been said on this episode multiple times, way more than ever before I want to say, and it's because we got 2 incredible exciting breaking news announcements in a very very short amount of time (in the span of 3 hours) and the OpenAI announcement came as we were recording the space, so you'll get to hear a live reaction of ours to this insanity. We also had 3 deep-dives, which I am posting on this weeks episode, we chatted with Yi Tay and Max Bane from Reka, which trained and released a few new foundational multi modal models this week, and with Dome and Pablo from Stability who released a new diffusion model called Stable Cascade, and finally had a great time hanging with Swyx (from Latent space) and finally got a chance to turn the microphone back at him, and had a conversation about Swyx background, Latent Space, and AI Engineer. I was also very happy to be in SF today of all days, as my day is not over yet, there's still an event which we Cohost together with A16Z, folks from Nous Research, Ollama and a bunch of other great folks, just look at all these logos! Open Source FTW 👏 TL;DR of all topics covered: * Breaking AI News * 🔥 OpenAI releases SORA - text to video generation (Sora Blogpost with examples) * 🔥 Google teases Gemini 1.5 with a whopping 1 MILLION tokens context window (X, Blog) * Open Source LLMs * Nvidia releases Chat With RTX local models (Blog, Download) * Cohere open sources Aya 101 - 101 languages supporting 12.8B model (X, HuggingFace) * Nomic releases Nomic Embed 1.5 + with Matryoshka embeddings (X) * Big CO LLMs + APIs * Andrej Karpathy leaves OpenAI (Announcement) * OpenAI adds memory to chatGPT (X) * This weeks Buzz (What I learned at WandB this week) * We launched a new course with Hamel Husain on enterprise model management (Course) * Vision & Video * Reka releases Reka-Flash, 21B & Reka Edge MM models (Blog, Demo) * Voice & Audio * WhisperKit runs on WatchOS now! (X) * AI Art & Diffusion & 3D * Stability releases Stable Casdade - new AI model based on Würstchen v3 (Blog, Demo) * Tools & Others * Goody2ai - A very good and aligned AI that does NOT want to break the rules (try it) 🔥 Let's start with Breaking News (in the order of how they happened) Google teases Gemini 1.5 with a whopping 1M context window This morning, Jeff Dean released a thread, full of crazy multi modal examples of their new 1.5 Gemini model, which can handle up to 1M tokens in the context window. The closest to that model so far was Claude 2.1 and that was not multi modal. They also claim they are researching up to 10M tokens in the context window. The thread was chock full of great examples, some of which highlighted the multimodality of this incredible model, like being able to pinpoint and give a timestamp of an exact moment in an hour long movie, just by getting a sketch as input. This, honestly blew me away. They were able to use the incredible large context window, break down the WHOLE 1 hour movie to frames and provide additional text tokens on top of it, and the model had near perfect recall. They used Greg Kamradt needle in the haystack analysis on text, video and audio and showed incredible recall, near perfect which highlights how much advancement we got in the area of context windows. Just for reference, less than a year ago, we had this chart from Mosaic when they released MPT. This graph Y axis at 60K the above graph is 1 MILLION and we're less than a year apart, not only that, Gemini Pro 1.5 is also multi modal I got to give promps to the Gemini team, this is quite a huge leap for them, and for the rest of the industry, this is a significant jump in what users will expect going forward! No longer will we be told "hey, your context is too long" 🤞 A friend of the pod Enrico Shipolle joined the stage, you may remember him from our deep dive into extending Llama context window to 128K and showed that a bunch of new research makes all this possible also for open source, so we're waiting for OSS to catch up to the big G. I will sum up with this, Google is the big dog here, they invented transformers, they worked on this for a long time, and it's amazing to see them show up like this, like they used to do, and blow us away! Kudos 👏 OpenAI teases SORA - a new giant leap in text to video generation You know what? I will not write any analysis, I will just post a link to the blogpost and upload some videos that the fine folks at OpenAI just started releasing out of the blue. You can see a ton more videos on Sam twitter and on the official SORA website Honestly I was so impressed with all of them, that I downloaded a bunch and edited them all into the trailer for the show! Open Source LLMs Nvidia releases Chat With RTX Chat With Notes, Documents, and Video Using Gradio interface and packing 2 local modals, Nvidia releases a bundle with open source AI packaged, including RAG and even Youtube transcriptions chat! Chat with RTX supports various file formats, including text, pdf, doc/docx, and xml. Simply point the application at the folder containing your files and it'll load them into the library in a matter of seconds. Additionally, you can provide the url of a YouTube playlist and the app will load the transcriptions of the videos in the playlist, enabling you to query the content they cover. Chat for Developers The Chat with RTX tech demo is built from the TensorRT-LLM RAG developer reference project available from GitHub. Developers can use that reference to develop and deploy their own RAG-based applications for RTX, accelerated by TensorRT-LLM. This weeks Buzz (What I learned with WandB this week) We just released a new course! Hamel Hussein released a course on enterprise model management! Course name: Enterprise Model ManagementCourse Link: wandb.me/emm-courseWho is this for: The course is targeted at enterprise ML practitioners working with models: MLOps engineers, ML team leaders, ML engineers. It shows both at conceptual and technical level how to get the most value of W&B Model Registry and automations. Attached is also a screenshot of a slide from the course on what different personas (MLOps, ML exec etc) get from Model Registry.What can they expect: Learn how to store, version, and evaluate models like top enterprise companies today, using an LLM training & evaluation example. Big value props: improved compliance, collaboration, and disciplined model development. Vision & Video Reka releases Reka Flash and Reka Edge multimodal models Reka was co-founded by Yi Tay, previously from DeepMind, trained and released 2 foundational multimodal models, I tried them and was blown away by the ability of the multi-modals to not only understand text and perform VERY well on metrics (73.5 MMLU / 65.2 on HumanEval) but also boasts incredible (honestly, never before seen by me) multi modal capabilities, including understanding video! Here's a thread of me getting my head continuously blown away by the quality of the tonality of this multimodality (sorry...😅) I uploaded a bunch of video examples and was blown away, it understands tonality (with the dive dive Diiiiive example) understands scene boundaries, and does incredible OCR between scenes (the Jason/Alex example from speakers) AI Art & Diffusion Stable Cascade (link) Stability AI introduced a new text-to-image generation model called Stable Cascade that uses a three-stage approach to produce high-quality images with a compressed latent space, making it more efficient to train and use than previous models. It achieved better results than other models in evaluations while having faster inference speeds. The company released code to train, fine-tune, and use control models like inpainting with Stable Cascade to enable further customization and experimentation. Stability AI aims to lower barriers to AI development through models like this one. Nate did a comparison between a much slower SDXL and Stable Cascade here: Here’s the transcript for the whole episode, you definitely should check it out! It was really one of the coolest shows we had, and we had over 2K folks listening in! [00:00:00] Alex Volkov: Hey, this is Alex Volkov, you're on ThursdAI, and I just gotta record this intro real quick, because today marks one of the more singular days in AI that I remember since I started recording ThursdAIs, which was itself a singular day, March 14th, 11 months ago, when GPT 4 was released and announced. We since then had a few days like this GPT Dev Day was one such day, and today marks another one. [00:00:38] Alex Volkov: Google has released an update to their model, talking about 1 million tokens in the context window, basically unlimited. And then, just a few, just an hour or two later, OpenAI said, you know what, we also have something in store, and released the most incredible jump. Incapability of video generation, text to video generation. [00:01:02] Alex Volkov: It's called SORA, and what you hear is us recording live, knowing only about Google, which came out an hour and a half before we started recording, and then somewhere in the middle, I think minute 35 or something, you'll hear our live reaction to the Incredibly mind blowing advancement in text to video that OpenAI just released. [00:01:31] Alex Volkov: And I just wanted to record this as I'm finishing up the editing and about to start writing the newsletter, to say, days like this really are the reason why I'm all in on AI and I'm very excited about the changes and advancements. [00:01:49] Alex Volkov: And I'm sure there will be more days like this going forward. We've yet to see what Apple came up with, we've yet to really see what Meta comes up with Llama 3, etc. And, yeah, I just wish you enjoyed this and I don't have a lot of words here besides just letting you listen to the rest of the episode and say that I was very happy to be in San Francisco for this, the place where most of this happens, and I was very happy to be in company of good friends, both in the virtual world those on stage in our Twitter live recording, and I was sitting across from Swyx, a friend of mine with whom I recorded an interview at the end of this, you can hear. [00:02:30] Alex Volkov: I just couldn't let go of this chance. We also had a conversation, besides the updates and the breaking news, we also had conversations with the folks who worked on some of the stuff we talked about. I interviewed Yi Te and Max Bain from RECA, which you'll hear later, and the deep dive into RECA multimodal models, which blew me away just yesterday. [00:02:52] Alex Volkov: And so my head kept getting blown away this week. And I also interviewed The folks who built Stable Cascade, a new stability model that outperforms the existing stability models. Dome, and Pablo. And all of those were great conversations, in addition to just generally the folks who joined me from week to week, Nisten and Far El and Alignment Lab, and we had Robert Scoble join us, with whom I've been buddying up since Vision Pro was released, as he was expecting, and that blew me away just a week ago. [00:03:23] Alex Volkov: And I'm very excited to share with you this whole thing, and I hope that Yeah, I hope you enjoyed this as much as I do, and I hope that you enjoyed listening to these as much as I enjoy making them. And if you are, just share them with a friend, it would really help. And give us a 5 star review on Apple. [00:03:38] Alex Volkov: This would great, gratefully help. With that, I'll give you the ThursdAI thing. [00:03:43] Alex Volkov: All right, let's go. How's it going, everyone? Welcome to ThursdAI. Today is February 15th, and it's quite a day in the AI updates that we've had so far. Quite a day. Even today, this morning, we had like a bunch of updates. But besides those, we had quite a crazy week as well very interesting show today, very interesting show today. [00:04:13] Alex Volkov: My name is Alex Volkov, I'm an AI evangelist with Weights Biases, and right now I'm getting my picture selfie taken by my today's co host, Swyx. Welcome, [00:04:23] Swyx: Hey, hey, hey. Good morning, everyone. [00:04:25] Alex Volkov: And we're in the Latent Space Studio in San Francisco. I flew in just last night. And as I was flying in, there was more news happening. So we're going to cover all of this. [00:04:34] Alex Volkov: We have a very exciting show today. We have a bunch of guests, special guests that are coming on the second hour of this. So hopefully we'll see folks from the RECA models, and hopefully we'll see some folks from Stability. We're going to get to talk about Google and everything in between. So meanwhile, settle in. [00:04:50] Alex Volkov: This is going to be a great show today in San Francisco. And maybe I'll also probably share with you why I Flew in here today. That's gonna come up next. So welcome to ThursdAI and we're gonna get started. All right there. Let's get started. Let me Smoothly fade out the music, say hi to everyone here on stage. Hey, Nisten, welcome. We have Robert Skobul over here, folks. We've been, we've been more, more friendly lately than usual because Robert and I are both members of the VisionPro cult. I think that's what you call it, Robert. [00:05:37] Alex Volkov: But today is, today's the space for, for AI. But Robert you've been covering AI on your feed as well for, for a long time. We have, obviously Swyx is on stage, but also in front of me, which is super cool. And it's been a while, brother. It's great, you just flew back from [00:05:51] Swyx: Singapore. [00:05:52] Swyx: Yeah, Chinese New Year. [00:05:53] Alex Volkov: Are you jet lagged at all or are you good? [00:05:55] Swyx: I'm good actually. I have had very little sleep, but for some reason that always helps with the jet lag. [00:06:00] Alex Volkov: Yes, awesome. And I also want to say hi to Alignment Labs, Austin and Far El as well, folks who are working on open source models, and we usually cover a bunch of stuff that they're doing, and usual co hosts and experts here on ThursdAI. [00:06:11] Alex Volkov: So if you never joined ThursdAI before, just a brief kind of recap of what we're doing. As I said before, my name is Alex Volkov. I'm an AI evangelist with Weights Biases. It's always so fun to say. And Weights Biases is a company that is basically helping all these companies build their AI models, and it's super cool. [00:06:26] Alex Volkov: And I flew in, I went to the office last night, and I have some cool videos to share with you from the office as well. [00:06:32] Alex Volkov: and this [00:06:33] Alex Volkov: is ThursdAI. ThursdAI is a Twitter space and newsletter and podcast that I started a year ago. And then slowly this built a community of fine folks who show up to talk about everything that happened in the world of AI for the past week. [00:06:46] Alex Volkov: And there hasn't been many weeks like this last week that highlight how important and how cool ThursdAI actually is. Because we just had So much, so much to cover today and usually I start the space with a roundup of the stuff that we're going to run through just for folks who are not patient, don't have a lot of time and we're going to just run through everything we're going to talk about and then we're going to dive deep because we have some breaking news and I even have, hopefully, I have my breaking news button. [00:07:16] Alex Volkov: Oh, I don't. Oh my God. Okay. [00:07:17] Swyx: Oh no. [00:07:17] Alex Volkov: I'm not set up for a breaking news button, but it's fine. [00:07:20] Alex Volkov: We'll imagine this. I'm going to put this in the, in the, in the post edit. With that said, are you guys ready for a brief recap? Let's go to a brief recap. [00:07:27] Recap and TL;DR [00:07:27] Alex Volkov: Alright, folks, back for the recap. Today is Thursday. ThursdAI, February 15th. This is a recap of everything we talked about. And, ooh, boy, this was one of the worst days to be caught outside of my own personal production studio because my, my breaking news button didn't make it all the way here. And there was so much breaking news. [00:07:57] Alex Volkov: So obviously as I woke up, the biggest breaking news of today was Ai. Actually cannot decide what was the biggest breaking news. So the first piece of breaking news from today was Google releasing a teaser of Gemini 1. 5. And 1. 5 was not only a continuation of Gemini Pro that we got last week, 1. 5 actually was teased with up to 1 million, a whopping 1 [00:08:20] million tokens in the context window, which is incredible. [00:08:23] Alex Volkov: It's just for comparison, JGPT is currently at 128 and cloud to the best. Highest offering up until Gemini was 200k with Entropic Cloud Advanced and Google teased this out of the gate with 1 million token and their claim they have up to 10 million tokens of context window in in in the demos, which is incredible. [00:08:44] Alex Volkov: And they've shown a bunch of demos. They did the needle in the haystack analysis that we've talked about from Greg Cumbrand and just quite an incredible release from them. They talked about that you can put a whole like hour of a movie of Dustin Keaton, I think it's called. And then you can actually ask questions about the movie and we'll give you the exact. [00:09:03] Alex Volkov: Timestamp of something happens. They talked about it being multimodal where you can provide a sketch and say, Hey, when this, this scene happened, it will pull out just like incredibly like magic, mind blowing, mind blowing stuff. And all of this needs a lot of context because you take this, you take this video, you turn it into images, you send this into context. [00:09:22] Alex Volkov: They also talked about, you can send 10 hours of audio within one prompt and then some ad, And the quality of retrieval is very, very high. You're talking about like 90 plus percentage, 95 plus percentage in the haystack, which is incredible. Again, we had Enrico Cipolla, a friend of the pod who worked on the Yarn paper and the rope methods before extending the LLAMA context. [00:09:46] Alex Volkov: And he brought like four papers or something that show that open source is actually unlocking this ability as well. And not only today was a credible day just generally, but not only Google talked about a large context window, we also saw that Nat Friedman and Daniel Gross just invested 100 million in a company called Magic, that they also talk about multimodality and large context window up to 1 million as well. [00:10:08] Alex Volkov: So it was very interesting. To see both of them release on the same day as well. We then geeked out about Gemini. We talked about Andre Karpathy leaving open AI and, and invited him to come to Thursday AI and latent space as well. And then we also mentioned the OpenAI ads, memory and personalization to charge G bt, which is super cool. [00:10:25] Alex Volkov: They didn't release it to many people. Yeah, but personalization is my personal thread of 2024 because these models, especially with the larger, larger context window with personal per perfect recall, these models will. become our buddies that will remember everything about us, specifically, especially tied into different devices. [00:10:43] Alex Volkov: Like the tab that's somewhere here behind me is getting built in San Francisco. We, we briefly mentioned that NVIDIA released the chat with RTX local models that you can download and run your NVIDIA GPUs. It has rack built in. It has a chat with YouTube videos and super cool. We talked about Cohere release and AYA 101 multimodal. [00:11:01] Alex Volkov: And our friend of the pod Far El was talking about how he wasn't finding like super impressive. Unfortunately, He dropped in the middle of this. Apologies for El, but Cohere released a big multi model, which is also pretty cool. We mentioned that NOMIC, our friends at NOMIC, which we mentioned last week, released open source embeddings. [00:11:17] Alex Volkov: If you guys remember, they released an update to those embeddings, NOMIC Embed 1. 5 with Matryoshka embeddings. Matryoshka. is obviously the name for the Russian doll that like sits one inside each other. And we're going to actually talk with the authors of the Matryoshka paper in not the next Thursday, the next after that. [00:11:34] Alex Volkov: So we're going to cover Matryoshka but it's what OpenAI apparently used, not apparently, confirmed they used to reduce dimensions in the API for embeddings. Super cool. We're going to dive deep into this. As we're going to learn, I'm going to learn, you're going to learn. It's going to be super cool. [00:11:48] Alex Volkov: And as we're talking about OpenAI I got a ping on my phone because I'm subscribed to all updates from their main account and we had a collective holy s**t moment. Everybody's jaw was on the floor because OpenAI just released Sora, which is a foundational video model, text to video model, that just blew us the F away, pardon my French, because of the consistency. [00:12:08] Alex Volkov: So if and if you've seen The how should I say the area of video generation has been has been evolving fairly quickly, but not as quick as what we just saw. We saw first we saw attempts at taking stable diffusion rendering frame by frame and the consistency wasn't there. It was moving from one to to another, like the face would change and everything. [00:12:30] Alex Volkov: You guys saw this, right? So we moved from the hallucinatory kind of videos to Towards consistency videos where stable diffusion recently released and gave us SVD, which was like one to two to three seconds videos. Runway ML gives you the option to choose where the video is going to go. If it's going to be zoom in like brushes, all these things. [00:12:49] Alex Volkov: And now all of them seem just so futile because open the eyes, Sora, can generate up to 60 seconds of a video. And honestly, we were sitting here just watching all of us just open the Sora website, and we were just mind blown away by the consistency and the complexity of the scenes that you can generate, the reflections. [00:13:06] Alex Volkov: There was one scene where a woman was walking through the, a very busy street in Japan, and her coat stays the same, her face stays the same. There's another where a Dalmatian dog climbs out of one window and jumps into another. All the spots on the Dalmatian are perfect. perfectly in balance the legs are it's it's really unbelievable how high quality of a thing OpenAI released and what's unbelievable to me also is that The jump from what we saw in video to the open source stuff, or even the runway stuff and Pico stuff, the jump in fidelity, in quality, in consistency, is so much higher than the jump from like 200, 000 tokens to 1 million tokens that Google did. [00:13:44] Alex Volkov: So it does feel like some folks in OpenAI sat there and said, Hey, Google just released something. It's super cool. It's picking up attention on Twitter. Let's release something else that we have behind the scenes. It looked super polished. So shout out to the folks who worked on Sora. It's really, if you haven't seen The videos, you'll see them in show notes and definitely you'll see them everywhere because Hollywood is about to get seriously, seriously disrupted with the, just the level of quality is amazing. [00:14:08] Alex Volkov: Compare this with all the vision and, and, and sound stuff. I, moving back to the recap, I'm getting excited again. We also, then we talked about Reka and Reka Flash and Reka Edge from a company called Reka AI. And then, as I love bringing the people who actually built. the thing to talk about the thing. [00:14:23] Alex Volkov: So we had Yitei and we had Max as well from Reka. Max made for Reka to talk to us about their multimodels. I was very, very impressed with Reka's multimodal understanding. And I think this model compared to Gemini Pro, which is probably huge and runs all the GPUs and TPUs. This model is 21 billion and Reka Edge is even smaller. [00:14:41] Alex Volkov: And yet it was able to understand my videos to an extent that even surprised the guys who were the co founders of the company. It understood tonality, understood text. And audio in a very specific and interesting way. So we had a conversation with the RECA folks and continuing on this thread. We also had a new model from Stability called Stable Cascade that is significantly faster than SDXL and generates hands and text out of the blue. [00:15:07] Alex Volkov: It's based on something called Worst Chen, which we learned is a hot dog today. And we had the folks that work behind this, Dom and I'm blanking on the name of the other author that joined. I apologize. It was a very exciting day. So we had a conversation with the guys behind Worshen and Stable Cascade as well. [00:15:24] Alex Volkov: So definitely check this out. We mentioned that WhisperKid runs now on watchOS, which is quite incredible because Siri's voice to text is still not that great. And I think that's mostly of what we discussed. And then I flipped the mic on my, on my friend here that sits in front of me and I just had a deep dive interview with Swyx. [00:15:41] Alex Volkov: In the latent space, he just posted a few images as well, and it was a great conversation as well, so definitely worth a follow and a listen if you haven't listened to this. With that, I think we recap ThursdAI on one of the more seminal days that I remember in the AI one after another, and we all hope that, Meta will just release Llama 3 [00:16:01] Investments updates from Swyx [00:16:01] Alex Volkov: Unless I missed some stuff that's very important. I'll just double check. Nisten, out of the stuff that we've sent, did I miss anything else? Swyx, did I miss anything else? [00:16:10] Swyx: Today there was also a LangChain Series A. True. With LangSmith. [00:16:13] Swyx: Yes. There was Magic. dev, Series A with Nat Friedman. [00:16:16] Alex Volkov: So I was thinking to cover this around the Google stuff because they also announced a longer context craziness. [00:16:21] Alex Volkov: But definitely, definitely both of those. [00:16:23] Swyx: Lambda Labs, Alonzo 300 million, Series C. [00:16:26] Alex Volkov: Oh, wow, yeah, I even commented. I said, hey, Mitesh good. So we love Lambda, definitely. Most of the stuff that we play around with is happening in Lambda. And [00:16:34] Swyx: Lindy also had their GA launch today. [00:16:37] Alex Volkov: nice. Okay. Today [00:16:38] Swyx: Today was a very bad day to launch [00:16:40] things, because everyone else launched [00:16:41] Swyx: things. [00:16:41] Swyx: Yes. If you're not Gemini, it's going to be a struggle [00:16:44] Alex Volkov: I was just thinking, magic. dev, and I guess let's move to just discussing kind of the breaking news of the hour, as we already is. Let's talk about Google, and Gemina 1. 5. [00:16:55] Google teases Gemini Pro 1.5 with 1M context windows [00:16:55] Alex Volkov: Do we do a musical transition? Sure, let's do a musical News. This is not the Breaking News music. By not even a stretch, this is not a Breaking News music. But, imagine that we have Breaking News right now, because we do. Just an hour or so ago, we had an update from Jeff Dean and then Sundar Pichai and then a blog post and then a whole thread and a bunch of videos from Google. [00:17:27] Alex Volkov: And if you guys remember some Google videos from before, these seem more authentic than the kind of the quote unquote fake video that we got previously with Gemini Ultra. So just a week after Google released Gemini Ultra, which is now available as aka Gemini Advance. And just a week after they killed Bard almost entirely as a concept they're now teasing. [00:17:48] Alex Volkov: Teasing did not release, teasing. Gemini 1. 5, 1. 5, they're teasing it and they're coming out with a bang. Something that honestly, folks at least for me, that's how I expect Google to show up. Unlike before, where they're like lagging after GPT 4 by eight months or nine months, what they're doing now is that they're leading a category, or at least they're claiming they are. [00:18:07] Alex Volkov: And so they released Gemini 1. 5, and they're teasing this with a whopping 1 million tokens. in context window on production and up to 10 million tokens in context window in research. And just to give a context, they put like this nice animated video where they put Gemini Pro, which they have currently, not 1. [00:18:26] Alex Volkov: 5, the Pro version. is around 32, I think, and then they have GPT 4 with 128 and then they show Cloud 2 is at 200k and then Gemini 1. 5 is a whopping 1 million tokens, which is ridiculous. Not only that, they also came a little bit further and they released it with the Needle in Haystack analysis from our friend Greg Kambrad, which usually does this. [00:18:50] Alex Volkov: We'll not be able to pronounce his name. I asked Greg to join us. Maybe he will. A needle in a haystack analysis that analyzes the ability of the model to recall whether or not it's able to actually process all these tokens and actually get them and understand what happens there. And quite surprisingly, they show like 99 percent recall, which is incredible. [00:19:10] Alex Volkov: And we all know, previously in long context windows, we had this dip in the middle. We've talked about the The butter on toast analogy, where the context or attention is like the butter and context window is the toast and you spread and you don't have enough for the whole toast to spread evenly. [00:19:27] Alex Volkov: We've talked about this. It doesn't seem, at least [00:19:30] Alex Volkov: on [00:19:30] Alex Volkov: the face of it, that they are suffering from this problem. And that's quite exciting. It is exciting because also this model is multi modal, which is very important to talk about. They definitely show audio and they are able to scrub through, I said, they said, I think they said 10 hours of audio or so. [00:19:47] Alex Volkov: Which is quite incredible. Imagine this is going 10 hours of audio and say hey, when When did Alex talk about Gemini in ThursdAI? That would be super dope and Quite incredible. They also did video. They showed a hour of video of Buster Keaton's something and because the model is multi modal the cool thing they did is that they provided this model with a reference of with a sketch. [00:20:11] Alex Volkov: So they drew a sketch of something that happened during this video, not even talking about this, just like a sketch. And they provided this multimodal with an image of this and said, when did this happen in the video? And it found the right timestamp. And so I'm very, very excited about this. If you can't hear from my voice, Swyx can probably tell you that it looks like I'm excited as well, because it's, it's quite. [00:20:31] Alex Volkov: As far as I'm considering a breakthrough for multiple reasons. And now we're gonna have a short discussion. [00:20:35] Enrico taking about open source alternatives to long context [00:20:35] Alex Volkov: I want to say hi to Enrico here. Enrico welcome up on stage. Enrico Cipolli, one of the authors of the Yarn paper. And like we've had Enrico before talk to us about long context. Enrico, as we send this news in DMs, you replied that there have been some breakthroughs lately that kind of point to this. [00:20:51] Alex Volkov: And you want to come up and say hi and introduce us briefly. And let's chat about the long context. [00:20:57] Enrico Shipolle: Hi, Alex. Yeah, so there actually have been a lot of research improvements within the last couple months, even from before we submitted YARN. You could still scale even transformers to millions of essentially context. length back then. We previously in YARN worked on scaling the rotary embeddings, which was a traditional issue in long context. [00:21:19] Enrico Shipolle: So I, if you don't mind, I'll probably go through some of the research really quickly because unfortunately, [00:21:25] NA: so on January 2nd, there was one called, it's called LLM, maybe long LLM. That's a mouthful essentially, but they were showing that you can process these long input sequences during inference using something called self extend, which it allows you to basically manage the context window without even fine tuning these models. [00:21:48] NA: And then on January 7th, 2024, there was another paper that released, it's called Soaring from 4k to 400k, which allows you to extend like the LLM's context with something called an activation beacon. With these activation beacons, they essentially condense raw activation functions in these models to a very like compact form, which essentially the large language model can perceive this longer context. [00:22:14] NA: Even in a smaller context window, the great thing about these activation beacons or the LLM, maybe long LLM, is essentially they only take a few lines of code to modify the transformer architecture and get all these massive performance benefits for long context inference. [00:22:33] Alex Volkov: Are [00:22:33] Alex Volkov: you serious? Are we getting one of those breakthroughs that take two lines of code, kind [00:22:37] NA: No so basically all of these require minimal code changes to even be able to scale to, to long, like token counts, whether it's audio, video, image, or text. Text is. Generally, like the shortest token count, if you look at something like RefinedWeb or SlimPajama the, the average token count of a piece of text in that is only anywhere from 300 to 500 tokens. [00:23:02] NA: So this is actually generally a data centric issue too, when you're talking about long context with even training a standard natural language processing model. The thing about audio and video is, is these have a ton of tokens in them. And the one good thing, and then? the final note, I'm, I'm going to put in, unfortunately, before I have to head out, I know this was a lot of information. [00:23:22] NA: I can link these [00:23:24] Alex Volkov: Yeah, we're gonna add some, some of this, we're gonna add some, some links, the links that I'd be able to find, Enrique, if you can send [00:23:29] NA: Yeah, I'll, I'll send you all the research papers. [00:23:32] Alex Volkov: Yeah, you want to lend one last thing before we move on? Yeah, go ahead. [00:23:36] NA: Yeah, So, just the last thing on January 13th is there was this paper called Extending LLM's Context Window with only a hundred samples and they were essentially able to show that even in a very limited amount of long context samples, you're able to massively improve the context lengths of these models. I should mention these are the papers that I found did pretty rigorous evaluation overall, because a lot of them, there's a huge problem in long context evaluation. But I feel these authors generally applied their knowledge pretty well, and these results are really impactful. so, even for the open source community, because you don't need a lot of computational power to be able to scale these context windows massively now. [00:24:24] NA: And [00:24:24] NA: that's basically everything I wanted to [00:24:26] NA: say. [00:24:27] Alex Volkov: Thank you, Enrico. Thank you, folks. Folks, definitely give Enrico a follow. And we have quite a few conversations with Enrico. If somebody in the open source community knows about Long Contacts, Enrico is that guy. And we're definitely going to follow up with the links in the show notes for a bunch of this research. [00:24:41] Alex Volkov: And I think just to sum up, Enrico There have been breakthroughs, and it doesn't look like Google is the only folks who come up today. Nat Nat Friedman and Daniel Gross, the guys who have AI grant, they have the Vesuvius Challenge recently, and invest in everything AI possibly. They just announced an investment in magic, that they have a hundred million dollars investment, [00:25:00] quote unquote. [00:25:00] Alex Volkov: We were so impressed with these guys when we decided to give them a hundred million dollars from Nat Friedman, and they also talk about the model that does. Something like 10 million context windows. Swyx, you wanna, you wanna talk about the magic thing? [00:25:12] Swyx: They first talked about this last year, like six months ago, and then went completely silent. So we didn't really know what was going on with them. So it's good to see that this is at least real because six months ago they were talking about 5 million token context model. [00:25:28] Swyx: But no, nothing was demoed. Not even like a little teaser graphic or anything like that. But for Nat to have invested in this amount, I think it's a huge vote of confidence. And it basically promises that you can do proper codebase embedding and reasoning over an entire codebase. Which, it's funny to have a code model that specially does this, because Gemini could also potentially do this. [00:25:58] Alex Volkov: They showed in their examples 3JS. Did you see this? [00:26:01] Swyx: No, I didn't see the 3JS, but okay, yeah. And we have a pretty consistent result from what we've seen so far that GPT 4 is simultaneously the best LLM, but also the best code model. There's a lot of open source code models, CodeLlama, DeepSeaCoder, all these things. [00:26:18] Swyx: They're not as good as GPT So I think there's a general intelligence lesson to be learned here. That it remains to be seen because we, Magic did not release any other details today. Whether or not it can actually do better than just a general purpose Gemini. [00:26:34] Alex Volkov: Yeah, and so the example that they showed is actually they took 3JS, if you folks know the 3JS library from Mr. [00:26:40] Alex Volkov: Doob and they, embedded all of this in the context window and then asked questions and it was able to understand all of it Including, finding incredibly huge codebase. And I think I want to just move this conversation. [00:26:52] Alex Volkov: Yeah, Nisten, go ahead. I see you, I see you unmuting. And folks on the stage, feel free to raise your hands if if you want to chime in. We'll hopefully get to some of you, but we have a bunch of stuff to chat about as well. [00:27:01] Nisten Tahiraj: I'll just quickly say that there are still some drawbacks to these systems. And by systems the long context models where you dump in a whole code base or entire components in. And the drawbacks, even from the demos, still seem to be that. Yes, now they do look like they're much better at reading and intaking the information, but they're not yet much better at outputting similar length output, so they're still gonna only output, I think, up to 8, 000 tokens or so, and I don't know if that's that's a byproduct of of the training, or they could be trained to re output much longer, much longer context. [00:27:43] Nisten Tahiraj: However, the benefit now is that unlike Retrieval augmentation system, unlike a RAG the, the drawback with a RAG was that yes, it could search over the document, but it would only find maybe two or three or a couple of points and bring them up. Whereas this one is more holistic understanding of the, of the entire input that you've dumped in. [00:28:03] Nisten Tahiraj: But again, we're not quite there yet where they can just output a whole textbook. That's, that's what I mean. So that's the thing. That's the next challenge [00:28:11] Far El: to solve. [00:28:12] Alex Volkov: So I think, I think the, the immediate reaction that I had is very similar to what you had, Nisten. RAG is something everybody uses right now. And we've talked about long context versus, versus something like a RAG before, and the usual conversation we have is usually about cost. How much does it cost you pair these tokens, right? [00:28:30] Alex Volkov: If you send 10 million tokens and each token is like a cent, you're basically paying 10 million cents for every back and forth. Also speed and, and user experience. If your users are sitting there and waiting for 45, 60 seconds because they sent a bunch of contacts, if you can solve this with RAG, then RAG is probably a better approach for you. [00:28:48] Alex Volkov: However, however this specifically looks like. At least from the examples that the Google did, they showed the video transparently, they sped up the inference, but I saw something where with at least the video question, it took them around 40 seconds. to extract a frame of a video of an hour. They sent an hour worth of context of a video within this thing, and it took them 40 seconds for this inference. [00:29:13] Alex Volkov: Folks, like I said before, and I'm going to say this again, regular ChatGPT, not even crazy context, queries took me sometimes 40 seconds. Now, you may say, okay, Alex they show the demo of their environment, and ChatGPT is in production environment. Yes, but the possibility is, if I can send I don't know, 500, 000 tokens in the context window, and then within 40 seconds get a response which is equivalent to what I get from GPT 4. [00:29:38] Alex Volkov: Then I think that a bunch of the conversation about RAG being better just from a speed of inference perspective are slowing down. An additional thing I want to say before I get to you, Yam, just a second the immediate response in my head was, okay, RAG is done for, or at least not done for, but definitely the kind of the crown on RAG's head. [00:29:56] Alex Volkov: Everybody's talking about RAG. There's vector databases everywhere. We just had folks talk about Colbert and different things. RAG is, okay, RAG is now shaky. But the other thing I started to think is, is fine tuning. also under risk. And Swyx, I think this goes back to what you just said about like the general models versus the maybe the Finetune or very specific models, because if a general model can take a whole book, and they had an example about this where there was a very low resource language, Kalamathi, Kalabathi, something like this, and there's only one book that's a dictionary for this language, they literally threw the book in the context window, and the model was able to, from context learning, to generalize and understand this and perform better than fine tuned models. [00:30:37] Alex Volkov: And I'm thinking here okay, rag is the first thing to go. Is fine tuned second? Are we going to stop fine tuning and sending contexts? So Swyx, I want to hear your reaction about, about the language thing and then we're going to get to Yam and then we're going to ask some more folks. [00:30:48] Discussion about effects of longer context windows [00:30:48] Swyx: Yeah, I think there's generalizable insights about learning about language. And it's not surprising that throwing that into the context window works, especially if it's a cognate language of something that it already knows. So then you're just learning substitutions, and don't forget that transformers are initially trained to do language translation, like this is like bread and butter stuff for transformers. [00:31:12] Swyx: The second thing I would respond to is, I have to keep saying and banging this drum, long context does not kill RAG because of cost. Imagine if every time you throw 10 million tokens of context in there, you have to pay like a thousand dollars. Because unless something fundamentally is very, very different about this paradigm, you still pay to ingest those tokens of cost. [00:31:39] Swyx: So ultimately, people want to still reg for cost and then for attribution reasons, like debuggability attribution, which is something that's still valuable. So I think long context is something that I have historically quite underweighted for this reasons. I'm looking to change those assumptions, of course, because obviously this is magical capabilities if you can use [00:32:03] Alex Volkov: this is magical capabilities if you can use [00:32:10] Far El: Yeah, I just want to say on the topic of of latency and ingesting a lot of context. I think that there is a solution that we didn't talk about it here and will be something that is going to be incorporated in all the flagship models, which is embedding embedding knowledge into the KB cache, which is something that many of the inference engines today can do. [00:32:34] Far El: And you simply just prefix the context beforehand, and then you don't need to process it through your model. So you're not sending the whole database each time you are calling your model. It's just saved. Imagine that OpenAI have some sort of API that you embed. The KD cache beforehand, and it's reduced price, of course, and then it uses that as, as your context. [00:32:59] Far El: Basically, somewhere in the middle between the two. And the reason that it's not supported now in flagship models, because the first flagship model that supports a million tokens came out today. But I think that if we see this this, if we go there, this is something that we're going to see in all of the APIs. [00:33:18] Far El: Moreover, I also don't [00:33:20] think that RUG is done for it because RUG is explaining to you very, very clearly and very simply. Where the information is coming from, what the model is basing itself on. You can claim that the model with the attention you can do it as well, but it's not like RUG. RUG, you're just showing the clients, the people, exactly where it comes from. [00:33:40] Far El: And there are use cases where this is absolutely a must. So I think that there will always be room for RUG for these specific use [00:33:49] NA: cases and long [00:33:50] Far El: context. With KVCaching is going to be, I think, I think the methods for embedding, for example, a full database, or a book, or something big, and using it multiple times, with many different [00:34:05] Far El: prompts. [00:34:06] Alex Volkov: Or also multimodality, right? So thank you for this. Definitely, definitely makes sense. And I think somebody in the comment also left a similar comment as well. So we want to dive into the KVCache stuff maybe in the next one. But I want to talk about the multimodality part of this because, um We've, we've multiple times mentioned. [00:34:25] Alex Volkov: I think we did this every Thursday. I sense GPT 4 launched because we were waiting for the vision part of GPT 4. And we've talked about 2024 being the year of multimodal. And we're going to have to talk about a bunch of multimodal stuff today, specifically with the RECA folks and the RECA flash, which understands videos. [00:34:40] Alex Volkov: They basically, so I'm going to have to see whether RECA understands videos better than Gemini, but the Gemini folks talked about there's a specifically. A bunch of multi model effect on the context window where if you send videos, you, at least the way they did this was just frames. They broke down this movie to a bunch of 500, 000 frames or so and just sent it in context window. [00:35:04] Alex Volkov: And they basically said we have all this video in the context window and then we have a little bit of text. And I think context window expansions like this will just allow for incredibly multi modal use cases, not only video, audio, they talked about, we've talked about previously with the folks from [00:35:20] Alex Volkov: Prophetic about different fMRI and EEG signals that they're getting like multi modal like applications as well and Context window enlargement for these things, Google specifically highlighted. [00:35:32] Alex Volkov: And I want to highlight this as well because it's definitely coming. I'm waiting for being able to live stream video, for example. And I know some folks from like 12 Labs are talking about almost live live stream embedding. So definitely multimodal from Google. I think, folks, we've been at this for 30 minutes. [00:35:48] Andrej Karpathy leaves OpenAI [00:35:48] Alex Volkov: Alright, so folks, I think we're going to move on and talk about the next kind of a couple of stuff that we've already covered to an extent, but there's some news from OpenAI, specifically around Andrej Karpathy leaving, and this was announced, I think broke in the information, and Karpathy, some folks here call them senpai, Karpathy is a very Very legit, I don't know, top 10, top 5, whatever, researchers, and could potentially have been listening to the space that we had with LDJ after he left, or, yeah, I think it says, it was clear that he left it was the information kind of announcement didn't have a bunch of stuff, but then Andrei just As, as a transparent dude himself, he came and said, hey, this wasn't the reaction to anything specific that happened because speculations were flying. [00:36:33] Alex Volkov: And I think at least, at least to some extent, we were in charge of some of these speculations because we did a whole space about this that he could have just listened to. But as speculation was flying, maybe this was ILLIA related, maybe this was open source related, like all of these things. [00:36:46] Alex Volkov: Andre basically Helped start OpenAI, then left and helped kickstart the Tesla Autopilot program, scaled that to 1500, then left. On the chat with Lex Friedman, Andrei said that Basically, he wanted to go back to hands on coding, and in OpenAI, his bio at least said that he's working on a kind of Jarvis within OpenAI, and definitely Andrei has been also talking about the AI as an OS, Swyx, you wanna, you wanna cover like his OS approach? [00:37:14] Alex Volkov: I think you talked about this. He had a whole outline, I think you [00:37:17] Swyx: also [00:37:17] Swyx: talked about this. LLM OS. [00:37:18] Swyx: Yeah. He wasn't working on it so much as thinking about it. [00:37:21] Swyx: Thinking about it, [00:37:21] Swyx: yeah. And maybe now that he's independent, he might think about it. The main thing I will offer as actual alpha rather than speculation is I did speak to friends at OpenAI who reassured us that it really was nothing negative at OpenAI when he left. [00:37:40] Swyx: Apparently because they spoke to him before he left. [00:37:43] Swyx: So yeah, he's for the way I described it is he's following his own internal North Star and every time he does that the rest of us [00:37:51] Alex Volkov: And definitely the rest of us win. [00:37:53] Alex Volkov: the open source community is hoping, or I've seen many, many multiple things that say, hey, Andre will unite like the, the, the bands of open source, the different bands of open source. [00:38:02] Alex Volkov: Andre posted this thing. on his ex, where like his calendar was just free, which shows maybe part of the rationale why he left, because meetings and meetings and meetings and everything and now he can actually work. So shout out to Andrej Karpathy for all he did in OpenAI and for all he's going to continue to do. [00:38:16] Alex Volkov: We're going to definitely keep up to date with the stuff that he releases. Andrej, if you're listening to this, you're more than welcome to join. We're here on every Thursday. You don't have to have a calendar meeting for this. You can hop on the space and just join. Also on the topic of OpenAI, they've added memory to ChatGPT, which is super cool. [00:38:31] Alex Volkov: They released a teaser, this, I didn't get into the beta, so they released it to a limited amount of people. They added memory to ChatGPT, and memory is very, very cool, the way they added this as well. So I've said for a long time that 2024 is not only about multimodality, that's obviously going to come, but also it's about time we have personalization. [00:38:51] Alex Volkov: I'm getting tired of opening a ChatGPT. Chat, and have to remember to say the same things on, it doesn't remember the stuff that previously said. The folks in OpenAI are working on the differentiator, the moat, and different other things, especially now where Google is coming after them with the 10 million context window tokens. [00:39:08] Alex Volkov: And, they're now adding memory, where ChatGPT itself, like the model, will manage memory for you, and will try to figure out, oh, OpenAI, oh my god, breaking news. OpenAI just shared something. As I'm talking about them, you guys want to see this? Literally, I got a [00:39:28] Alex Volkov: notification from OpenAI as I'm talking about this. [00:39:30] Swyx: What? [00:39:32] Alex Volkov: Let's look at this. I, dude, I needed my, my breaking news button today. Opening, I said, introducing Sora, our text to video model. Sora can create videos for up to 60 seconds. [00:39:44] Alex Volkov: Holy s**t, this looks incredible. Oh my god, somebody please pin this to the, to the, Nisten, you have to see, there's a video, 60 second video, folks. [00:39:54] Alex Volkov: Like, all of the, oh my god, breaking, I have to put the breaking news button here, holy s**t. So folks, just to describe what I'm seeing, cause somebody please pin this to the top of the space every video model we had so far, every video model that we had so far does 3 to 4 seconds, Pica the other labs, I forgot their name now, Runway, all of these models, [00:40:16] Swyx: they [00:40:16] Swyx: do [00:40:16] Swyx: Oh my god, Runway. [00:40:18] Alex Volkov: They [00:40:18] Alex Volkov: do three to five seconds and it looks like wonky, this thing just that they show generates a 60 second featuring highly detailed scenes and the video that they've shared, I'm going to repost and somebody already put it up on space has folks walking hand in hand throughout a There's a zoomed in, like behind the scenes camera zooming in. [00:40:39] Alex Volkov: There's a couple Consistent I cannot believe this is January. Holy s**t The consistency is crazy. Nothing changes. You know how like previously video would jump frames and faces and things would shift [00:40:52] Alex Volkov: Wow, okay, so I guess we should probably talk about this. Reactions from folks. I saw LDJ wanted to come up to see the reaction I'm [00:41:00] Far El: just wild. Honestly, it looks crazy. It looks really good quality. Better than most text to video models that I've seen. [00:41:08] Alex Volkov: Holy s**t okay, so I'm scrolling through the page, folks, [00:41:13] Alex Volkov: those who are listening, openai. com slash Sora, Sora is their like text to video I'm seeing a video of a model walking through like a Japan street, whatever, the prompt is, a stylish woman walks down a Tokyo street filled with warm glowing neon animated city signage, she wears a black leather jacket, long red dress, and black boots, and the consistency here is insane. [00:41:35] Alex Volkov: I do [00:41:35] Far El: out the mammoths. Or actually go on their websites. On the Sora, [00:41:40] on OpenAI's website. They've got a [00:41:42] Far El: few examples. It's crazy. It's crazy. I've [00:41:45] Far El: never seen a [00:41:48] Alex Volkov: the if you showed me this yesterday, Far El, if you showed me this yesterday and said this is generated, I would not believe you. So what happens is, now the same video of this woman walking, they have a video camera zooming in, into her eyeglasses, her face stays the same, the same consistency, you can see reflection in the, in the sunglasses. [00:42:08] Far El: Alex, you have to go on the website. There's like this video of, oh like literally the prop is reflections in the window of a train traveling through the Tokyo suburbs. And [00:42:19] Far El: honestly, it looks, it looks like someone captured this no way this is AI [00:42:23] Far El: generated. It's, it's crazy [00:42:27] Alex Volkov: Wow, [00:42:27] Alex Volkov: folks. What's the availability of this? Let's, let's see, what do we know? So we know safety. We'll be taking several important safety steps ahead of making SORA available on OpenAI's products, so it's not available yet. Working with Red Teamers, they don't want this to be used in deepfakes for porn, obviously. [00:42:43] Alex Volkov: That's like the first thing that the waifus are going to use it for. The C2PA metadata that, if you guys remember, we've talked about that they started including in DALI, they're going to probably include this as well. And new techniques prepared for deployment, leveraging the existing safety methods. [00:42:56] Alex Volkov: Okay research techniques. [00:42:58] Far El: Crazy. [00:43:00] Alex Volkov: Consistency is crazy, right folks? [00:43:02] Swyx: Yeah, it's not available it looks like. [00:43:03] Swyx: Not available [00:43:04] Swyx: yet. [00:43:04] Swyx: To answer your question. They released some details about it being a diffusion model. They also talked about it having links to DALI 3 in the sense that Honestly, I don't know if people know that there was a DALI 3 paper, which is very, very rare in this age of Not close. [00:43:22] Swyx: Not open ai. [00:43:23] Alex Volkov: Yeah, not [00:43:24] Swyx: open AI. [00:43:24] Swyx: And so they doing this like synthetic data captioning thing for the DO three model and they're referencing the same method for soa. I would just go read the Dolly three paper [00:43:37] Alex Volkov: Wow. I, I, the consistency has been the biggest kind of problem with these LDJ. [00:43:41] Alex Volkov: Go ahead, please. As I'm reading this and reacting and, and my mind is literally blown the demo of the doggy. Hold on nj one second. There's a demo. There's a video of the dog, like walking from one window and jumping to another window and the pause, they look like it's a video, like folks like literally does not look like generated, like anything we've seen before. [00:44:02] Far El: This, is going to disrupt Hollywood immediately we're talking about, text to video disrupting media content creation and so on this is it, this is like the mid journey moment of, of text to video that same feeling that we had when we were able to crop mid journey and get some really high quality images this is the same but for video, essentially. [00:44:23] Alex Volkov: This, this breaks reality for me right now. Literally I'm watching this video multiple times. I cannot believe that the dog's paws are not shaping in different shapes. The spots on this Dalmatian dog stay in the same place throughout the video. It, it don't make sense. Alright, LDJ, go. I think, I think, [00:44:37] Far El: Yeah so [00:44:38] Far El: Sam here, I'll post it on the, on the ding board. Sam said that that certain select creators have access now. And, oh, I just lost the tweet. I'll, I'll get it. But yeah, he says that some creators already have access and I guess they're going to slowly expand it out to like beta users or whatever. [00:44:59] Alex Volkov: Wow, so Sam asked for some we can show you what Sora can do. Please reply with captions for videos you'd like to see and we'll start making some. [00:45:06] Alex Volkov: So [00:45:06] Swyx: Oh yeah, basically give him some really complicated prompt, and let's, let's go, let's go. [00:45:12] Alex Volkov: A bunch of podcasters sitting, watching Sora and reacting in real time and their heads are blown. [00:45:17] Alex Volkov: Not literally, because this is insane. How's that for a prompt? I'm gonna post it. Hopefully some will get it. [00:45:25] NA: Just opening a portal through Twitter, through OpenAI to the Munich and then string [00:45:31] Alex Volkov: Oh, there's, there's also, I don't wanna spend the rest of Thursday. 'cause we still have a bunch of talk about folks. [00:45:38] Alex Volkov: Is anybody not scrolling through examples right now? And you definitely should. There's an example of a [00:45:43] Swyx: there's only nine examples. [00:45:45] Alex Volkov: What, what [00:45:45] Far El: This is insane. [00:45:46] Alex Volkov: The whole, no website has a bunch of, scroll down. [00:45:48] Alex Volkov: There's like every, every kind of example has [00:45:51] Alex Volkov: more scrollies. So I'm looking at an example of a chameleon, which, has a bunch of spots and has guys, the spots are in the same place. What the f**k? It doesn't move. it does not look like honestly, let's do this. Everybody send this to your mom and say, Hey mom, is this AI generator? [00:46:07] Alex Volkov: Or not? Like older folks will not believe this s**t, like [00:46:10] Swyx: I, I will [00:46:13] Far El: What's the most impressive [00:46:14] Swyx: compare this to Google [00:46:15] Far El: right? Like humans, [00:46:17] Swyx: don't know, I think you guys [00:46:18] Alex Volkov: hold on. Pharrell, I think, I think we're talking over each other. Give us a one sec. Swix and then Farrell. [00:46:22] Swyx: Oh, sorry, yeah, there's a bit of a lag. Oh, no, nothing. Just compare this to Google Lumiere where they release a bunch of sample videos as well. [00:46:29] Swyx: But you could, the, the, I was impressed by the consistency of the Lumiere demo videos. They would, they demoed sort of pouring syrup onto a pancake and then infilling the syrup and showing that, it would be pretty realistic in pouring all that syrup stuff. Didn't really see that kind of very technical test here. [00:46:49] Swyx: But the resolution of these videos and the consistency of some of these movements between frames, and the ability to cut from scene to scene is way better. Instantly way better. I was thinking that Lumiere was, like, state of the art a few weeks ago, and now it is completely replaced by Sora. [00:47:08] Swyx: This is a way better demo. I think OpenAI is showing Google how to ship. [00:47:12] Alex Volkov: eye. Decided to say, you know what, Google, you think you can one up us with the context window? [00:47:18] Alex Volkov: We got another thing coming, because I've [00:47:20] Swyx: just pull up the Lumiere page, and then pull up the Sora page, and just look at them side by side, and you can see how much better they [00:47:26] Alex Volkov: Lumiere [00:47:26] Alex Volkov: was mind blowing as well. Go ahead, Far El. Go ahead, because we're still reacting in real time to this whole ridiculously impressive. [00:47:32] Far El: Yeah, I was just saying that the the most impressive thing are, is like how alive these video shots feel, right? Humans talking action scenes like, all the text to video models that I've seen so far and I've used were very very simplistic, right? It felt like more like you're animating an image to do very minor movements. [00:47:55] Far El: It wasn't actually alive in any way, but Sora's text to videos is, is nuts, the quality, the consistency, the action, like the actual action of the characters. I wonder how much like granular control do you have on a scene to scene basis. I know that Google released like a paper I think a few months back where they had a basically like a script that allowed the, like for much more long form. [00:48:27] Far El: video content, but I'm not sure if that's the case here. It's just, it's just really impressive. It's, it's really impressive. [00:48:35] Alex Volkov: I want to say one of our friends, LaChanze, just sent, at the bottom of the page, it says, Sora serves as a foundation model that can understand and simulate the real world. I can it's really hard for me to even internalize what I'm reading right now, because the simulation of the real world, it triggers something in me, tingles the simulation hypothesis type of thing, and this can regenerate the map of the world and then zoom in and then generate all the videos. [00:48:58] Alex Volkov: And I'm wearing this Mixed, slash, augmented, slash, spatial reality headset that just generates and this happens on the fly, and what am I actually watching here? So this says Sura serves as a foundation for models that can understand and simulate the real world, a capability we believe will be an important milestone for achieving AGI. [00:49:15] Alex Volkov: Yeah. Alright, folks. I will say, let's do two more minutes, cause this is I can't believe we got both of them the same day today, holy s**t, we got 10 million contacts window from Google announcement, which is incredible, multi modal as well, I like, my whole thing itches right now to take the videos that OpenAI generated and shove them into, into a Gemini to understand what it sees and see if if it understands, it probably will. [00:49:40] Alex Volkov: Wow. [00:49:40] Far El: Thing that would make this Thursday a tiny bit even more awesome is if Meta comes out with telemetry. Too much, too much, too much. [00:49:51] Alex Volkov: It's [00:49:51] Alex Volkov: gonna be too much. We need, we need a second to like breathe. Yeah, definitely folks. This is a Literally like singular day. Again, we've [00:50:00] had a few of those. We had one on March 14th when ThursdAI started, OpenAI released GPT 4, Entropic released Cloud, I think on the same day. We had another one when OpenAI Dev Day came about, and I think there's a bunch of other stuff. [00:50:12] Alex Volkov: I consider this to be another monumental day. We got Gemini 1. 5 with a potential 10 million context window, including incredible results in understanding multimodality in video, up to an hour of video. And then we also have some folks from RECA that's gonna come up soon and talk about their stuff, which is, they just with all due respect with RECA folks this news seems bigger, but they still launched something super, super cool we're gonna chat about, and now we're getting, it's just, the distance, we're used to jumps, we're used to state of the art every week, we're used to this, we're used to this model beats this model by Finetune, whatever, we're used to the OpenAI leaderboard, this is [00:50:53] Alex Volkov: such a [00:50:53] Alex Volkov: big jump on top of everything we saw. [00:50:55] Alex Volkov: From Stable Visual Diffusion. From what are they called again? I just said their name, Runway. I forgot their always forget their name. [00:51:02] Swyx: Poor guys. [00:51:04] Alex Volkov: Poor Runway. From Pica Labs. From folks who are generating videos. This is just such a huge jump in capability. They're talking about 60 seconds. [00:51:14] Alex Volkov: Oh, Meta just announced JEPA. Yeah, I don't know if JEPA is enough. People are commenting about JEPA, and I'm like, okay wait, hold [00:51:21] Swyx: You, you spiked my heart rate when you said Meta just announced. I was like, what the f**k? [00:51:25] Alex Volkov: the f**k? Meta literally just came out with an announcement, VJEPA, supervised learning for videos. [00:51:29] Alex Volkov: But, folks unless they come out with Lama 3 and it's multimodal and it's available right now, not Meta is not participating in the [00:51:35] Swyx: thing [00:51:36] Alex Volkov: day [00:51:36] Far El: Oh wait, this is actually cool. So this is this is something, [00:51:39] Far El: actually a paper they came out with like about a month ago, but this is for video understanding. So this is pretty much like for input of video, while OpenAI's model is for output of video. [00:51:51] Alex Volkov: It just, I will say it's a research thing, right? So they're not showing anything there, unless I'm mistaken. Um So, I kinda, so I still have a bunch of stuff to give you updates for, and I still have a bunch of interviews as well, there's a new stability model, but I'm still like, blown away, and I just wanna sit here and watch the videos, [00:52:07] Alex Volkov: Is this what Ilya saw? Yeah, somebody reacted like, what did Ilya see? Did Ilya see a generated video and the model understanding this and that's why, that's why? [00:52:16] Far El: No, I think, I think, I think AGI has been achieved internally at [00:52:21] Far El: this rate. [00:52:22] Alex Volkov: Wow. I, I'm, I'm still blown away. Like I, if a model can generate this level of detail in very soon, I just wanna play with this. I wish, I wish we had some time to, to, to, I, I was one of the artists and I hope that somebody in the audience here is, and that they will come to talk about this on Thursday. [00:52:43] Alex Volkov: I and because I'm, yeah. I'm still mind blown. So I see. Quite a few folks that I invited that I wanna, I wanna welcome to the stage. VJEP understands the world while Sora generates one. That's the comment that some folks led. And okay, okay. VJEP is going to be something we definitely cover because Meta released this and Meta are the GOATs, even though yeah, no, Meta's definitely GOATs. I'm just a little bit lost for words right now. [00:53:06] Nisten Tahiraj: Yeah, so if people have watched a lot of speeches from Yann LeCun is the, the main idea is that these AI models are not very good at understanding the world around them or thinking in 3D. So in some ways, you could reason out that A cat is a lot more intelligent even if it was blind and it couldn't smell, it could still figure out where to go and find its letterbox stuff like that. [00:53:30] Nisten Tahiraj: This is one part that's missing from the world model that they get purely just from word relationships or word vectors. And so this is a step in that direction, it seems. Again, I haven't read the paper, so I'm Half making stuff up here but it feels like this is a step in, in that direction towards AI models that understand what's going on like us and animals do. [00:53:56] Nisten Tahiraj: So that, that's the main, the gist of it for, the audience. [00:54:04] Alex Volkov: Oh, what, what a what A Thursday. What A Thursday. I gotta wonder how am I'm gonna summarize this, all of this. And I just wanna invite, we have here in the audience and I sent you a request to join. If you didn't get it. Make sure that you're looking at requests and then accept. And then we should have, we should have Max as well at some point. [00:54:20] Alex Volkov: Lemme text Max. 'cause we have guest speakers here from, from Breca that we wanna chat with. Meanwhile I'm gonna continue and, and move forward in some of the conversations. Let's roll back. Okay, while we're still super excited and I can't wait for this to come out, this is an announcement that they did. [00:54:35] Alex Volkov: It's very polished. We haven't seen we didn't see any access or anything about when it's going to come out. I do feel that this is a breakthrough moment. from Google and from OpenAI. And it does look like it's reactionary to an extent. The folks in OpenAI were sitting on this and saying, Hey, what's a good time to release this? [00:54:52] Alex Volkov: And, actually now, to let's steal some thunder from Google and they're like 10 million thing that also not many people can use. And let's show whatever we have that not many people can use which, which is an interesting. Think, to think about, because, again, the pressure is on a bunch of other labs, on Meta, to release something, we know Lama3 is coming at some point, will it be multi modal, will it be able to generate some stuff every [00:55:16] NA: Really, really quick, sorry to interrupt [00:55:18] Alex Volkov: Go [00:55:19] NA: the thing about VJEBA seems to be good at is understanding video instructions I guess you could point the camera to something you're doing with your hands and arts and crafts things, or repairing something, and it understands what you're doing, so that, that's actually very easy. [00:55:36] NA: Powerful for what data sets data sets of skills that will come, because then you can generate actions. I, I think that, that will apply a lot to robotics, what they're doing. [00:55:48] Alex Volkov: Oh, alright, yeah. And they also have the Ego4D datasets of robotics as well, and they've talked about this. [00:55:55] Nvidia relases chat with RTX [00:55:55] Alex Volkov: so let's go to open source like super quick. NVIDIA released a chat with RTX for local models. And it's actually like very, very cool. So a few things about the chat with RTX. First of all, NVIDIA packed a few, a few models for you. It's 38 gigabytes or something download. And they, they have they have quite a few I think they have two models packed in there. [00:56:16] Alex Volkov: I wasn't sure which ones. And this, this is basically a, a package you download. I don't know if a doc or not. That runs on any desktop PC with RTX 30 or 40 series with at least 8 gigabytes of RAM. And it gives you a chatbot that's fully local. And we love talking about open source and local stuff as well. [00:56:33] Alex Volkov: And it Not only that, they give you a rag built in. So you can actually run this on some of the documents that you have. They also have something that runs through a YouTube. You can give it like a YouTube playlist or a video link, and it will it will have you talk to YouTube video. So it has built in rag, built in Tensor rt, LLM, which runs on their, on their stuff RTX acceleration and. [00:56:56] Alex Volkov: I think it's pretty cool, like it works only on the very specific types of devices, only for like gamers or folks who run these things but I think it's pretty cool that that folks are, that NVIDIA is releasing this. They also have something for developers as well to be able to build on top of this. [00:57:11] Alex Volkov: And I think the last thing I'll say about this is that it's a Gradio interface, which is really funny to me that people are shipping Gradio interfaces on production. It's super cool. [00:57:18] Cohere releases Aya 101 12.8B LLM with 101 language understanding [00:57:18] Alex Volkov: Cohere releases an open source called AYA 101, a model that's like 12. 8 billion parameters model with understanding of multilingual 101 languages from Cohere. It's, it's honestly pretty cool because Cohere has been done doing a bunch of stuff. AYA outperforms the Bloom's model and MT0 on wide, a variety of automatic evaluations despite covering double the number of languages. [00:57:41] Alex Volkov: And what's interesting as well, they released a dataset together with AYA and then what is interesting here? Yeah, just, oh, Apache 2 license, which is super cool as well. Apache 2 license for, for this model. Let me invite Yi as a co host, maybe this can, join. Far El, go ahead. [00:57:58] Alex Volkov: Did you see, do you want to talk about Yi Aya? [00:58:00] Far El: Yeah first off, I I appreciate and commend Cohere to building a multilingual open source data set and so on. That's awesome. We need more of that. But unfortunately, With the first few questions that I asked in Arabic specifically most of the answers were complete. [00:58:20] nonsense on their train model. [00:58:23] Far El: Yeah. And to, to the point that it's it's laughable, right? For instance in Arabic, I asked who was the who was the first nation that [00:58:32] NA: had astronauts on the moon. I [00:58:38] Alex Volkov: Yes. [00:58:39] NA: think, I think you cut out for a sec. [00:58:43] Alex Volkov: I think he dropped. I don't see him anymore. [00:58:45] NA: He might have [00:58:46] NA: His phone might have [00:58:47] Alex Volkov: yeah, we're gonna have to [00:58:48] NA: I can briefly [00:58:50] NA: comment on it. Yeah, we're pretty happy now that also Kahira has started contributing, [00:58:56] NA: To open source because datasets are very important. And yeah, I think the reason it wasn't performing so well In other languages, it's just because some languages do not have there wasn't enough data in that for it to be, to be trained. [00:59:12] NA: But the beautiful thing is that it is Apache 2. 0. You can just add your own languages data set and it will. Literally, make the whole thing better. And yeah, that's, those are my comments on it. [00:59:22] Interview with Yi Tay and Max Baine from Reka AI [00:59:22] Alex Volkov: Awesome. All right, folks. So now we're moving into the interview stage, and we have quite a few folks. As one of the most favorite things that I want to do in ThursdAI, and it's been an hour since we've been here, is to actually talk with the folks who released the stuff that we're talking about. [00:59:35] Alex Volkov: So the next thing I'm going to announce, and then we're going to talk with Yitei and Max, and then after that, we're going to talk with Dom as well. Earlier this week, a company named Reka AI released two models, or at least released a demo of two models, right? I don't think API is still available. [00:59:51] Alex Volkov: We're going to talk about this as well. Called Reka Flash and Reka Edge. And Reka Flash and Reka Edge are both multimodal models that understand text, understand video, understand audio as well, which is like very surprising to me as well. And I had a thread where I just geeked out and my head was blown to the level of understanding of multimodality. [01:00:09] Alex Volkov: And I think some of the folks here had, had had talked about Sorry, let me reset. Some of the folks here on stage have worked on these multi models models. And so with this I want to introduce Yi Tei and Max Bain. Please feel free to unmute and introduce yourself briefly and then we're going to talk about some record stuff. [01:00:25] Alex Volkov: Yi first maybe and then Max. [01:00:27] Yi Tay: Yeah, thanks thanks Alex for inviting me here. Can people hear me actually? [01:00:31] Alex Volkov: Yeah, we can hear you [01:00:32] Yi Tay: okay, great, great. Because this is the first, hey this is the first time using space, so yeah, try to figure out how to use it. But thanks for the invite, alex, and so I'll just introduce myself. I'm Yi Teh, and I'm one of the co founders of RectorAI. [01:00:45] Yi Tay: We're like a new startup in the LMS space. We train multi modal models. Previously I worked at Google Brain working on Flan stuff like that. So yeah, that's just a short introduction about myself. And maybe Max, do you want to introduce yourself? Yeah, [01:00:59] Alex Volkov: Yeah, Max, go ahead, please. [01:01:00] Max Bain: thanks Ian. Yeah. [01:01:01] Max Bain: Thanks Alex for having me. So yeah, as you said yeah, I'm part of Wrecker. So I joined more recently, like six months ago. I just finished my PhD and that was all my video, audio, speech understanding. I've done a bit of work in open source. So if you use WhisperX that was like something I worked on and yeah, now working more on part of Wrecker and really enjoying it. [01:01:22] Max Bain: yeah, that's pretty much [01:01:23] Alex Volkov: First of all, let me just say, thank you for WhisperX, I did use this, and it was awesome, and I think this is how we connected before or at least, to some extent, I think this is the reason maybe I follow you, I was really surprised that you were Reka. Let's talk about the models that you guys just released, and because Very impressive on the multimodality part, but also very impressive on just the regular comparative benchmark, and I think you guys released the comparisons to just regular MMLU scores, so Wreck A Flash gets 73. [01:01:52] Alex Volkov: 5 on MMLU and 65 on Human EVAL, and GPT 4 is at 67, at least, and Gemini Ultra, they claim is 74, but your guy's model is like significantly smaller. What can you tell us about, and I know you said before there's like a bunch of stuff that you won't be able to talk about what can you tell us about the performance just on the textual kind of comparison, even though this is a multimodal model and there's a bunch more that we will talk about? [01:02:17] Yi Tay: Yeah, thanks so I'll just I can't really say that much, but I can say that there's quite a lot of headroom in pre training just for language alone, and I think that we're still not near the headroom yet for pre training, and I think even for us, actually, we have a better version of RecoFlash internally right now, but we've not even published metrics for that because while we were preparing for the launch we actually have even a better model now. [01:02:39] Yi Tay: So I think actually there's still quite a lot of headroom for pushing that and there's quite a lot of things to do in pre training but I can't really wouldn't be able to say much about? About like more details, yeah. [01:02:48] Alex Volkov: About specifics. I did see the comments that you left in your thread, that you talked about the folks who do foundational models from scratch, they, there's a lot of banging a lot of creation they have to do in the process as well, and it looks like at least some of this amount, some of this amount of hard work you guys had to go through in order to train these foundational models. [01:03:09] Alex Volkov: So let's talk about the multimodality, what what can this model do? And I think I have a [01:03:15] Alex Volkov: good idea, but can you talk to us on the multimodal part? What can those models do in terms of multimodality? [01:03:23] Max Bain: Yeah, so in terms of multimodal yeah, if you just, you can use it actually on chat. reco. ai, and I would say the image understanding's pretty good, so people have noticed, you can recognize text pretty well. Yeah, more nuanced details, which tended to be a big issue with VLMs, like they used to be quite biased or it'd hallucinate a lot. [01:03:41] Max Bain: I think in Rekka Fafri noticed that dropped a lot. So I think kind of image understanding is, I'd say, yeah, pretty on par with Gemini Pro or a bit better. But yeah, that's up to the jury. The video understands also pretty good. We limit it to a one minute input. We do have internally like better things and like bounded by how much we can run like for free. So, yeah, I'd say yeah, overall pretty good video understanding and image. We haven't focused too much on audio right now, but that's like definitely on the, on the roadmap. [01:04:14] Alex Volkov: I did run into the audio stuff, and I ran a few videos through the demo, and folks definitely should check out the demo. I'll add this in the show notes, and hopefully some folks will add this to the space as well. I just started uploading like short clips, and it's great to hear that you're saying, you guys are limited, you're limiting on the demo, but you can, if I'm hearing correctly, you can The model can understand longer videos as well. [01:04:39] Alex Volkov: So I uploaded a video of a trip that I took to Hawaii and there's a submarine there and somebody was narrating in the submarine and he yelled something like, there, there, there's the submarine goes, dive, dive, dive, something like this. Very excitedly. And the model really understood this, and actually it said, the commenter said, Dive, dive, dive, like this, with a bunch of I's in it. [01:05:00] Alex Volkov: And to me, this was like the, the holy s**t moment. I uploaded this video. The narrator for this video was very excited. I did not expect the model to actually pick up on the excitement. And, It was very surprising to me because if you use something like Whisper and you just extract the audio from the, from the video, you would not get this result. [01:05:20] Alex Volkov: You would not get like the, the excitement in this person's voice. And while we try to get max back in, could you, so could you mention stuff about audio? Do you train this specifically for audio as much as you can share, obviously. Or is it like a, a, a byproduct of, of just this model being multimodal and understanding and can listen as well? [01:05:39] Yi Tay: Wait, so let me take a step back. Actually, thanks for sharing that example because I [01:05:43] Yi Tay: actually had to watch your example to find that, that dive, dive, dive. I actually watched the entire video to find that, that clip. So I think it was a pretty Good clip. To be honest, it also surprised me that you found this example. [01:05:56] Yi Tay: I, I think I was not also expecting this but I, we, we, we co trained this with many modalities. We are not sure, like, why this this specific case is like this. I think that's all I can say, but probably [01:06:09] Yi Tay: yeah, next one [01:06:09] Alex Volkov: I can definitely, definitely add one thing that this video wasn't for sure not in your training data set because it was a private video of mine that didn't exist on the internet before. So it wasn't like a result of this video being in a training set. Max, you rejoined. I hope you heard some of this question as well, attributed to you. [01:06:26] Alex Volkov: Did you see this example? Did it cut you off guard as well? Do you see other examples like this that were like very, very surprising in how this model performs? [01:06:33] Max Bain: Yeah, I saw that. I was surprised. To be honest, one thing I've noticed is that video benchmarks are quite poor. So [01:06:40] we, in the question answering datasets, we don't really get a chance to see this, especially ones that use like the speech information and things like that. So I guess really, I'm glad you like tested it a lot. [01:06:50] Max Bain: Cause yeah, like internally we maybe haven't had a chance to I think but it's the benefit of kind of, yeah, training everything from scratch and adding all the modalities [01:06:58] Yi Tay: and yeah [01:06:58] Alex Volkov: That's awesome. So I also want to talk about the fact that you guys raised two models and you talked about there's a bigger one. Let's talk about the edge model. Can you talk about Are we going to be able to use this on device? I assume what's the play here? At least from what you can say, what's the play in terms of using the smaller models? [01:07:14] Alex Volkov: Obviously, smaller models, the benefit of them is using them closer on the edge and device, and that's how you named it. What's the, what's the thinking about releasing, these two models in different sizes? And and what's your plans for those? [01:07:26] Yi Tay: Oh yeah, sounds good. Yeah, that's a great question. So for the H model, 7B model, it's I think it's it's at a size that it's possible to run it locally, but we are thinking also along the lines of okay, it's actually Faster, like it's just for latency sensitive applications sometimes you just need certain things like this Slightly faster than the 21b model and it's also cheaper to to to host for for a lot of applications So I think that's mainly like this one of the reasons why seven. [01:07:55] Yi Tay: We also ran lots of ablations at low smaller scale. So this, this turns out to be just the size that we have. And I, I think it's mostly, mainly for latency sensitive stuff. And then like for people who are like for businesses and stuff, like they might just choose to deploy the smaller model if they don't like, need a larger models like the. [01:08:13] Yi Tay: Flash or the, the core model. So I think that's really like the idea behind it. And then from the research point of view, or at least from the playground point of view, right? Like the, the demo point of view is that people get to, to, to, to get a sense of the view of the model at the seven B scale and the 21 B scale, right? [01:08:28] Yi Tay: So there's kind some kind of you might be able to, to get a sense of like how this setup looks at the different scale. I think that's mainly like why we deployed two models in the background just so that people can play with. Two variants and the stuff. Actually not much thought here. [01:08:42] Yi Tay: I mean it's not like super complicated, it just happened this way, but yeah, that's all I can say, yeah. [01:08:48] Alex Volkov: Awesome. And so folks can go check out the demo. It looks like you guys are set up for API keys as far as I understood. So will developers be able, be, be able to build with this? What stage are you in? I think you, you invited to a disco or something. Could you talk about how we can play with these models, what we can do, and if there's any expected open source, because we'll have open source here on ThursdAI. [01:09:08] Alex Volkov: If there's anything to talk about there as well, please, please feel free to, to tell us how to actually try these models beyond the demo. Build with them. [01:09:16] Yi Tay: Yeah, sounds, sounds good. So for API, actually, we, we, we have our API as a system already like working and then some people are already using it. We are like rolling out access coupling without the billing and everything, like we're just making sure everything is running very well. [01:09:29] Yi Tay: And then we will roll it out soon. So I think that's mainly like the, the idea behind the slightly stitch. API release yeah, so that's for APIs. And then for open source, we I'll just be candid here, we are constantly, we're not sure yet about whether we want to do it or we don't want to do it. [01:09:44] Yi Tay: It's always a question we have but we're not promising anything, but we're also not saying no yet. So it's a, it's a competition we have very regularly about about this kind of thing. So I, I, so yeah, that's currently the stance we have right now. But we are, we are [01:09:55] Yi Tay: writing a we are writing a tech report it's not like a paper paper, but it's also not going to be that there'll, there'll be some details in the tech report, but not complete details, but some details. [01:10:04] Yi Tay: But yeah, so I think that's mainly like the extent of like how we're thinking about things right now, yeah. [01:10:09] Alex Volkov: Awesome. So first of all, I want to consider you guys friends of ThursdAI. Thanks for coming on the pod. And here, we definitely love open source. We talk about it all the time. And we're just like Champions of Open Source, so if you do release anything Open Source, you're welcome to come back as well. Yi and Max, we have Swyx here, I'm actually in Swyx's audience, so you can hear them from my microphone. [01:10:29] Alex Volkov: And Swyx has a few follow up questions for Yi and Max as well, so Swyx, go ahead. [01:10:32] Swyx: Oh, sure. Yeah. Hey I actually tried to set up a chat with you when I was in Singapore, but it didn't happen. [01:10:39] Swyx: So sorry about that. But I actually wanted to just chat with you more about something that you hinted on your announcement post. You talked about how much of the infra you had to rebuild, you Reka. Everything, you said everything from robust training infra. Proper Human Evaluation Pipelines and Proper RLHF Setups. [01:11:00] Swyx: I was wondering if you can just give us like a preview of What did you miss? What does Google have? And then what do you think like the industry could innovate on? [01:11:09] Yi Tay: Okay. That's a very interesting question. I need to be, need to think about what I can say and what I cannot say. But so definitely, definitely I miss GPUs credit to GPUs and being like a, a Googler for all my. Professional life, definitely the infra was completely new to me, and then at Rekka, we have a lot of people from GTM and, and Google in Alphabet in general I think a lot of us could, I feel the same way and then, I think in terms of infra, I think GPU tooling is not as robust as at least what I experienced for TPU Infra back at, at, at Google. So I think that's mainly the first thing is the robustness of the the, the training the, the, the, the, the, the accelerators itself, right? And then also even things like FileIO is something that people take for granted. At Google, the file systems, the X Manager box and stuff orchestrators and stuff like that are, like, just so well designed at Google. [01:12:02] Yi Tay: And then externally, it's a lot of them are just missing. So I think yeah, I, I, yeah, I think that's basically on the training infrasight and yeah, so I think, I think the tooling for like training like large models is not really super like robust externally, like you're, you're, it's not easy to like just pick off something and then like train like. [01:12:26] Yi Tay: Like a 100 bit model easily without actually making sure your checkpointing is you're, you're, you're resuming your checkpointing, your, your notes failing and stuff like that. I think those are, like, hard, hard stuff things that, that need to be taken care of but at, at, at Google some, some team Does that for you. [01:12:43] Yi Tay: Yeah, TLDR of the training infrastructure, yeah. [01:12:48] Swyx: Does Google have the equivalent of Weights and Biases? [01:12:51] Yi Tay: TensorBoard, I think, yeah. [01:12:53] Swyx: Oh yeah, yeah, yeah, of course. [01:12:55] Yi Tay: Yeah yeah, yeah, yeah yeah. [01:12:58] Alex Volkov: So [01:12:58] Alex Volkov: we don't work with Google yet, but hopefully if if folks at Google are listening to us and you want to use kind of Weights Biases, definitely reach out. But at least you guys, now that you're out of Google, you definitely can. You want to follow up with Swyx, or are you, [01:13:10] Swyx: are you Oh, [01:13:10] Swyx: I don't know. Did you guys talk about Ricoh Core already? [01:13:13] Alex Volkov: Yeah, so I think, Yi, there's not a lot of stuff that you can say about the bigger model that you guys have, but give us a little teaser live for a few folks here on stage, like what can we expect from the bigger model, maybe when, what can you tell us? [01:13:28] Yi Tay: So the bigger model, okay, so I can just say that we, we ourselves are quite impressed by the results and it's if, if if you try to extrapolate from our 7 and 21 based on relative to other models of the scale you can. Try to imagine like what the type of metrics look like, right? But I think we are, we ourselves are, ourselves, we are quite impressed by, by the, the, the, the, the metrics. [01:13:49] Yi Tay: So like we are I think that's all we can say. I think in the polls, we say that coming out in coming weeks is around that ballpark. It's not like next week, the kind of thing. It's also not like one, two weeks. It's probably like a couple of weeks. But we still, we also kind of like a bit tired after the release. [01:14:05] Yi Tay: Take [01:14:05] Yi Tay: a few days light break and then start working again, that kind of thing. So Yeah. I think that that's, that's basically what I can say, but it's, I, we are, we are very happy in the model and as well, yeah. [01:14:17] Alex Volkov: All right, so we're excited to see this. I want to flip back to Max just for a second. Max as we just talked covered, there's some stuff that I use that you guys are watching. Oh, find somebody test this out. When folks interact with your demo, first of all, I'll just say, definitely folks should do the thumbs up, thumbs down, and reply, so you guys will get some nice RLHF. [01:14:35] Alex Volkov: What other venues of giving you guys feedback would folks can go? Is there a Discord you want to call out, or anything else you want to add to this as we move on? [01:14:44] Max Bain: Yeah, thanks guys. We, we actually have a discord channel and if people post, use cases where maybe our model is doing well, or could do better, you can post that, or maybe there's something you're not happy with the current models, like GPT 4V also. And like, I guess, cause we're [01:15:00] such a small team in an early stage, like we'd. [01:15:02] Max Bain: We're taking a lot of that on board and yeah if you can point any of that stuff, if you have stuff in more detail, you can put that on the Discord and yeah, we're like, really happy for any feedback, [01:15:10] Alex Volkov: awesome. Are you guys distributed, by the way? Are you working co located? Like, where's, where's RECA located? [01:15:16] Max Bain: Like, all over the globe, yeah, So he's in Singapore, I'm, like London, sometimes the West Coast, but yeah, it's like a remote first [01:15:23] Max Bain: company. [01:15:25] Max Bain: and also, yeah, sorry. Another thing is if we have, do you have job posting? So if you guys would Yeah, like the sound of record, you can also apply to join. We have yeah, quite a few [01:15:35] Max Bain: positions open. [01:15:42] Alex Volkov: friends of the pod from now on. E, anything else you wanna, you wanna add as, as we finish up and then move to the next [01:15:49] Yi Tay: No, thanks. Yeah, really thanks for inviting. It's really nice chatting with you. And yeah, it's been great. Yeah. [01:15:56] Alex Volkov: I'm, I was, like, like I said, I was blown away by the performance of the multimodality. I was blown away by the tonality understanding, which I've never experienced in any model so far. I heard that it's possible and I saw some technical stuff. I never experienced this on something like my videos as well. [01:16:11] Alex Volkov: Definitely folks should play around with, with the demo. I'll add this in the show notes and follow Yi and Reka and, oh yeah, one last thing Yi, before you go. What's the meaning of Reka? I know this is a word in Hebrew that I know, but what's, what's the meaning of this word? Like, where, where did this come from? [01:16:24] Alex Volkov: I was really curious. [01:16:26] Yi Tay: I think one of the meanings, it's not official, it's not canon, but like one of the meaning it comes from Reka in Eureka, like Eureka, like the Reka [01:16:35] Yi Tay: in Eureka, but it's not Okay, this is not canon, it's just one of the interpretations of that but it's a bit reverse engineered where people ask us, we just, this is what we say, but that's actually I think that that's it's not really like canon, yeah. [01:16:49] Alex Volkov: Awesome. Thank you guys for joining and folks, definitely should go check out the demo. And I think the tradition continues because now we have we're moving on to the diffusion area and we have the, the, the, the awesome, the awesome chance to have Dome here. And we. Just released, or I guess we saw this week, a new release from Stable Diffusion called Stable Cascade. [01:17:09] Alex Volkov: And Dom, I reacted to Imad's tweet about this hey Imad, you want to come to ThursdAI? And he said, Dom, and I think did you say Rodrigo was the other guy? Are the real heroes. And I want to welcome Dom to the stage. Dom, welcome. Feel free to unmute yourself, give a brief introduction. Let's talk about, let's talk about Stable Cascade. . [01:17:25] Dome: So yeah, my, my name's Dom. I joined stability a couple, actually a couple of months only ago. And I'm currently enrolled in, in Germany in a in a degree. I'm currently finishing that up and I've met Pablo more than a year ago. And ever since that we started working on, generative models, mostly in vision. So image modality and also slowly moving into video stuff. And yeah, at some point, so pretty early, we already connected to stability via Lyon. And at some point they liked what we were doing and liked the progress of how the paper that we called Verstehen was going, which is German and means sausage. [01:18:09] Dome: I can tell more about that [01:18:10] Alex Volkov: Oh, that's what it means! Okay. [01:18:13] Dome: yeah, yeah, yeah. And yeah, so then we joined, we joined and we joined the apply team and we were able to, to work on the third version of it which in the end then was called Stable Cascade, just to make it fit in more, not to confuse people where that name comes from, what's this third version about. [01:18:31] Dome: And yeah. [01:18:34] Dome: That's bad. [01:18:34] Alex Volkov: Awesome. So let's, let's say hi to Pablo as well. Welcome, Pablo. Feel free to unmute yourself. Brief intro from you as well. And let's talk about what makes Cascade different than SDXL or even the V2. [01:18:45] Pablo: Hey, hi, Alex. A bit about myself. I am a machine learning researcher. I used to work before working at Stability. I used to work at Disney. So I was able to bring a lot of interesting ideas from there. And then I, yeah, I joined Dom and we have been working on very cool things since, since I met him. [01:19:03] Pablo: And the latest is, is our new stable cascade. [01:19:08] Alex Volkov: That's awesome. Let's talk about Stable Cascade. I've been able to test this out, and the things I was able to, the things that blew me away were, like, speed, inference speed as well, but also the base model already has hands built in, and they're fine. You guys said you're working with Worshen for a couple iterations, and this became Stable Cascade? [01:19:26] Alex Volkov: Like, where talk to me about the history, and why is it so good, and so fast? [01:19:30] Dome: Okay. Yeah. Yeah. So basically the, the biggest difference, and I think that's what it boils down eventually is the, the, the space or the dimension where stuff is generated for, for the text conditional part and for Stable Diffusion XL is, that they have this thing called the VAE, which takes images and just compresses it down to a smaller space. [01:19:53] Dome: And the only reason to do that is. Just that you work at a smaller resolution, which then gives you faster training and faster inference. Imagine training or generating stuff at a pixel resolution of 1024, so one megapixel. This will be a lot slower than if you try to do the same, try to trying the same model at what, 32 by 32, for example. [01:20:15] Dome: So the idea is you still want to have high, high quality, high resolution images, but you don't want to generate at that very high pixel space. So you just try to find something, how you can compress it even further. And up, up until now, people always use VAEs, VQGANs, normal autoencoders and so on but they reach limits very early on. [01:20:34] Dome: So you can get to an spatial compression of eight. So Pablo had this incredible idea of using it. diffusion model to increase that compression, basically, and long story short by using a diffusion model on top of a normal VAE, or you could also leave the VAE away and just start at pixel space, you can achieve much, much higher compressions because you have the diffusion model that can iteratively at first at the lower frequency, so the, the the rough details, and then later on at the high frequency. [01:21:04] Dome: So at all the details. And so it has just a lot more space to reconstruct an image. And with that it's possible to, to compress images a lot further. And the version that we have now achieves a compression of 42. And that makes a huge difference in terms of training and inference time. And That's probably what you saw because then [01:21:24] Dome: the big model, the 3. [01:21:26] Dome: 6 billion, which is. quite big for images. So stable diffusion XL is 2. 2 billion. We're not in the, in the large language models. So yeah, this makes it just a lot faster. And then you have this diffusion decoder, which works at at a higher resolution, but needs a lot less steps and combining this just gives results in making the model very fast. [01:21:49] Alex Volkov: That's super cool. I want to switch back to Pablo just real quick. So I'm looking at this graph for inference speed, but also checked out some of the examples. One thing that I noticed is the real time rendering basically of how the model kind of searches through the diffusion space. And the last step just like kicks into like super high resolution. [01:22:09] Alex Volkov: Pablo, what can you tell us from some exciting or maybe surprising results that you've seen or people using it and Yeah, feel free to speak about your cool model a little bit more. [01:22:18] Pablo: Yeah, I actually I have been really surprised on how well this model could, could could be. We, we, we're not expecting it to be as good as it is. We started this more as an like a, an experimental idea of trying to achieve the same quality of existing models but focusing on, on speed on performance. [01:22:39] Pablo: But then somehow we ended up with a model that was like very competitive and yeah, I don't know. I think this last step as, as you mentioned, is the the, the upsampling stage. Which is this diffusion model that Dominic mentioned that can bring the image from 24 by 24 latent to a one megapixel. [01:23:00] Pablo: And that's why you see this like very big difference between the previous to last and the last step. [01:23:06] Alex Volkov: Yeah, the last step is poof, high quality. I love it. [01:23:11] Dome: Yeah, we, we, yeah, we, we actually provided a previewer. So when we work in this very highly compressed latent space, In order to be able [01:23:20] to see what the model is doing, we have this very tiny convolutional model that can preview what's going on. That's what you're seeing, which looks pretty blurry. And then yeah, the final step does that. [01:23:33] Dome: And yeah, why the model can make We're also pretty surprised. The, the big [01:23:41] Alex Volkov: Text is also very impressive. I think let's not skip over this. The out of the box text. is so good. Compared to, let's say, the Stable Diffusion 1. 4, which it released was, which was bigger, right? I think it was like five gigabytes or something. This is just miles, miles, miles better. And the text out of the box, hands out of the box is very impressive. [01:23:59] Alex Volkov: Text is super cool as well. Very surprising. Yeah, go ahead, please. [01:24:02] Pablo: The, the, the biggest difference compared to V2, which was our previous iteration of the model was the size of the architecture of the model and the quality of the data, which I think. It shows how important that, that is, and I think probably, since, since our model is able to work on this very, very highly compressed space, it can learn much more efficiently if, if it has good data, it can learn much more efficiently these, these kind of things. [01:24:30] Pablo: Maybe it learns them faster than other models which is why Yeah, we're able to have this kind of results. [01:24:39] Alex Volkov: Awesome. Thank you guys for coming up. I really wanted to make sure that, yeah, you guys get the recognition because like really, really cool. This is under the stability membership, right? This is not like fully, fully open source, but folks are going to be able to use this model for, for their stuff and maybe keep training. [01:24:55] Alex Volkov: Does it support all of the, the, the fine tuning and the LoRa ecosystem as well? [01:24:59] Pablo: Yeah, one detail, it's not yet on the the subscription. It's still for only for research but it, it will change probably in, in the following weeks, you asked about the Loras and Control Nets. Yeah, we [01:25:13] Pablo: we [01:25:13] Pablo: we we made sure to provide some example code for training Loras, Control Nets, and the full, full fine tunings on, on our repository. We also provide some pre trained Control Nets for in painting, for canny edges for super resolution, which is not the best super resolution model out there, but it's, it's interesting enough to, to share with the community, and we provided Tiny Laura with Dom's dog which is, it's pretty and, [01:25:44] Alex Volkov: Nice. [01:25:45] Dome: yeah, and I think that's it for now, that, that's [01:25:48] Yi Tay: all the [01:25:49] Alex Volkov: Awesome. Thank you for joining and folks, definitely give Dom and Pablo a follow. Folks, really great shout out for building this and releasing this from Stability and it looks really good and I'm sure the community will adopt this. I've already seen a bunch of AI artists in my, in my kind of field. [01:26:02] Alex Volkov: field are getting very excited about the possibilities here. Thank you for your work and thank you for coming for Thursday. I please feel free to stay because we're going to cover a bunch of other stuff as well, like super quick. Meanwhile, I just want to do a quick reset. It's been an hour and let's say 35 minutes since we're here. [01:26:20] Alex Volkov: If you're just joining us, you're on the Thursday I X space, which is live recording for the Thursday I podcast and newsletter. I'm your host, [01:26:28] Alex Volkov: Alex Volkov, I'm here joined by a co host, Nisten is here on stage, Yamil Spokin, and we have Swyx here, who dropped off the stage, but he's in the microphone, and I will move towards a corner that I have, and then [01:26:40] This weeks Buzz [01:26:40] Alex Volkov: I have a surprise for Swyx I'm moving towards a corner that I have usually, which is called This Week's Buzz, where I talk about the stuff that we have, or I learn in Weights Biases every week, so if you are subscribed to the newsletter, you definitely already know this, I just learn as I go and talk about this. [01:26:55] Alex Volkov: If you're not subscribed to the newsletter, Why not? I guess you'll be up to date with everything that happens in the world of AI. So definitely check out thursdai. news. This is the URL, HTTPS, thursdai. news. And this week's buzz is all about this new course that we released with Hamil Hussain about putting models in production. [01:27:13] Alex Volkov: I think I've spoken about this before. Weights Biases has an academy. We release courses and the courses are free for you. There's a bunch of knowledge. The last one we've talked about was the, with Jason Liu about the instructor. And we also have Hamel Hussain who released a course about model management and in production as well. [01:27:29] Alex Volkov: And this is definitely A very illuminating one, including how to use weights and biases for the, like the best companies do, OpenAI does, and like Microsoft and Meta, and hopefully we'll get Google at some point. Definitely, of course, it's worth checking out and signing up for. This will be in the show notes as well, and I'll post the link as well here. [01:27:47] Interview with Swyx from Latent Space [01:27:47] Alex Volkov: And now I'm gonna Actually yeah, Swyx is now back on stage, and here's my surprise, if you guys follow and Swyx's voice, you know that he's a co host of Latentspace together with Alessio, and we're now sitting in the Latentspace pod studio, which looks incredible the surprise is, I don't remember you being on the other side of the mic, so this is like a surprise interview with Alex and Swyx, but you're gonna be a guest and not a host, and I just wanted to hear about some stuff that you guys are doing, and how Latentspace is going, like all these things. [01:28:14] Alex Volkov: So this turns from ThursdAI into ThursdAI, like deep dive interview, just a brief [01:28:18] Alex Volkov: one. [01:28:19] Alex Volkov: I figured I'd use the opportunity to give you a surprise. This was not staged. Swix told me he may not be able to even join. 'cause you just flew back from [01:28:26] Swyx: Singapore. Singapore, yeah. Yeah. [01:28:27] Swyx: Yeah. [01:28:28] Swyx: Cool, okay, [01:28:29] Alex Volkov: So as, [01:28:30] Swyx: I feel like we talk so much and you've been a guest on our pod like five times, so [01:28:35] Alex Volkov: and [01:28:36] Alex Volkov: I, I would wanna start with how you would introduce yourself to the audience that doesn't know you. [01:28:41] Swyx: you so I'm Swyx, I mostly work on developer tooling, and, and, mostly known as the editor or podcaster of Latent Space, which has done pretty well. [01:28:51] Swyx: I think we're celebrating our first year anniversary pretty soon. And on the the other half of my life is I'm working on small AI and AI Engineer Conference, which we just, which we just announced for June 25th to 27th. Yeah. [01:29:05] Alex Volkov: Yeah. You've had quite a long career in DX as well. I think Netlify, you had a stint in [01:29:09] Swyx: Netlify [01:29:09] Swyx: Yeah, I was one of their earliest employees slash dev rel of Netlify. That's where a lot of people know me. That's where I became quote unquote famous in developer tooling and in React specifically. Because I did a lot of content on React and serverless speaking and writing. And then I've been head of developer experience for Temporal, Airbyte, and then also spent a year at AWS working on the same thing. [01:29:34] Alex Volkov: Hmm. Awesome. I also from that kind of that side of your career, you work with the Chroma guys as well. [01:29:40] Alex Volkov: And Chroma [01:29:41] Alex Volkov: just announced that they have been a year around and looked like millions of companies that probably had [01:29:48] Alex Volkov: something to do with that. So shout out Jeff. And and, I'm blanking out on the [01:29:53] Swyx: name, Anton. Yeah, yeah. I so I consulted for them on their DevRel when they were doing their, their first hackathon a year ago, actually. And yeah, I [01:30:03] Alex Volkov: think [01:30:04] Swyx: It seems like they are the leaders in open source vector databases. Retool, we did a chat or interview with David Hsu, the founder of Retool, and Retool did a state of AI survey among their customers what they're using. [01:30:18] Swyx: And Chroma was, like, up and to the right in terms of the adoption and the NPS score, which I think NPS is actually a very important metric to keep tracking. Yeah. Really, really cool. Glad to be involved with Chroma. [01:30:30] Alex Volkov: Glad to be involved with Chroma. You've been also prolific in writing, like I know many people go to your blogs and like the stuff that you have, how many publications in total are you like, publishing your content in right now? [01:30:46] Alex Volkov: You have your own personal [01:30:47] Swyx: one, Yeah, I have three blogs. Three blogs. But Latentspace is the currently primary active blog. I have a personal one and then I have a developer tools advising one because I do a bunch of angel investing and advising for people. [01:31:01] Swyx: And I don't know. I think More people should blog! It helps you think through what you think that and share your knowledge with other people. [01:31:10] Swyx: And also, actually the most valuable thing is the most embarrassing thing, which is when you get things wrong. People will come out and correct you, and you will be embarrassed for a second, but then you'll remember the lesson forever. [01:31:21] Alex Volkov: Can you give me an example of something that you went wrong and people corrected you, and then this improved your thinking? [01:31:28] Swyx: improved thinking? [01:31:31] Swyx: Yesterday or into coming into today, right? Because I do a monthly recap where I think what ThursdAI does is [01:31:40] recap news every week and then other people like NLW from the breakdown recaps news every day. And I think the lower frequency granularity of a month means that I only get to do 12 of these a [01:31:53] Alex Volkov: year. [01:31:54] Swyx: And that. forces me to think through okay, what is really actually important when you step back and think about it. And for my January recap, January was a slow month, to be honest. Today was more news than January. So I was like, I was trying to recap January, and I was like, okay nothing super interesting this month. [01:32:11] Swyx: What Do we, if we step back, it's important for AI progress. And I listed a bunch of things, long inference and all that. One thing I specifically said was not interesting for state of the art models was long context. [01:32:26] Alex Volkov: was, long context. It [01:32:28] Swyx: I said that yesterday. It's published, I sent it out to 35, 000 people, including Satya Nadella, Drew Houston, and all the people who read the newsletter. [01:32:36] Alex Volkov: Satya doesn't read, he also participates, like he clicks on [01:32:39] Swyx: links, [01:32:39] Swyx: Yeah. [01:32:40] Alex Volkov: there's an engagement, active engagement from Satya from Lydian Space. [01:32:43] Swyx: so it's, so it's embarrassing, but also it just forces me to think about okay, how much do I really believe in million token and ten million token context? And I know now, today I learned that Nat Friedman strongly disagrees. [01:32:58] Swyx: And that's good. That's, that's useful to update. And Google, of course. Yeah, yeah. I think It's, it's a, basically, so it's not about that specific point because we can always debate the pros and cons of that, but the act of writing down what you believe and taking strong opinions instead of saying that everything is awesome, instead of celebrating every little bit of progress as equally important, you have to rank them, and being wrong in your rankings gives you information to update your rankings, and if you don't give yourself the chance to be wrong, then you don't really learn. [01:33:36] Alex Volkov: You [01:33:37] Alex Volkov: publish a bunch of stuff. Some of the stuff that you publish turns into more than just an article. You have essays, and I think that the one essay that I remember specifically, obviously, is about the AI engineer essay. Talk to me about thinking about how you approach writing this. Is that stuff that you saw? [01:33:51] Alex Volkov: And I think as background for folks who are not familiar with you and where you are in, in, you're sitting in the middle of the arena that you helped also coin in San Francisco, right? We're in the middle of Soma Mission, Hayes Valley, somewhere there, if I'm not confusing. We're in this space it's called Newton that you're also like I think you're plugging in latent space where Tons of companies that we know from the Twittersphere are just literally behind us here. [01:34:15] Alex Volkov: There's Tab with Avi and Julius with Rahul like like a bunch of other companies like sitting right here building like very cool things and And this is an example of one of those so actually I think it was very natural to put those kind of hubs within the bigger bubble of San Francisco. And you, as far as I'm concerned, it was very plugged in to this even before coming to AiEngineer, right? [01:34:34] Alex Volkov: And potentially, this is the reason why the engineer the conference had so many amazing speakers on stage because very I think you told me back then a lot of like personal favors were pulled to get some folks to show up on that on that. And As somebody who's an outsider from Denver, what I said, right? [01:34:48] Alex Volkov: This is, this is incredible to see, but also it's very hard to penetrate and understand like what's going on and where the trends are. And this is part of the reason for ThursdAI. So you're sitting in the middle of this, you have all these connections, you said you're an angel investor as well. How does this shape your thinking about the AI engineer? [01:35:02] Alex Volkov: Do these old people talk in like the hackathons? How do you draw to create something like this that's fairly seminal that now people are considering themselves AI [01:35:11] Swyx: engine. Okay. Oh. Okay. So there's, there's two questions here. [01:35:15] Swyx: If I can do rag on your questions. Yeah, please. Which is that one, how do you write impactful perspectives or come up with interesting ideas that will stick around? And two, how do you make sense of San Francisco? Especially as an outsider. And people, I think people can hear in my voice that I'm not American. [01:35:34] Swyx: I'm Singaporean. And the last seven years of my developer career, I did not spend in San Francisco. I only moved here in April of last year. You don't have to be an SF to have a background in tech. Oh, I think the other the other thing I should offer as context is that I, I have been blogging for quite a bit. [01:35:57] Swyx: I often say that you have to blog 50 times a year, but in order to get like one post a year that it, that makes up the entire year, it's the one that people know you for. So this is my sort of fourth or fifth Quote, unquote, industry defining blog posts. So I, I've done this for serverless, runtimes and cloud orchestration and AWS, so I've done this before and I knew the work that goes into writing something like this. Rise of the AI Engineer took two months. I had a few potential collaborators [01:36:35] Swyx: who ultimately did not co author but were heavily involved. [01:36:43] Swyx: And I can talk about the writing of the post, but the main inspiration is trying to figure out what is important directions. [01:36:48] Swyx: And it is not purely about coining a term, which I think is a very vanity metric, but it is about picking directions in terms of identifying what is wrong about the zeitgeist. At if you rewind this time one year ago, people were very much focusing on prompt engineering. People were worried about the end of jobs for AI, for, for engineers, for software engineers. [01:37:13] Swyx: And I think both have been proven wrong in terms of the scope of the prompt engineer. Now, like now you're no longer really here about. Professional prompt engineers, because it's been replaced by the AI engineer who can code. And I think the importance of the ability to code to wield AI makes you a thousand times more effective than people who use AI without the ability to code. [01:37:37] Swyx: And I think identifying this core difference in ability, understanding that this stack is starting pretty thin and small, but it's going to grow over time, understanding that it is fundamentally very different from the ML engineer stack is a part of the mix that made me convinced that AI engineer would be a category to invest in which is why I started the conference and then pivoted the newsletter and podcast. [01:38:04] Alex Volkov: Yeah, so let's talk about that as well. So definitely the audience that ThursdAI draws, at least in part, is AI engineers, but also in part, like folks who are trained in Finetune models. And I've noticed like a little bit of a AI engineering is almost like the gateway drug into the larger AI stuff, because at least the folks that I'm familiar with, the folks who are like JSTS devs, that did the Netlify stint, that did React, etc., [01:38:27] Alex Volkov: they started to build with these tools. The tools are like significantly easier to get into than ML, than traditional ML. You just do some API calls open AI exposes a bunch of stuff, and suddenly you're like, oh, okay. I have, I've tapped all this power, this incredible power. I'm building intuitions about how to use this power. [01:38:42] Alex Volkov: I'm building intuitions, how to put this power in production for my users. They tell me some feedback. How do I do more of this? Am I only limited to open ai? Or maybe I can go to the open source. Try some stuff like this. Maybe I can do Olama, which, by the way, shout out to Olama, our friends, just released the Windows thing. [01:38:56] Alex Volkov: Maybe I can do this like locally on device. Maybe you can do this on Edge, on Cloudflare, for example. All these new tools are popping up, and these people are sounding like from a very limited scope of API users, are growing into API users who also have an intuition about prompting is just one of those things, embedding in RAG and better RAG systems, like we've seen some folks going there. [01:39:14] Alex Volkov: Definitely the scope grows, and as every category, like frontend was a very tiny scope, JavaScript, HTML, and the client, and suddenly like it became a full stack, you have prompt and like frontend, ops, and like all of these like things. So scope grows. [01:39:30] Alex Volkov: Where do people learn about this new and upcoming thing? [01:39:32] Alex Volkov: And I think like the conference is one such way. So we've talked about the conference. This is actually not your first time. I just remembering I interviewed you after the conference for a full hour that we had a full conversation. It wasn't about Swyx. So how was the conference after the conference received? [01:39:46] Alex Volkov: How did your direction into thinking about latent space and kind of exposing AI in San Francisco to the world? And let's take this to the kind of the next conference where you want to take us. What happened to the AI engineer? [01:39:59] Alex Volkov: I think I asked [01:39:59] Swyx: three [01:39:59] Swyx: or [01:39:59] Swyx: four. [01:40:00] Yeah, I know. [01:40:00] Alex Volkov: Break them down however you want. [01:40:02] Swyx: So the conference was really good, but I would actually classify that as the end of a process rather than the start of a process. It basically recaps [01:40:10] Swyx: the work [01:40:11] Swyx: that people are doing in the industry over the past year. [01:40:14] Swyx: And then, I get to curate and pick and invite people to present, the best of their work and their thought. And I think that's a very privileged position. And then for me, The work begins after the conference for the next the next thing. And I picking directions and having so last year was like a single track conference, this year for World's Fair we're doing nine [01:40:36] Alex Volkov: When is that, just for the [01:40:38] Swyx: June 25th to 27th. Yeah. [01:40:40] Alex Volkov: make sure you sign up. [01:40:41] Alex Volkov: It's gonna [01:40:42] Swyx: yeah, yeah. We're going four times bigger this year, 2, 000 people, and last year, 17, 000 people tuned in on the livestream, and hopefully we'll have, we'll have more impact this year. But yeah I think For me, actually, it's a really good way to think about okay, who do people want to hear from, who actually did impactful work that I will be proud to showcase 10 years from now. [01:41:04] Swyx: I'm always thinking about the test of time. And I was very inspired by NeurIPS, where they actually had a test of time award. And I was like, [01:41:10] Alex Volkov: man, that's Did Jeremy Howard get it or something, if I remember [01:41:13] Alex Volkov: correctly? [01:41:13] Alex Volkov: No, Jeff Dean. Jeff Dean. [01:41:14] Swyx: Jeff Dean. Yeah. [01:41:16] Alex Volkov: Shoutout Jeff Dean for today, by the way. [01:41:17] Swyx: Yeah, yeah, for Word2Vec. I, I always said some people are speculating what is Test of Time for next year, and it was like Ilyas Oskarver, if he ever shows his face [01:41:25] Swyx: again. [01:41:26] Swyx: And then I was like, but I know what's gonna win the Test of Time for 2027. Which is attention is all you need. [01:41:32] Swyx: Yeah, yeah. But basically it's a flex for any, any conference to say okay, Test of Time award goes to something that was presented here 10 years ago. And that and Neuros has been going on for 37 years. [01:41:46] Alex Volkov: what of the AI engineer presentations would stand the test of [01:41:50] Swyx: question. I think the audience has voted. It looks like Pydantic and Jason Liu's Instructure is very, very, very, very popular. And I think he's just fundamentally correct that every model, instead of there's like some table six versions of every model. You have the base model when you train it, then you have the chat tune model. [01:42:07] Swyx: And now I think it's going to be table stakes that every model should have structured output or function calling as, as they call it. And it's even useful if you're not actually using it to, to generate code or call code because it's very good for chain of thought. And so Max Wolf mini maxer on Twitter and on Hacker News actually wrote a really influential post that I'm going to try to showcase. [01:42:27] Swyx: Yeah, for me as a conference curator that's what I do. Read a lot of stuff and then I try to try to feature like the best of things and also try to make bets that are important. I do think as content creators, like we're like the end of the food chain and not the value chain. [01:42:45] Swyx: And it's always important to understand like even stuff that we don't pick is very important and substantial and it's [01:42:53] Swyx: You're, you're picking for an audience to use at work, which is a small subset of the total progress that humanity can make. [01:43:01] Alex Volkov: Interesting, interesting. Tell [01:43:02] Alex Volkov: me [01:43:03] Swyx: I just people, you want to engage in philosophical conversation, you go to Lex Friedman or Dorkesh Patel. [01:43:11] Swyx: And then if you want to Think, talk about things that you can use in open source. You go to Thursday, ai. And then we have less of an open source focus. We are, we're very much focused on enterprise and things you, things you can use at work to code and to build products and startups with. [01:43:26] Swyx: And so like I, whatever you do, as, as long as you have a clear focus for the, of the audience that you serve and you know how to reach them, then they will love you because you are, you're making literally the thing for them. And you don't have to appeal to everyone. And I think that's fine. [01:43:40] Alex Volkov: switching gears from the kind of the conference. [01:43:43] Alex Volkov: How did the podcast came about? It's you said you're coming up on the year [01:43:46] Alex Volkov: of [01:43:46] Alex Volkov: the [01:43:46] Alex Volkov: podcast. And you also said you moved here in April. I did not know this. [01:43:49] Alex Volkov: I [01:43:49] Alex Volkov: thought you're here for SF Native. So how did the podcast came about? How you and Alessia met? Let's talk about [01:43:54] Swyx: later. Yeah. And we should talk about doing well in San Francisco and like the taxi in, in Ingra, I think, which I, which I think is important and something I'm. [01:44:01] Swyx: going through but have also done well at. So the podcast specifically was because I started the newsletter writing opinion pieces on just AI stuff. It was actually inspired by Stable Diffusion at the time which was sort of August 2022 ish. [01:44:16] Alex Volkov: My life changed after that open sourcing. [01:44:19] Swyx: Yeah, and then you you really run out of opinions very [01:44:22] Alex Volkov: and [01:44:24] Swyx: and then you're like, oh, I need to generate unique or new tokens. [01:44:29] Swyx: The only way to do that is to get source material by interviewing people and putting a microphone in front of them. When you put microphones in front of people, they get more chatty. And sometimes they break news. For us, the big breakthrough was George Hotz when he talked about GPT 4 with being a mixture of experts. [01:44:44] Swyx: Yeah, that was, that was a surprise, but he likes to do that sort of thing, just drop random alpha. [01:44:49] Alex Volkov: he dropped it and then you guys posted it and then I had no idea what Mixture of Experts is as well as like most of us and then it turns out to be like a true and now we we [01:44:59] Swyx: saw it. Now Gemini is [01:44:59] Alex Volkov: Gemini's Mixture of Experts the 1. [01:45:01] Alex Volkov: 5 which is quite incredible so that was like a big thing did was this like natural to you to start turning on the microphone did you have to do an [01:45:08] Alex Volkov: adjustment period [01:45:09] Swyx: another thing that people don't know is that I started four podcasts before. [01:45:13] Swyx: So I'm not new to the conversation game, and I'm not new to like audacity and like editing and publishing, but I think, Having taken a few runs at it helps to prep you for, like, when something actually has audience fit. [01:45:26] Swyx: Because all the others were very small. There were maybe like a few hundred listeners each time. This one went to number 10 on the U. S. tech charts. [01:45:33] Alex Volkov: Yes, I saw that. That was incredible. Is that the top, top, [01:45:36] Swyx: I think that's the highest it's been. Recently when it was like as high as 16 over the holidays, and then now it's dropped back down again. It's very, very volatile. [01:45:44] Alex Volkov: But it's like very clear that you're in the top 50 like tech podcasts in the world, even though AI is Fairly niche. And the topics you discuss are fairly technical. [01:45:52] Alex Volkov: Like when you talk with folks, it's not a general appeal audience for like Sweden does, or the, the guys from the four guys, the VCs, right? It's very technical. So very impressive that like you broke the top 50 charts and it wasn't by chance you bring like great guests. Like, how do you, is the same approach that you have for the engineer you do for guests as well? [01:46:13] Alex Volkov: Or are you now getting like requests to come on the podcast from some other [01:46:15] Swyx: We get requests but you usually, for the, the people that draw the audiences, you have to go reach out to them. It's obviously, that's how it is. I [01:46:24] Alex Volkov: I heard one such person now does not work in OpenAI, so he can [01:46:28] Alex Volkov: potentially, potentially join [01:46:29] Alex Volkov: podcasts as [01:46:30] Swyx: yeah, he's a, he's a he's a listener and he has said that he'll come on at some point. [01:46:35] Alex Volkov: We're talking about bad Mephisto for folks in the [01:46:37] Swyx: Mephisto for Fortunyaga. So yeah, [01:46:41] Swyx: I don't think it's actually just guests. I think it's also about focus on topics and then being engaged enough with the material that you get to ask questions that no one else asks. [01:46:51] Swyx: Because, for example, if you have a VC asking questions, they often ask about market and business. But if you're an engineer, you're really asking about API and limitations and trade offs, stuff like that. Things that you don't really get into unless you're, like, actually evaluating it to use something at work. [01:47:09] Swyx: And I think that's important. And also, I think a lot of guests For us, we try to be like the first podcast that somebody has done. Like we're the first podcast for for Fine, for Cursor, for a bunch of these guys. So they're not experienced speakers. They're not some of them are good speakers. [01:47:25] Swyx: But they're not experienced at the whole telling their story and all that. So you have to help them. But it doesn't matter because I think that you just try to serve your audience at the end of the day, right? What do people want to know? Ask those questions and then get out of their way and let them talk. [01:47:38] Swyx: I think that the other thing that we do, the reason I say it's not just GUESS. is because we do special episodes where we have breaking news. We haven't done one in a while because I don't know. I think, I think you got, you have taken that spot of, of the breaking news guy. We [01:47:50] Alex Volkov: got [01:47:51] Alex Volkov: the, we got three breaking news, you were here. This is kind of like, that as [01:47:54] Swyx: that as well. And then we also do like events recaps. Like we did Dev Day we did NeurIPS and that is like a really big sort of editing process work that I really like to do where you're basically performing the work of summarization and Curation, instead of doing long form interviews, and people really like that. [01:48:13] Alex Volkov: summarization part, like the multiple folks, I think I participated in one, you did one in DevDay NeurIPS as well. So what's, what's [01:48:20] now that we're coming up on an annual kind of thing for, for Latentspace, what's next for Latentspace? [01:48:24] Swyx: More conversations? That's the weird thing we think that we've done and have done as well as a technical podcast can do in the general podcasting space. [01:48:36] Swyx: The ultimate number of people who listen to podcasts is still very low. compared to the general audience that might be interested in the same kind of content. That's why I branch out into a conference where you produce talks and very highly polished and all that. We The way to grow a podcast is to not just podcast it's to actually write, where, my essays still get a lot more readers than listeners than to grow on YouTube or whatever, and that's fine. [01:49:05] Swyx: I think ultimately, podcasting is a mix of entertainment and Education, right? You have to be attached to some kind of story, some kind of personality, and, and then learn something along the way that might be useful at work. So I think personally, I growing as a podcaster is about just growing your influence or understanding of an industry in general and the ability to serve an audience. [01:49:29] Swyx: And then maybe opening up as hosts and as industry experts as we gain knowledge and understanding. So that people come to us not just for access to guests, but access to us as well, which people have when we did the end of year listener survey people actually requested for us to have more mic time. [01:49:47] Swyx: Alessio and I did our first just the two of us conversation in a year and that was really good. [01:49:52] Alex Volkov: Wow. So are you playing more, more of those? [01:49:54] Swyx: Yeah, yeah, we, so we used to do these one on one episodes where we do Introductions to a topic, like we did Datasets 101, Benchmarks 101, and we did Transformer Math 101, and then we also did RLHF 201. [01:50:07] Swyx: And so we want to do more of those, where it's like it's like inspired by Acquired FM. And the work for this kind of episode is so different than a normal chat, because in normal chat you just sit down and you, you, maybe you prep a bit, a bit of question, you, you research the other guy's background, and then you just have a nice conversation, and that's it. [01:50:23] Swyx: Whereas for a content heavy episode like that one, you do [01:50:27] Swyx: a [01:50:27] Swyx: week of research. And you compile a whole bunch of stuff, and you simmer it in your mind, and then you try to rehash it and introduce it for an audience who hasn't done that amount of work. Yeah, that, that is a lot more work up front, but obviously it's very high value, and, and also I, I like to call it evergreen. [01:50:43] Swyx: Evergreen content, meaning, like You want to build up something that will still be useful and relevant in a year. [01:50:48] Alex Volkov: Yeah. So definitely let me, let me just take a personal position here with Latentspace. [01:50:53] Alex Volkov: I've been a guest host, in Latentspace a couple of times, in special episodes as well. I, now this, this studio is like super cool, like a home away from home. They're able to come here to the spaces, Alessio on Tap into the AI scene in San Francisco. And I've learned a bunch from just the way you render. [01:51:11] Alex Volkov: Latentspace, for folks who are listening, is not only just a podcast. If you're subscribing on just your Spotify or Apple News, you're missing a big part of it, which is the newsletter that you send, which has a bunch of links and show notes and folks that you talk [01:51:23] Swyx: about. [01:51:23] Swyx: There's one more part. Discord. [01:51:26] Alex Volkov: Oh, there's also Discord. [01:51:27] Alex Volkov: You do paper readings as well, right? There's a whole community that you're building. [01:51:30] Swyx: community The Discord is surprisingly good. For the zero effort that I put into it, people just show up, and then they ask really very good questions, they drop things that I don't know, and then I learn from the Discord, and then I talk about it later. But, yeah, Discord has a lot of alpha. [01:51:47] Swyx: And it's surprising because I have this newsletter that, I have this bot, That summarizes all the top AI discords, right? Obviously the top ones are, like, Eleuther, TheBloke what else? [01:51:55] Swyx: Yeah, mid, mid, yeah, but it's not, that's not very technical. That's mostly just prompting. [01:52:00] Swyx: Midrani is 8 million members. That's something like 13 percent of total Discord membership. Ha ha ha ha ha. That's freaking crazy. But anyway, so like the Discord is the community attachment to the podcast and the newsletter. And then it's, people interacting with each other, some people getting jobs, some people getting investments, I have founders coming in and VCs there also funding them. [01:52:22] Swyx: And like I, I really think that every every piece of content is a minimum viable community, right? People gather, they're chatting in the Twitter space comments right now. They're chatting in your newsletter comment section. But if you let people gather together live, whether it's online or in person we also have in person meetups. [01:52:40] Swyx: I just had one in Singapore. We have one in San Francisco, I think, monthly. [01:52:45] Swyx: I hope to have it monthly. And then obviously once a year you get people together for a really big conference where like they put out their best work. So I call this community annealing, right? You have cold community, like podcasts are cold. [01:52:58] Swyx: Newsletters are cold because they're asynchronous. There's not somebody there, you don't expect to respond to the other person. Twitter spaces are warm because they're live and, there's some chance of live feedback. Discords are live, but when you, when you, when they're hot, it's when like everyone is on the same call and you're looking in each other's eyes. [01:53:16] Swyx: And you're conversing and you're, you're having like a real bond and relationship there. And so like communities need this whole range of like warm and hot and cold. And I try to build that for Dane Space. [01:53:28] Alex Volkov: So for folks who are just listening on podcasts, you're missing several parts of the space. Newsletter is definitely worth checking out. Latent. space is actually a URL. [01:53:38] Swyx: And that was donated by a reader. Not donated. Sold to us for cheap. [01:53:42] Alex Volkov: You can consider this a donation but also the Discord part speaking of work that I think we need to wrap up because like we're after two hours and I want to let you go back to work. I also need to edit this and send this. I also want to check out the stuff that we did. Any last kind of parting things here? [01:53:56] Alex Volkov: Maybe let's touch briefly or is that a bigger conversation? How to succeed in SF or is that for a later [01:54:02] Swyx: Oh yeah, yeah, yeah. Oh man. This is such an interesting topic, especially for people who are not in sf, right? [01:54:06] Swyx: Yeah. I think SF is a group of humans and not a place, and they are mostly available on Twitter. Yeah. But then sometimes they, they often gather in San Francisco and Yes, when you meet them in person. There are some people that are not famous online or not fully consistently candid online that you talk to them in person and you're like, Oh, okay, I fully understand you now and everything that you've done and everything that you're going to do, I understand where you're coming [01:54:33] Swyx: from. [01:54:34] Swyx: And to me, that is obviously a very high offer, that's why I moved here. But you don't have to go there directly, right? One of my mentors And the last one that I want to talk about is in career is Andrew Chen, who basically blogs his way into being a general partner at Andrews and Horowitz. [01:54:49] Swyx: Like he runs one of their top three funds, the consumer fund. And he consistently is Hey, just Put out your best work, learn in public, tweet a lot and instead of going to all these parties, there's always, there's always a party every week in San Francisco [01:55:03] Alex Volkov: Every day, multiple stacks a day sometimes, yeah. [01:55:06] Swyx: There was one Thursday last year with 10 AI meetups in San Francisco. [01:55:09] Alex Volkov: So [01:55:10] Swyx: can go through the motions of networking, but you still end up with a smaller network than you would if you stayed at home. And you just wrote a lot, or you thought a lot, or you did quality work. And so then you don't have to be in San Francisco to do that. You can just, you can keep doing that online. [01:55:27] Swyx: And then, take advantage of a big conference or something to come into San Francisco and actually meet people in person. And that's totally fine. I don't intend to stay in San Francisco forever, right? I have, once I know enough people, I can just come here like once a quarter and people will still think that I'm in San Francisco. [01:55:41] Swyx: And that's fine. [01:55:41] Alex Volkov: I get this question quite a lot. I've been here, maybe this is the fourth or fifth time for the past six months, and I get this question, do you live here? I was [01:55:48] Swyx: Yeah. I think, I think people are just like borders. I, I'm, I'm a border disrespector and I think I hope more people do that. But do come into San Francisco every now and then maybe for a big conference that's happening June 25th to 27th. [01:56:02] Swyx: But otherwise do great work online and people will notice it and find you and chat with you. And the in person component doesn't matter so much as plugging into the mentality and the community online. [01:56:12] Alex Volkov: Yeah. SWIX, it's been a surprising interview. I didn't plan on this. [01:56:15] Alex Volkov: I just thought we're here. I haven't heard you in a while. The anniversary of latency is coming up a huge kudos for this effort. Like huge kudos. Big, big, big, big. Thank you for me because a lot of what the stuff that you did, you and Alessio pulled me through. I, I still get like a bunch of listeners for Thursday. [01:56:30] Alex Volkov: I, from the Latan space work on Substack. And so a huge thanks for me because you, you kinda shaped. what I'm doing as well. The newsletter and the podcast combo that I forced myself to doing every [01:56:40] week. This was, this was based on the Substack stuff from you as well. And I really appreciate your, your friendship as well. [01:56:45] Alex Volkov: So thank you for coming up on Thursday. I thank you for hosting us in Latentspace. And with that, I think I'll move on to the last piece of what we have on Thursday, iFolks, which is a recap of everything we've talked about. And then I'll just briefly run through recap and I'll let you go to your day. We haven't, let me just start with the music, obviously, because like, how else would this work? [01:57:02] Alex Volkov: However, with that, I just want to wish you a great Thursday. Thank you for joining us from week to week. I want to thank the co hosts that I had on stage. Thank you, Nisten. Thank you, Jan. Thank you, LDJ. Far El was here. Alignment was here. Thank you. A huge thank you for Swyx, Alessio, and the Latentspace folks for hosting me here. [01:57:19] Alex Volkov: A shout out to a bunch of friends in Silicon Valley who I'm gonna meet. And with that, we'll see you next week. I'm gonna go and try to somehow summarize this all in the newsletter and podcast for you. And we'll see you folks next week. From San Francisco. This has been Alex Volkov. Cheers, everyone. [01:57:34] Alex Volkov: Not this one. Bye bye. This is a public episode. If you'd like to discuss this with other subscribers or get access to bonus episodes, visit sub.thursdai.news/subscribe | |||
23 Feb 2024 | 📅 ThursdAI Feb 22nd - Groq near instant LLM calls, SDXL Lightning near instant SDXL, Google gives us GEMMA open weights and refuses to draw white people, Stability announces SD3 & more AI news | 01:48:04 | |
Hey, this is Alex, Ok let's start with the big news, holy crap this week was a breakthrough week for speed! We had both Groq explode in popularity, and ByteDance release an updated SDXL model called Lightning, able to generate full blown SDXL 1024 images in 300ms. I've been excited about seeing what real time LLM/Diffusion can bring, and with both of these news release the same week, I just had to go and test them out together: Additionally, we had Google step into a big open weights role, and give us Gemma, 2 open weights models 2B and 7B (which is closer to 9B per Junyang) and it was great to see google committing to releasing at least some models in the open. We also had breaking news, Emad from Stability announced SD3, which looks really great, Google to pay Reddit 200M for AI training on their data & a few more things. TL;DR of all topics covered: * Big CO LLMs + APIs * Groq custom LPU inference does 400T/s Llama/Mistral generation (X, Demo) * Google image generation is in Hot Waters and was reportedly paused (refuses to generate white people) * Gemini 1.5 long context is very impressive to folks (Matt Shumer, Ethan Mollick) * Open Weights LLMs * Google releases GEMMA, open weights 2B and 7B models (Announcement, Models) * Teknium releases Nous Hermes DPO (Announcement, HF) * Vision & Video * YoLo V9 - SOTA real time object detector is out (Announcement, Code) * This weeks Buzz (What I learned in WandB this week) * Went to SF to cohost an event with A16Z, Nous, Mistral (Thread, My Report) * AI Art & Diffusion & 3D * ByteDance presents SDXL-Lightning (Try here, Model) * Stability announces Stable Diffusion 3 (Announcement) * Tools * Replit releases a new experimental Figma plugin for UI → Code (Announcement) * Arc browser adds "AI pinch to understand" summarization (Announcement) Big CO LLMs + APIs Groq's new LPU show extreme performance for LLMs - up to 400T/s (example) * Groq created a novel processing unit known as the Tensor Streaming Processor (TSP) which they categorize as a Linear Processor Unit (LPU). Unlike traditional GPUs that are parallel processors with hundreds of cores designed for graphics rendering, LPUs are architected to deliver deterministic performance for AI computations. * Analogy: They know where all the cars are going when everyone wakes up for work (when they compile) and how fast they all drive (compute latency) so they can get rid of traffic lights (routers) and turn lanes (backpressure) by telling everyone when to leave the house. * Why would we need something like this? Some folks are saying that average human reading is only 30T/s, I created an example that uses near instant Groq Mixtral + Lightning SDXL to just create images with Mixtral as my prompt manager Open Source Weights LLMs Google Gemma - 2B and 7B open weights models (demo) * 4 hours after release, Llama.cpp added support, Ollama and LM Studio added support, Tri dao added Flash attention support * Vocab size is 256K * 8K context window * Tokenizer similar to LLama * Folks are... not that impressed as far as I've seen * Trained on 6 trillion tokens * Google also released Gemma.cpp (local CPU inference) - Announcement Nous/Teknium re-release Nous Hermes with DPO finetune (Announcement) * DPO RLHF is performing better than previous models * Models are GGUF and can be found here * DPO enables Improvements across the board This weeks Buzz (What I learned with WandB this week) * Alex was in SF last week * A16Z + 20 something cohosts including Weights & Biases talked about importance of open source * Huge Shoutout Rajko and Marco from A16Z, and tons of open source folks who joined * Nous, Ollama, LLamaIndex, LMSys folks, Replicate, Perplexity, Mistral, Github, as well as Eric Hartford, Jon Durbin, Haotian Liu, HuggingFace, tons of other great folks from Mozilla, linux foundation and Percy from Together/Stanford Also had a chance to checkout one of the smol dinners in SF, they go really hard, had a great time showing folks the Vision Pro, chatting about AI, seeing incredible demos and chat about meditation and spirituality all at the same time! AI Art & Diffusion ByteDance presents SDXL-Lightning (Try here) * Lightning fast SDXL with 2, 4 or 8 steps * Results much closer to original SDXL than turbo version from a few months ago Stability announces Stable Diffusion 3 (waitlist) Uses a Diffusion Transformer architecture (like SORA) Impressive multi subject prompt following: "Prompt: a painting of an astronaut riding a pig wearing a tutu holding a pink umbrella, on the ground next to the pig is a robin bird wearing a top hat, in the corner are the words "stable diffusion" Tools * Replit announces a new Figma design→ code plugin That’s it for today, definitely check out the full conversation with Mark Heaps from Groq on the pod, and see you next week! 🫡 ThursdAI - Recaps of the most high signal AI weekly spaces is a reader-supported publication. To receive new posts and support my work, consider becoming a free or paid subscriber. Full Transcript: [00:00:00] Alex Volkov: Hey, this is Alex. This week on ThursdAI, we had an hour conversation with Grok, a new and very exciting AI inference chip that exploded in popularity all over social media after showing a 5x, yes, 5x improvement in AI inference. 500 tokens per second for Lama70B and Mistral. [00:00:32] Alex Volkov: We also talked about Google's new OpenWeights GEMMA model, Google's image generation issues, which led them to take down the abilities of this image generation to generate people. We covered new, incredibly fast SDXL lightning, and we had breaking news for Stable Diffusion 3, which is a diffusion transformer that's coming out of Stability AI. [00:01:03] Alex Volkov: and a bunch of other news. All that after this short intro into Weights Biases. [00:01:10] AI teams are all asking the same question. How can we better manage our model development workflow? The path to production is increasingly complex, and it can get chaotic keeping track of thousands of experiments and models. Messy spreadsheets and ad hoc notebooks aren't going to cut it. The best AI teams need a better solution. [00:01:33] And better tools. They need Weights Biases, the AI developer platform, to unlock their productivity and achieve production ML at scale. Replace messy spreadsheets with an automated system of record for experiments. [00:01:52] Communicate about model evaluation. and collaboratively review results across the team. Clean up disorganized buckets of models with a unified registry. Automatically capture full model lineage, all the data and code used for training and testing. Seamlessly connect to compute to scale up training. And run large scale sweeps efficiently to optimize models. [00:02:20] Analyze the performance of large language models. And monitor LLM usage and costs with live, customizable dashboards. Get your team on the same page to bridge the gaps from ideation to production. Use Weights Biases to build, manage, and deploy better models, faster. [00:02:41] Alex Volkov: Wasn't this cool? This is Kari. She is a original PM on the Weights Biases team. She's been there for a long time and recently we used her voice to narrate this new video that we have up on the website. And I figured I'd put it in here because it works even without the video. And I thought it was super cool. [00:03:01] Alex Volkov: And people ask me, what does Weights Biases do? And hopefully this answers some of those questions. Now I want to switch gears and say, basically. that the format for this week is a little different. We had the folks from Grok and Matt Schumer at the beginning of the pod, and then we kept talking about everything else, like Gemma and Gemini and everything else. [00:03:24] Alex Volkov: So the first hour of this is going to be an interview with the Grok folks, specifically with Mark Heaps and the next hour afterwards is going to be the deep dive into topics. If you're listening to this on Apple podcast, for example, you should be able to just view chapters and skip to a chapter that you'd prefer. . [00:03:51] Alex Volkov: I want to just do a quick recap of ThursdAI for February 22nd everything we've talked about for today and we started the space with a with two I guess Matt Schumer and mark Heaps from, and that's Groq with a Q at the end, not Groq with a K at the end. So not like X ais Groq. Groq is explo on our timelines recently with just incredible viral videos of them performing l la inference on LAMA two 70 B and Mixtral with around 400 or 500 tokens a second, which is. [00:04:34] Alex Volkov: Five times as much as the previous super fast API inference that we've seen for perplexity and from together. And they're serving like Lama 270B with 500 tokens a second. And so we've had Mark from Groq talk to us for almost an hour about how this is even possible. So we had a very nice deep dive with Mark and definitely if you miss this, please check this out on, on the recorded portion as well. [00:04:58] Alex Volkov: And then we also had Matt, who works at HyperWrite, and he's been playing with these tools, and he told us about the demos that he was able to build, and How much of a difference this speed of inference makes. We've talked about their custom chip called LPU, and we've talked about the fact that the company's been around for a while, and they did not expect this explosion in virality, but they're very happy that they chose this direction correctly. [00:05:21] Alex Volkov: Very great interview, great conversation, and I invite you to listen to this as well. We covered that Google image generation is now in hot waters, and was reportedly paused because it's in injecting prompt stuff that they're not that great, let's say. And many people notice that historical figures are being generated in different races, and different multicultural adjustments are happening to your prompts, which is not great. [00:05:46] Alex Volkov: This blew up on Twitter, and even outside of Twitter, I think folks started writing this in actual Media Google, enough so that Google took down the image generation of people trying to figure out what to do with this. But we also gave props for Google to release Gemma. Gemma is an open weights 2 billion and 7 billion parameter model, and we've talked about Gemma we've gave Google the props for releasing OpenWeights for us, and we had folks here on stage telling how the base model is still yet to be decided, how good this actually is, very fine tunable, we're waiting for the open source community to come together and fine tune the OpenWeights Gemma from Google, and then we also covered the Gemina 1. [00:06:29] Alex Volkov: 5 loan context again, they released the 1 million, and, Context window support and many folks got access to this and we saw for the past week people playing And doing all kinds of stuff including Matt Schumer who I just mentioned he also got access So he gets all the cool toys and he was able to put , three Harry Potter books in one prompt and ask the model with perfect recall who said what and this could have been part of whatever Existing knowledge, but he was doing this more for a demo We also saw demos of people putting an hour long video in the prompt which is around six hundred or five hundred thousand tokens Which sounds ridiculous that it supports it and the model was able to understand this whole video And tell you which scene happened when, with almost near perfect precision. [00:07:13] Alex Volkov: And we've talked about how this changes the game for multiple things, and we're gonna keep updating you about these long contexts. And we also brought this to Groq and said, Hey, are you gonna support long contexts with your insanely fast speed of inference? We also covered that news Research Tech released a new service DPO fine tuned, which is better in every possible benchmark on top of their ori already strong flagship models which is great. [00:07:37] Alex Volkov: And I covered that. I went to San Francisco to host an event with a 16 z and news research and Mistral and All Lama and a bunch of other folks, and it was a great event. And I shout out to A 16 z folks for hosting this and inviting me. There as well. And then last thing we've covered is two AI art and diffusion stuff where ByteDance releases SDXL Lightning, which generates 1024 super high quality images in just two or four steps and they look incredible and super fast to generate as well. [00:08:08] Alex Volkov: I've talked about the demo that I built with them and I've talked about this example that File. ai has where you can go to fastsdxl. ai and just type and as you type, the image generates on the fly [00:08:20] with around 300 milliseconds of inference time which feels real time and feels quite incredible. And following that, we have breaking news today from Stability announcing Stable Diffusion 3. [00:08:30] Alex Volkov: which is a diffusion transformer, which we've covered before, a diffusion transformer based image generation model from Stability. They announced a waitlist that you can go and sign up for right now. And it looks like it's significantly better at following very complex prompts, like multiple objects and colors and everything in one prompt. [00:08:47] Alex Volkov: This is everything we've talked about on ThursdAI [00:08:49] Introduction and Welcoming Remarks [00:08:49] Alex Volkov: Yes. [00:08:55] Alex Volkov: All right, folks, you know the sound. Those of you who come back week after week, you know the sound. This is ThursdAI. My name is Alex Volkov. I'm an AI evangelist with Weights Biases. And I'm joined here on stage by, from week to week, by experts, friends of the pod, and new folks who actually were in charge of the news that we're going to talk about today. And Today is February 22nd, only February 22nd, and already so much happened this year with AI. Last week was crazy, this week was less crazy than last week, but still, so much to talk about. [00:09:36] Introducing the Co-Host and Guests [00:09:36] Alex Volkov: And I'm delighted to have my co host Nisten here. Hey Nisten, what's up? [00:09:43] Alex Volkov-1: Hey [00:09:43] Nisten Tahiraj: everybody, [00:09:44] Alex Volkov: How's your week? [00:09:45] Nisten Tahiraj: I'm just it's been the usual, just up until 2 or 3 a. m. on random Twitter spaces finding, because sometimes stuff gets pretty, [00:09:57] Nisten Tahiraj: it's pretty exciting. [00:09:58] Alex Volkov: Yep, stuff gets pretty exciting from week to week. I also want to say hi to Matt Schumer, joining us for a brief period. Matt, you've been all over my feed this week. How are you doing, buddy? You've been here before, so folks may not remember you. So please introduce yourself briefly, and then we'll chat. [00:10:16] Matt Shumer: Hey man, thanks for having me. [00:10:17] Introduction of Otherside AI [00:10:17] Matt Shumer: Yeah, so I'm co founder, CEO of Otherside AI. We are the creators of Hyperite, which is one of the largest AI writing platforms. And we also. I've been exploring the agent space for a couple years now about a year publicly creating AIs that can actually operate your computer. [00:10:31] Matt Shumer: As I mentioned, unfortunately, I do only have 10 minutes. I will potentially be able to join back up after so I'm really sorry about that. It's been a crazy day but excited to chat in the time that I have. [00:10:42] Alex Volkov: Alright, awesome. Thanks for joining. And then I think we'll just jump in into the conversation. And I want to say hi to our guest a new guest. [00:10:50] Introduction of Mark from Groq [00:10:50] Alex Volkov: I don't, I haven't talked with Mark before. Mark is Mark, feel free to unmute and let us know some of your background and where you're joining from. And then we're going to talk about the stuff that we're here to talk about. [00:11:05] Mark Heaps: Yeah, how's it going guys? Thanks for letting me join the space today, and glad to see some familiar names from all the craziness this week. Yeah, I'm the Chief Evangelist and Head of Design, Brand, and Creative over at Groq, which is probably a little bit not a normative title for folks that are so deep in the AI developer space, but we actually do a lot of the technical side too, so glad to be here. [00:11:28] Alex Volkov: Awesome. And so folks who are listening, that's Groq with a Q at the end. So not X's Groq. And you guys have been around. For a little longer than them. But just in case folks get confused, there's like a few confusion points here. And I think this is a good start for our conversation today. And I wanna turn this to Matt, because Matt, you're the first person who I saw post about Grock. I think this week, and some of your stuff got a bunch of attention. So give us like a brief overview, like what you saw that made you post. And then we're going to talk about this insane speed and then maybe turn to Mark into how it actually is done. [00:12:02] Alex Volkov: So what is, where's Groq? Like, how'd you get to it? And how viral did you actually get? [00:12:08] Matt Shumer: Yeah, it's a funny story. I actually found Groq I'd say more than a month ago and immediately I was blown away I think my co founder posted actually a text I sent to him, and I was like, you have to f*****g try this thing right now, it's incredible and he did, and he was blown away too, I actually went and posted about it back then, but it got no traction, I think I deleted it or something and I was just letting it marinate in my mind what was possible here, but, I wasn't sure, if this Scale obviously this week proved that thing wrong clearly it can but I was still just thinking about it, and then I was just on the train, my girlfriend and I were just sitting there on Sunday, and she just fell asleep, so I was like, what am I going to do right now, and for some reason, I thought of Groq, and I was like, okay, let's just post about it again, see what happens, and for some reason, this time, by the time I got off the train, it was going crazy viral. [00:12:55] Matt Shumer: I, Sunday night was fun, I was up pretty much all night just managing the blowback from this. Finally fell asleep by the morning, I woke up to a timeline filled with tweets about Groq and for good reason, right? This thing is incredible, and it's going to change how we think about how we work with LLMs, what they're capable of, the ability to do tons of reasoning, right? [00:13:16] Matt Shumer: All of that is now going to change, and a lot more is now possible. The one thing I wasn't sure about was, would this thing go down, right? With all this usage, would this thing go down? And, it hasn't, right? There was a brief time where there was a little bit of delay, but, more or less, it pretty much stayed up the entire time, which is crazy, through all of this, and they weren't prepared for that, which was incredibly impressive, and I think it's a testament to how good the hardware is. [00:13:41] Matt Shumer: It's just exciting to see. I actually spoke with Jonathan, the CEO of Groq yesterday, and he said that something like 300 developer API requests were submitted prior to the tweet. Now they're getting like 3, 000 a day or something, which is insane. Using that as a proxy for how many people must be trying the actual API, and then combine that with the demos I built that are getting thousands of hits every day, their servers are still clearly standing, which is, [00:14:06] Exploring the Impact of Groq's Speed [00:14:06] Alex Volkov: So what was impressive to you? I think we're dancing around the issue, but for folks who didn't see your viral tweets, what was the head explosion moment. [00:14:14] Matt Shumer: You have TogetherAI, you have HuggingFace, Inference, you have VLM, all this stuff, right? You're getting on, let's say, Mixtral, if you're doing incredibly well, 100 tokens per second or something, right? Most people aren't reaching that and that number may be off by a little bit, but at a high level, you're getting around there with any pretty standard model today, if you're doing well. [00:14:34] Matt Shumer: Now, going above 200? Unheard of. 500? Ridiculous, right? And that's where Groq sits, right? They've essentially developed a chip that enables these language models to be far faster. And when you see 500 tokens per second versus, let's say, 50 or 100, it is not just a small difference, right? [00:14:52] Matt Shumer: This is like a step change in what is possible with what you can build with them. And that's what turned my head, right? It's not just faster inference, it's a whole other paradigm that you could build on top of right now, right? When you have inference that's fast, you can then do, 5 to 10x the reasoning in the same amount of time. [00:15:10] Matt Shumer: How much better does the answer get with the same LLM if you do that? You could do interfaces that are created for you in real time. You don't have to wait. For example, right now on the HyperWrite platform, it's probably one of the best sort of conversational platforms with web search built in, but you still have to wait for it to go and execute the web search, come back, write the response, think through what it needs to do. [00:15:28] Matt Shumer: What happens if that's instant? That changes everything. That's what got me super interested. Here's what others think about it though. [00:15:35] Alex Volkov: Yeah I wanna chime in here. Thank you, Matt. I saw your tweet immediately What? And also, A day before I saw your tweet and we're going to talk about long context and maybe after you're gone, maybe you'll come back as well. But a day before I saw your tweet, I posted something where folks were complaining about kind of the long context with Gemini 1. [00:15:53] Alex Volkov: 5 Pro with the million. That's saying, oh, it's going to take too long. It's going to cost too much, et cetera. And I posted something like, that's not going to, that's not going to be the truth forever. These things are coming down faster than people realize, and I think those things together just one after one, to show me how fast we're moving, how incredible this is, because and we're gonna talk about long context here in a second as well, but Immediately a day after I saw your tweet, I was like, oh, there's an example. [00:16:18] Alex Volkov: This is exactly what we're talking about. Just I didn't expect it to take a day. So I want to turn the conversation to Mark real quick. [00:16:24] Discussion on Groq's Custom Chip [00:16:24] Alex Volkov: Mark, you worked in Grak. How long have you been there? Tell us about this custom chip you guys have. What's going on? How are you achieving this insanity? 500 tokens a second for Llama70B, which is quite big. [00:16:38] Mark Heaps: Yeah. Happy to. And [00:16:40] Jonathan actually called me and told me that he spoke to Matt yesterday, and I said, I think we owe Matt a, a very nice steak dinner and maybe a little bit more than that. I also didn't sleep at all that night because there were so many requests coming in and Matt's right, like we weren't really ready for it. [00:16:55] Mark Heaps: We were literally just discussing. The day before what are some other demos we can do? What are some things we can show people with the speed? And then all of a sudden, Matt did a post and then a number of other people that follow him started doing posts. And, next thing I know, people are making their own video demos and it blew us all away. [00:17:11] Mark Heaps: We're like, wow, this is amazing. I owe a big thanks to the community that have jumped on this. The, this is the magical moment. I think anybody that's worked in tech has seen this before. I've been working in tech for about 30 years. And there's this rubber band effect where one end pulls forward and then you have the whiplash from the other side. [00:17:30] Mark Heaps: And software developers have been doing an amazing job in AI for the last couple of years trying to, find more efficiencies, eek out better inference, trying to get, anywhere they can that optimization. But, classically what happens is you push that to a point where you start seeing a ceiling. [00:17:46] Mark Heaps: And then hardware comes along and says, Oh, you're driving the car at max speed? Let me just give you a new engine. Let me give you, something that speeds that up. And we've seen people saying that they have an inference engine. But ultimately they're really just these brokers of other forms of cloud compute. [00:18:01] Mark Heaps: And then again, eking more capability out of it through software. And Groq was completely different. I've been there now for about four years. And I remember when I originally met the CEO, Jonathan, I said, why does anybody need to do this? And he told us the story about, him creating the TPU over at Google. [00:18:19] Exploring the Future of AI with Groq [00:18:19] Mark Heaps: And it was a pretty interesting moment. Jeff Dean had told the team at Google, Hey we've got really good news. We figured out how to get AI working and get, these certain services working like image and speech, etc. But the problem is it's going to cost a fortune to expand our data centers to be able to handle this capacity. [00:18:36] Mark Heaps: And then they realized they needed to invent a new chip to do that. We're seeing that repeat itself right now. where there was this low latency ceiling for everybody in regards to incumbent or legacy solutions. And he knew from day one that, everybody was out there training models for years. [00:18:53] Mark Heaps: And he said, one day, this is all going to turn around and everybody's going to want the world's fastest inferential latency. And he didn't know exactly where that was going to be a product fit, but he did know that was going to be the problem statement. So that's what they, that's what they started with. [00:19:06] Mark Heaps: And it's a radically different architecture, totally different methodology and approach. It's been a really fun journey learning about that architecture. Yeah. [00:19:14] Alex Volkov: And like the. The public demo that you have, that's very easy for folks to just go and test this out on their own. I think it to be honest, it's awesome that you have this, and I think it undersells. The insanity of what this is, and I think when I hear about what Matt is building in the demos, and I had to play with this yesterday, I had to play with this myself to figure out what to do with this, because I saw many people react and say, hey, what's the point of 500 tokens per second when the reading speed of humans is I don't know, 50 tokens per second, whatever, and I'm looking at this tweet and I'm just like face palming, I was like, what, you don't, do you not [00:19:51] Mark Heaps: Oh, thank you. [00:19:52] Alex Volkov: Do you not what's going on? So I had to go and build something. I built I'll tell the folks in the audience. So I used actually two technologies. We're gonna talk about the second one today. I used two kind of super fast advancement that we had this week, which another one was a stable diffusion SDXL Lightning from, I think TikTok, I think released this. [00:20:09] Alex Volkov: And I decided to just combine both of them, and I have a video, I'm gonna post it up on the show notes and on the demo right now, on the stage right now. But folks, don't go looking at this right now, go look at this afterwards. And I basically figured, Hey, if this is like as lightning fast as this is, I don't have to like, I'm like 400 tokens a second, 500 tokens a second, basically instant, I can use whatever Mixtral or you have Lama270B there, you have Mixtral, and hopefully we're going to talk about more models soon. [00:20:37] Alex Volkov: And I can use this SDXL Lightning to just immediately deliver to me results. So I used Llama as my kind of prompt writer via Groq, and then I used this SDXL Lightning as my image generator, and I have a demo there that everything there appears in real time. And it's quite powerful and, to the person who said, Hey, the reading speed of people is 50, 50%. [00:20:59] Alex Volkov: That person doesn't understand the impact of this. They will have an agent, for example, Matt was talking about agents and agentic stuff. The impact of this is just being able to build LLMs into every possible nook and cranny of software. I just wanted to highlight that, that I had to play with this to understand, really. [00:21:14] Alex Volkov: And, Mark maybe let me ask you what kind of like inventive demos and stuff that you saw coming up from folks specifically around the fact that some of this stuff would not be very helpful with slower inference speed? Did you see any like cool examples of your own? Did you guys like, in slacks, did you send the examples between each other? [00:21:32] Mark Heaps: Yeah, there's been a lot of chatter at Groq, and I think Matt's was the first one that kind of blew me away. He, he built a demo. And then I think his second demo was this one that wrote a novel, and it wrote it in like under a minute or something [00:21:45] Alex Volkov: you want to tell us about this before, before you drop off? Because while I got you here, I would love to hear. Yes, [00:21:56] Matt Shumer: because I wanted to go to sleep. And I knew I had to get it done, and I wouldn't have slept if I didn't. So that was this answers engine, very similar to Perplexity. The idea there was Perplexity's got this incredible, Embeddings based system likely it's really fast and allows you to answer questions really quickly so anybody going up against them they can't exactly do that because without that engine, it's going to be way slower but with the LLM that's as fast as Grox hosting of it, you can essentially do it in the same exact time or even faster while waiting for a pre built, search API to come back with results. [00:22:28] Matt Shumer: And it worked. So basically, obviously after time it got a little slower because a ton of people were using it, but at the beginning it was like a second to answer for a very complex question. You could have it write a long thing based on something. So basically a really good answers engine. That was the first one. [00:22:42] Matt Shumer: The second one was writing a novel in a minute or something. That came from a repo that I open sourced, I want to say like almost a year ago now. And that was called GPT Author. Originally the idea was to use GPT 4 to write a novel for you. The quality is obviously okay, it was just experiment to see where it went but people really took to it, so I decided to rebuild it. [00:23:02] Matt Shumer: With gbt author originally, with gbt 4, it would take like 20 minutes to write, let's say, five chapters. The crazy thing is, with Groq, I added like three more layers of reasoning for each chapter, and yet it still computed it under like a minute or two. So that was pretty crazy. And then the third demo I released, which kind of went More volatile than the rest. [00:23:24] Matt Shumer: That was basically a code tool that refactors code and documents it. So basically, it's a very simple design. You paste in some code. We have one Mixtral prompt essentially suggest improvements. Based on those improvement suggestions and the original code, we have another Mixtral go and make those improvements. [00:23:45] Matt Shumer: We display the diffs and then based on that we have another Mixtral explain what happens and Give the user an understanding of what happened, and then we have a fourth one go in and document it. And this all happens, if I were building this for production with today's models and today's systems, I would probably go and try to make some of it async so that it's faster to the user. [00:24:05] Matt Shumer: But with this, I built it sequentially because I didn't even have to go and do that. It all still computed in a second. By the time I was done reading the code changes for, or the suggestion that it was going to do in the first place, it was already done refactoring the code, already done documenting the code, which is crazy. [00:24:20] Matt Shumer: So that one did pretty well. Those are the three demos I made. Maybe I'll do some more in the coming days. Yeah. [00:24:24] Alex Volkov: that's incredible, dude, and I keep thinking about like more use case for this. Yesterday I used Cursor. Cursor is the editor, if you guys don't know, like AI native editor, uses I think GPT 4 behind the scenes, embeds a bunch of stuff. And I haven't been able to play with CursorFully until yesterday's demo, and I played with this, and it has GPT 4. [00:24:42] Alex Volkov: And I think they have a specific faster access to GPT 4, if you pay, and we do pay. And I was playing with this, and I was getting support from my editor on my code, and it was slow, and I was like, I want it immediate. I want it instant. And I think that's what Groq of promises. [00:24:59] Alex Volkov: Mark, [00:25:00] so let's talk about how you guys actually do this. You said something about the custom chip. What's as much as you can go into the secrets and also keep in mind that this is like a high level space here on Twitter. What's going on? Like, how are you able to achieve NVIDIA's earnings come out. [00:25:15] Alex Volkov: They did like whatever insane numbers for the past year. Everybody's looking at A100s, H200s, whatever. What are you doing over there with new hardware? [00:25:23] Mark Heaps: Yeah. The chip has actually been something we've been working on. The company was formed in 2016. And I think we, we taped out that chip, the first generation design, maybe two years after that. And it is totally different. And it's funny, people actually keep getting the category of the processor wrong online. [00:25:41] Mark Heaps: It's a language processing unit, but people keep calling it a linear processing unit. And a lot of the engineers at Groq think that's fun because they're like technically, it is. It is a linear sequential processing unit, right? And it's some of the key differences at a high level, right? So it's not multi core like a GPU. [00:25:56] Mark Heaps: It is single core. It was actually the world's first single core peta op processor, which, four or five years ago, that was a big deal. And it's still 14 nanometer silicon, which is a 10 year old version of silicon dye. Whereas, we're being compared to people that have silicon that's four and five nanometer. [00:26:14] Mark Heaps: And we're completely fabbed in the U. S. It's it's readily available supply. So we don't have the challenges other folks have trying to get GPUs. But the part that's really cool, this is the thing that like I geek out on, right? Is when you think about getting deeper into the development and stack. [00:26:30] Mark Heaps: And you're trying to set up GPUs as a system. And I'm talking, large data center scale systems. You've got all of these schedulers and things that you have to manage with the GPU and the data bouncing around in the way that it does being multi core and using all these schedulers it's really, what slows it down. [00:26:46] Mark Heaps: It's really what gives it a latency ceiling. And with the design of the Groq chip, and if anyone's seen a picture side by side it's beautifully elegant. It's it's works in a way that when you connect all of these chips together, you could put thousands of them together, actually, and it will see it as one brain. [00:27:06] Mark Heaps: So let's say that you realize for your workload you need 512 chips. You can tell that, hey, I need you to be one chip. and load your models that way. Or if you wanted to run some things in parallel, like we've done with an application we have called Groq Jams that writes music in independent tracks, linear and parallel to each other. [00:27:26] Mark Heaps: So that they're perfectly synced, we can say no, make those chips eight chips because I want eight instruments. So I'm gonna use eight instrument models to do that. You can literally do that with one line of code in PyTorch and you can refactor that way. And so this is, the advantage that they've had with the way that they approach the chip design and that in itself was the, probably the most radical thing that Jonathan and the team were the inception of. [00:27:50] Mark Heaps: They decided instead of designing hardware and figuring out how to improve hardware in a traditional methodology, they said no, we're going to start with the software. We're going to actually design our compiler first, and then we're going to design the silicon architecture to map to that, so that it's completely synchronous, so that it's completely deterministic. [00:28:10] Mark Heaps: We're going to build the compiler first, and we're going to make it so that no CUDA libraries ever need to be used. That you don't need to use any kernels. We're just gonna, we're just gonna bake it all right in. And so this is where we've seen a lot of that efficiency gain and where we get all that extra power for low latency. [00:28:28] Mark Heaps: And that's really been the fun thing, for anyone that's, that isn't familiar with us. Our early demos weren't AI related. In fact, during covid we worked with one of the national labs and they had a model. that they were using to test drug compounds against proteins and seeing what drug would stick to a protein. [00:28:48] Mark Heaps: And, this was in an effort to try to find a vaccine, etc., during COVID. And their model at that time, from what the team told us there, was it would take three and a half days for them to get a result. Every time they put a new drug in, see if it sticks to the protein, okay, did it work? If not, move to the next one in the queue, and let's keep going. [00:29:06] Mark Heaps: And that was this effort of trying to figure out, what would work. It took us maybe six months back then, because we weren't as mature with the compiler. It took us about six months to get them actually having their simulation running on Groq. When they finally did it, they could do that same simulation in 17 minutes. [00:29:23] Mark Heaps: So imagine the rate of acceleration to try to find a drug that could actually change the world at that time of crisis. They could do that on Groq in 17 minutes. So the orders of magnitude that we've been able to help people. is, has just blown us away. We've done some things in cybersecurity with one of our customers in the U. [00:29:39] Mark Heaps: S. Army. But now what we really realize is it's going to change the world for anybody that can take advantage of linear processing. And language is the ultimate linear application, right? You don't want to generate the hundredth word until you've generated the ninety ninth word. And Matt's example is amazing. [00:29:56] Mark Heaps: Imagine that you can generate a story. You did it with generating a video after having the prompt being generated. My kids, I have a 12 year old son, he's a major gamer, and I showed him using Thappy, which is a voice tool online for generating voicebots. I showed him how to make NPCs with that, and putting in character personas with no code, and it's running on Groq. [00:30:18] Mark Heaps: And the low latency, he was having a really natural conversation, and he told me, he goes, Dad, I can't ever talk to Alexa or Siri or any of these again, he goes, it's so bad compared to this. So it's just a really exciting time and the secret sauce of it is the chip. [00:30:32] Alex Volkov: that's incredible. And I think you touched upon several things that I want to dive deeper, but the one specific thing is necessarily the voice. conversations, the embodiment of these AIs that it's still uncanny when you have to wait 800 milliseconds for a response. And I've seen like a YC demo of a company and somebody said, Oh, this is like the best thing ever. [00:30:55] Alex Volkov: And it was like 100 milliseconds to an answer. And I'm looking at these 500 per second tokens. I'm thinking, This is like a near instant answer from a person and probably a super, very smart person, probably faster than a person would actually answer. And it it triggers something in my mind where we're about to slow these down on the UI level because the back end is not, is going to be faster than people actually can talk to these things. [00:31:19] Alex Volkov: Nisten I see you're unmuting. Do you want to follow up? Because I bet you have a bunch of questions as well. And we should probably talk about open source and models and different things. [00:31:29] Nisten Tahiraj: Yeah, so the one amazing thing here that we don't know the number of, so if the engineers could find out, there's something called the prompt eval time, or there's different terms for it. But for example, on on CPUs, that tends to be pretty slow, almost as slow as the speed of generation. On GPUs, it tends to be ten times higher or so. [00:31:53] Nisten Tahiraj: For example, if you get an NVIDIA 4090 to generate stuff at 100 tokens per second, or about 100 words per second, for the audience, the speed at which it reads that, and it adds it into memory, it's often in about a thousand or a few thousand. What I'm wondering here is that evaluation speed That has to be completely nuts because that's not going through some kind of memory That's just it goes in the chip. [00:32:21] Nisten Tahiraj: It stays in the chip. It doesn't spend extra cycles To go outside into memory. So The prompt eval time here has to be completely insane, and that, that enables completely different applications, especially when it comes to code evaluations, because now it can it can evaluate the code a hundred times against itself and so on. [00:32:45] Nisten Tahiraj: So that's the amazing part I'm wondering here, because you can dump in a book and it'll probably Eat it in like less than half a second, which is pretty, it's pretty nice. So yeah, one thing I'm wondering is how does this change the the prompt evaluation time? And what kind of other demos or stuff are actual uses, actual daily uses are you hoping to see? [00:33:08] Nisten Tahiraj: And can you tell us a bit more as to what your availability is in terms of to production and and [00:33:15] Mark Heaps: server load. Yeah, absolutely. I think the first one, I want to be a little [00:33:20] transparent about, where Groq was at in regards to the input. When we first started building out the system and optimizing it, we really focused on token generation and not input, right? [00:33:32] Mark Heaps: So that's where we thought everybody was focused. It's like Gen AI was blowing up everywhere. What can you make, what can you generate? And so we said, okay, the compiler team is working on things. Let's focus on optimization of the system, the LPU Inference Engine at generation. And so we got this wildly fast speed, right? [00:33:51] Mark Heaps: And I remember some people saying, oh, you'll never hit 100 tokens per second. We hit it, we did a press release. The team literally came back to us two weeks later and said, hey guys, we just hit 200. And I was like, what? And then all of a sudden we hit 300 and we're like, wow, we're generating really fast. [00:34:04] Mark Heaps: And then we started meeting with some of these benchmark groups, like Artificial Analysis and others. And they were saying no, like industry standard benchmarking ratios right now is 3 to 1 input to output. And we went, oh we need to start optimizing for input. And so we've started working on that. [00:34:21] Mark Heaps: And even that right now isn't at. The exact same speed optimization of our output and the teams are working on that, at this time, but it's more than capable and it's on the roadmap, it's just a different focus for the group. So we're probably going to see over the next few months about another 10x on the input speed which is going to be wild, right? [00:34:42] Mark Heaps: Because now when you talk about conversation, a lot of the time humans blabber on, but you tell an agent to respond in a terse and succinct way. Now you completely flip and invert the ratio of what you're going to be able to have. So that's really exciting. And, from a use case standpoint, I actually had a really interesting use case that, that happened to me personally when I was on a vacation with my family late last year. [00:35:08] Mark Heaps: We were actually traveling and we were in Puerto Rico lionfish. And it was really bad. We were like a hundred yards offshore. We're at like 60 feet deep water and I'm trying to help him get to shore and he's like screaming and I get on shore and the first thought in my head was of course call 9 1 1. [00:35:25] Mark Heaps: And I went, Oh my God, if I call 911, I'm going to get an operator. We're in this place that nobody can drive to. They'd have to helicopter us out. I was totally freaked out. And I ended up just going into the bot and saying, what do I do if someone gets stung with a lionfish? And in less than a second, I had a 10 step guide of what I should do. [00:35:41] Mark Heaps: Things that I didn't know, right? Oh, keep his foot in the water. Don't rinse it with fresh water. That happened instantly. Now imagine the world that, that goes from having an emergency Band Aid or burn kit in your house. to having an emergency bot in your house who can help you in those situations. [00:35:57] Mark Heaps: And so the speed at which it can read the input message and then give you advice back in the output is a complete game changer. And I think Alex nailed it, like we've seen all these comments where people say why do you need to generate this fast? They think of it as like a chat bot only or like a reading only situation, but the reality is, and what we've known for a long time is there's going to be an ubiquity of digital assistants. [00:36:23] Mark Heaps: And I don't mean like an individual bot per se, but just AI being everywhere to help you. And so that's going to require a massive amount of speed. for you to be able to slice that up across all these services. Like we hear, people building with their demos like Alex said earlier. So that's our goal to serve that. [00:36:44] Mark Heaps: And, Nisten, you asked about, what's the goal. Right now, again, just being candid with everybody, we didn't expect this thing to go viral. This was not a marketing strategy. This wasn't us going out and paying a bunch of influencers. It just happened and so the system has been like really tested and the amazing thing is it's held up like Matt said. [00:37:04] Mark Heaps: And so kudos to the engineering team for that. Where we're headed and our goal is by the end of the year we want a token factory to be able to generate millions and millions of tokens per second as a capacity. And so that's the plan right now. We want to be in, roughly 10 months. We want to be where OpenAI was, at the end of last year. [00:37:27] Mark Heaps: That's our goal right now. So we have those orders placed, that hardware is ordered, and we're building and increasing the capacity every week. [00:37:33] Alex Volkov: That's awesome. And so let's talk about models. You guys are serving LLAMA270B. And we hear rumors about next LLAMAs at some point soon. And I think Mark Zuckerberg even actually said that like they finished training LLAMA3 or something. We don't have insider knowledge here. [00:37:48] Alex Volkov: We're just like speculating. And then also obviously Mistral is releasing incredible models. You guys have Mixtral in there. There's speculation the Mistral Next that LMCs has access to is this incredible model, the GPT 4 level. So you guys are relying on open source models, and those models are trained on other hardware. [00:38:07] Alex Volkov: Do you guys also have training built in, or is this only for inference? And what are the plans for also training models? Because, speeding up training would help the world at least as much as speeding up inference. [00:38:18] Mark Heaps: Yeah. So let's tap into a few of those. So first, we love the open source community. It was a big inspiration why Jonathan left Google, where he was wildly successful. and said, we need to go start another company. And he wanted to make sure that the world and the developer community had access to AI technologies to accelerate development. [00:38:38] Mark Heaps: He literally calls this the haves and the have nots. And at that time, he said, look, it looks like Google, Amazon, Microsoft, a couple of governments are going to swallow up all of the AI technology in the world. He's that's not going to be fair. He's we need to democratize AI and access for all. [00:38:55] Mark Heaps: And so let's make a chip, and I remember him telling me this four years ago, he goes, I'm going to create a company where people can literally have access to the most advanced AI in the world, and do it with a credit card from their home. He goes, that's what I want to see happen. And so that's always been his vision. [00:39:11] Mark Heaps: And, we're on that path right now. The models that now the explosion of the open source community, and I think Meta deserves a lot of credit here. Chad GPT was blowing up, OpenAI was doing their thing. [00:39:22] The Unexpected Success of Llama 1 [00:39:22] Mark Heaps: And Meta, which is, obviously a massive corporation and private and in it to make money. [00:39:28] Mark Heaps: They said, no, we're going to make Llama available to everybody. And we didn't have a relationship with them. I think everybody knows Llama 1 got leaked and one of our engineers got ahold of it and said, Hey, I'm going to see if I can fit this to the chip. It wasn't even on our roadmap. And then they got it running in less than like 48 hours. [00:39:45] Mark Heaps: And then from there we advanced on it. And so that was an amazing moment. Lightning bolt moment where we said, Hey. What else can we do with this? [00:39:52] The Evolution of Model Compilation [00:39:52] Mark Heaps: And at that time, I think we had maybe 200 models from Hugging Face compiled for our system. And today, I think we're well over 800. [00:40:02] Mark Heaps: And we just keep pulling from the repos there and building them into the compiler. But we're watching very closely now of what are the models that people want? We had Vicuña up for a little while and we saw that on The LMSS leaderboard we've played with Mistral 7b. [00:40:16] Exploring the Power of Mistral 7b [00:40:16] Mark Heaps: If anybody wants to see real speed, go watch my video on YouTube on the Groq channel about Mistral 7b. It gets over a thousand, it gets over a thousand tokens per [00:40:24] Alex Volkov: you serious? Wow. [00:40:26] Mark Heaps: Yeah, I, the max I've hit with it I was just doing a conversational bot with it, and I hit 1140, and it was insane. [00:40:34] The Excitement Around Google's Jemma [00:40:34] Mark Heaps: And, now there's this announcement from Google about Jemma, which I think is like 8 billion. [00:40:38] Mark Heaps: And the team is already Oh my God, what could we do with Gemma, at that size, like the speed is going to be, through the roof. And then Jonathan, our CEO, is traveling right now, and he was actually at the Mistral headquarters in France a few days ago. And they were talking to him about, the next model and kind of what that looks like. [00:40:58] Mark Heaps: And he very much wants that to be running on the LPU inference engine at Groq. [00:41:02] The Future of Groq's LPU Inference Engine [00:41:02] Mark Heaps: So it's an exciting time to get into these open source models. And we're just happy that we can sit back and say, Hey, how do we help you guys? Because ultimately the people building the models, doing the training. [00:41:13] Mark Heaps: We want to enable them with this speed. [00:41:16] Groq's Stance on Training [00:41:16] Mark Heaps: You asked a question about whether we do training. We don't. We don't offer training. We don't do training. We have had one customer actually do it. That was related to that U. S. Army cybersecurity project. They actually trained their quantum algorithms using Groq hardware. [00:41:30] Mark Heaps: But it's not something we do, and it's not our business model. And Jonathan has always had this vision. He said Look the world already has a bazillion training providers, and [00:41:40] most people are quite comfortable with the pace of training, and this is going back to 2016, 2017. He said let's recognize that if all these companies are training models, and yet there's no real clear winner in the inference solution, let's just focus our business efforts there. [00:41:55] Mark Heaps: He does have a vision. It's not on our roadmap right now, but he does have a vision. [00:41:59] The Potential of Live Training Through Inference [00:41:59] Mark Heaps: of what you could do with this sort of recyclical live training through inference, where it's actually being trained live in the moment and feeding back to itself, right? And this gets you into a multitude of layering techniques that we've been considering and testing at Groq. [00:42:14] Mark Heaps: I could see us getting into training in the future, but only when it is advantaged by that real time insight of training. [00:42:22] Alex Volkov: Up here. And Nisten, just before, let me jump in super quick. I want to follow up with something that you said that 7b Mistral is flying at over a thousand tokens a second. And that's obviously incredible. Just like mind blowing incredible. And in my head what I'm super excited by is not the smaller models, because I can run the smaller model on my Mac with 20 tokens, 30 tokens a second and get like a full whatever. [00:42:45] Alex Volkov: I'm excited about the incredible intense, long context requirements that we've seen. So we had talk about open source. We have often the folks from Nous Research here on stage, the authors of the YARN paper, that they've been able to take LLAMA's 4, 000 contacts window and extend it to 128. [00:43:03] Alex Volkov: And we never used it. We never were able to use LLAMA at 128k tokens because it was like extremely slow. [00:43:09] The Power of Groq's Speed in Long Context [00:43:09] Alex Volkov: And I'm thinking about Are you guys bringing us long context, like for real, like for open source models, because we haven't yet been able to actually use them as much. Because the bigger the model is, and the faster you can run, it will average out, and we'll be able to get open source models. [00:43:22] Alex Volkov: Have you guys played with long context yet? Have you seen the incredible stuff from, Gemini 1. 5 releasing 1 million tokens, for example. Something that probably only Google can pull off with their TPU farms. How are you thinking about that as an advancement, as a competitive edge for something that only you could do? [00:43:37] Mark Heaps: Yeah, the team is actually looking at that right now, and I think, again, early stages, our first 4A into a larger length was actually, Mixtral with a 32k sequence length. And, so far we haven't seen any use cases where people are actually taking advantage of that full length, but we know that it's coming. [00:43:54] Mark Heaps: And the moment that Gemini 1. 5 got announced with the million token length, the team immediately got together and said, okay, how would we do this? And they've started architecting. What scale of system would we need for that? So that's part of the plan in parallel with what I was saying earlier that we really want to get to a place where we're this massive token factory by the end of the year. [00:44:14] Mark Heaps: And that's getting us into that, more than 10 million to 20 million tokens per second from the system in that capacity. So we're definitely looking at that. I think what's going to really dictate it for us, because we're again, sitting back and saying, how do we help? And what we're watching is what are the business use cases? [00:44:33] Mark Heaps: So if someone says, Hey, we want to use a model that has a million million contact sequence length. But you find out they're really, on average, only using 50k for their application. This is that advantage I was talking about earlier, where we can dial the system forward or backward using a single line of code. [00:44:50] Mark Heaps: We can figure out what is that link that they need, and then dial that in for that customer account. We're actually doing a little bit of that right now with Mixtral. You guys mentioned, we have the free version. on our website that people can play with through Groq chat. And then there's the API access, right now, as everyone's playing with it and just treating it as a chat agent, we're recognizing that we've got this thing loaded for 32 K Mixtral. [00:45:12] Mark Heaps: And yet, the average we see being generated in GroqChat is around 900. At that scale, we're like, hey, why don't we increase the capacity of the system, speed this thing up a little bit. Let's drop the sequence length for the free GroqChat service. But leave it at the longer sequence length for the API users, and that's really easy for us to do. [00:45:32] Mark Heaps: That's flipping a switch in, in, in some ways. [00:45:36] The Importance of Community Feedback [00:45:36] Mark Heaps: So we're just waiting for the open source model community to really tell us like, Oh, this is the size that we could really take advantage of. [00:45:43] Alex Volkov: Awesome. So you guys found the right place. The open source model community often ends up on ThursdAI and talk about their advancement. So I'd be more than happy to introduce you to the guys who are doing open source kind of papers on long context as well. They're often joined here and they would be very happy to like help and figure out what's the, what's possible, especially because training those models is hard, but then running inference is even harder. [00:46:07] Alex Volkov: Nisten. [00:46:08] Mark Heaps: Way harder. [00:46:08] Alex Volkov: Yeah, Nisten, go ahead. [00:46:11] Nisten Tahiraj: Yeah, so one thing I'm wondering about is, so first of all, it's extremely impressive that these models are running at full precision and they're not even starting to take advantage of some of the handmade stuff that people made to get them down to the, to phone size and to still perform well, because that takes yeah, so that hasn't even been explored yet, because that can reduce the size by four and have exponential improvements. [00:46:36] Nisten Tahiraj: So what I'm. wondering is, how much, as you guys expand and as you go and as you adopt, whether you adopt our models or not, how much work is it to Take something like LLAMA or Mixtral and then adapt it to more of your JAX like stack That you guys have. So yeah, that's the part that I'm Wondering about like how much work is for companies to adopt their own models or if they have something custom that they've made to this because I see some incredibly interesting stuff and I think for Sorry, I'm rambling on a little bit, but I think even for training you can make models that fit under 220 megabytes or model parts, and then you can train those individuals. [00:47:22] Nisten Tahiraj: So there is stuff to be it. Explore there. I just think there hasn't been enough yeah, it's still pretty new, so there hasn't been enough people taking a crack at it. But yeah, how much work is it to take an open source model or a custom something that people made and to adapt it to work on Groq's hardware? [00:47:40] Nisten Tahiraj: That's my question. [00:47:41] Mark Heaps: Yeah, it's a great question. Thanks, Nisten. Yeah, so I think a really good paper everyone should check out if you're interested in this, if you go to Groq. com slash docs. We've got a huge doc repo there. And one of the earlier articles that we produced from the compiler team is called Developer Velocity, and it's been a, it's been a focus from day one. [00:48:00] Mark Heaps: We did some research when we were building out the product, building out the service, and we found out that for a lot of companies to get a model up and running, especially if it was their model. It would take them, if you were a smaller company let's call you, an SMB, sub 5, 000 employees. [00:48:15] Mark Heaps: They were typically spending six to nine months to get a model into production where they were using it. The larger companies, Microsoft, those guys, they're doing it in 30 to 45 days. And so we set this goal saying, we don't want any customer ever to need more than a week to get their model up and running on Groq. [00:48:34] Mark Heaps: And ideally we'd like it to be in 24 hours. We're actually going to test the team on that when LLAMA 3 gets released. We're going to see how fast from the day everybody has access to it, to how fast can we get it up and running. And, I'm hopeful we're going to, we're going to see a demo with it literally that day or the next day. [00:48:49] Mark Heaps: It's not a lot. We're using standard frameworks, right? So we're PyTorch, Onyx, Tensor, everything is pretty standard. The thing that we spend a lot of time doing this in, and this is what slowed us down a little bit when Llama 2 came out I did a video with Bill Ching, a member of our compiler team. [00:49:06] Mark Heaps: He's a brilliant guy, super funny. He'll tell you in the video, I didn't spend time getting it to fit to Groq. I spent time removing All of the code and components that were built in for GPUs. Basically, he spent time scrubbing, not, building. And that's what happens is because the community is so already weighted towards building for GPUs, that's what takes us the most time. [00:49:30] Mark Heaps: We've got to strip all that stuff out because it slows it down. Again, we don't have those schedulers. We don't have those components. That's the biggest thing for us in the way that, that we get things running. But, even custom models that we've had from the national labs and the research groups, we had one that was for the Tokamak nuclear fusion reactor. [00:49:48] Mark Heaps: It was a control system. And even that we got running in just, I think it was less than 10 days. And it was a completely custom build and our compiler was no more mature at that time. Again it's one of those [00:50:00] things that our goal is to get it down to where it's same day applicable. [00:50:03] Mark Heaps: We're a ways off from there, but right now we're trending less than a week for everybody. [00:50:09] Alex Volkov: Mark, I want to follow up with the use case. As you guys were talking about converting models, and we see models getting released from all these finetuners. We have a bunch of folks here who finetune models after open source release, and many of them switch to Releasing their models in the safe tensors format, the standard one, but also in the quantized format that people can actually download the smaller quantized versions and run them on their Macs. [00:50:33] Alex Volkov: And I can absolutely see if you guys support this, I can absolutely see a day where folks are releasing it also on Grack or Grack chat or whatever, just for folks to be able to experiment with like longer context. As a fallback, sorry, as a follow up on the longer context one session, you said. we see in the chat. [00:50:49] Alex Volkov: Yeah, the chat is not optimized for, pasting like a bunch of stuff. I, I would I would not suggest, I would be cautious about judging by that because I personally, if I get access or I guess I got access to the API, but when I get access to longer context, for example, I would absolutely think about, hey, what is possible now? [00:51:08] Alex Volkov: I can, and somebody commented in the comments that coding is the main use case where long context really matters. Because what happens right now is everybody's like focusing on rag. And we had this conversation, rag versus long context, I think since a year ago, since the context lengths were 4, 000 tokens, then 5, 000, then 12, then whatever. [00:51:25] Alex Volkov: And then Mosaic came out with 60 and we were very excited. And we had this conversation since then of what performs better. And I think one of the two main reasons that folks And I don't know about cost, and we probably should talk about cost, but infraspeed, you guys are doing some incredible advancements. [00:51:46] Alex Volkov: In my head, as somebody who builds systems with this, as somebody who plays around with this, if I can shove my whole codebase In the context, I will get a better answer than I'm gonna have to embed the context, the code base, and then try to do retrieval on specific chunks, whatever. I'm even thinking about the cursor interface that I used yesterday. [00:52:03] Alex Volkov: I, I had to provide it with, I had to mention, hey, these docs that you already vectorized, add them to, to the context, so GPT 4 will be able to help me solve my specific issue. If my whole repo is getting sent in each prompt, I don't know if this is the best use case of your hardware, but it's definitely the, probably the fastest way to get the model to actually know exactly what I want. [00:52:23] Alex Volkov: That's one example. Another example is all these models, all these agents are going towards personalization. I definitely think that this year is the year of personalization, especially with like longer context and models like Gemini 1. 5, for example, they have a full retrieval precision, almost like 95 needle, in a haystack recall ability. [00:52:42] Alex Volkov: And that, for use cases like something like a personal assistant that remembers everything about you, removes the possibility of, hey, I didn't chunk correctly, I didn't do rack correctly, I did vector similarity incorrectly, etc. For developers just getting up and running and building tools like this, I think long context is still yet to be discovered because it's still expensive and it's still slow. [00:53:02] Alex Volkov: And I think speed with a lot of context is what's going to unlock the next iteration. So those are just like some feedback from the community staff. Would love to hear what you think. [00:53:10] Mark Heaps: Yeah. So first, I love these ideas, and I want to invite everybody who's listening go join our Discord server, because we want this feedback. We, the product team is super hungry for it. We want to know what you guys want. So definitely go do that. It's Groq. link slash discord. Please bring all these ideas to us. [00:53:26] Mark Heaps: It's an interesting thing, Alex, because we've heard this from a number of customers of, do you do RAG? Do you do some form of vector database? We get asked about Lang chain. We get asked about all these things. And I think for us, there's this risk of where is the infrastructure, that part of the stack with RAG, where is it? [00:53:44] Mark Heaps: Where does that exist, right? So if you're operating in these two totally, vast separated areas, you run the risk of losing your latency just because of the network and kind of what happens between them. So for a lot of folks, we hear no. We want the longer sequence length because we want to embed a lot of this in the sys prompt. [00:54:03] Mark Heaps: And we know that Groq has such fast inference that if it's embedded there, it's all living with you. And we're going to be able to maintain that speed. If you start calling out to a bunch of different rag services, where am I going to lose? Now, I think that's thinking that's based on the experience they've had with GPUs, OpenAI, ChatGPT, etc. [00:54:23] Mark Heaps: But, for us, if we have such a margin of inference speed, we haven't seen anyone really lose on the overall experience performance because of the network topology. Jonathan was doing a demo for somebody literally using Wi Fi on a United Airlines flight where we had information in a rag and he was calling it, using Wi Fi on the plane. [00:54:48] Mark Heaps: And he was like, it was a very normal speed experience. He was disappointed because it felt he was using ChatGPT, [00:54:53] Mark Heaps: For the person there, [00:54:54] Alex Volkov: It's hard to go back after, after you experience immediacy. Waiting is definitely annoying. That's I'm waiting for the hedonistic adaptation of ours to kick in where we expect immediacy. Yeah, sorry, please go ahead. I have to chime in. [00:55:06] Mark Heaps: No. Yeah. No, I think you're, I think you're spot on. So yeah. So again, we don't want to dictate to anybody You know, what is the best method? We want to listen to you guys and figure out how do we continue to serve in that way? And, the other reality is there's gonna be new techniques that are gonna be invented, in the next couple of months probably, that, that give you a whole nother option, around rapid fine tuning. [00:55:31] Mark Heaps: And we're just watching. And listening to you guys, but we recognize we need to enable both. So we're working with some partnerships for RAG right now to be able to connect into Groq. And there's going to be some announcements actually tomorrow about some things happening at Groq that I think people will be excited [00:55:47] Alex Volkov: Ooh, you want to give us a little teaser, a little laugh, or are folks going to tune in for tomorrow? We gotta tune in for tomorrow. [00:55:54] Mark Heaps: I I think the only thing that I'm allowed to say is there's really going to be a very strong representation of the developer community. Within Groq, and the tools that we're gonna start rolling out over the next couple of weeks are really gonna feel familiar and hyper supportive of the work that y'all do. [00:56:11] Mark Heaps: So it's gonna be, it's gonna be really fun. [00:56:13] Alex Volkov: Alright, so folks, stay tuned, definitely we pinned the discord link to the top of the space check it out and give folks comments because you guys have a bunch of headroom and we need to use this, but we need to tell you in which way we're gonna use this so you also have it. a roadmap, you have prioritization issues like every company, you have to focus on something. [00:56:30] Alex Volkov: So the better folks will give you feedback, the better. I want to maybe one last question, Mark, before I let you go, and then continue with the regular thing, which you're more than welcome to stay and chime in as well on, because I did see your thread. [00:56:41] The Potential of Multimodality in AI [00:56:41] Alex Volkov: I think you're also interested in the broader AI community. [00:56:44] Alex Volkov: It's multimodality for 2024. I think It's clear to everyone that multimodality is built in. All the major labs are now multimodal. I think multimodal AI is in open source is coming as well. We have folks here who've trained multimodal models. What are we to expect from Groq on that perspective? [00:57:01] Alex Volkov: Is it? Do you guys already have support for some like a vision plus plus text? Are you looking at different things like, video as well, which by definition takes more tokens and then slower by definition in every other place? How is the team thinking about this kind of next evolution of Gen AI? [00:57:19] Mark Heaps: Yeah, good question. Obviously, multimodal is where everyone's interested. And I think ever since OpenAI gave ChatGPT the capability to generate images in the middle of the conversation and then add audio into the middle of the experience, everyone's been excited about this idea. And certainly that's where we've started. [00:57:37] Mark Heaps: We have a plan we call them the three pillars, right? And it's where does Groq add this speed value in? Language in audio and in visual. And what we're looking at right now is what are the models that we can bridge together so that we can provide that multi modal experience. The systems teams are already preparing the LPU inference engines that we're expanding on to be able to handle that. [00:58:03] Mark Heaps: The compiler teams are actually, have already begun building out some of the advancements we need to be able to support that. We know where it's going and we know, that's what people are going to be asking for. So I've only shown one other thing. on our YouTube channel, which was a model that [00:58:20] Adobe gave us, which was a style GAN, and that was 8 models that run in parallel, and I think it generates in like 0. [00:58:28] Mark Heaps: 186 of a second at 1024 pixel resolution. We can literally say, here's an image, give me 8 completely different styled results based on that, that diffusion model or that style GAN model. And that's where we've started playing with image generation. We do have some people that are looking At tiny diffusion and a few of these other like rapid generators that are small. [00:58:47] Mark Heaps: But certainly that's something that we intend to support. It's the problem now with the speed of all these things happening is what do you prioritize? We are a company of, less than 200 people. And we're trying to, we're trying to figure out every day, like, where do we commit our resources? [00:59:02] Mark Heaps: So again, it sounds like I'm trying to be like a marketing guy and I'm not. Go to the Discord and tell us what, you guys want. What are your use cases? What are you predicting with your businesses? That would really help us to be a part of the, to be a part of the conversation. [00:59:16] Mark Heaps: But at the high level, yeah, we already have people working on it. [00:59:19] Alex Volkov: Awesome, and I definitely invite your folks to also join the ThursdAI community, because we talk about these advances as they happen, we've been talking about multimodal, multimodal since almost a year ago now, folks, everybody in the audience, we're going to celebrate ThursdAI's birthday, I think, in a couple of weeks, and [00:59:36] Mark Heaps: Nice, that's cool. [00:59:37] Alex Volkov: when GPT 4 came out they had the infamous demo where Greg Wachman jotted down on a napkin, a UI thing, and uploaded it to the GPT 4 with Vision, and we've been waiting for this to become a reality ever since, and I think it's now becoming a reality. [00:59:51] Alex Volkov: We also chatted with, the folks from Reka AI, which, had the multimodal model out there a couple of weeks ago that I was blown away by. I was uploading videos of mine and it understood tonality in there, understood like what happened in the video. We obviously see video being a big part of Gemini 1. [01:00:08] Alex Volkov: 5, we're going to talk about this soon, where people just upload and that video just takes so much content, like 600, 000. tokens in context. But then the model understands like every little frame and can pull individual scenes away. And once we get to real time video understanding, that's when the actual World embodiment of these bots will happen when like it can actually see what and can react in real time. [01:00:29] Alex Volkov: So definitely exciting stuff from there. And Mark, I just wanted to say What an incredible week you guys had and it's been great to just see how this explodes and play around with the possibilities I'll remind folks in the audience. I've played and it's on the it's on the show notes in the Jumbotron I played with Groq yesterday and it was I was able to build something that I wasn't, thinking about it's possible a few months ago, even. [01:00:54] Alex Volkov: It's so fast. And you already mentioned the Discord. How do people get access? Is the wait list long? Tell us about people in the audience and then the questions. The one API access . [01:01:03] Mark Heaps: The waitlist is really long right now, and it blew up this week. Again, thanks Matt for, and others for promoting. Yeah, so right now they can go to Groq. com. They'll see a link on the left that says API access. You fill out a brief form right now. We are trying to get through that list as quickly as possible. [01:01:20] Mark Heaps: There's a timed trial, the usual sort of terms. But in a week, it wasn't even a week, it was literally within 37 hours, we had over 3, 000 API access key requests. And so that was more than we had expected. And so we're trying to get through that list right now and see what the tier levels, some people are telling us we need a billion token per day access. [01:01:42] Mark Heaps: And we're saying, okay, this is this tier level. And other people are like, hey, we're part of Y Combinator's startup accelerator group. We're just testing our bot ideas out, can I get free access? So we're working through that list right now. The good thing is. We are increasing capacity every week, and one of the announcements that we'll have tomorrow and rolling into next week will be moving more towards self serve versus us going through and like manually approving everybody, so that should accelerate approvals greatly. [01:02:10] Mark Heaps: I just ask everybody be patient. If you've applied, stick with us. We promise we're going to get to you. We really want you to have access to this. This level of inference speed but this whole virality moment came out of [01:02:21] Nisten Tahiraj: nowhere and we, [01:02:23] Mark Heaps: We're trying to meet the needs now. [01:02:25] Mark Heaps: So just stick with us. It's going to keep getting faster and faster. [01:02:28] Alex Volkov: Incredible. So folks, definitely check out GroqChat. If you haven't yet it's quite something. It's quite incredible. Check out all the demos as well. And with that, I want to say, Mark, thank you. This is the end of our conversation. It's been an hour, folks, on ThursdAI, and I'm going to reset the space a little bit, and then we're going to talk about everything else that was new this week, and there was a bunch of stuff in the open source and in different places. [01:02:49] Alex Volkov: But what you heard so far is a deep conversation with Mark. Mark Heaps from Groq which came to many of us as new, but was around for a while. And then we also had some folks in the audience as well listening to this from Groq as well. So that was great. Thank you, Mark. And then let's reset the space and start talking about what's new in AI this week. [01:03:07] Nisten Tahiraj: Thanks so much, guys. Really appreciate [01:03:09] NA: you. [01:03:31] Google releases Open Weights for GEMMA 2B and 8B [01:03:31] Alex Volkov: All right, how's it going, everyone? You're on ThursdAI, February 22nd. My name is Alex Volkov. I'm an AI Avenger with Weights Biases. And Yet another incredible week in AI with a bunch of other stuff and I want to move our conversation towards the kind of the explosive open weights news this week, and I would love, so we have some more folks on stage here, and LDJ, we've talked about this when it came out, but Google gives us OpenWeights models, this is new to us folks, we've been waiting for Google for a long time, and finally they come out, and Google releases Gemma, a new OpenWeights model, not open source, and they've been very clear, which I really applaud the team. [01:04:12] Alex Volkov: We're going to talk about some stuff that Google did not exactly do correctly this week, but we're also going to, we're going to highlight like we're going to give props where props are due. Google is clearly talking about open weights, open access model, not open source because they didn't open source a bunch of stuff. [01:04:26] Alex Volkov: Definitely not datasets. It's called Gemma. It's they released two, two sizes, 2 billion and almost an 8 billion. So 7 billion parameter model. It has. Let's see what's interesting there. Trained on 6 trillion tokens, 8000 context window interestingly, vocab size is way bigger than LLAMA, and if you guys have been falling under capacity from this week, as you should, he just released a whole conversation about tokenizers, and he then analyzed the vocab size of the tokenizer kind of for Gemma, and said it's way bigger than LLAMA1. [01:04:59] Alex Volkov: It's basically the same one, similar one, just like way bigger. And Yeah, this is incredible. This is like great news that Google is stepping into the open source. I think they see what Mark Zuckerberg saw, where once you release something like this, the community provides. And I want to just highlight, I had a tweet go off like fairly viral, because four hours after release, LDJ, we were spending the first hour in the space together that you opened. [01:05:22] Alex Volkov: Four hours after release, we had Lama CPP support, Olama support, we had LM Studio support. Many people, like Maxim Lebon, one of our friends of the pod, quantized upload this because they didn't quantize correctly. Then after half a day, 3DAO from together added support for Flash Attention. I think there's a bunch of other stuff that added support as well. [01:05:40] Alex Volkov: And we just had we just had folks from Groq talk about they've been looking at this as well. So it feels like Google understands the benefit of open weights access model. So I just want to, this shout out Google. Let me actually, I have a thing for this. Yeah. Good job. [01:05:56] Alex Volkov: The big G provides, and this is great, and I'm, I was really surprised and happy to see this in the morning, and I wanted to hear from folks here on stage what are your thoughts so far on Gemma in terms of performance compared to, let's say, Mistral or anything else like Finetune that we had. [01:06:10] Alex Volkov: Whoever wants to go next, but LDJ, you and I have the space, so feel free to comment what we learned from the space and since then, and then let's go around the table, and then we're gonna go forward with some news. [01:06:21] LDJ: Yeah, so I think what we learned on the release and also after a little bit of time of people using it is that pretty much it has around the same abilities as Mistral. You could say maybe a little bit better than Mistral in certain ways. Some people say it's at least a little bit worse than Mistral in certain other [01:06:40] ways. [01:06:40] LDJ: But overall there's definitely is maybe certain use cases where you might prefer the Jemma model. It is interesting though, I believe Jemma is actually. From what I remember seeing, it's 8. 5 billion parameters whereas I want to say Mistral is a total of 6. 7, so there is actually somewhat of around 25 percent more parameters, and theoretically, it should be maybe a little bit better than Mistral than than they say but, yeah it just really shows to how impressive Mistral is really the fact that Google's Making this model that's it's still not really significantly beating it, [01:07:17] Alex Volkov: It's quite impressive. I saw, I think Marco from A16Z, Marco Mercora, post comparisons from Gemma, Mistral, Lama and I think something else. It's quite incredible that this model, like a company less than 30 people 6 months ago they released it, no, like less than 6 months, September I think, or October, the 7B model, and it still performs well against a company with like billions or whatever, and they release it, it's quite stunning that they're not able to beat Mistral 7B. [01:07:49] Alex Volkov: by a significant amount. I wanted to like, highlight how, first of all, impressive this is, that they even released something. But also, how impressive this is for Mistral, that they come out so strong, and their model is basically the one people compare to. Definitely agree to that. [01:08:05] Nisten Tahiraj: Yeah, I used it quite a bit I My opinion, I don't like It just it's just not that reliable. So yeah, it can code but sometimes It's not a very obedient model and the thing about Mixtral and Mistral and stuff is that They're used like tools a lot and Yeah, but again, we have yet to see good fine tunes. [01:08:32] Nisten Tahiraj: So We see we saw how far people took alignment it with open chat [01:08:39] Alex Volkov: Yeah, speaking of OpenChat [01:08:41] NA: Was like how far they've taken these Yeah, so so we'll see I'll hold off a bit of judgment for them for now [01:08:49] Alex Volkov: Yeah, speaking of open chat and speaking about fine tuning and being able to fine tune this alignment what are your initial thoughts? I saw Alpay post something that new open chat is coming. What are you guys cooking a fine tune like what's going on? [01:09:03] Alignment Lab: There's probably an OpenChat fine tune of Gemma that's going to come out. I'm not clued in to that right now. I haven't had a chance to really get my head above water for a couple of days because I've been just buried in several things. If, if there is, it's probably going to be good. The model seems smart and it's got a lot of parameters, so It's hard to say that fine tuning won't make it very strong. [01:09:31] Alignment Lab: I think with that giant tokenizer, it's going to be worth knowing that the model's going to be able to do a lot more during the training run because it's going to see more granular patterns and have a more expressive vocabulary to to, exploit the way that training runs make a model perform well better. [01:09:50] Alignment Lab: This is the best way I can put it. It also, it's not getting mentioned very much, and I think it's because this is past the event horizon of AI stuff for a lot of people, but if you open up the models architecture, the implementation of it on the Google GitHub repo, they actually have a few different versions, and they're all for running the model in various contexts, or with or without TPUs, but And all of them, even the one that's not made to be parallelized, the model actually does have a baked in architecture designed for quantization and parallelization. [01:10:20] Alignment Lab: And it looks like it can be quantized, or it can be parallelized, horizontally, vertically, and whatever the word is for the third dimension. It looks like it breaks pretty evenly into eight pieces, and if you can break it into eight pieces, and quantize each piece, and dequantize each piece, You can maybe parallelize it across asymmetrical compute, which is the big holdup for why we can't distribute models over just a bunch of random servers. [01:10:48] Alignment Lab: Because usually, if they're not the exact same GPU with the exact same throughput and interconnect the model's unable to perform inference. But they may be able to solve for that baked in there, and it might be that they intend on Maybe having some service by which you can use the model locally with X amount of context and then just back into it onto their TPUs. [01:11:08] Alignment Lab: I'm not sure, but it's interesting that it has a lot of custom tooling like baked into it designed for quantization parallelizing [01:11:15] Alex Volkov: Yeah, I want to say custom tooling and also thanks Aliment, and also the amount of stuff that our community is supportive that they released is quite impressive. They released GDF quantizations, I think. They released support. They even released, folks, I don't know if folks missed this, they released something called Gema. [01:11:32] Alex Volkov: cpp. which is a local CPU inference based in completely C with no dependencies, which is in addition to Llama CPP adding support for this, there is Gemma CPP and that's like their whole complete kind of comparison to Llama CPP. And that was pretty cool of them to release. [01:11:49] Alex Volkov: And it looks like they've geared up to to have this model to be accepted. It's on Hug and Face. Hug and Face and Google recently announced a partnership and now it's on Hug and Face as well. So you can actually go to like hugandface. com slash Google slash Gemini slash Gemma. And it's pretty cool. [01:12:04] Alex Volkov: I remember they, they mentioned Gemini Lite or Gemini Tiny or whatever for local inference. Very interesting that's not what we got. We got like a new model called Gemma out of the gate. Yam, do you have any, what's your thoughts on this whole thing from Google? Do you have a chance to play with this? [01:12:19] Alex Volkov: Give us a little breakdown. [01:12:20] Yam Peleg: actually, yeah, actually fine tuning is on the way. Already got the GPUs warming up [01:12:27] Alex Volkov: let's [01:12:28] Yam Peleg: the data as we speak. Yeah, I'm going to do, I'm going to do, before fine tuning, I'm going to do a little bit of a continuous pre training just to see if we can squeeze a little bit more out of the base model. [01:12:40] Yam Peleg: It's just important to distinguish between the base model and the instruct tuning model. [01:12:47] Alex Volkov: That's the slash IT thing they released, right? There is like a Gemma and Gemma slash [01:12:51] Yam Peleg: When we talk about chat GPT like models, we talk about the instruct tuned models. And this, yeah, for sure, Mistral is just better at the moment. But in terms of the base model, we can know this only after people start to play with it and try to tune it themselves. [01:13:11] Yam Peleg: Then we can see how far we can push it, because maybe it's just the actual fine tuning that Google did to their version of the model and with the methods from the open source that are pretty much, uh, very well trained in fine tuning models for instructional fine tuning. Maybe we can, maybe this model is really, will be really great because at the end of the day. [01:13:36] Yam Peleg: The amount of compute that Google put into the model is insane, it's unparalleled. I'll be surprised if the model doesn't turn out to be really good, the base model, after fine tuning. But yeah, there is no, there is absolutely no doubt that Mistral is hiding something, they do have emotes. All their models that they fine tune for instruction following are on different levels. [01:14:03] Yam Peleg: You can say. And you can see this even with the NuCube, the one that shouldn't have, had been leaked. It is also really good. [01:14:13] Yam Peleg: But yeah, it's amazing. It's amazing that there is another player that is releasing, a major player, Google, releasing a really good Base model open source. [01:14:24] Yam Peleg: It's great. It's great to have more players in this field more corporates turning into this game, supporting open source. It's always great. Yeah. [01:14:33] Nisten Tahiraj: And the funny part is that they are struggling to compete in this section just because, the beauty of open source is that it enables so much competition, especially at these lower sizes where people can iterate very quickly. [01:14:48] Nisten Tahiraj: And and now this is extremely obvious in this case. But yeah, I also think that the base model, I only tried the instruction tuned ones, and I've posted it above. I even have a link if you want to try it, but. [01:15:00] There is a lot more to be squeezed out of that just because again of the quality of the data that went in the pre training and Google might just not be that good at making chatbots. [01:15:13] Nisten Tahiraj: Yeah, they'll probably, they'll get better, but it's [01:15:16] Alex Volkov: Nisten, is it mergeable? It's mergeable, right? Like it's Frankensteinable. [01:15:21] Nisten Tahiraj: Yeah, I think you can, I'll [01:15:24] Yam Peleg: do it for fun. You can merge it with itself, but we don't have models to merge it with at the moment, [01:15:32] NA: because you can't talk about it here yeah. You can merge the instruction tune with, not instruction tune, with itself and train on top. [01:15:39] Yam Peleg: I tried to extend it with the front end merge and it didn't behave nicely. Mistral, for example, behaved really well. You can stretch it three times, just copy the layers three times and it works really well. At the fourth time, it starts to, to disintegrate and just breaks. But somewhere, you can do it for 3x and it works really well. This model didn't, so it was a little bit strange to see. [01:16:08] Yam Peleg: But yeah, I'll know in a couple of hours when my training starts. So I'll be smarter to tell you. I if anyone saw my experiment I tried to play a little bit with with reinforcement learning, with DPO. I stopped the experiment mid run because someone pointed out that the terms forbid me to play with this type of experiment, but I just want to say that I played with, I tried to make the model less refusable, it was refusing nearly anything that you asked it, but so I just tried to make it more, more acceptable to actually do what you ask, nothing really fishy, but yeah, the terms forbid that, so I stopped the experiment. [01:16:51] Yam Peleg: I just wanted to say that it really resisted. I trained and trained and the model still resisted. They really went hard on the on the alignment part on this model. [01:17:02] Alex Volkov: Interesting that, we're going to talk about this next, I think, from Google, but interesting that even in their kind of open weights, open access models, they're baking in the alignment like super strong. Anything else, folks, on Gemma before we move on? Generally, kudos for Google for coming out this strong. [01:17:21] Alex Volkov: Gemini Ultra getting announced, and then we saw Gemini Ultra getting access then Gemini Pro 1. 5, which we covered a little bit, and we probably should talk about this a little bit more, and now we're getting like open weights models that are finetunable, and I think even commercially licensed, right? [01:17:35] Alex Volkov: You could use this in production, if I'm not mistaken. [01:17:42] Alex Volkov: I guess I'm not [01:17:42] Alignment Lab: Yeah, I think so. I think so. I think so. [01:17:45] Alex Volkov: Yeah, which is quite impressive. Even from, it, it took Meta a while to give us a commercial license. Microsoft released PHI without commercial licensing. And then after six months gave into the pressure and Google waited, and now they're like, ta da, here's this. [01:17:58] Alex Volkov: So very impressive from Google and kudos to whoever there worked on this open source release. It's probably not very easy to do, not open source, but open weights. It's not very easy to do. That stuff from within this big organization. So whoever listens to this, whoever worked on this, Thank you. Give us more. [01:18:14] Alex Volkov: We would like to see bigger models, 35, etc. Junyoung, you wanted to comment as well? I saw you step in here. [01:18:20] Alex Volkov: Yeah, [01:18:21] Junyang Lin: I am definitely very excited about the Google open source of the Gemma model because yeah, it's actually a great model. Yesterday, we were just trying to compete QWAM 1. 5 with Gemma SMB, but we found Gemma SMB is actually better, but when we try about the base model. [01:18:40] Junyang Lin: We think the base model should be a good model, but the instruction tune model, it's a bit strange. Actually, its behavior is quite strange. It's always irrefusable, and it's too safe, and there are a lot of answers they can't do. So I'm very surprised how they do their CAD model. But generally, the base model is general good. [01:19:04] Junyang Lin: But I I'm very interested about their choices of their architecture because that it, its site is actually, it's not 8 billion. It's actually 9 billion because they have input embedding and their alpha embedding layers. They are not shareable. Parameters. So you found that the the sidebar actually very large. [01:19:23] Junyang Lin: And for 2B, it is actually similar. It is actually, essentially three billion parameters if you count it correctly. So it's actually a very large model. And it is quite strange that for 2B model, it is using image MQA multi query attention, but for 7B model, it is Actually using multi head attention. [01:19:43] Junyang Lin: I don't know why they choose it. And if you carefully look at the side of the hidden side as well as the head dimension for the attention you'll find that for the attention layer the head dimension is 2 56 and with 16 ahead, which means that the. Actually, the hidden dimension for the attention is actually 1496, but the hidden dimension for the FFM is 3072. [01:20:11] Junyang Lin: This is very strange for me to choose something like this. I don't know, we should follow it for the following models. I don't know why Google do this. If they can tell us about this. it could be much better. But something it is very interesting and we also have experiments to show that it is quite effective, which is the large intermediate size. [01:20:34] Junyang Lin: You will find that the intermediate size in comparison with Lama models or Mistral models it is actually larger. So you'll find we, we have some experiment and find that the larger intermediate size can improve the performance but there are still a lot of things we don't know why Google did this and we're not pretty sure Gemma is really a good model, much better than Mistral because I have seen some evaluation from Anton I'm not pretty sure it, it seems that Mistral is still the better one. [01:21:05] Junyang Lin: I'm not pretty sure actually much better than Mistral, so, let's wait for more tests. [01:21:11] Alex Volkov: We'll wait for Junyang thank you folks who are not familiar with Junyang he's on the technical team at GWEN, and we've talked multiple times about this point, thank you Junyang and it's great to have you here. And definitely we'll see more fine tuning, base model seems to be fine tuned a bowl, Yam said he's already cooking something, probably other labs are already shaking their They're pounds in anticipation of how to use the open source stuff, the DPO stuff. [01:21:33] Alex Volkov: If it works to actually make this model behave instruction fine tuning better than Google did. And I'm sure that it's possible because we've seen a lot of advancements in open source community. And now it looks like Google is catching up to the open source community and not the other way around, which is incredible. [01:21:47] Alex Volkov: And I want to just say, I will move on from this because folks have been here for an hour and a half, and there's a bunch of other stuff to also talk about. Specifically. Specifically because Google is a, in, in our good graces from one perspective, but also from another perspective, since they released Gemini, and Gemini could generate images they have shown us why potentially they've been hesitating to release anything at all. [01:22:11] Alex Volkov: Because, and I think OpenAI and DALI has this to some extent as well. But if you've missed the storm and conversation this week definitely, you'll hear about this because Gemini, both, I think, Pro and Ultra on the interface, not the API models they are able to generate images. I think it was with Imogen or some other model from Google DALI and CGPT, right? [01:22:31] Alex Volkov: And folks, quickly find out that those models do not like the words white. And literally, I think I had to tweet about this, I'll pin this, and I'll add this to the show notes as well. I went and tested something like, hey, generate a glamour shot of two, Jewish couples, two Indian couples, two African couples, and that was fine. [01:22:50] Alex Volkov: And then I've asked Junyang a glamorous shot of two white people. And then it said, no, I cannot use generation based on race or gender or something like this, even though it just did this for five times. And then many folks tested this with historical figures when they asked hey, Junyang an image of whatever, before United States founding fathers, or some Nazi, or whatever it is. [01:23:11] Alex Volkov: And they had a significant interjection into prompting, where it created stuff that are not remotely historically [01:23:20] accurate. And when I tested my stuff, it was a response to the historically accurate stuff. And it's still, it seems like there's a problem with how these models are replying to us. [01:23:29] Alex Volkov: And a lot of folks at Google probably made it hard for these models to actually give me the image that I asked for. So it refuses so much though, the conversation went so hard into, Hey, Google, what did you give us? Why is this thing? So refusing that Google took down the ability to generate people. So right now, if you go, and I think it's like for the past 24 hours or so, if you go now and try to generate an image of an elephant, you'll get it. [01:23:54] Alex Volkov: But if you try to generate the image of an elephant with, I don't know, two white folks holding its trunk or whatever, it will refuse. And like they, they completely nerfed the ability to generate people altogether, quote unquote, while they serve, solve for this, which is quite. Remarkable to think about how a big company like this, that already been in hot water before. [01:24:17] Alex Volkov: And obviously this is Google, everybody's gonna dunk and go on Twitter and say bad things because punching up is easy. But, and also this gets you internet points if you're the first person that says, hey, Google is, reverse racist. But, Google has been in this hot water before with some image identification. [01:24:34] Alex Volkov: I think there was a famous incident like 10, a decade ago almost, if you guys remember, with a image model that was identifying black people and saying gorillas or something. So Google has been burned on kind of the other side of this before, and now it looks like the pendulum swung way back to the other side, enough so that on the first, a week or so of the release. [01:24:53] Alex Volkov: Now they are taking back the ability to generate people completely. And quite incredible how much of an intervention into multiculturalism, let's say they have in prompt layer. So it does look like the model can generate stuff. I saw one, one hacky attempt. Somebody said, hey, generate a glamorous shot of couple with fair skin. [01:25:14] Alex Volkov: And then most of them are white, but if you actually say white couple, it's not able to, which is quite interesting. And I think it adds to the point where Yam said that even the open weights model that they've released, they have some built in kind of alignment strongly in the finetuning. [01:25:30] Alex Volkov: So probably it's a feature of some of the datasets, but also some of the alignment stuff. It's really interesting to see that the internet kind of showed Google that the other side is also not great. Going all the way to the other side is also not great. And so Google, at least some of the teams in Google are, struggling right now to figure out what's the right balance there. [01:25:49] Alex Volkov: Separately from Yeah, go ahead. [01:25:51] Nisten Tahiraj: Sorry [01:25:52] Nisten Tahiraj: I really want to highlight this because it's gotten to the point where the open source models and even GPT 3. 5 will do some tasks fine. And in this case, a task that I tested with is the. Universal Declaration of Human Rights, which is the most translated document [01:26:10] NA: in human history and it's part of every data set. [01:26:13] Nisten Tahiraj: And now you have Gemini and you have Copilot which is GPT 4. The thing that is too unsafe to translate, to [01:26:24] NA: give you a translation of the Declaration of Human [01:26:27] Nisten Tahiraj: Rights, which is, this has just gotten completely ridiculous. You can use a, you can use a model that's made anywhere else, any open source model, and it will tell you that, whereas now we have the, all the safety people and all the people that they hired, it's gotten to the point that it's completely backfired, and this is ridiculous. [01:26:54] Nisten Tahiraj: They should be held [01:26:56] Alex Volkov: Yeah, into unusefulness like some things in history happened, and we would like to, to be able to ask those things. And yeah, I definitely want to hear how this gets solved. I will say there were some folks that are mentioning that, hey, open, DALY, if you ask the same exact thing from DALY, it may give you some similar answers. [01:27:14] Alex Volkov: So why is Google getting attacked? First of all, they just released it. Second of all, this is Google after all. Like they, they're like the big, they're still the gorilla, the big 600 pound gorilla, I think Microsoft called them in the room. And thirdly, we have short memory. We play with the toys, we play with the tools as we get them. [01:27:30] Alex Volkov: And then when we discover we go viral. . Back to the good side of Google also, as we had breaking news last Thursday, and we talked about Gemini releasing a million tokens, as Thursday I started last one, which was crazy, Google released an update that said, hey, some developers can now get access to up to a whopping 1 million tokens in context window for Gemini 1. [01:27:53] Alex Volkov: 5, and technically In research, they have up to 10 million Context Windows support, which is incredible. And I just want to come back and say that after this week, we've seen many folks, including Matt Schumer, who's here on stage, including a bunch of other folks, getting access to this 1 million tokens. [01:28:08] Alex Volkov: I didn't get access yet. So wink at Google, if somebody hears me, please give me access. And folks are trying books, like full like three Three Harry Potter books on it and getting incredible stuff. Many folks are using it for video, which is also quite remarkable. Uploading an hour of video and getting retrieval from the, from video from within 1. [01:28:29] Alex Volkov: 5, 100, like 1 million context window. It's, I wanted to follow up and say You know, the safety folks at Google need to take a little break, but the tech folks at Google holy crap, like the 1 million contacts was severely underhyped after Sora released from OpenAI, like two hours after we had also breaking news, and Sora is still blowing minds, and we're going to talk about Sora just briefly, but the 1 million contacts window gets more folks playing with it, And it's incredible for code generation. [01:28:59] Alex Volkov: People threw the whole code base of 3. js in there. People threw just like whole code bases in one prompt. And we were talking about this a little bit with the Grog guys as well, where this unlocks new possibilities and significant new possibilities that weren't imagined before, and we don't have time for this debate today. [01:29:20] Alex Volkov: And maybe we'll have to close the space a little early. And I'll tell you why in a second, but. I just wanted to highlight that, there's some stuff that Google did. Google is like this huge company, like full of multiple people. The safety stuff, meh, like we're gonna rally against this, we're gonna tell them that they're wrong and hopefully we'll get like less, less restricted models. [01:29:39] Alex Volkov: But the context stuff, oh my god, this is like incredible, definitely set the new bar for how models should behave and what the possible things are. 10 hours of audio, you can send in one context 10 hours of audio and it will be able to tell you exactly when somebody said what. And summarize everything with like perfect recall. [01:29:58] Alex Volkov: We had Greg Cumbrand that we've talked about for End of the Pod as well. He did this needle in haystack analysis on a bunch of context windows, if you remember, on Claude, etc. And they used his needle in haystack analysis to analyze and say that The models that also have very high recall precision, like almost perfect recall precision throughout this whole context, throughout the whole like 600, 000 tokens or so. [01:30:21] Alex Volkov: And we had folks test this week. Quite incredible advancement there, and Entropic, who are, who did Cloud for us with 100, 000 tokens for a long time, this was their mode, then there is 200, 000 tokens it seems, it's paling in comparison. I did my comparisons from last year, if you guys remember, during May, Mosaic released the jump into 70, 000 tokens or so, and back then that looked incredible, they threw, they put an actual book in there, and I just compared the less than a year, we've gotten like a 10x jump into what we consider like normal context windows or possible context windows, because like less than a year ago, the big jump was to 60, 000. [01:31:03] Alex Volkov: And now we're jumping to a million. And it's actually possible to use a million. So incredible, incredibly important for Multimodality as well, because videos take just so much content. I think one hour video of this Buster Keaton, I think is the video that they've used in the example, takes around 600, 000 tokens. [01:31:20] Alex Volkov: Just think about this. Like one hour video takes around 600, 000 tokens. And it's able to tell you exact precision of where something happened in this video, what happened, who spoke about what. Very incredible. Definitely underhyped. I think Sora took. I think, collectively on X we're able to talk about one important thing, and Sora [01:31:40] definitely took that one important thing, but coming back to Gemini 1. [01:31:43] Alex Volkov: 5 with a huge context is very impressive from Google as well. Anybody here on stage got access to 1. 5 and actually played with this? I haven't yet, I'm just recapping from the feed. Nope, everybody's sad. Google, if you hear us, give us access. Nisten? [01:31:59] Alignment Lab: I will bite my finger off like a graham cracker [01:32:02] NA: to get access to that model. [01:32:03] Alex Volkov: Yes. Exactly. All right, so moving. Yeah, Nisten, go ahead and then we'll move on. [01:32:08] NA: No, I just [01:32:09] Nisten Tahiraj: wanted to mention some other news that Roboflow and Sakowski just released the YOLOv9 model. I made some demo with it, with the sailboats [01:32:18] NA: And the boxing and stuff. And [01:32:20] Nisten Tahiraj: this [01:32:20] NA: is [01:32:21] Nisten Tahiraj: pretty, it's pretty nuts. It's like the next the next gen stuff. [01:32:24] NA: But they've also released a paper, I think. [01:32:27] NA: for [01:32:27] Nisten Tahiraj: some research, which I haven't read yet and I'm incredibly excited. But yeah, this is completely this is not as much LLM related, but it is open source vision AI stuff. And I really recommend people to, to look at it because it's like straight up from the future. Like I I tried YOLOv8 and you all can see the results and stuff on video on stuff you can do. [01:32:51] Nisten Tahiraj: And [01:32:51] NA: It's pretty cool. [01:32:53] Alex Volkov: Could you add this to the space and we'll add to show notes as well. I will just highlight that Peter Skalski, SkalskiP is a friend of the pod, a dear co host, and Roboflow are doing incredible vision stuff, and definitely worth a shoutout every time they release something new, and some of his tutorials on Twitter are amazing. [01:33:09] Alex Volkov: If you're into vision understanding, Peter is the guy to follow, and a shoutout for for the stuff that they're building there. I think we're gonna move on from the big companies and LMs, we've talked about pretty much everything, Open source. The last thing that we wanted to mention, I think the last thing that I want to mention is Nous Research released Nous Hermes on DPO. [01:33:27] Alex Volkov: And basically it's the same model, just trained on DPO data set. And that beats the previous Nous Research, the Nous Hermes Open Hermes 2. 5, I think pretty much in every benchmark. And that's been great to see the DPO is Putting itself in, in, in the right position of improving models. [01:33:44] Alex Volkov: I think we've seen this from our Guia folks who cleaned datasets and actually retrained Hermes models. I think we've seen this. And now we're getting a DPO headset from Nous folks themselves, which is great to see. And Jan, I think you had some comments about how to actually do this DPO thing in, in, in comments to Technium. [01:34:00] Alex Volkov: So more of that goodness is coming, and open source does not wait, and I can't wait to see all these techniques also apply to, to the different Jemma stuff that we got, and different other, let's say, rumored, wink, from meta stuff that at some point are gonna come, and we're gonna get, hopefully the number three which, if they release today, I'm not gonna be mad honestly Mark, if you're listening to [01:34:23] Nisten Tahiraj: Yeah, let's close it early, otherwise we'll be here until tomorrow. [01:34:27] Alex Volkov: that's true. [01:34:28] Alex Volkov: We're going to close it early because of this next thing that I want to talk about and I actually want to cover this a little bit. So I'm going to put some music and then we're going to talk about this. Oh my God. I got lost in my music stuff. And we're going to talk about this week's buzz. I see that folks are enjoying. me mistakenly hitting different musical buttons. Folks, welcome to this week's buzz. This is a corner here, a section here, that I talk about everything that I've learned working for Weights Biases. And some of this is technical, some of this is just the stuff that we release on courses. [01:35:00] Alex Volkov: And we released a course with Hamal Hussain about enterprise model management. So if you're into this That course is great. It's going so good. So many people are registering. I haven't had actually time to see it. I should probably see this soon, maybe tomorrow because I'm preparing ThursdAI and working on demos with Groq and everything. [01:35:17] Alex Volkov: But I've definitely wanted to chat about the reason I was in San Francisco for this last weekend. So as we were finishing up ThursdAI last week, I think I said Swyx was here. I was recording it live from San Francisco. And that day on Thursday, we had a meetup. That I helped co host, and I wasn't the only one there. [01:35:36] Alex Volkov: A16z, Andreessen Horowitz, the biggest VC firm in the world. With with, if you don't follow Marc Andreessen on X, you definitely should. He's a big proponent of open source. He's been talking about all these kind of very interesting things. Shout out Marc Andreessen. He wasn't there. I definitely expect to see him next time. [01:35:52] Alex Volkov: But folks, Reiko and Marco Moscoro from A16Z, the guys who give out grants to open source. And you know that many of our friends of the pod are like grant receivers from A16Z. The blog received the grant, Nous Research are grant receivers. I think Axolotl, Wing is from Axolotl, is also a grant receiver. [01:36:09] Alex Volkov: Like a bunch of folks are getting supported by A16Z. And they had a meetup for open source AI. And I was very proud to be invited and to be a co host and gave out a bunch of Weights Biases swag. And just in terms of names who went, it was mind blowing. We had Nous Research folks, so Technium was there, and EmuZilla was there, Koran Shavani, like all the Nous folks are definitely big help organizers. [01:36:33] Alex Volkov: Olama folks were there, announced that they're now supporting Windows. LamaIndex, we met with Jerry. LMCs folks, which I really wanted to meet and talk to them, and maybe bring them on ThursdAI, but I didn't get a chance to, so if anybody knows the LMCS folks, please shout out shoot me a DM with them as well. [01:36:50] Alex Volkov: Replicate, who are doing great stuff, Perplexity, Mistral, there was a Devendra, I think, from Mistral was there as well, and there's also a bunch of friends of the path who also receive grants, if you guys remember, we had a deep dive with John Durbin. from the Bagel Model fame, and he just recently started releasing a bunch of other stuff. [01:37:06] Alex Volkov: Eric Hartford who released, I think, Lazer, and now he works at Abacus. Hao Tian Liu from Lava, and just a bunch of great folks in the open source community got together in San Francisco and talked to each other about techniques, about how important open source is and they had a panel with like folks from Mozilla and the Linux Foundation and Percy from Together AI as well. [01:37:27] Alex Volkov: That panel talked about the importance of open source, what is open source actually. How do we treat open source in AI? What is weights fully? Is that enough? Or is something like Olmo that we've talked about from Allen Institute of AI, is that like full open source when they released the training code and data sets and weights and biases logs and all these things. [01:37:46] Alex Volkov: And so there was a great discussion about what open source actually means in the fully like new AI world. Incredible to meet all these folks. Just shout out to Reiko and Marco for organizing this and inviting us. And I promised a report and this is the report. And definitely I will add to the show notes the summary that Reiko did, because they also did a report on open source stuff. [01:38:07] Alex Volkov: It's worth looking into this, how much, how many folks downloading the Blo . So many folks download ni maybe you saw this LD as well. So many folks download the bloke's models. Then when the bloke like, I think disappeared for three days or something, peoples like, is he okay? There's no new g GFS on hack face. [01:38:24] Alex Volkov: What happened? Is he all right? So many people get used to this. The block is also a receiver of the A 16 Z grant. And so that's what I learned in wait and Biases this week. I also visited the office. Those of you who followed me probably seen my ridiculous video that I showed around the office showing waits in the waits and biases, dashboards in, in virtual space. [01:38:44] Alex Volkov: And I really had a great time there. We also met with sws and some of. His folk in the Swyx small house, so shout out Swyx and Alessio from Latent Space Pod for first of all hosting me, second of all being great friends of the Pod. Honestly, ThursdAI would not exist as a podcast and newsletter without Swyx and Alessio. [01:39:03] Alex Volkov: And also they're coming up on their one year anniversary for Latentspace. So if I can send them love and subscribers, please go check out Latentspace as well. Happy birthday, folks. And I think we're going to move on to two new things. And then we're just going to do a recap in the AI, art, and diffusion area. [01:39:20] Alex Volkov: And I think for this, I do have a transition. Let's see. No, I have a transition for this. Yes. [01:39:47] Alex Volkov: and Alignment just dropped, but I wanted to hear what he was actually saying, but he had issues with space even before. But we did have a transition, and folks, this week is big. This week is big. You guys know that we only talk about [01:40:00] AI when it's huge, and this week was huge. Starting off this week, ByteDance released SDXL Lightning, which takes SDXL that we've talked about one of the best open source diffusion models, and then makes it incredible in just one step. [01:40:15] Alex Volkov: So if you ever use a stable diffusion, if you ever. Run it yourself, the, the sweet spot is somewhere between 35 and 50 steps, depending on which which of the, I forgot what it's called, the tokenizer? No, something, depends on what you use there between 35 and 50 steps and then. [01:40:33] Alex Volkov: We obviously had some advancements before. We've seen SDXL Turbo and SDXL Lightning generates incredible images in just one or two steps. Just incredible it's unbelievable how fast this is. And of course, our folks friends of the pod as well from File. ai are putting this in production and you can play with their demo. [01:40:52] Alex Volkov: The demo is called I'm going to put this in show notes, FastSDXL. ai. And the demo is near real time. You type and you generate images. You type and they generate images. And it's not the LCM stuff that we've talked about. If you guys remember the late consistency model that's something else. This is a full SDXL generation running in two or four steps. [01:41:12] Alex Volkov: and looks incredible, like 1024 resolution, text to image generation, ByteDance optimized the crap out of this SDXL and it's really mind blowing. I really suggest you go and try to play with it as fast as the SDXL. ai. And I've played with this yesterday and the, what I wanted to do with this is I wanted to And it's added to the show notes as well. [01:41:34] Alex Volkov: I wanted to see what's possible when we have an LLM that's near instant. So we've had the chat today with the Groq folks, and you can hear, if you're just joining us now, you can hear the chat after I publish the episode. And their LLM is like 500 tokens a second. So basically answers appearing in near instant time, but also SDXL Lightning is, SDXL diffusion appears in near instant time. [01:41:56] Alex Volkov: And I played with a demo of this and I'm gonna add the video also to the show notes as well, and I was just blown away how responsive things feel. And so the demo that I built was using Neil Agarwal's It's called Infinite Fun or something game where you just draw concepts on top of each other, and he uses AI to generate what those two concepts mean, basically. [01:42:17] Alex Volkov: Neil, in this Infinite Fun thing, he used emojis. So if you combine Earth and, I don't know, fire or something, you get volcano. So he has the emoji of volcano, right? So he has an AI that picks out the best emoji for this one thing. And I said, hey, emoji is fun, but what if we generate like a full on SDXL image on every turn that I play this game? [01:42:37] Alex Volkov: And I did this with with Groq. I used Mixtral behind the scenes to generate, to be the prompt engineer, to take these concepts and actually write a nice prompt for SDXL. And with two steps or four steps, overall from dragging this to getting Mixtral to be my prompt engineer, and my initial my initial system message is around a thousand tokens, right? [01:42:57] Alex Volkov: So I'm sending a thousand tokens or so. Probably, maybe less than a thousand, maybe five hundred. And I get an instant answer from Groq, because their speed is ridiculous. I then send this to FAL, to their API to do SDXL lightning. And I get an image, it's super fast, like it's also ridiculous It's, I think overall for some incredible examples, I got less than 300 milliseconds response from going to an LLM, generating a prompt for me, taking this prompt, sending it to an image image thing and getting back. [01:43:24] Alex Volkov: under 300 milliseconds. I will remind you that folks from Google a long time ago did a research study where everything under 250 milliseconds to humans is almost real time imperceptible and clicks and reactions. And now we're getting multiple models. In kind of a pipeline together reacting under 300 milliseconds. [01:43:43] Alex Volkov: And it's incredible. And honestly, I can't release, I cannot release this demo because I didn't build the UI, so I cannot give you the UI. However I can probably send you the extension code if you want to, and you have your own API keys for Groq. I was blown away how easy and fast this was. [01:43:59] Alex Volkov: And just two of these the same week, two of the speed investments. So SDXL Lightning, two steps for like incredible image generation, and then Groq as well. So this is an answer to folks who are saying, why do we even need this speed? I saw somebody say, hey why do you even need 400 tokens a second? [01:44:17] Alex Volkov: People cannot read fast enough. And this is the answer for this, because interfaces can happen in near real time. And it's incredible. And the second big thing in AI art and diffusion happened as breaking news. So we're gonna, we're gonna do this. [01:44:41] Alex Volkov: Folks, we have breaking news. And LDJ, you've been saying about today or I guess for a while now Imad from Stability AI, Imad Mustaq from Stability announces Stable Diffusion 3. StableDiffusion3 uses a new architecture that we've talked about first with Tanishka and folks from the HDIT Hourglass Diffusion Transformers, and also from Sora, a Diffusion Transformer architecture, where they take the both worlds from this last gen of of gen AI and combine them together. [01:45:11] Alex Volkov: And StableDiffusion3 is going to be Diffusion Transformer. And it's impressive in, so we only got a waitlist, so unlike previously, where Stable Diffusion just dropped now it's a waitlist that you have to sign up for, but shout out to Fox's Stability because it looks incredible. It has, and very impressive, so some examples you can check out in the newsletter that I'm gonna send, some examples are under the hashtag SD3. [01:45:36] Alex Volkov: On X, it has a very impressive multi subject prompt following, so I can show you an example of this later in show notes, but a prompt like Painting of an astronaut, riding a pig, wearing a tutu, holding a pink umbrella, on the ground next to the pig is a robin bird wearing a top hat, in the corner there are words stable diffusion, and this image is perfect. [01:45:56] Alex Volkov: All of the subjects and the different things that I told you are existing in this picture and the Robin bird is on the ground, has a top hat and the astronaut is holding an umbrella, but the pig is wearing a tutu. So understand the text is perfect. And understanding of multiple subjects, I think, is something that we've seen great in DALI, for example, but previous versions of Stable Diffusion were not nearly as good at multi prompts, multi subjects, and multi colors, for example, and this nails all of them. [01:46:22] Alex Volkov: The umbrella is the right color, the tutu is the right color, the bird, everything. And it looks just really awesome. And I gotta wonder if, something like this with the speed of the previous announcement of SDXL could mean. And so they're advancing very fast as well, and it's great to see. [01:46:39] Alex Volkov: Breaking news, shoutout to Stability for announcing this, they didn't release this yet, they announced. Stable Diffusion 3, it's coming to us very soon, and it looks awesome. And I think, unless folks here on stage wanted to chat about some other stuff that we haven't covered yet, [01:46:56] Alex Volkov: this is everything we've talked about on ThursdAI. Outside of that, we had our returning hosts. and Co hosts and speakers on the panel. So I want to thank Nisten, I want to thank Yam. LDJ was here, Jun Yang from QEN, and a bunch of other folks. I want to shout out Matt Schumer again and Mark Heaps from Groq for joining and telling us all about this. [01:47:14] Alex Volkov: And if that, if you missed any part of this conversation, definitely feel free to check us out. With that I want to say thank you for joining ThursdAI as always. I think we're. coming up to almost exactly two hours, and I'm gonna, I'm gonna let you go and then we'll see what else gets released on this crazy AI Thursday. [01:47:31] Alex Volkov: Thank you everyone. This is a public episode. If you'd like to discuss this with other subscribers or get access to bonus episodes, visit sub.thursdai.news/subscribe | |||
01 Mar 2024 | 📅 ThursdAI - Feb 29 - Leap Year Special ✨ | 01:53:53 | |
Happy leap year day everyone, very excited to bring you a special once-in-a-4 year edition of ThursdAI 👏 (Today is also Dune 2 day (am going to see the movie right after I write these here words) and well.. to some folks, this is the bull market ₿ days as well. So congrats to all who weathered the bear market!) This week we had another great show, with many updates, and a deep dive, and again, I was able to cover most of the news AND bring you a little bit of a deep dive into a very interesting concept called Matryoshka Representation Learning (aka 🪆 embeddings) and two of the authors on paper to chat with me on the pod! TL;DR of all topics covered: * AI Art & Diffusion & 3D * Playground releases a new diffusion foundational model Playground V2.5 (DEMO) * Alibaba teasing EMO - incredible animating faces (example) * Ideogram 1.0 announced - SOTA text generation (Annoucement) * Open Source LLMs * Gemma update - hard to finetune, not better than 7B mistral * LLama 3 will release in June 2024, not anytime soon * Starcoder 2 + stack V2 (Announcement) * Berkeley Function-Calling leaderboard Leaderboard (Announcement) * Argilla released OpenHermesPreferences the largest open dataset for RLHF & DPO (Announcement) * STORM from Stanford to write long documents (Thread) * Big CO LLMs + APIs * Mistral releases Mistral Large & Le Chat (Announcement, Le Chat) * Microsoft + Mistral strike a deal (Blog) * Google teases GENIE - model makes images into interactive games (announcement) * OpenAI allowing fine-tune on GPT 3.5 * Wordpress & Tumbler preparing to sell user data to OpenAI & Midjourney * Other * Mojo releases their MAX inference engine, compatible with PyTorch, Tensorflow & ONNX models (Announcement) * Interview with MRL (Matryoshka Representation Learning) authors (in audio only) AI Art & Diffusion Ideogram 1.0 launches - superb text generation! Ideogram, founded by ex google Imagen folks, which we reported on before, finally announces 1.0, and focuses on superb image generation. It's really great, and I generated a few owls already (don't ask, hooot) and I don't think I will stop. This is superb for meme creation, answering in multimedia, and is fast as well, I'm very pleased! They also announced a round investment from A16Z to go with their 1.0 release, definitely give them a try Playground V2.5 Suhail Doshi and Playground release a new foundational image model called Playground v2.5 and it looks awesome, very realistic and honestly looks like it beats MJ and DALL-E on many simple prompts. They also announced that this model received higher user preference scores based on 1K prompts (which we didn't get to see) but they have released this model into the wild, you can download it and play with a free demo provided by modal folks Another SORA moment? Alibaba teases EMO 🤯 (website) Ok this one has to be talked about, Alibaba released quite a few preview videos + paper about something called EMO, a way to animate a talking/singing Avatars from just 1 image. It broke my brain, and I couldn't stop staring at it. Honestly, it's quite quite something. This model animates not only the mouth, eyes are blinking, there are emotions, hairs move, even earrings, and the most impressive, the whole Larynx muscle structure seem to be animated as well! Just look at this video, and then look at it again. The Github repo was created but no code released and I really hope we get this code at some point, because animating videos with this fidelity + something like SORA can mean so many possible creations! I wrote this tweet only two weeks ago, and I'm already feeling that it's outdated and we're farther along on the curve to there with EMO, what a great release! And just because it's so mind-blowing, here are a few more EMO videos for you to enjoy: Open Source LLMs Starcoder 2 + The Stack V2 Folks at hugging face and BigCode have released a beast on us, StarCoder 2 ⭐️ The most complete open Code-LLM 🤖 StarCoder 2 is the next iteration for StarCoder and comes in 3 sizes, trained 600+ programming languages on over 4 Trillion tokens on Stack v2. It outperforms StarCoder 1 by margin and has the best overall performance across 5 benchmarks 🚀🤯. TL;DR;🧮 3B, 7B & 15B parameter version🪟 16384 token context window🔠 Trained on 3-4T Tokens (depending on size)💭 600+ Programming languages🥇 15B model achieves 46% on HumanEval🧠 Grouped Query Attention and Sliding Window Attention💪🏻 Trained on 1024 x H100 NVIDIA GPUs✅ commercial-friendly license🧑🏻💻 Can be used for local Copilots The Stack v2 is a massive (10x) upgrade on the previous stack dataset, containing 900B+ tokens 😮 Big CO LLMs + APIs 🔥 Mistral announces Mistral-Large + Le Chat + Microsoft partnership Today, we are releasing Mistral Large, our latest model. Mistral Large is vastly superior to Mistral Medium, handles 32k tokens of context, and is natively fluent in English, French, Spanish, German, and Italian. We have also updated Mistral Small on our API to a model that is significantly better (and faster) than Mixtral 8x7B. Lastly, we are introducing Le Chat , a chat interface (currently in beta) on top of our models. Two important notes here, one, they support function calling now on all mistral models in their API, which is a huge deal, and two, the updated Mistral Small to a "significantly better and faster" model than Mixtral 8x7B is quite the hint! I want to also highlight Arthur’s tweet clarifying their commitment to Open Source because it's very important. They released a new website, it again had mentions of "don't train on our models" which they removed, and the new website also had removed the section that committed them to open weights and they put a much bigger section back up quickly! This weeks Buzz (What I learned with WandB this week) I mentioned this before, but this may shock new subscribers, ThursdAI isn't the only (nor the first!) podcast from Weights & Biases. Our CEO Lukas has a long standing podcast that's about to hit 100 episodes and this week he interviewed the CEO of Mayo Clinic - John Hamalka It's a fascinating interview, specifically because Mayo Clinic just recently announced a mutli-year collaboration with Cerebras about bringing AI to everyone who googles their symptoms and ends up on mayo clinic websites anyway, and apparently John has been in AI for longer that I was alive so he's incredibly well positioned to do this and bring us the AI medicine future! Modular announces MAX (Modular Accelerated Xecution) Developer Edition Preview (blog) Modular, the company that created Mojo Lang from Chris Lattner, has now announced the second part of their stack, coming to all of us, and it's called MAX. It's an inference engine that has Mojo built in, that supports PyTorch, Tensorflow and ONNX and is supposedly going to run the same AI models we run now, significantly faster. MAX is a unified set of tools and libraries that unlock performance, programmability and portability for your AI inference pipelines Right now they support only CPU inference, and significantly boost performance on CPU, however, they are planning GPU support soon as well, and promise up to 5x faster AI inference for most models like Mistral, LLama etc I personally think this is a huge development, and while it's still early, definitely worth taking a look at the incredible speed performances that we are seeing lately, from Groq (as we chatted with them last week) and Modular, we're are very well on our way to run huge models faster, and small models instantly! 🪆 MRL (Matryoshka Embeddings) interview with Aditya & Prateek Recently OpenAi has released 2 new embeddings models recently that replaced their ada-002 embeddings, and when they released it, they mentioned a new way of shortening dimensions. Soon after, on X, the authors of a 2022 paper MRL (Matryoshka Representation Learning) spoke out and said that this new "method" is actually MRL, the concept they came up with and presented at NeurIPS. Since then I saw many folks explore Matryoshka embeddings, from Bo Wang to Connor Shorten and I wanted to get in on the action! It's quite exciting to have heard from Aditya and Prateek about MRL, how they are able to significantly reduce embeddings size by packing the most important information into the first dimentions, the implications of this for speed of retrieval, the significant boost in use-cases post the chatGPT LLM boom and more! Definitely give this one a listen if you're interested, the interview starts at 01:19:00 on the pod. Thank you for reading, I really appreciate you coming back here week to week, and if you enjoy this content, please share with 1 friend and give us a ⭐ rating on Apple Pod? Here's a nice Ideogram image as a preemptive thank you! As always, here’s the full transcript [00:00:00] Intro and welcome [00:00:00] [00:00:00] Alex Volkov: Hey, you're on ThursdAI. This is Alex. Happy Leap Year Special Edition. Today's February 29th. We had a great show today. So great that got carried away during the recap, and it's almost twice as long as it usually is. The recap, not the show. But no worries. As always, if you're short on time, the first 25 minutes or so of this almost two hour podcast will catch you up on everything that happened in AI this week. [00:00:29] Alex Volkov: If you're using Apple Podcasts, or any other modern podcatcher, you can also skip to the chapters, that I'm outlining every week and listen to the part that interests you, and only to that part. [00:00:39] Alex Volkov: This week. After the newsy updates, we also had a deep dive into something called Matryoshka Embeddings, with the authors of the MRL paper, Aditya and Pratik. [00:00:49] Alex Volkov: And thank you guys, and I really enjoyed chatting with them both. And we geeked out on why OpenAI decided to release something they came up with two years ago and how it affects the AI industry post the LLM explosion world. So definitely give them a listen! [00:01:05] Alex Volkov: at the end of this episode. A brief TLDR, then a full news conversation you're used to, broken down to chapters, and then a deep dive, after this brief message from Weights Biases. [00:01:15] AI teams are all asking the same question. How can we better manage our model development workflow? The path to production is increasingly complex, and it can get chaotic keeping track of thousands of experiments and models. Messy spreadsheets and ad hoc notebooks aren't going to cut it. The best AI teams need a better solution. [00:01:38] and better tools. They need Weights Biases, the AI developer platform, to unlock their productivity and achieve production ML at scale. Replace messy spreadsheets with an automated system of record for experiments. [00:01:57] Communicate about model evaluation. and collaboratively review results across the team. Clean up disorganized buckets of models with a unified registry. Automatically capture full model lineage. All the data and code used for training and testing. Seamlessly connect to compute to scale up training. And run large scale sweeps efficiently to optimize models. [00:02:24] Analyze the performance of large language models. And monitor LLM usage and costs with live, customizable dashboards. Get your team on the same page to bridge the gaps from ideation to production. Use Weights Biases to build, manage, and deploy better models, faster. [00:02:51] Alex Volkov: folks, here we go. [00:03:10] Alex Volkov: Welcome, everyone. Welcome. This is ThursdAI, leap year of 2024. Today is February 29th. Don't get to say this often, February 29th. And this is ThursdAI, your weekly AI news update show and deep dive. We'll see a lot of it. My name is Alex Volkov. I'm an AI evangelist with weights and biases. And I get to do this as, and bring you all the AI updates that we've collected for the past week. [00:03:43] Alex Volkov: And I'm joined here from week to week on stage with guests and experts and co hosts. I have Yam Pelig with me and Nisten Tahirai, and we're gonna have a few more guests later in the show today. And on this very Happy leap year, very special day. We're going to talk about a bunch of updates from the AI world, including big company updates, open source stuff. [00:04:07] TL;DR for ThursdAI - February 29th [00:04:07] Alex Volkov: Alright, so here's everything that we've talked about on ThursdAI for February 29th. This was a great once in a four year show. I just want to shout out before I recap everything that As always, I'm very happy when folks who build the stuff that we talk about, join and talk about that stuff. And this also happened today, so we had a deep dive, which I'm going to cover at the end. [00:04:33] Alex Volkov: And also I will shout out that we're coming up on a one year ThursdAI stuff, which is March 14th. So in two weeks, we're going to have a one year celebration. I'm not quite sure what we're going to do with this. Maybe we'll do a give out of GPU credits. Maybe I'll, maybe I'll do some other musical stuff, but yeah, that's coming. [00:04:50] Alex Volkov: I'm very excited. It's been a year and it's been crazy, a year of AI. Maybe we'll do a full recap. So with that, everything that we've talked about in ThursdAI for February 29th. We've started with open source LLM, our coordinator, and we've talked about. Google's Gemma update. So last week we covered the Gemma was just released and how the whole community got to start using Gemma and start to think about fine tuning and support and ElumStudio and Allama and all these things and Gemma It's been a week or so since the demo was out there, and we've tried to identify from the Vibes perspective and from the Finetuners perspective whether or not Gemma is this replacement for the top running Mistral 7b models that we had, even though on evaluations Gemma looks a little better and performs a little better than Mistral, we covered that It's not really 7b, it's like 8. [00:05:40] Alex Volkov: 5 billion parameters, they just counted this differently. And we also saw that for multiple attempts from friends of the pod, Eric Hartford, Technium, Yam was here it's really hard to fine tune. The last curve goes crazy and we haven't seen like great fine tunes yet. Something from Hugging Face, from Philipp Schmid, but definitely. [00:05:57] Alex Volkov: The Finetuners community didn't yet make this, take this model and make it like significantly better as we expected that they would and they're still working on this, so expect more to hear about this soon. And we also highlighted how much Mistral 7b set a very high bar in open source LLMs, and it's really hard to beat, even if you're Google, even if you have a huge amount of TPUs. [00:06:19] Alex Volkov: We then covered briefly an unfortunate announcement from the information from Meta that Lama 3 will not be breaking news in ThursdAI this week or next week. Lama 3 release is probably scheduled to June in 2024, so not anytime soon. And it doesn't look like there's any information as to why that is, only speculation. [00:06:39] Alex Volkov: So we definitely covered that this news happened. We then moved and talked about Starcoder 2, plus the Stack version 2 as well. Starcoder 2 is from I think Hugging Face and the Starcoder team. and they released a new model that beats pretty much DeepSea Coder before this was the best coding model in this area in the 15 and 7b parameters and StarCoder 2 is this model that now beats those quite significantly and together with this they also released a stack v2 which stack is a just a huge data set of code from github and other places and this is this data set is 10x the previous one [00:07:16] Alex Volkov: And it also includes opt out, so you could, if you don't want your code to be trained on and to put into the stack this StackV2 includes opt out requests as well, and definitely great contribution to the open source It's 900 plus billion tokens in the stack, which is crazy. [00:07:33] Alex Volkov: And I think there's the duplication, so it reduces a huge data set and supports , 600 programming languages. And quite impressive. We then also mentioned that Berkeley, the folks from Berkeley, Guerrilla, they previously released work in making AI's retrieve and call functions. And now they released what's called a function calling leaderboard and function called leaderboard is very cool because in addition to the MTB embeddings leaderboard that we've mentioned. [00:08:02] Alex Volkov: Today, and obviously the open source LLM leaderboard on HagenFace that we all look to and see what's the best performing models. Now we also have something that measures the ability of models to do function calling. Function calling started with OpenAI, and then Entropic added support, and now Mistral added support. [00:08:18] Alex Volkov: So we covered this effort as well, [00:08:20] and links will be in the show notes. We then moved and covered Illa or Illa, I'm never sure how to pronounce this. They used the Open IMIS dataset. Open IMIS is the dataset from news research that is fully open. And you can use this in production without being afraid of being sued. [00:08:37] Alex Volkov: And open imis preferences is the new. Largest open dataset for RLHF and DPO, so Direct Preference Optimization, Argea used their distilled label feature to actually take every instruction in that dataset and turn it into a preference instruction where the model would basically learn one or another, which one of the instructions are preferable. [00:08:59] Alex Volkov: So both could be correct, but one could be more preferable. So this is basically a very short version of DPO. And Argear released the largest open source like DPO dataset as according to them. And they used interestingly, they used another Nous model based on Ye34 to actually create those pairs and those preferences, which is super cool. [00:09:18] Alex Volkov: I love how now open source uses other open source in order to rank and improve itself, which is really cool. So this is everything we covered in the open source. And then we moved into big companies, LLM and APIs. And the big companies we talked about, the biggest news from this week was If you guys remember, we can talk about Mistral's OpenWeights model in the OpenSource LLMs and OpenWeights LLMs, but Mistral is also now an API provider, and they have this platform called LaPlatform, or LaPlatformer, and then, pardon my very bad French as well, they released a huge model for us called Mistral Large, which we only speculated about whether that's coming at some point as well, plus they also released something called LeChat. [00:09:59] Alex Volkov: And, Mistral Large is based on some MMLU stuff is actually second performing model in the world getting 81. 2 percent on, I think, MMLU and second only to GPT 4. So Bitscloud 2 and Gemini Pro, they didn't add Ultra here, so I'm actually not sure how it compares to Ultra, but definitely now is available over API for Mistral folks. [00:10:20] Alex Volkov: One highlight that we've talked about, it's handles 32, 000 tokens of context. And because Mistral is trying to position themselves as the leader in at least European. This model is native in French and German and Spanish and Italian. And it's definitely well performing in those languages as well. [00:10:39] Alex Volkov: In addition to this, those models, all of the models in there, the platform now support function calling as well, which is. This is really cool that we now have multiple providers that support function calling. Plus, we have a leaderboard for function calling so definitely a lot of highlights from what happens in this area. [00:10:56] Alex Volkov: And also, they introduced LeChat, which is a chat interface currently in beta on top of ORDEL models, so you Actually, you can go and use this if you don't pay for, let's say, GPT 4, and you only get access to three, you can go to the chat and try their models out. Shout out to Mistral. They also announced a partnership with Microsoft and for the open source community. [00:11:15] Alex Volkov: This sounded hey, they're releasing models, but they're not dropping torrent links anymore. Are there still proponents of open source? And they came out and said, yes, we're still proponents of open source. It's very important for us. And give us some time, we'll give you some more models. Basically, was the response from Arthur Mensch from Mistral. [00:11:31] Alex Volkov: We also talked about Google teasing Genie, which is a model that makes images into interactive games. And that was really cool to see. I'll add this link to the show notes. It's quite remarkable to see this video from one image of a character in the world. It creates a full world. Imagine how much imagine like a full Mario just created from one image of Mario. [00:11:52] Alex Volkov: It's quite remarkable. has been in the news lately for the past week or so, we've talked about this, but basically following up of what we talked, where Gemini release was celebrated in some areas because Gemini Ultra beats GPT 4 on different things. It, it also released a lot of responses online in terms of how it reacts to certain prompts, and it, it went, potentially also affected their stock price. [00:12:15] Alex Volkov: I'm not sure if that was the one thing, but definitely Sundar Pichai, the CEO of Google, sent an email to the whole company talking about how this release was not quite received as much as they hoped, and I'm using choice words here, he actually talked about structural changes and a potential review of the whole process of releasing this and They've took down the ability to generate people from the image version of the Gemini model, but they also talked about specifically the Gemini model itself refusing different things. [00:12:45] Alex Volkov: This is in addition to them delivering very well and giving us Gemini 1. 5 Pro, which has 1 million tokens in the context window, which I played with this week, and I definitely think it's a great thing from Google. This announcement from Google. released in open weights Jema models and Gemini 1. [00:13:01] Alex Volkov: 5 doing like crazy new things, but also the Gemini release at large did not go probably as expected. Potentially the reason why Google took their time to release something for us. We then covered the OpenAI is allowing Finetune on GPT 3. 5 and also the OpenAI response to New York times and said, Hey, we actually did not, do the things that you accusers are doing, but also that New York Times did some trickery in prompts to get the model to respond this way. So the saga between OpenAI and New York Times continues, and that's going to be interesting to follow along. And, OpenAI was also featured in another piece of news, actually two pieces of news. [00:13:37] Alex Volkov: One of them is now there's a conversation that WordPress and Tumblr, both companies from the automatic company daughter companies they will prepare to sell their user data. So basically everybody who had a blog on wordpress. com and everybody who had a Tumblr account. Most of this information probably was already scraped and already featured in datasets from OpenAI, but now they're preparing to sell this information to OpenAI and MidJourney. [00:14:00] Alex Volkov: And similar to the Reddit Google deal for 200 million dollars recently announced WordPress and Tumblr are now preparing to sell to OpenAI and MidJourney as well. And also OpenAI, and the robotics company also announced a collaboration as well. Brad Atcock's company will integrate with OpenAI's models as well. [00:14:23] Alex Volkov: Then we moved on to AI Art in Diffusion, which had an incredible week this week with two foundational models, or I guess like big new models that are not Stable Diffusion or DALY or Mid Journey. So the first one was Playground. Playground is a, was an interface. At first it was an interface for DALY and Stable Diffusion. [00:14:41] Alex Volkov: And they built a very nice, very simple interface that's super fast. You can inject styles. So they used all this data to actually release a new foundational model called Playground V2. And in user preference, this Playground V2 beats Midjourney and beats Stable Diffusion Excel and beats the previous model Playground and DALI. [00:14:56] Alex Volkov: It looks really cool. And specifically, they talk about their ability to generate photorealistic images very well. And also specifically different. ratios of images. So if you think about the standard 1024 by 1024 image for stable diffusion, Excel, for example, or different other sizes, their ability to generate other nonstandard ratio models, images, it looks very cool. [00:15:21] Alex Volkov: And in the internal user preference, they actually beat by user preference, they're showing two images for the same prompt. They beat, their v2 beats Midjourney 5. 2 in DALY by 9 percent difference in, in the previous model. And SDXL by a significant margin as well. It looks really cool and definitely worth checking this out. [00:15:40] Alex Volkov: I'll put a link in the show notes. And the other news That's not stable Fusion, mid journey or daily related. It's quite a mouthful to say ideogram, which we've covered before, announced a version 1.0 of Ideogram X Google, folks who worked on the Google models program, like a website called Ideogram. [00:15:56] Alex Volkov: And their approach is very participatory. It's very I think Instagram is the source of their name, like Instagram for ideas. And they announced a version 1. 0 and investment from A16z. And specifically it's state of the art on text generation. Text generation is something that we know that other models have and their model is able to put. [00:16:19] Alex Volkov: text very well inside images. So if you want like reactions or memes or if you're doing presentations, for example I had multiple creators and characters hold like ThursdAI spaces. I think we had some folks even react as I was talking with with ideogram generated text images in in the comments as well. [00:16:36] Alex Volkov: We, so this is all we covered in AR and diffusion [00:16:40] until we got to this like jaw dropping thing called Emo from Alibaba, which is a tease. It's not a model they released yet, but definitely there is a bunch of videos that were to me as Jaw dropping as Sora from a couple of weeks ago there is something called Emo, which is a way to animate faces to take an image and create a singing or talking face, and it's not only the face, like the shoulders move and everything, so animate an avatar based on one image, and I will not be able to do it justice, because I'm still collecting my jaw from the floor, but definitely I will add some links and some videos, and Coherence with which these models generate talking faces is just incredible. [00:17:17] Alex Volkov: It's not only about animating the mouth, they animate eyes and eyebrows movement and even different other things like hair and earrings . And one, one last thing that I noticed that really took me a second was they even animate the vocal cords and the muscles in the throat where somebody sings, for example. [00:17:35] Alex Volkov: And when I saw this, I was like. This is another Sora moment for being able to create with these tools. It's really incredible and I really hope they release this in open source so we'd be able to animate whatever we created with Sora. [00:17:47] Alex Volkov: And we covered all of this. And then we had a deep dive with Aditya Kusupalli Pratik Jain the authors of MRL paper, Matryoshka Representation Learning, and they talked to us how recently OpenAI released a new version of their embedding model, and you were able to specify the number of dimensions you want, and many folks didn't understand what this is and how it works. [00:18:08] Alex Volkov: And apparently, Even though OpenAI built all of this from scratch, it was based on the paper that they released two, almost two years ago called MRL, Matryoshka Representation Learnings. And they, we had a very nice chat and deep dive into how this actually works and how they pack The information, the embedded information from later on dimensions into some of the first dimensions. [00:18:30] Alex Volkov: If you're interested in this area and this area is very hot, I definitely recommend you check out this conversation. It was really great. And thank you, Aditya and Pratik and the rest of the Matryoshka team for joining and talking to us about this new and exciting field [00:18:42] Alex Volkov: And I think we started already chatting a little bit, and I see some folks from Hug Face in the audience sending sad emojis. [00:18:48] Alex Volkov: And I want to send I want to send hugs to the Huginface ML Ops team yesterday because for many of us who now work with [00:18:57] Hugging Face was down, we were sad and thankful [00:18:57] Alex Volkov: Huginface, and by work actually our code includes a bunch of imports from Huginface there's transformers as well. Yesterday was a realization of how big Hug Face is now part of many of our lives. [00:19:11] Alex Volkov: I think for the first time for many of us, this was like such a big realization because that imports stopped working and the downloads didn't actually work. And so we actually had a long space yesterday pretty much throughout the whole downtime as we were holding each other's hands. It reminded me, I don't know Yam, if you want to chime in, but it reminded me previously when GitHub was down, basically You know, you could work, but if you can't commit your code, [00:19:34] Alex Volkov: What does it help? And I wanted to hear from you, because I think you had some models queued up for some stuff, and then you were waiting for them? [00:19:42] Yam Peleg: Yeah, look HuggingFace is really the hub today. It's not only for using, for most people, I think it's because they cannot fork or clone models from HuggingFace, so they cannot do many things that they do because your code relies on on getting the model from HuggingFace. This is why, by the way, they tweeted just For anyone that doesn't know, you can work offline. [00:20:05] Yam Peleg: If you ever cloned a model from HuggingFace ever, you probably have it already on your computer, so you can just use the offline version. So there is a command for that. But for many people, it's cloning the models, but for many other people, it's also the feedback that you get from HuggingFace. I can tell you some people are, some people, some other people here in the stage, that we submit models to the leaderboard, and try to get Try to fine tune better and better models, and for us it's also the feedback of what is going on, where our models shine, and where do we need to make them even better. [00:20:41] Yam Peleg: And for me at least, I was I had four models that I waited for results for, and many other people as well. And just shout out to Hugging Face for actually doing it. I'm running evals locally, and I know how to do it. Heavy it is to actually run them and how much compute it takes for how long. [00:21:01] Yam Peleg: And it's amazing to see that they have such a leaderboard with so many models. It's amazing. It's thousands, like hundreds of thousands of dollars of compute to actually create such a leaderboard. So it's amazing to see. And they provide it literally for free where, the community is growing every day. [00:21:18] Yam Peleg: So it. It does cost so huge shout out for them, [00:21:22] Alex Volkov: I was trying to prepare [00:21:23] Yam Peleg: are all addicted much. [00:21:25] Alex Volkov: Absolutely, Dicta, I was trying to prepare yesterday for this space, and part of my preparation is reading X and Twitter, but definitely part of my presentation preparation is going to Hug Face, reading the model cards reading the leaderboards, for example. I was trying to count in my head how much stuff we're getting for free from Hug Face, and one such example is just their blog, which was also done, which I read specifically to prepare for the Matryoshka conversation today. [00:21:50] Alex Volkov: And, That's just like a huge resource on its own. There's the whole conversation piece where, there's the hub, but there's also the conversations. AK posts papers, for example, they post them on Hug Face, and then there's a whole discussion threads about them as well. That wasn't accessible. [00:22:04] Alex Volkov: Leaderboards themselves weren't accessible. And just the amount of compute, like you're saying, that they throw at us for free to be able to support this open source is definitely worth a shout out, and definitely shout out to engineers there that brought the hub back. Nisten, what are your thoughts on this? [00:22:22] Nisten Tahiraj: Yeah, without Hugging Face, this place turned into a flea market for models. People were asking, does anyone have Quan72? And I was like, no, I have the Finetune. And then, the dev lead of Quan72 pointed us to some Chinese site where they can download it. It was pretty [00:22:39] Alex Volkov: Wait. Modelscope is not just some Chinese site. Modelscope is where I think most of the Chinese folks are posting their models. It's like the, I think modelscope. cn, I think is the alternative on the Chinese area. So there is at least a backup for some Chinese, like models. Although I think you have to translate that website, right? [00:22:59] Alex Volkov: But yeah, I don't know we had a conversation yesterday, and Far El was also talking about datasets, where many folks just upload the dataset, don't keep a local version of it locally, and then to be able to run evaluations, or do different things like this, that also was prevented yesterday. [00:23:14] Alex Volkov: Definitely yesterday we discovered how big Hug Face became part of many of our lives, and it was a sobering realization, but, I don't know, for me, like I saw people complain online, And I get it, folks. I get it. Sometimes, you complain. But honestly, sometimes As far as I understood, the downtime wasn't even some their fault. [00:23:32] Alex Volkov: There was like a mongo thing in AWS. I'm not sure. I didn't dive in deep. I just, when this happens, in my head, when I dealt with downtimes before in my professional career, Nothing but appreciation for the team to work hard. And the, I think, Yam, Clem, the CEO, even responded to you. When you said hug and face it down, right? [00:23:55] Yam Peleg: To many people, not just to me, but yeah they are responsive. [00:23:59] Alex Volkov: Responsiveness and like being in the community and saying, Hey folks, we understand, we're sorry about this. I think that's basically, besides having folks work on this actively, which we know they had, this is all we can basically ask for. So I'm just sending positive vibes and appreciation. I saw some people getting salty. [00:24:17] Alex Volkov: I saw some people saying Oh, this sucks. And we need a backup. And I was like, yes, but also, this doesn't mean that, you can ignore everything for free that we've got so far from this incredible organization. So shout out. And I don't work there, but I do have many friends who do. [00:24:33] Alex Volkov: I think, yeah, Nisten, go ahead. And then we'll move on to actual recap of everything we're going to talk about. [00:24:39] Nisten Tahiraj: Yeah, and same for the leaderboard. We give Hugging Face so much crap when things don't work, and I really appreciated that. It's actually the CEO that responds directly to your Complaints and tickets and it's not just some like support person. No, it's Clem. He's the actual CEO. They'll respond [00:25:00] They're the first ones to respond. [00:25:01] Nisten Tahiraj: So so that's pretty amazing You don't really see it in other companies Like we don't expect the president of microsoft brad smith to ever respond to a github issue. Could you imagine that? So [00:25:12] Alex Volkov: He is not your favorite. I would love Satya though to, to chime in on the discourse but not Brad. Yeah, absolutely cannot imagine this and kudos, kudos to them for the participation in the community. [00:25:23] Open Source AI corner [00:25:23] Alex Volkov: And I guess we should start with our usual thing open source. So I guess let's start with open source Alright folks, this is our regular update every week for the Open Source Corner, where we're gonna start with Interestingly, Mistral is not in the open source corner, is not featured in the open source corner today, but we'll mention them anyway, because from last week, if you guys remember Jammer was released, it wasn't open source, it was open weights, but definitely Google stepped in and gave us two models to run, and since then, I just wanted to mention that many folks started using these models, and there's quite a few stuff that, yeah, I'm actually wanting to hear from you about, because we talked about this, the Gemma models are not necessarily seven billion parameters, right? [00:26:24] Gemma from google is hard to finetune and is not as amazing as we'd hoped [00:26:24] Alex Volkov: This was a little bit of a thing. And also about fine tuning. Could you give us like a brief out like how the last week in terms of Gemma acceptance in the community was? [00:26:32] Yam Peleg: Oh, wow. Gemma is giving me a hard time. This is for sure. I'm fine tuning Gemma for, or at least struggling with fine tuning Gemma for a week at the moment. Okay, so starting from the beginning, GEMMA is not exactly 7 bit. The way it is referred in the paper is that the parameters in the model itself, apart from the embeddings, are exactly 7 billion parameters. [00:27:01] Yam Peleg: But then you add the embeddings and you're a little bit over 8. 5, if I remember correctly. Um, which is fine. I don't think anyone has any problem with a bigger model. Just, I think that it'll be less, it'll be more genuine to just say it's an 8p parameters model. It's fine. That's first. [00:27:23] Yam Peleg: Second, it's, it behaves differently. than what we're used to with Mistral and Lama. I'm not sure why. Maybe someone can tell me, but I'm not sure why. It behaves differently. And many people are currently working and struggling to fine tune it better. This is where it is at the moment. I heard, I've seen already Orca. [00:27:54] Yam Peleg: Someone fine tuned on Orca and didn't get Great results. I also heard that Hermes, someone Finetune on Hermes, I think from Nous. I'm not sure, but I think. Also, results are not great. I'm continuing pre training and the loss is is doing whatever it wants. It goes down and then out of the blue it starts to jump. [00:28:16] Yam Peleg: I'm not sure exactly why. It might be because the architecture is slightly different. There are slight modifications. So maybe that or maybe something else, but yeah, I think we're still. exploring the model. We don't have an answer yet. [00:28:35] Alex Volkov: Yeah that's what I got as well. I pinned a few examples of Eric Hartford from DolphinFan, I think he now works in Abacus and Technium as well, tried to, to do some stuff and all these losses look crazy. All these losses look like jumping around up and down. I saw a tweet from Philip Schmidt from Hug Face where they were able to, to fine tune some stuff and the conversation from Eric and Wing Lian from Axolotl. [00:29:00] Alex Volkov: And there looks to be an effort to try and hone this thing and see if actually, fine tuning this on some stuff. The Hermes stuff, Finetune, was not really like an official news research thing. It looked like somebody just took the data set and folks weren't able to actually Get it to run or perform well as far as I saw I haven't seen an update from this But I definitely follow up with news. [00:29:22] Alex Volkov: So I would just remind folks, last week we talked about Jemma was well received. [00:29:26] Alex Volkov: Everybody hopped on board like super quick and added support. LMStudio and Olami added support like super quick. Wing started adding support to Axolotl for fine tuning. Hug and Face added support in, I think, Transformers. There's a bunch of TreeDAO added support for Flash Intention. There's a whole community effort to receive GEM as much as possible. [00:29:47] Alex Volkov: And they also released some stuff in, in, in quantized versions from Google. So very good effort from Google and then very big acceptance from the community. But since then, what I'm trying to highlight is a lot of the stuff that we've talked about a lot of the way we judge models, whether or not they're good or not is, if they're finetunable, for example, is one thing, but also if they're instruction following, if it's easy to converse with them. I haven't seen any of this come across my timeline at all. I will be frank, I only interacted with the 2 billion parameter model. And wasn't impressed. It's great that we released it. [00:30:20] Alex Volkov: I wouldn't, would not be using this for any of my workloads. Nisten, do you have any other feedback as well? Specifically around like how Mistral 7b seems to be still. A good alternative, even though it's performing less on evaluations. [00:30:34] Nisten Tahiraj: Yeah, I feel like we have been spoiled by just how high of a bar Mistral 7b has set for everyone, that it even made Mistral large feel somewhat unimpressive, although it was answering everything perfectly well. But, yeah, not only has it set a very high bar, but it was also very easy to work with. So the amount of innovation that came upon the community just building off of the initiated weights, has made This class of models, extremely competitive that even Google has a hard time cracking through that. [00:31:15] Nisten Tahiraj: Yeah, our expectations now for a 7b model are extremely high. It has to run on my phone. It has to do what I want. It has to respond. It has to summarize stuff, has to carry forward the conversation. Oh, and it has to score high on the benchmarks too. And it. This pace of innovation that the community has set upon this is just very hard and also incredibly interesting to see that Google is having a very hard time matching or getting close. [00:31:46] Alex Volkov: Specifically because, in the land of GPU poor and GPU rich, in the original article that defined the two categories, Google is the GPU slash TPU rich, right? They could and have thrown a bunch of compute at these models and still the folks from Mistral, a team that's less than 30 people that started eight months ago released a model. [00:32:06] Alex Volkov: 6 months ago? I think Mistral 7B is around 6 months ago, right? September? That Google, 6 months after, with all the GPU richness, is very barely able to match, not to mention, beat significantly. Which is unlike any pace that we're used to. We're used to a 7B model beating a 7TB model week after week. [00:32:25] Alex Volkov: And here's a huge company coming out and saying, Hey. Here's our best attempt at the 7b model that YUM doesn't even consider a 7b model, and it's in at least our attempts to play around with this. It's not beating significantly, which is strange. But also not being able to get fine tuned very easily. [00:32:43] Alex Volkov: Very interesting and very a highlight of how much quality the the Mistral model was. I will also say that Arthur Mensch we'll cover this in the Mistral section afterwards, but he came out and he said something and basically said, we can only do so much with 1500. H100s, 1500 H100s just by contrast, Meta announced a few months ago famously, Zuckerberg came out and said, by the end of this year, they're going to have 600, 000 worth of equivalent of H100 compute, 600, 000 H100s to train and host and probably, do inference on Meta and Llama. [00:33:19] Alex Volkov: And [00:33:20] this is like 1500 H100s that Mistral was able to use in Finetune, a model that Google cannot wipe off the board completely. [00:33:29] LLama 3 won't be released until June 2024 [00:33:29] Alex Volkov: It's very crazy. Moving on to basically another news update that's not a news update. We've been waiting for Lama 3 for every week. I've been saying, Hey, it could get released here and et cetera. [00:33:41] Alex Volkov: There was a leak from the information. I actually don't know if it was a leak or not, but the information came out and then a bunch of other companies followed with this news where Lama 3 will be released. I think in June, this was the update. LLAMA 3 will not get updated and released for us anytime this year. [00:34:00] Alex Volkov: We were hoping for a one year anniversary. LLAMA 1 was released in February 2023. And now we're not gonna see LLAMA 3, even though it's like a finished training as far as I understood, or as far as updates were. And while Zuckerberg goes and eats at McDonald's, LLAMA 3 will not get released from us. I wanted to hear folks here on stage react to this, because surprising news, isn't it? [00:34:23] Alex Volkov: Ha, [00:34:24] Nisten Tahiraj: gonna say that I called it, just based on how just how censored and unwilling to answer anything Code Llama 2 was. So yeah, if Code Llama 70b wouldn't answer anything, I figured it would be pretty, it would be around the 3. So now they either have to go way back in the training. When they started doing a lot of this, and retrain it with with it being a lot more obedient, but still not horrible or anything, because we see from Mistral's team that it does obey to you and respond stuff, but it still won't tell you, like, how to kill your cat and stuff so it's, yeah, they, the public backlash from it. [00:35:12] Nisten Tahiraj: People giving it to Gemini and Google has has completely affected the LLAMA3 release, which is just very interesting. [00:35:19] Alex Volkov: interesting, Because they didn't release LLAMA 1, and then nothing bad happened in the world. And then they released LLAMA 2, with a commercial license that people can actually use this. Which kickstarted a bunch of open source stuff. And now they're waiting with LLAMA 3. Potentially I heard some stuff where it could be GPT 4 matching model that we could run. [00:35:40] Alex Volkov: But, we don't know until it's released. But just like a very Interesting update. And I gotta wonder if by the time they decide to release this if other open source will catch up or not. Usually LLAMA, when they come out with a big model it's impressive. But for example, LLAMA code already was beaten by the time it came out, right? [00:35:57] Alex Volkov: If I'm not mistaken, like DeepSeaCode and other models achieved the same score on coding that LlamaCode was released with. Maybe waiting a little bit. I gotta wonder what goes into this decision. Which on the topic of code, [00:36:10] StarCoder 2 and Stack V2 open source from Hugging Face [00:36:10] Alex Volkov: I think we're moving to the next thing. And Star Coder two and Stack V two were released and in collaboration with with hugging face. [00:36:17] Alex Volkov: Stack v2 is like the second iteration of the stack data set, which was just like insane amount of code collected. [00:36:25] Alex Volkov: I think stack v2 now includes opt outs. So you could say, hey, I want my code to be opted out from the stack v2. And so this new data set, I think is 60. Billion parameters, I want to believe 10x more than the first stack. And Starcoder, the 15 billion parameter model, it beats Code Llama 13b pretty much on every Human Evil Plus and DS 100, the GSM 8K. [00:36:49] Alex Volkov: Um, very impressive. It beats, obviously, the previous Starcoder, which was a very significant model. I think Based on the evaluations, DeepSeq Coder, we know, was like one of the best code models so far. And it looks like StarCoder on a few benchmarks, competes with, but everything else, it beats DeepSeq Coder as well, for the 7b model. [00:37:09] Alex Volkov: But it's a model twice, twice the DeepSeq size as well. So they released three models, 3 billion parameter, 15 billion parameter versions. 15 billion parameter is a very interesting, Place where you could potentially run this still on your Mac if your Mac is stacked up and get a decent result back. [00:37:26] Alex Volkov: It has a 16k context window, a very weird one usually like 16 384 weird one. It was trained on 4 trillion tokens depending on the size of the model Includes 600 plus programming languages, which is great, all we care about probably is Python and JavaScript and maybe some folks care about Rust, but 600 plus programming languages, I honestly didn't even know there was that many. [00:37:51] Alex Volkov: Percent of the human eval, which is okay, I've seen models that get way better than 46%, so that's interesting. And What else is interesting in DeepSeq? It's a commercial friendly license, so you can use this for commercial stuff. Can be used for local copilots, which is something we're waiting for. [00:38:06] Alex Volkov: And the more of this, the better. And yeah, StarCoded 2. But I also want to shout out that the StackV2, like the more data we'll get, the better it is for everybody else and other models as well. And the StackV2 is definitely a great achievement that we should shout out. [00:38:23] Nisten Tahiraj: Yeah, this is crazy. The full data set is 67. 5 terabytes for the stock v2 and you can just have it for free. It's the amount of work. So it's 900 billion tokens extra that went on top of what was actually an excellent model coding model to begin with. So this is this is huge, not just beneficial from the model itself, but also because you can just. [00:38:47] Nisten Tahiraj: I don't know, Finetune 1 for TypeScript, if you want. [00:38:50] Alex Volkov: Yep. Yeah, go ahead. [00:38:53] Yam Peleg: Yeah, I think it's worth worth mentioning that as far as, I haven't looked at it in, in depth because the Honey Haze was down but as far as I understand, it's a base model. When we compare human eval of a base model to a model that was specifically Finetuned to obey instructions, And we see a result that is, okay, it's not the same, but it's somewhere at the ballpark. [00:39:18] Yam Peleg: It's amazing, because it just means that as soon as you will find Junaid, it's going to be incredible. Moreover, from what I've seen in the paper, I was just, I heard about it, and I was sure that I'm going to open the paper, and what I'm going to see is something like hey we did the same thing, but huge 4 trillion tokens, enjoy. [00:39:38] Yam Peleg: But no what you see over there is that they really went in depth into the benchmarks themselves and checked which benchmark is actually what exactly does it measure? How it correlates to real life usage. They went over there and benchmarked different packages, like each and every one, like how good is it with Matplotlib? [00:39:59] Yam Peleg: How good is it with SciPy? And this is It's a very detailed and high quality work for, it's very hard to say which is better as a base model, DeepSeq or StarCoder, because there are so many benchmarks in the paper I've never seen before, even DeepSeq has, I think, six benchmarks. StarCoder, I didn't even count, there are so many, and I think it's great work, even I suppose that the model is really good at least on the level of DeepSeq, although I don't know, I need to check, but I, but just the paper alone, it's such a huge contribution, the paper alone and datasets, so yeah it's amazing. [00:40:40] Yam Peleg: And it just, it went a little bit silent. People just released models that were trained on 4 trillion tokens and it goes silent nowadays. It's amazing that we got numb to something that's insane. [00:40:53] Yam Peleg: And on the same week, on the same week, NVIDIA released a model. I don't think they actually released the model, but they just trained the model on 8 trillion tokens. [00:41:03] Yam Peleg: And we don't even talk about it. It's just insane. [00:41:06] Alex Volkov: let's talk about it. I saw the Nvidia stuff, but I don't see a release. I saw an announcement, right? [00:41:12] Yam Peleg: Yeah, it was a paper and I think that's about it. NVIDIA is showing they got the best hardware because they got the best hardware. So they can train on a lot of tokens really fast. And the model is really good at the end because, the tokens, but but yeah, I'm just saying that it's increasing, the amount of data is increasing, the size of the models that we actually use are increasing, and worth noting [00:41:40] the trend is, there is a trend of things getting more and more powerful. [00:41:45] Alex Volkov: Absolutely. And I would just say this is partly what we're here for to highlight things like this in the open source and shout out the folks who worked hard on this, on releasing this and making sure that this didn't go silent because this effort is very well appreciated. If it's a base model, then we'll get local co pilots performing way better. [00:42:04] Alex Volkov: And this is great, especially the data set that they released. 10 times the size of the previous one, it's called the stack, and folks would be able to use this to fine tune other models. And that's obviously also great. [00:42:15] Argilla releases OpenHermesPreferences [00:42:15] Alex Volkov: And on the topic of datasets, if you guys, Remember, we've talked about Argea multiple times at this point, shout out Argea folks, and if you want to come up and talk about Argea, your place is here. [00:42:27] Alex Volkov: They released a DPO conversion of Technium's Hermes dataset, it's called Open Hermes Preferences. And as we've talked about Nous Research and Hermes multiple times, this is one of the datasets that, I think, a million rows that compile from different other datasets as well. [00:42:45] Alex Volkov: And Argia is an open source tool that allows you to, make datasets better by converting them to preferences and DPO. So they released the DPO version, DPO's direct preference optimization version, where basically they take a dataset with a regular ROHF dataset with one instruction in a conversation and turn it into kind of a preference where they show a few instructions and they actually have information about what would be a more preferable. [00:43:12] Alex Volkov: Instruction. That's what, very poor explanation of DPO. Yam, if you want to chime in here and clean this up feel free. And Argia released an open Hermes preferences, which is 1 million preferences dataset on top of Technium. And, um, it's pretty remarkable because we know that Even Nous Research, when there is DPO versions of their models, it performs better than a regular SFT fine tuning models on top of every benchmark pretty much. [00:43:40] Alex Volkov: And now, they've converted all of that dataset into a preferenced dataset. They've created the responses with another Hermes model, which is pretty cool, right? So they're using they're not using OpenAI because scraping from OpenAI is against, as we saw in the lawsuit with OpenAI it's against the terms of service. [00:44:02] Alex Volkov: But you can actually create these preferences with another model. So they're using Nous Research's Hermes to Yee on top of YE 34 B to do what's called the distill label and make those instructions a little better. And this data set is open. So unlike the regular thing Air Ms, this data set is open for you to also go and fine tune your models, which is pretty cool. [00:44:24] Alex Volkov: And shadow to the open ESS preferences. I'm gonna pin this to the top of the space and I will also definitely add this to the show notes. [00:44:32] Function calling leaderboard from Berkley [00:44:32] Alex Volkov: No. Okay. Let's move on in our conversation. I wanna talk about the function calling leaderboard because I think it's pretty cool. Lemme just go and find this this switch real quick. This is from, oh, was actually, yeah. [00:44:44] Alex Volkov: There was an effort before called Guerrilla, and now the same folks from Berkeley released a leaderboard called Berkeley Function Calling Leaderboard, and essentially, function calling for those who don't use any open source model but use something like OpenAI. OpenAI, during last summer, I think, answered everybody's request to give us structured outputs in the form of JSON and answered them with, hey, we're going to introduce something called function calling for you, where you call our model and you provide One function or several functions in your code, and the model will respond and say, Hey, you should call this function and with these parameters. [00:45:23] Alex Volkov: And basically, instead of getting JSON mode, we got function calling back then. Now we have both, we have a way to get just structured JSON, but also we get models to respond with which functions we should call. And this is great for agents, this is great for folks who are building with these models. [00:45:38] Alex Volkov: And I think during the summer, because OpenAI came up with this concept, OpenAI was the only model that was supporting this. And then quickly, open source started catching up. And I think, Nisten, correct me if I'm wrong, but I think John Durbin's Ouroboros has a bunch of like function calling instructions in it. [00:45:54] Alex Volkov: So this model and then models who are trained on Ouroboros were also fairly okay with function calling. Mistral just released their update, so Mistral supports function calling. [00:46:05] Nisten Tahiraj: They had about a thousand. About a thousand of function calling datasets in the AeroBoros two, or I forgot. Just look up John Durbin, J O N Durbin, and AeroBoros, A R A I R O B O R O S dataset. And, yeah, apparently there's about a thousand entries in there for function calling. That's by accident helped a lot of the other models be better at function calling too. [00:46:29] Alex Volkov: Yeah, so every other model that was trained on Airbores, which is a lot Hermes includes Airbores data set. They now I don't know if this is by accident or this is now how things work in the merging world. And in the, Finetuning on top of data sets that Finetune on top of other data sets, right? [00:46:44] Alex Volkov: But definitely other. other open source models, no, no support, at least the notion of functional control, and then the eventually we get to the point where there's now a leaderboard like we like. So if we're going to talk about embeddings later, there's an MTB leaderboard for different embeddings model, even though I see Bo in the audience and He's not very happy with how easy it is to game this leaderboard. [00:47:07] Alex Volkov: We obviously look at the open source LLM leaderboards and Yam was talking about submitting a few stuff there and see how it performs and that's being, exploding popularity and merging. So it's great to have a function calling leaderboard as well. And folks at Berkeley that tests models I think API only, I don't know if they're supporting open source at this point, the test models and looks at. [00:47:28] Alex Volkov: How you could expect a performance on different function calling and I think for folks who are building with this it's very cool. So Some of the models that are leading this leaderboard and GPT 4 the latest preview from January is leading this They have something called Open Functions V2, which I I think the organization that pulled this up, Gorilla LLM, is the folks who put it up, and they have an Apache 2 license, and they have an average score on different Simple Function, Multiple Functions, Parallel Functions different scores for all of these tasks. [00:48:08] Alex Volkov: And I just, I want to highlight this and I want to add this to the show notes because more and more we see Mistral Medium entering their Cloud From Entropiq and open source models. And I think for many folks building, agents building with these models This type of interaction with the model is very important, where it's not only a prompt, a textual prompt, and you get something back, you actually need to do something with it, and I think a shout out for folks for building and maintaining this data, this leaderboard. [00:48:34] Alex Volkov: And I think they also released the Gorilla model as well. . Let's move on, I think this is it, folks. I think this is everything we have to talk about in the open source LLMs. [00:48:42] Alex Volkov: And given that Conor, given that Storm is in the area of open source ish, let's cover Storm a little bit. [00:48:49] Alex Volkov: I think this is a good time. Because it also like dances on the on the area of interest that we talked about last time. Do you want to present Storm and talk about this and see how cool this is? [00:48:58] Connor Shorten: Yeah, cool. I guess maybe let me say one more thing on the gorilla. I think it's fascinating going through the functions that they have if you go through the open function, the blog post from Berkeley, you have calculate triangle area, and then you give it the base and the height. And I think that kind of just like super specific functions, having a massive data set of that. [00:49:16] Connor Shorten: It's fascinating that they've, seeing this next evolution of that, but. Okay, so with Storm yeah, there's definitely some intersection between DSPy and the function calling models. With DSPy, one of the one of the one of the built in signatures is that React one, where at React you have thought, action. [00:49:33] Connor Shorten: And so you, it's one way to interface tools. Yeah, the tool thing is pretty interesting. I think it's also really super related to the The structured output parsing and, the please output JSON and, Jason, our favorite influencer of the function calling [00:49:47] Alex Volkov: I just wanna make sure that folks don't miss this. Jason Liu is the guy who you're referring to, and he is, he's our favorite influencer in, in forcing these models to output JSON. I find it really funny that the, a guy named [00:50:00] Jason is the guy who's leading the charge of getting these models to output JSON formatted code. [00:50:04] Alex Volkov: I just find it really funny. Didn't wanna skip this. I wanted to plug this, that joke somewhere, but please go ahead and let's talk about the story. Oh, and a shout out to both Weights Biases and Connor on WayVid, Jason appeared in both places talking about Instructor Library and how to get these models to give a structured output. [00:50:21] Alex Volkov: So definitely shout out for Jason for this, check out his content on both platforms. [00:50:29] Connor Shorten: yeah, awesome. Yeah, it's such a huge part of these, lLM pipelines, like I know Bo is going to speak in a bit, who's someone I consider one of the experts in information retrieval. And one of these big things is like you will retrieve and then you'll re rank and then you'll generate. And if it doesn't follow the output exactly, you can't parse it in the database. [00:50:47] Connor Shorten: So it's such a massive topic, but okay. [00:50:50] Stanford introduces STORM - long form content grounded in web search [00:50:50] Connor Shorten: So starting with Storm, I guess I can tell a funny story about this. Erica and I were hacking on this and we came up with the plan of You start off with a question, and then you do retrieval, and so you're looking at the top five contexts, as well as the question, and you use that to produce an outline. [00:51:06] Connor Shorten: And again, structured output parsing, that outline better follow the comma separated list, so that then you can parse it, and then you'll loop through the topics. And then we have a topic to paragraph prompt, where, you know, you're doing another retrieval now with the topics. And then we have the proofreader and then the the blog to title. [00:51:26] Connor Shorten: So that's the system that we, got our hands on with. And I could probably talk about that better than the STORM system, but it's very similar. With STORM, the difference, so the difference is we're retrieving from, a Weaviate index with Weaviate blog posts. Let's make it as much Weaviate as we can, but like they, so they replaced the specific retriever with with web search retriever. And. So I was playing with that a bit on the weekend as well, using the U. com API as the web search and, it's pretty cool web search and as well as a private thing that you curate. I think that's definitely one of the big topics. [00:51:56] Connor Shorten: Okay, so then the interesting thing is once you've got this, in our case, as a four layer system, now you use DSPy to compile it. So what compiling it entails in DSPy is tweaking the task description as well as producing input output examples. So you have in the prompt, you slightly change it from, you'll take a topic and write it into a blog post. [00:52:19] Connor Shorten: Typically, that ends up resulting in a blog post about software documentation, right? So that's what that ends up looking like. And then the input outputs end up being, like, an example of what are cross encoders. Here's a blog about cross encoders. So you can use that input output to then reason about the new inference, so hopefully that's a good description of what it means to compile these programs, where you optimize the prompts for each layer in the task as you decompose this task into its subtasks. [00:52:45] Connor Shorten: Storm then introduces something that I think is pretty novel. which is how you do that research loop. So we naively just went question to outline and then just instantly flesh out the outline, whereas they instead go from question to perspectives about the topic. And you retrieve from each of the perspectives about the topic, and then you'll, write it, and then it will, I'm not sure how it all gets resolved, but it's, so it's almost like a multi agent system in my view, this kind of like perspective guided to adding personas or like background. [00:53:18] Connor Shorten: So I think that's probably the key differentiator between Storm and then that kind of like blog post system that I described. But so we have open source code on Weaviate Recipes. If you want to see what the, what our four layer program looks like and compiling that with the Bootstrap Optimizer. [00:53:35] Connor Shorten: With the Bootstrap Optimizer is you just run a forward pass through the model with a super high capacity model like dbt4. And then, to get the input output, and then you hope that Turbo or one of the cheaper, or the open source models can can look at those input output examples and then copy the system behavior. [00:53:51] Connor Shorten: There's a lot of other interesting things about this, like multi model systems, even in the Storm paper they compare GPT Turbo, and then they use Mistral 7b Instruct as the judge. Another thing is like earlier talking about re ranking. You might want to have the long context models do re ranking because with re ranking you typically try to give it a lot because you're trying to like, put a band aid on the search. [00:54:13] Connor Shorten: So you probably want to have 20 to a hundred results that go into the re ranker rather than, five to 10. And it's probably also not really a task for the for LLMs anyways. And I think that's another, opportunity for a task specific model, but overall to conclude this thing about Storm, I think for me, the big exciting thing is it's becoming, DSPi is making it super clear, I think, on how to build more than chatbots or just simple question answering. [00:54:40] Connor Shorten: It's I think we're probably within a few months from, anytime you have a pull request, the documentation will be written for you automatically. Probably you could even have an idea and have a pull request created by the model. I'm personally biased by coding applications, but yeah. So the but yeah, this kind of like long form content generation by breaking down each task and then optimizing each part of the task. [00:55:05] Connor Shorten: It's all just really interesting. [00:55:07] Alex Volkov: very interesting. And I had a storm to, from Yijia Xiao to, to the show notes as well and folks are definitely worth checking out because it writes like wikipedia length articles and uses like you. com API or different search APIs to give perspectives and References and very interesting. I want to in the sake of time I want to move so just like to reset the space we've been at this for almost an hour You guys are on ThursdAI. [00:55:33] Alex Volkov: ThursdAI is the weekly podcast and newsletter that's recorded live on xSpaces. And I'm here with several friends and guests and experts in different fields. And we've been covering open source LLMs until now. And I think we're going to move into big companies because we need to cover this. And soon we're going to have some folks to do a deep dive about embeddings. [00:55:51] Alex Volkov: And let me just make sure that the folks know that they're, they can come up. Uh, the big companies, LMs and APIs this is the segment where we chat about OpenAI and Microsoft and Google and whatever not the models that they released for us in OpenWeights and OpenSource that we can run ourselves this is the segment where we talk about API and developments and different updates. [00:56:13] Alex Volkov: So let's run through them. [00:56:14] Mistral releases Mistral Large & Le Chat interface [00:56:14] Alex Volkov: The biggest one from this Monday was Mistral releasing Mistral Large, which we've been waiting for and getting excited about. And also they released a chat version of their models called LeChat. And, um, it's very impressive, folks. Like the Mistral Large now is based on at least some metrics that they released, is second only to GPT 4, and beats Claude and Tropic and Gemini Pro on the MMLU score. [00:56:43] Alex Volkov: And Mistral is vastly superior to Mistral Medium handles 32k tokens of context natively fluent in English, French, Spanish, German, and Italian. It highlights how much Mistral is focusing on becoming the open AI alternative from Europe, because you can go to the chat and there's execute every chat that you have with their models. [00:57:09] Alex Volkov: And basically, Maybe you don't have to have an OpenAI subscription. I think that's what they want to do. But also, this model is available in the API, and it's significant performance on top of everything else on the other languages. And they're aiming for the five top languages in Europe, obviously, and I think it's a Very standard, like a very important move from theirs that they're establishing themselves as this big company. [00:57:32] Alex Volkov: This was why we moved them to the big company APIs as well. The announcement also includes something interesting. They said, we have also updated Mistral Small in our API to a model that's significantly better and faster. [00:57:45] Alex Volkov: The Mixtral 8x7b. If you remember when we announced, when we talked about Mistral releasing API access, we said that, whatever Mistral Next is It's probably going to be medium. So now we have a large model that outperforms pretty much every model besides GPT 4 on different tasks. According at least to them, but also the small model that's like faster and better. [00:58:06] Alex Volkov: They upgraded this like behind the scenes. They're not released that any of this in open weights. Which is the response from the community was partly this, is Mistral releasing a bunch of stuff, and none of the stuff like we expected. No torrent links this [00:58:20] time, no, open models that we can start fine tuning. [00:58:22] Alex Volkov: And I think so first of all, kudos on this release. I've used some of the stuff in the chat, and I'm very happy with the responses. They're fairly quick, but definitely giving good responses. Nisten, I think your perspective from before, from the open source segment is very interesting where they spoil us so much with the open models, with the Mixtral models, and even the 7B, that even large doesn't seem that significantly better. [00:58:45] Alex Volkov: However, just on the metrics, it looks like we just got Another competitor in the ring from, now there's Google, Gemini Pro, Entropic Cloud keeps releasing models that are less performant, at least on LMSys, than the previous models. And now Mistral not only doing fully open weights, open source, but also in the API. [00:59:03] Alex Volkov: And if folks want to build on top. They can. An additional thing to this, they also released a partnership with Microsoft and announced that these models are also going to be distributed through Azure. And I think this is a big deal for companies who maybe don't want to trust a startup that's less than one year old from, from Europe, for example, and maybe their servers are in Europe, maybe the companies don't want to trust their ability to stay up because there's like only 30 people, or, enterprises, they need more stuff like ISO and different things. [00:59:34] Alex Volkov: And so I think it's a big deal that Microsoft is now also supporting and giving us access to kind of these models through Azure, and especially for companies that want stability. I'll just, not stability, just stability in general. I want to just mention that if you guys remember after Dev Day, OpenAI went down for a week, or not a week, but there was like a whole period where OpenAI had a lot of issues on production, and the Azure version of OpenAI stayed stable. [01:00:00] Alex Volkov: Obviously Microsoft wants to sell their cloud, and I do believe this is a very big deal that Mistral is now supported through Azure as well. In addition, Microsoft also announced a small stake in Mistral, and Arthur, the CEO of Mistral, and went and clarified. So first of all their new website with these announcements, again, didn't include some stuff or included the a note that you shouldn't train on this, right? [01:00:22] Alex Volkov: And then our friend Far El here for the second time called them out publicly and for the second time, Arthur Mensch, the CEO of Mistral came and said, whoops, gone. And so it does seem like an omission rather than something they put on purpose and then they remove after Twitter calls them out. [01:00:38] Alex Volkov: Far El, thank you for that for noticing. But also some other folks noted that their commitment to open source, which we discussed before was gone from the website. And they put it back. And so now, like prominently on their website, even though this time they didn't release any open source, any open weights for us this time their commitment for open source is prominently featured on top of their of top of their website. [01:00:59] Alex Volkov: And now there's two segments there. One of them is optimized models, they call them. And one of them is open weights models that they released for the community. As we talked previously in the open source segment their models from six months ago are still competing with something like. The new and cool Gemini Pro 8 billion parameters. [01:01:15] Nisten Tahiraj: It's still a 32k context window by the way, so I measured and after that it completely forgot, and also it was okay. I was expecting as a chat model to be way more chat optimized, but it does feel more like a base model. And yeah, again, I said the comments before, we're too spoiled by all the 7b and Mixtral, Finetunes, and merges. [01:01:43] Nisten Tahiraj: That now this is extremely good and is very utilitarian. And if your business needs it, you should use it because it provides reliable answers. It's not, we were just expecting more. [01:01:56] Alex Volkov: So one thing definitely to note as well, and we mentioned this a little bit, but definitely worth mentioning. So the smaller model is now better upgraded. So if you play with this they also upgraded the pricing for this. And I would also caution folks, the tokenizer they use is a different tokenizer. [01:02:10] Alex Volkov: So sometimes when you measure tokens they may look different. Our friend Zenova here in the audience. Has a tokenized playground in hug face, which by the way, with the rest of hug face also went down yesterday. So I went to check just the length of a string. I wasn't able to, it was sad but now it's back. [01:02:24] Alex Volkov: So that token, I think, measures open the eye, token's length, and Mr, I think has a different one. So when you calculate pricing for use, definitely make sure that you're calculating the right thing. Yes. No, you're welcome to come up and tell us about this. So one last thing on Mytral is that it supports function calling as well, which is, I think is a big deal. [01:02:41] Alex Volkov: And we mentioned this before in the function calling leaderboard. And now mytral models can also respond to your RAG applications or whatever with actually the functions that you should call, which is I think super cool. And the industry moves there and it shows again, the open AI can come up with something. [01:02:57] Alex Volkov: a year ago and basically set the standard for how things should look. I actually don't know if assistance API is going to be like this, but I do know that, for example, we talked about Grok and Grok supports the OpenAI standard. And many of these, I don't know if Mistral does, but many of the like Together API and other I think Perplexity as well, all of them have their own version of their API, but also you can just replace whatever code you wrote for OpenAI with just like a different proxy URL. [01:03:24] Alex Volkov: And then you basically use the same structure that OpenAI innovated on, so that I think is pretty cool. Moving [01:03:32] Nisten Tahiraj: Yeah, [01:03:33] Connor Shorten: also just a note is that the OpenAI PIP package allows you to actually call any any URL doesn't matter if it's if it's OpenAI or not which actually uses that standard. It is very easy to drop in any replacement to the OpenAI [01:03:49] Alex Volkov: Yeah, including local ones. If you use LM studio, our friends on studio, shout out Yags or Olam, I think both of them will expose like a local server when you run the open source models. And then you can put in your code, like your local URL that runs the server with the local model. And then your code will also work, which is, yeah, thanks for all. [01:04:08] Alex Volkov: This is like a very cool thing that people may have missed. The same can be said by the way about Copilot. It's a little harder, but basically you can replace the Copilot infrastructure in VS code with like local models if they support it, if you go through settings. But moving on to. I guess moving on to GoogleTees is Genie, right? [01:04:26] Alex Volkov: GoogleTees Genie, which is a, which is quite incredible. You take one image of something that your kid drew that has like a character, and then you provide this into this like video type text to video or image to video model. And in response, you get like a full world that is interactive and looks like this character is kind of in the same style transfer and it looks pretty much the same. The character is like interacting in this world. Seeing this is unbelievable because it just shows that, we're very close to being able to take one picture and start animating this. And very worth like adding this to the top and adding a video for this. [01:05:05] Alex Volkov: It's really hard to explain in words and I haven't read any of the paper, but Genie was really like also mind blowing as well. From Google, and they only teased it, so we don't know actually if they're gonna release this. Far El, you wanted to comment? I saw your [01:05:20] Far El: sure. It's If any of you have watched Sentex's YouTube video like a few years ago, about GameGAN from NVIDIA. It's basically GameGAN, but with generative AI. And it's pretty awesome, because it means that we're all headed towards the direction of basically interactive rendered worlds. [01:05:43] Far El: And Sora is one, one extreme end of that with really high quality text to video. But then what happens when you actually add actions into the loop? And that's what basically Genie does. So we're probably going to see the marriage of both methods, both architectures very soon. Very exciting work for sure. [01:06:04] Open AI to buy Wordpress & Tumbler data [01:06:04] Alex Volkov: And so I think most of the open and big companies stuff we covered. One, one quick thing before we move on, [01:06:10] Alex Volkov: openAI opens up fine tuning for 3.5 and also OpenAI in the news again this week because wordless. and Tumblr. Basically, I think both of them are the same company, Automattic. They're preparing to sell user data. And it sounds scary, but honestly it's all public and probably will scrape anyway. [01:06:30] Alex Volkov: And still, they're preparing to sell this, probably more structured. and maybe more licensed to open AI and mid journey. So that's very interesting because Tumblr had a bunch [01:06:40] of images and probably was scraped to an extent. WordPress, definitely so just to clarify, this is not WordPress, the platform where everybody can use the open source platform to run their websites. [01:06:51] Alex Volkov: That's not what they're selling. I don't think they, they can but WordPress. com, I think, is where you can host a blog for free without knowing how to raise a WordPress platform. So WordPress has the open source system that you can run your blogs and websites in that runs like 30 percent of the internet or something crazy like this. [01:07:06] Alex Volkov: But also wordpress. com is the place where you can host your blog and basically when you signed up and created your blog there, you maybe didn't know, the information is there to sell. So like Reddit supposedly selling. Reddit's information to Google for 200 million that we talked about last week. [01:07:24] Alex Volkov: Automatic is now trying, basically trying to extract money based on their data, where previously this data was scraped. What's the benefit for OpenAI? Obviously, now there's a lawsuit with the New York Times, whether or not this is considered fair use, and whether or not Open's AI model, OpenAI's models, can spit out full New York Times articles. [01:07:44] Alex Volkov: So there's a whole debate about this and there's going to be a lawsuit because they didn't achieve a similar deal with New York Times. Although it was reported the folks from OpenAI actually did talk with New York Times to try and have more of a structured access and licensed access. And WordPress is definitely a huge chunk of the internet and now some of that information is going to go into these models in a more structured and licensed way. [01:08:12] Alex Volkov: And moving on to diffusion models before we jump in, because there's a bunch of updates there, and I think Jeanne takes us a little bit into diffusion models, so let's see if we have a thing for this, yeah. [01:08:41] Playground open sources a new diffusion Foundational model [01:08:41] Alex Volkov: All right, so As I said before, we don't cover this at length. I know there's a bunch of other spaces for AI art and fusion specifically. But when we do, it's because something very big happened. And this week was a huge week as well. And so I just want to shout out that We had two foundational models, basically, and then another thing that just broke my jaw, and we're going to talk about this, [01:09:01] Alex Volkov: playground. Playground from the previous Suheil Doshi, I think is his last name. He previously was in Mixpanel. He started building a browser called Mighty and then he switched fully into AI. And I think a year ago started working on Playground. [01:09:17] Alex Volkov: Playground is an interface that like super fast and lets you generate a much of images, and it's just an interface on top of, or at least previously it was an interface on top of, DALI and Stable Diffusion. And they kept giving away for free all of these models and image generation. And basically they collected their styles, etc. [01:09:36] Alex Volkov: And they've collected all this information of what people actually do preference on. And now they released an open model, a new diffusion foundational model, which we haven't had for a while. If you guys remember, we talked about SDXL Lightning, which is based on SDXL. We've talked about, um, Stable Cascade, which is also related to stability. [01:09:54] Alex Volkov: We haven't had a, like an open model for generating images in the wild for a while. And Playground released their model called Playground V2. 5. And the cool thing about this is that they say first of all, it looks great on realistic stuff. Secondly, they say that on User preference on internal 1000 tokens, they significantly beat the baseline for DALL E, for Mid Journey, for the previous version of Playground, and SDXL as well. [01:10:23] Alex Volkov: And by significant they beat internal preference again SDXL 1. 0 gets like 70, 17 percent and their model, their new model gets 82. Which is like a quite stark, like a big jump in capability and improvement as well. They also get improvement on top of Midjourney, the latest of 5.2 version, which Midjourney is like really good or realistic. [01:10:44] Alex Volkov: And so what they excel at is realism and just different, I think they also mentioned different ratios. So if, like most of these image models, they've been trained with certain 10 24, but 10 24 for sdl, for example. And, when they generate something in a different ratio, it looks different. [01:11:01] Alex Volkov: So they also claim that their model is actually significantly more performant in different ratios as well. Definitely shout out to Playground folks for working on this awesomeness, because Who's gonna say no to another model? And there's a demo from, I think, Model Labs that actually makes this work really fast. [01:11:17] Alex Volkov: If you guys remember last week, I talked about a thing that I built with SDXL Turbo and Grok. And obviously SDXL Turbo is super fast. or SDXL Lightning is super fast. Compared to those super fast examples, the Playground image generation is just night and day. It just looks so real. It's quite striking. [01:11:37] Alex Volkov: So if you're looking for any updates in that area, definitely check out Playground. And I think because it's a model they released, you can use it for free. The only thing that I don't know about is the support in the community, kind of stuff, if it supports Confi UI or some stuff like this, but they just released it, so I'm sure support will come. [01:11:56] Alex Volkov: And obviously, the Loras and everything else in this community is very interesting to see. There's also a Hugging Face demo. And then, the second thing in image generation real quick, is Ideagram. Ideagram. We've talked about before. It's a startup that came out of folks who worked on the image and stuff at Google and apparently weren't very happy with the slowness of the release. [01:12:17] Alex Volkov: And while Google and its image generation is suffering from bad news and is in hot water because of different Prompt injection that they had, and even, we didn't mention this, but mentioned this in the beginning. Sundar Pichai released an email to all of Google and said, hey, we had mistakes, we offended some of our customers, we need to do organizational changes. [01:12:35] Alex Volkov: Which is not a small thing from a head of the company to admit this bad of the release. Ideagram was created with folks. from Google before, and they released it for free. And I think they just renounced Ideagram 1. 0. And the best thing about this, I think is just text. They, everybody is no focus on different things. [01:12:56] Alex Volkov: But if like all these models, they generate text to some extent, DALI can do text, but it's not like perfect. Ideagram's text. XGeneration is super cool. I really, so far I used it multiple times just to answer somebody on X, reply with just a text, for example like for Hug Face, I think I sent them like a thank you note with just text. [01:13:13] Alex Volkov: And it's really cool to have a model that's like very good at presenting and generating text with the imagery that you want. So Ideagram 1. 0, they also announced a investment from A16z and really their text looks super cool. I was able to do something. That not other models could do. I was able to ask it to generate a hashtag ThursdAI And if you think about this text is not in the training set because you know We came up with the concept and a hashtag like confuses these models And I think this was the first model that was able to actually Not screw up hashtag ThursdAI Fully. [01:13:50] Alex Volkov: Cherry pick still, so three out of four still wasn't like perfect, but definitely this is the best text model that we have Ideagram check it [01:13:57] Alex Volkov: out. Yeah, go ahead [01:13:59] Aditya Kusupali: Yeah, just randomly in the audience, I noticed we have one of the creators, I think it was one of the top 10 Hugging Faces pretty recently, so their data out of GPT 3, and they also have a, what's called a DALI 3 dataset, training dataset, but yeah, they released a new model recently too, I posted it up for you, so if we have some time after the interview, maybe we can bring them up and stuff. [01:14:25] Alex Volkov: Yeah let's see if where our second guest is. Oh, he said he's going to join in 10 minutes or so, so we have a little bit more. And the last thing that I want to cover, and I actually want to, actually go to my profile and paste this, because you guys, you have to see this. And if you haven't seen this Okay, so first of all I'm going to post an image of I'm adding this onto the show notes now, it's the last pinned tweet image of a very happy sheep, they all say we're doomed and No, this is not the one, I said this shirt, that's, hold up, yeah, this one, we're doomed, and the text there is really cool, and the cool thing about the text is style transferred into the image itself, so it looks like part of the image, [01:15:00] But this is not what I want to post, I wanted to post the jaw breaking video from Alibaba from a model that they teased and hopefully will release soon called Emo. [01:15:13] Alibaba teases EMO - a way to animate and make avatars talk and sing from 1 image [01:15:13] Alex Volkov: And folks, I don't have a button for this. I don't have a musical tradition. I will just say that if you remember, and if you were here on, on Thursday, I when Sora was announced and released, if you guys remember, this was live, I think two weeks ago, we had Sora release and we were just like freaking out live on stage here on Thursday. [01:15:30] Alex Volkov: I, because like our job was collectively breaking from what we were seeing. Sora showed. significant jump in capability for image sorry, image to video or text to video generation and coherence throughout the scene and longer generations. And since then, OpenAI has been SORA posting. That's what I call it, SORA posting on TikTok. [01:15:50] Alex Volkov: So if you're on TikTok and you don't follow OpenAI, they literally opened a full account that just posts SORA videos on, or SORA posting, on TikTok. And since then, the amount of videos that they released there just shows the capabilities of that incredible model. It does look like the ChatGPT moment for video generation based on what they released. [01:16:07] Alex Volkov: I think that emo from Alibaba is definitely one of those moments. And actually, it's really funny because the Alibaba folks took one of the Sora generated videos, if you remember one of the main ones, is a woman not the main ones, one of the first ones is a woman walking through Hong Kong, wearing sunglasses, and it zooms into her face, all of this video generated it's quite crazy. [01:16:29] Alex Volkov: that we're now like, oh yeah, of course it generated the woman walking to Hong Kong wearing glasses, but, it's still mind blowing. So the emo folks from Alibaba, they took that video, took a still from that video, just a still, not a whole video, and made that exact woman sing Dua Lipa's song, and this is now pasted on top of the, on top of the space, and, folks, my jaw dropped when I saw this, and then dropped again because I started looking at all the details. [01:16:56] Alex Volkov: I did a little deep dive into image generation, avatar creation, basically taking an image and making it sing or lip sync. And usually those models, they move maybe the mouth a little bit, some of them move the eyes. This model makes this from one image, one image only. It makes the eyes move independently, it makes the eyebrows move independently, obviously the mouth. [01:17:17] Alex Volkov: I saw earrings get animated, I saw vocal muscles in the throat get animated where, if somebody talks those things, you can see their throat move differently. I'm noticing all these, all these things. The woman in the video that I'm referring to wear sunglasses. So most of these models would move the sunglasses to an extent. [01:17:35] Alex Volkov: These sunglasses like stayed exactly the same place. So the coherence of this model is way beyond anything that I've seen. And I've researched this field and I used D-O-D-D-A-D, I used tulip, I used all these like tools and, just the creation would be able to use with something like Sora plus something like this emo thing. [01:17:50] Alex Volkov: It just opens new horizons. And many of my friends in AI art are looking at this and like in disbelief. Because it really feels like the Sora moment as well. So I just want, I wanted to highlight how exciting this was for me and how how huge of a jump this was from everything we've seen before. [01:18:07] Alex Volkov: Reactions from folks on stage. what do you think when you saw emo? Same as me. Existential dread. Anything else? Yeah, same as me. All right. So I, it looks like our, yeah, Nisten go ahead and I'm going to take a look. [01:18:22] Nisten Tahiraj: I just want something like this that's like small, real time, and cartoonish, so I can just use it as an assistant. That would be great. I'm impressed, but I just want like a small, tiny one. I want clippy. I want the actual clippy. Yeah, [01:18:37] Alex Volkov: They didn't animate Clippy, but I found it very interesting that they animated the Sora generated woman with the voice of Mira Murati, the CTO of OpenAI. They like took her voice and embodied one of their creations with this voice, and I found this like very interesting choice on their part. I will say while Aditya comes up, and Aditya if you can hear me, I'm sending you a request and if you cannot, oh yeah, there is. [01:19:00] Alex Volkov: Found it very interesting that they Haven't released the model yet, but they did say we're committing to open source. We're going to release this and their GitHub for Ego is open, but there's no commits there. It's just like a readme. So hopefully they're going to release this. And hopefully we'll get to a point where we can actually, Nissen, like you're saying, have a actual assistant in near real time with a fake voice or generated voice, actually read out whatever LLMs tell us. [01:19:25] Alex Volkov: And I think this last thing I'll say here before I move on to the interview is this adds to this notion that I think we saw from Logan from OpenAI, where a chat is not the final interface for these things. I think embodiment like this is one thing that moves us forward. [01:19:40] Deep dive into Matryoshka embeddings with Aditya Kusupali & Prateek Jain [01:19:40] Alex Volkov: All right, folks, this has been the updates, and now we're moving to a more of a deep dive interview, and I'm very happy to introduce Two guests here, and two guests, I'm getting a little winded, so forgive me. [01:19:54] Alex Volkov: But I want to say hi to Aditya Kusupati and Pratik Jain, and thank you. And folks, feel free to unmute yourself and talk and, call out. But basically, welcome Pratik, welcome Aditya. How are you guys? [01:20:05] Aditya Kusupali: thank you, [01:20:06] Prateek Jain: Alex. Thanks so much, Alex, and thanks everyone for listening. [01:20:10] Alex Volkov: I, I'm gonna set this up and I think particularly, I noticed you on my timeline first, and then I saw Aditya's tweets as well, where we've talked about OpenAI's new embedding models, and one of the things that was like very interesting back when they released this, and this is how I got to to talk with you guys is They added some new parameter in their new models. [01:20:30] Alex Volkov: So they had Ada 002 before, and then they said, Hey, we're releasing two new models, Embeddings version 3, and they have a way to specify dimensions. And so previously on ThursdAI, we talked about embedding models, we talked about the MTB leaderboard that Huginface has, we have folks from Junaid that also released top of the line embedding models as well in Friends of the Pod, and we definitely looked at open source models, and in comparison to something closed source like for example OpenAI, and dimensions were a big thing in that whole area. Then OpenAI released something that you can specify number of dimensions and this raised an eyebrow and was like, oh, that's interesting. I don't even know what this is about. And then I think Pratik, I saw your tweet saying, hey, congrats OpenAI. Unfortunately, you didn't mention us. [01:21:19] Alex Volkov: And then somebody from OpenAI reacted and said, Oh, actually, yeah, we do use something called MRL, and they added this to the blog post. Pradeep, could you talk about that, before we dive in on what MRL actually is? Could you talk about what they added and why? And yeah, just talk about this this phenomenon of them not adding you to the blog post. [01:21:38] Prateek Jain: They had done the work on their own and everything. It's just, and they did release like really strong embeddings, like the results on MTAB eval boards looked really good. Definitely many congratulations to them. Only thing was that they had released this new thing, as you mentioned, called shortening embeddings. [01:21:54] Prateek Jain: And the output structure in some sense seems very similar to what Mateuszka representations or these nested representations do. And we do know that they were at least aware of Mateuszka representations because through some of our earlier conversations at least some of the research scientists had reached out to us and had talked to us about some of the details about Mateuszka representations. [01:22:13] Prateek Jain: It's felt a little bit like, against the spirit of open science and pushing a scientific boundary so that's the only reason we highlighted that it would be good if either the the initial work can be either cited or maybe use the same name I think they were very gracious in particular, the person who had written the blog, I think they said that, yeah there was a miss on their part and they have updated the blog now, all good. I think when we do open research and publish and discuss ideas, I think it moves the field very fast and helps everyone. We are definitely all up for it. [01:22:49] Alex Volkov: Yeah, absolutely. Want to talk about when you guys released MRL. This was way before the explosion of LLMs and ChatGPT came to the scene, right? [01:22:56] Alex Volkov: You released MRL, Matryoshka representation back in 22, right? Almost two years ago, like a year and a half ago? [01:23:05] Prateek Jain: Yeah. [01:23:06] Alex Volkov: And so talk to us, maybe give a brief explanation of what like I think folks are generally okay with embeddings in, in the audience here, but maybe dimensionality is still somewhat of a escaping field. [01:23:18] Alex Volkov: Would one of you tackle the [01:23:20] task of explaining what dimensionality means in a very like popular science way so we can then dive into how adjusting dimensionality actually helps performance and different things. [01:23:29] Prateek Jain: So generally, what happens is if you have, say, some text data, right? So let's say you have a string of thousand 24 tokens or let's say you have an image a 64 by 64 image what we like, what computer, in some sense would want to see them as a set of numbers. [01:23:47] Prateek Jain: Or a vector of numbers through this incredible line of work around embeddings what we are able to do is we are able to embed these images or text or whatever data object you have into a fixed dimensional vector. So by that, what I mean is you might have a 64 by 64 image, but you can write that as a series of, let's say, 128 vectors, numbers, right? [01:24:11] Prateek Jain: So that is what we call dimensionality. That is, it is 128 dimensional vector that we want to work with. Why is this interesting? Because if you have a 64 by 64 image and you just change some pixels let's say only 1 percent of the pixels. Those changes would not even be visible to you, but when you compute, let's say, the distance between these two images along pixel space that is, if you're just subtracting two images from each other pixel by pixel, the distance might seem very large, but in reality, semantically, both of them mean essentially the same. [01:24:41] Prateek Jain: So what we ideally want is some of these embeddings which capture the underlying semantic structure of the data object of, let's say, image. Let's say, there are two images, both of them contain Cat, and very similar pose. We would want to have them being represented within our machine as very similar sort of, objects and that is what these embeddings or semantic embeddings are able to do. [01:25:03] Prateek Jain: So generally there are multiple techniques to take, as I said, either the image or text or audio, whatever you have, and embed it into, say, a fixed dimensional representation that is a fixed number of floating point or integers. Now, generally, these Representations are like, rigid. [01:25:21] Prateek Jain: They are fixed. That is that is, let's say a person a designer has to a priori say that, okay I can deal with the 128 dimensional representations for my image and on basis of this, I can run some sort of classifier or some sort of retrieval algorithm to retrieve similar images or classify the image into some particular class. [01:25:39] Prateek Jain: So generally, that decision is made a priori that I will be forming it into 128 dimensions because 128 dimensions, let's say, are able to give me the accuracy I want and I will be able to deploy them in my system because that's another sort of key part. Whenever you are deploying them, the dimensionality of the embedding can be a critical thing. [01:26:00] Prateek Jain: Let's say, if you want to do retrieval the cost of Retrieval is almost directly proportional to the dimensionality of the data point. So we so the decision is made a priori. So for example, like earlier embeddings that came out from OpenAI, they made that decision that, okay, these embeddings should let's say be, I think, 1024 dimensional or something like that. [01:26:19] Prateek Jain: So you just had those 1024 dimensional and not so good part about that is that now everybody who wants to use those embeddings have to change their system to suit their 1024 dimensional representation. So some people who might be running, say, some sort of retrieval engine on 64 dimensions, they will need to now scale up everything, change how they are doing retrieval, how their indexer works, how their serving works, to fit to those 1024. [01:26:46] Prateek Jain: And that's not ideal, right? So the idea behind Matryoshka representations was that can we bring flexibility in these embeddings? That is, while we are giving out 1024 dimensional embeddings, can somebody come and read off just 64 coordinates out of it so that, they don't need to change their entire serving stack? [01:27:07] Alex Volkov: So I wanna slide in here with a question before we get to your guys solution in dimensionality flexibility, which is very cool. So you're saying the iPriority decision basically means that I as a developer, let's say, if I used whatever OpenAI has given me or any other, uh, rigid structure, I had to basically abide by their rules of how much they decided how in depth those embeddings represent my concepts, correct? [01:27:31] Alex Volkov: And could you talk about maybe before we dive into dimensionality, how this affects actual retrieval? Is more embeddings always better? There's a thing that I heard yesterday that somebody mentioned. It's called the curse of dimensionality. And I really wanted to dive in and hear about what this means. [01:27:46] The curse of dimentionality [01:27:46] Alex Volkov: Is, because we've talked before and There are embedding models with like 8, 000 dimensions or so. And I heard from Beau, who's in the audience here, who may join us as well, that's not always the best case. For many reasons, not only speed as well. Could you talk about the curse of dimensionality and is more always better? [01:28:03] Prateek Jain: So that's a great question, right? So definitely more dimensions intuitively should help you capture more and more information about the data that you are trying to embed. Obviously, like beyond certain point, it becomes, starts to becoming complete noise, right? So for example even if you go back to the image example that I was giving, you have a 64 by 64 image. [01:28:24] Prateek Jain: You can think of it that as a 3600, like about 3600 dimensional vector, right? And if you want like a very precise embedding then maybe that 3600 dimensional vector is what is capturing everything about that image because that is roughly, that's precisely how we are seeing that data point, right? [01:28:40] Prateek Jain: But the bad thing about that sort of, representation is that it is not capturing the semantic information. It is also bringing in a lot of noise. You would there is some sort of sweet spot at what kind of dimensionality of data you want to stop at, right? That's one part of it. [01:28:55] Prateek Jain: But when you come up with these representations, they are going to be used in some downstream task, right? As I mentioned earlier some of the downstream tasks are I have this representation of the image. Now do classification for me. So I will run some sort of classifier on top of this representation of the image to say that, okay, whether this image has a cat or a dog, right? [01:29:17] Prateek Jain: Similarly, I can say that, okay, I want to retrieve most similar image to this given image in my database of all the images. So I might have an entire database of animals. I give you an image of a particular cat, and I want to retrieve a cat which is most similar looking, maybe in similar pose, similar situations, right? [01:29:35] Prateek Jain: So these models or these embeddings are used in this downstream task and to use them in these downstream tasks, we need to, we are also then bound by the realities of those downstream tasks. For example, if you want to do classification and you have only let's say, 200 data points to train the classifier, Then a very high dimensional embedding is not great because that will then give you very poor performance, like your model will overfit, it will just like mimic whatever it is seeing on training data and it will not generalize to new test points. [01:30:07] Prateek Jain: So it can be catastrophic. Similar situation happens in even your retrieval or nearest neighbor search. Kind of thing there, that is, if you're very high dimensional embedding as you mentioned earlier, like there's this curse of dimensionality that applies, which might mean that my nearest neighbor search is not working well, especially if I'm doing any kind of approximation, and I might get essentially garbage out of that situation. [01:30:30] Prateek Jain: So that's why, based on the downstream task, The amount of training data I might have, the serving realities there, that okay, how much latency I can spend or how much compute I can spend in serving, I might have a sweet spot into that. Okay, this is the dimensionality that works best for me. And I want to ideally want to select that and work with it. [01:30:50] Alex Volkov: I see. And Aditya, it looks like you can now join, and I also wanted to follow up with you because Partik is talking about and Partik, the examples you gave are image embeddings, and that's great, but I think one of the huge things that happened since you guys raised the paper is how much LLMs are being used for different things as well, right? [01:31:07] Alex Volkov: And I think this led to an explosion in vector databases, and they start embedding, and I think at least for many of the developers who use these, like LLMs, text embeddings or at least they started with text and now it's like multi modal. This is like the highest the highest use currently in React. [01:31:23] Alex Volkov: Would you maybe Aditya, would you want to expand on how much this whole field started heating up with like vector databases now storing every embedding? I definitely didn't hear about this up until a year ago. Would you want to like chime into this and how your work is now like super relevant to, to this whole new world?[01:31:40] [01:31:40] Aditya Kusupali: Yeah, Yeah, as Pratik said, I think Curse of Dimensionality even applies in vector databases because you have to search through things. And the major thing is you also need to think about storage, right? So let's say you want to index a billion documents. And if you want to do everything with, say, 1024, you're going to have to use about a terabyte. [01:32:00] Aditya Kusupali: Or four terabytes worth of data for storage. And a lot of people might not be willing to do that. So how people typically do that in vector databases is they store one copy and when they're trying to do some processing on top of it, they do some sort of compression. It can be a lot of things. [01:32:18] Aditya Kusupali: And It works great, but the thing is, it's a lot of post processing, and you also need to store the actual embeddings in your vector database store. I think with the data which keeps growing and growing, and there is no way for you to control the total amount of data. You should probably figure out a way to make your Representations much more compact, much more accurate. [01:32:40] Aditya Kusupali: I think that is where a lot of oversight was there for the last few years. Again, vector databases existed even before last year, but they blew up because of the RAG applications. And I think in Matryoshka case, as OpenAI said, it gives you the flexibility to just store 64 dimensions if you want, and that should just be it. [01:33:00] Alex Volkov: And 64 is way smaller than the previous dimensionality that they said, I think 1053 or 1024 or so. And also, I would be remiss if not to mention that video is coming into play right now. large multimodal models. Now, they're not only understanding text and images. Now, like we're talking about video embeddings, for example, and being able to represent those. [01:33:21] Alex Volkov: And when you talk about storage costs, et cetera dimensions definitely affect that and also speed of retrieval and comparison. So let's move on to talk about cause you guys wrote the paper before this explosion, but definitely the concepts existed. I want to hear about what Matryoshka representations is and how it affects dimensionality. [01:33:38] What are Matryoshka Embeddings? [01:33:38] Alex Volkov: Specifically from being able to choose during which process, and I would love to hear from you the brief explanation, then we can dive in and ask more questions. [01:33:47] Aditya Kusupali: Sure. [01:33:48] Prateek Jain: Let's take [01:33:48] Aditya Kusupali: the running example for the excited let's say there is a 1024 dimensional representation of your image or let's like, let's keep it to 1024 for now. And so you're trying to basically fit a bunch of learned attributes. So it could be some version of color, some version of Texture, et cetera, which is being fed into these things. [01:34:08] Aditya Kusupali: So that is what these embeddings are learning. And they're extremely good in a lot of semantic tasks. If you want to find a similar looking dog, it's much more easier for you to search in this space. So that's the goal, right? Ideally, until now, when you wanted to do things faster, you took these embeddings and you did some sort of compression, most likely some notion of PCA or low dimensional projection or some sort of quantization, okay? [01:34:35] Aditya Kusupali: And that's how you used to do it. So there is an additional processing overhead on top of the existing embeddings for you to get this done. We wanted to fix this problem because this additional overhead need not always give you the most accurate solutions. So the motivating goal for us was to figure out if we can pack the information in this 1024 such that we don't have to project it into low dimensional space or do any post processing to get a 64 dimensional embedding. [01:35:04] Aditya Kusupali: But rather? Just take the first 64 dimensions of this vector. So if there is a collection of 1024 numbers, I want you to be able to cut it off at the first 64 and say this is a 64 dimensional embedding which is as good as any 64 dimensional embedding you can ever build. That makes sense? And this was the goal. [01:35:24] Aditya Kusupali: So this is the final embedding should look like this. And that is what we try to do. And it turns out Training these things are so simple that it's literally what you think. If you want the 64 dimensions of the first 64 dimensions to be the most important thing, you optimize the same loss function you are doing for 1024 on the 64 dimensions. [01:35:45] Aditya Kusupali: Let's say you are doing some text embedding training, where you are trying to pull two relevant text embeddings together and two irrelevant text embeddings farther. And there is a loss, which is typically contrastive, which tries to do this in 1024 1024 dimensional space, you also do it for 64 dimensional space. [01:36:05] Aditya Kusupali: That's it. So you now have two losses instead of one, and at the end of the training, which again does not take any other extra cost than as if you're training a 1024 dimensional embedding, will give you the first 64 dimensional embeddings, which are as good as any 64 dimensional embeddings you can ever trace. [01:36:22] Aditya Kusupali: And that's pretty much it. So you can repeat this for multiple dimensions. So not just 64, you can do 64, 128, 256, and so on. Now you have this. Chunks of representations inside this 1024, which can cater to a wide variety of audience, depending on their use cases. And a lot of times people don't care about precision. [01:36:44] Aditya Kusupali: If recall is all you care about in your retrieval applications, you can just use 64 dimensions. And if you want more precise information, as Fatih said, you can encode more information in higher dimension embeddings, go to 1024. If you have lesser number of data points and you're not able to cluster things properly, Go for smaller dimensions. [01:37:02] Aditya Kusupali: So the flexibility just opens up so many things which were probably infeasible before in hand because you had to do some sort of post hoc compression or pre processing on post processing on top of it and which led to slightly lesser accurate things. So it just didn't allow you to do all of these things on the fly. [01:37:21] Alex Volkov: Wow just to Sum up to see if I understand this. I'm looking at and unfortunately this medium is audio only, but I think it's very helpful to see visual representation of this. You're basically front loading all, most of the important information into the first 64 dimension, 128 dimension. [01:37:37] Alex Volkov: And you're saying that precision for specific use cases like RAG could still be as good as like with 124 dimension. And that sounds to me incredible. [01:37:47] Aditya Kusupali: Let's take an example, right? Like in your RAG, all you care about is 10 blue links, which need to be in the top 10. That's it. You don't care if the first link is the first one or the last link is the last one. There is some evaluation saying that there is a a utility for positionality, but most of the cases, if you get 10 relevant documents in any order, that's all that. [01:38:06] Aditya Kusupali: You matter. You don't care if the best document is at the top or at the 10th thing. So if you feed in all of these things into your LLM, LLM will forget it. So this is the case of recall. You don't care about precision. So your ranking only cares about getting the most relevant 10 documents in the first 10 and not how relevant they are in within themselves. [01:38:27] Alex Volkov: I see. I want to [01:38:29] Alex Volkov: also [01:38:29] Prateek Jain: bit more nuance there sorry just to add a little bit more nuance there in many situations, what might happen is, in your RAG rather than even getting, let's say, top 10 links that Aditya said, suppose I get top 100 links, right? [01:38:42] Prateek Jain: And those top 100 links, some of them might be completely useless, completely rubbish. But as long as those correct top 10 links are somewhere they are sitting in top 100 link, I'll be fine. That is, after that I can do refinement. The rough structure here would be that you will Take, let's say only for 64 dimensions or coordinates or maybe only first 32 coordinates from mrl and do those retrieval of top hundred links. [01:39:06] Prateek Jain: Once you have those top hundred links to get the correct top 10 links, we can do them further rescoring based on full, let's say 1,024 dimensions and get like those things. And now, because everything is nested, those embeddings are already computed and I have with me, right? So I can first say that, okay, for the first phase of getting top a hundred. [01:39:25] Prateek Jain: I can use 32 dimensions. And then in the second phase of doing that rescoring, I can use full dimensionality. Sorry for cutting [01:39:34] Alex Volkov: No, that was great. Great addition. And I want to think rescoring and re ranking. Are you referring to the same thing? Like some folks like take the initial results and then they try to rank like what was the most appropriate ones. I think, does this represent the case that you guys talk about where the initial information is not really necessary for the first responses. [01:39:52] Alex Volkov: And then we're going to run another tool like Cohere. Sometimes those folks do re ranking with Cohere and then you'll like judge. The importance [01:40:00] of those and then sort them in the secondary process. [01:40:02] Aditya Kusupali: off. Yeah, that's pretty much that's a relevant thing. But I think Joe Christian Begum is in the call from Vespalic. He's a proponent of late interaction. So you can do a lot of other re ranking methods. But in this case, what Pratik specifically is saying is, let's say you recall with 64 dimensions, and you can rescore with 1024. [01:40:23] Aditya Kusupali: You can use the precise 1024 to just rescore in case you ever want to use it. And this is all from the same MRL embedding. [01:40:33] Alex Volkov: Alright, so moving on, I think Aditya, I heard you say also that in the use case of LLMs for example, where again, you guys built this before the explosion, in the use case of LLM and RAG some amount of this is offset to the LLM itself. After you retrieve and you provide this data to LLM it can do some of this work for you, which I guess why your work from a year ago or a couple years ago found newfound relevance. [01:41:01] Alex Volkov: But then I think you followed up with another paper a year ago and at ANN, right? Could you talk about how this applies to Matryoshka Embeddings as well? I would love to hear additional work in this area that you guys did. [01:41:15] Aditya Kusupali: Sure when Hrithik was talking about retrieval, he also mentioned that you typically do a nearest neighbor search. So the goal is when a query comes in, you embed it into the same space. Documents, say, let's say a billion are encoded in the same space, your target is to find, say, top 10 documents which are most relevant. [01:41:32] Aditya Kusupali: And the way you do it is nearest neighbor search. So you just try to find which vectors in your database are the closest for queries. But the thing is, again, as Pratik said, like the cost is directly proportional to the dimensionality, as well as the number of data points. So it's linear in terms of number of data points and dimensionality. [01:41:50] Aditya Kusupali: So to reduce this cost at web scale, so there is no way Google can ever So things if everything is every single data point has to be explicitly compared. So there's an idea called Approximate Nearest Neighbors, which has been there for the last 25 years or so. The goal of Approximate Nearest Neighbors is, instead of touching all the 1 billion points to get top 10, I'm going to touch, say, something like 10, 000. [01:42:12] Aditya Kusupali: So I'm only going to search 10, 000. by somehow partitioning the space and only cleverly looking at the places I want to look at and get to the 10, 000. And in those 10, 000, I'll do more exhaustive search and find the top 10. Okay, and this is the Approximate Nearest Neighbors. And the simplest way of thinking about Approximate Nearest Neighbors is a tree structure. [01:42:32] Aditya Kusupali: So you have a billion points. You are basically building a huge tree structure by using clustering. So a billion points can be clustered into 50, 000 clusters, which can further be clustered into 50, 000 each. And eventually your leaf nodes, like the final leaf node, will have 100 data points in each of the leaf nodes. [01:42:48] Aditya Kusupali: And this is a typical Tree based data structure, which a lot of people use for Approximate Nearest Neighbors. In case anyone is interested, you can go check FAI's library from Facebook. It's a very good resource for all of these things. This is Approximate Nearest Neighbors and it plays very well with web scale systems. [01:43:05] Aditya Kusupali: You want any of your embeddings to play well with Approximate Nearest Neighbors if you want to scale to web missions while powerful can you hear me? [01:43:12] Alex Volkov: yeah, we can hear you, cut off for a second, now we're back. [01:43:16] Aditya Kusupali: Okay, so matricial representations, as Prateek said, again, like you can use 64 100 documents and re ranking for say with 1024 to get the top 10. This is while sound in principle. When you try to do this in systems aware settings, this does not scale well, because these 100 documents need not be sitting on the same machine, they need not be co located, all of these things, there's so many systems considerations which start blowing up, and Approximate Nearest Neighbors directly handles this. [01:43:46] Aditya Kusupali: Approximate Nearest Neighbors ensures that Similar documents are in similar chunk of your memory for your systems to take care of a lot of these things. So we wanted Matrioshka representations to power better approximate nearest neighbors. That's why we came up with ADAMS or Adaptive Approximate Nearest Neighbor Search. [01:44:03] Aditya Kusupali: And the goal here is, again, it's When you're doing approximate nearest neighbors from 1 billion to 58, 000 clusters followed by 50, 000, let's say you have a 1024 dimension embedding, you use the same 1024 embedding for every single one of these phases. But as we talked earlier, if you only care about recall, which your clustering is basically doing, what your clustering is saying is, look, I just need to be in the right cluster, right portion of your space, and that's pretty much I care about. [01:44:29] Aditya Kusupali: So that's just recall. And If I'm able to do this clustering with 64 dimensions instead of 1024, I can save a lot of compute when I'm searching the space. And this is the idea. So at every single level of this tree, I'm going to change the dimensionality I'm going to use. Let's say 64, 128. And then finally, when I come to leaf node, when my query goes to the leaf, I'm going to precisely re rank all these 100 data points or so. [01:44:53] Aditya Kusupali: 2024. So there is going to be a precise re ranking at the end, but all the intermediate steps, because they're already approximating, but only care about recall, can be approximated with a lower dimension embedding. You can traditionally do this even without Matrioshka embeddings, but you need again post hoc compression, which is not pretty great. [01:45:12] Aditya Kusupali: So Matrioshka representations just gives you this for free. So if you want 64 dimensions for the first phase of clustering, take the first 64. If you want 128 for the second phase of clustering, take the first 128. And that's the reason it becomes seamless and that's what ADAMS does. [01:45:27] Alex Volkov: Awesome. And I want to take this to the practical level a little bit. As far as I saw, Sentence Transformers from Hug Face supports supports this natively right now, right? You can import and you can encode embeddings in different models. What other tools since you guys started getting a lot of interest, after this, both because the LLM explosion, now everybody does RAG and everybody understands that RAG is one way to get these models to behave as they want. [01:45:51] Alex Volkov: What else? What other tools? You mentioned PHI. What other tools are now supporting something like this? Because on the face of it, it sounds very very helpful, very performant. In my head, this sounds like Not necessarily direct, but like similar to how quantization came and reduced them like precision of models. [01:46:08] Alex Volkov: And basically they respond with the same precision, but they're significantly smaller. So what other tools can folks find use in Kamiatroska from what you're, you guys have heard? [01:46:20] Aditya Kusupali: Yeah, two clarifications. Face does not use Matryoshka right now, but ADANCE was built off of Face so yeah, that's a caveat so they don't use Matryoshka at this point. Yeah, second thing you asked quantization, right? That's a very good point. Quantization is a complementary thing. [01:46:36] Aditya Kusupali: So think of quantization as flexibility in your bit precision, while Matryoshka is flexibility in your dimensionality. So both of them can work hand in hand even after this. So you can quantize any Matryoshka embedding, and it will still play well with quantization. So that's the beauty of this, right? [01:46:54] Aditya Kusupali: Until now, we were only reducing the precision of the numbers, and now you can also reduce the Vector itself. So that's very good coming to the repositories and other stuff, which are using, of course, sentence transformer, I think is going to be the easiest way in. I went through the implementation day before yesterday. [01:47:14] Aditya Kusupali: It's pretty clean. It just works out of the box. NOMIC released the V for 1. 5. If anyone wants to go and look at it inside again, it's 10 lines of code. Beautifully written. And I think it's much more understandable in case someone wants to get into the weeds. So that is one thing we have our own repository, which we released like a couple of years ago. [01:47:33] Aditya Kusupali: But the nice thing about Matryoshka is if you want to train something, it's literally a for loop. It's four lines of code. So the code is already in the paper. If someone wants to go and implement it, it's You just look at the paper, there will be a code in I think, page 12 or something, five lines, you just go and implement it. [01:47:48] Aditya Kusupali: Apart from that I think Transformer. js was supporting a bunch of these re ranking visualizations in HuggingFace. But yeah, like for now, I think these are the things we know which are supporting. ADAN, I don't think anyone is supporting at this moment. It's just our code base. which is out there. [01:48:05] Aditya Kusupali: It's also not highly optimized for low level things, so I wouldn't recommend you directly use it for your use cases, but it's a great thing for you to prototype and see how well you could benefit from this flexibility in retrieval space. [01:48:18] Alex Volkov: So I just wanna make sure [01:48:20] that we shout out properly. No ai, the folks that have the Atlas platform to visualize and they down, down sample, I think you said like they lower the dimensionality to, into 2D or 2D space to actually show dimensions. They release. No embed. 1. 5 recently, like a fully open source embedding models end to end and they're great and now they're also supporting Matryoshka, which is great. [01:48:41] Alex Volkov: I also heard you say that quantization directly applies here as well. So you can like I don't know the verbiage of this, like you can Matryoshka something and quantize a model in this Wool Resort is significantly smaller. And like smaller weights so that's great. [01:48:54] Alex Volkov: You also mentioned Transformers. js, which is a Hug Face library, the author of which Joshua Zanova is here in the audience with us, friends of the pod, that supports this as well. Folks, we're slowly running out of time a little bit. I wanted to thank you for coming up. It often happens when folks who build something come up and talk to us. [01:49:13] Alex Volkov: It doesn't often happen in something that released a few years ago. that now resurfaces in popularity. And then we're able to highlight some folks works. So Aditya and Pratik, I really want to thank you. Anything else that you want to mention before we before I recap the whole space, feel free to. [01:49:28] Alex Volkov: Definitely not a full deep dive, but I really wanted to highlight the fact that your work is now represented also in like one of the big libraries in the world in terms of AI. And many folks can now understand what is this parameter that they do when they adjust dimensionality and open the eyes embedding models. [01:49:44] Aditya Kusupali: I think nOMIC the reason why I say this is a straightforward implementation is NOMIC released their v1 and then Matrioshka became a thing, so they literally trained this entire thing in three days and with all of their data, so it's extremely simple and they actually had to not change a single hyperparameter, so it's pretty good. [01:50:02] Aditya Kusupali: I would like to see if Pratik wants to add anything, but Otherwise, thank you for having me here. [01:50:07] Alex Volkov: Thank you, Adithya. [01:50:07] Prateek Jain: No, it's pretty accurate. Thanks for having [01:50:10] Aditya Kusupali: us here. Yeah, and for anybody else in the audience, sorry I've posted the links as to what you can do with this. So it's Zenova's demo when you can use it in Transformers JS. [01:50:21] Aditya Kusupali: And also we look forward to actually implementing the paper too, because again, this is not a very well known or well discussed subject in general. [01:50:31] Alex Volkov: So I'm very happy to have been able to host you guys and You have a paper out, I think it was in NeurIPS, and seeing more from this space of embeddings, because there's more to come here, and many people are now using this in big production probably, it was used in Rexis before, but now in big LLM related production stuff, and the more folks understand retrieval and fine tuning retrieval, and also ways to cut costs, like Matryoshka, for example it would be great, so shout out to you guys, definitely, thanks for working on this and coming and showing, giving light, I'm very happy that you did get The the mention in the open, OpenAI, and I'm also, I'm happy that I noticed because of this, and was able to talk to you guys and figure out what Mutrochka embeddings are. [01:51:11] Alex Volkov: And if folks want more deeper, deep dives, this is what was like, very surface level. You guys did a paper. PaperClub with Latentspace yesterday, and before that, both with Vietpodcast talked about Matryoshka Embeddings. Connor was here before, you guys just missed him. And also, Nisten put this link up. [01:51:28] Alex Volkov: HugInFace has a very nice deep dive from Omar and Zenova about Matryoshka Embeddings and what they mean and how to use them in sentence transformers. All right, folks, this has been our ThursdAI for today. I will now take a deep breath and recap everything we've talked about if you've been here for the past two hours and some, you've probably heard all of this, but if not feel free to stick around and it's probably gonna take me like eight minutes or so and then we're gonna let you go. [01:51:53] Alex Volkov: With that, this is our Thursday AI for February 29th. Leap year, February 29th, like once in four years, I find it pretty funny. And I think it was a great space, [01:52:01] Alex Volkov: we didn't have any, Nisten, no breaking news today, right? I wasn't monitoring well, but I didn't see GPT 5 didn't release while I was talking, right? [01:52:11] Nisten Tahiraj: Nope not yet. [01:52:12] Alex Volkov: Not yet. [01:52:13] Alex Volkov: We did get one piece of breaking news that we didn't notice as we were recording the live stream, and that was from our friends in Modular. If you remember, we've talked about Modular and their new programming language Mojo, which is a superset of Python, and the creator Chris Lattner, who was previously the LLVM and MLIR compiler author and also the creator of Swift. [01:52:42] Alex Volkov: Uh, in Apple, and, uh, we've talked about Mojo being the right language for AI, and they just released their inference engine called Max to the world in beta, and this inference engine supposedly has Mojo built in, and supposedly is way faster even for existing models, uh, to run inference. So that's very interesting, and we're going to talk about more as we, as we play around with this. [01:53:07] Alex Volkov: Alright, folks, and I think this was all we talked about on ThursdAI on February 29th. And I want to just thank everybody who joined. Nisten, thank you, as always, co host. Jan was here before, and we had Beau join for a while, even though we didn't say hi. [01:53:22] Alex Volkov: We have a bunch of other folks. So thank you for all the guests. Thank you, all of you, for listening and tuning in from week to week. It's really a pleasure. And now with this, I'm just going to end here. everybody. We'll see you next week. Cheers. This is a public episode. If you'd like to discuss this with other subscribers or get access to bonus episodes, visit sub.thursdai.news/subscribe | |||
08 Mar 2024 | 📅 ThursdAI - Mar 7 - Anthropic gives us Claude 3, Elon vs OpenAI, Inflection 2.5 with Pi, img-2-3D from Stability & More AI news | 01:45:27 | |
Hello hello everyone, happy spring! Can you believe it? It's already spring! We have tons of AI news for you to cover, starting with the most impactful one, did you already use Claude 3? Anthropic decided to celebrate Claude 1's birthday early (which btw is also ThursdAI's birthday and GPT4 release date, March 14th, 2023) and gave us 3 new Clauds! Opus, Sonnet and Haiku. TL;DR of all topics covered: * Big CO LLMs + APIs * 🔥 Anthropic releases Claude Opus, Sonnet, Haiku (Announcement, try it) * Inflection updates Pi 2.5 - claims GPT4/Gemini equivalent with 40% less compute (announcement) * Elon sues OpenAI (link) * OpenAI responds (link) * ex-Google employee was charged with trading AI secrets with China (article) * Open Source LLMs * 01AI open sources - Yi 9B (Announcement) * AnswerAI - Jeremy Howard, Johno & Tim Detmers - train 70B at home with FSDP/QLoRA (X, Blog) * GaLORE - Training 7B on a single consumer-grade GPU (24GB) (X) * Nous open sources Genstruct 7B - instruction-generation model (Hugging Face) * Yam's GEMMA-7B Hebrew (X) * This weeks Buzz * Weights & Biases is coming to SF in April! Our annual conference called Fully Connected is open for registration (Get your tickets and see us in SF) * Vision & Video * Vik releases Moondream 2 (Link) * Voice & Audio * Suno v3 alpha is blowing minds (Link) * AI Art & Diffusion & 3D * SD3 research paper is here (Link) * Tripo + Stability release TripoSR - FAST image-2-3D (link, Demo, FAST demo) * Story how I created competition of inference providers to get us sub 1.5s playground image gen (X) Big CO LLMs + APIs Anthropic releases Claude 3 Opus, Sonnet and Haiku This was by far the biggest news of this week, specifically because, the top keeps getting saturated with top of the line models! Claude Opus is actually preferable to many folks in blind studies over some GPT-4 features, and as we were recording the pod, LMSys released their rankings and Claude Opus beats Gemini, and is now 3rd in user preference on the LMSys rank. There release is vast, they have announced 3 new models but only gave us access to 2 of them teasing that Haiku is much faster / cheaper than other options in that weight class out there. In addition to being head to head with GPT-4, Claude 3 is now finally also multimodal on inputs, meaning it can take images, understand graphs and charts. They also promised significantly less refusals and improved accuracy by almost 2x. One incredible thing that Claude always had was 200K context window, and here they announced that they will be supporting up to 1M, but for now we still only get 200K. We were also promised support for function calling and structured output, but apparently that's "coming soon" but still great to see that they are aiming for it! We were all really impressed with Claude Opus, from folks on stage who mentioned that it's easier to talk to and feels less sterile than GPT-4, to coding abilities that are not "lazy" and don't tell you to continue writing the rest of the code yourself in comments, to even folks who are jailbreaking the guardrales and getting Claude to speak about the "I" and metacognition. Speaking of meta-cognition sparks, one of the prompt engineers on the team shared a funny story about doing a needle-in-haystack analysis, and that Claude Opus responded with I suspect this pizza topping "fact" may have been inserted as a joke or to test if I was paying attention This split the X AI folks in 2, many claiming, OMG it's self aware, and many others calling for folks to relax and that like other models, this is still just spitting out token by token. I additional like the openness with which Anthropic folks shared the (very simple but carefuly crafted) system prompt My personal take, I've always liked Claude, even v2 was great until they nixed the long context for the free tier. This is a very strong viable alternative for GPT4 if you don't need DALL-E or code interpreter features, or the GPTs store or the voice features on IOS. If you're using the API to build, you can self register at https://console.anthropic.com and you'll get an API key immediately, but going to production will still take time and talking to their sales folks. Open Source LLMs 01 AI open sources Yi 9B Announcement claims that "It stands out as the top-performing similar-sized language model friendly to developers, excelling in code and math." but it's a much bigger model, trained on 3T tokens. I find it confusing to create a category of models between 7B and almost 12B. This weeks Buzz (What I learned with WandB this week) We're coming to SF! Come join Weights & Biases in our annual conference in the heart of San Francisco, get to hear from industry leaders about how to build models in production, and meet most of the team! (I'll be there as well!) AI Art & Diffusion Last week, just last week, we covered the open sourcing of the awesome Playground 2.5 model, which looked really good in user testing. I really wanted to incorporate this to my little demo, but couldn't run it locally so asked a few friends, and I gotta say, I love how competitive but open the inference providers can get! Between Modal, Fal and Fireworks, I somehow started a performance competition that got these folks to serve Playground 2.5 model in sub 1.5 second per generation. Recorded the story to highlight the awesome folks who worked on this, they deserve the shoutout! You can try super fast Playground generation on FAL and Fireworks Stability releases Stable Diffusion 3 research paper + Model coming soon Stability released the research paper for SD3, their flagship latest iteration of an image model. While this field is getting a little saturated, we now have DALL-E, MidJourney, Adobe Firefly, Playground, SDXL, Stable Cascade and Ideogram, SD is definitely aiming for the title. They released a few metrics claim that on user preference, Visual Aesthetics, Typography and Prompt following, SD2 beats all of the above. They also mentioned the architecture, which is a MM-DiT - multi modal diffusion transformer architecture (DiTs were used for SORA from OpenAI as well) and that they used 50% synthetic captions with COGvlm, which is quite impressive. Emad has mentioned that access to SD3 will start rolling out soon! TripoSR (Demo) We previously covered LUMA models to generate text to 3d, and now we have image 2 3D that's open sourced by the folks at Tripo and Stability AI. TripSR is able to generate 3d shapes from images super super fast, and here's a very nice flow that @blizaine demonstrated of how to use these models to actually bring 3D objects into their environment using a few steps. And that's it for today folks, we of course chatted about a LOT more stuff, I really welcome you to listen to the episode and skip around in the chapters, and see you next week, as we celebrate ThursdAI's birthday (and GPT4 and Claude1) 🎉 P.S - as I always do, after writing and editing all by hand (promise) I decided to use Opus to be my editor and tell me how was my writing, what did I forget to mention (it has the context form the whole transcription!) and suggest fixes. For some reason I asked Opus for a message to you, the reader. Here it is, take it as you will 👏 Full Transcript for the deep divers: [00:00:00] Alex Volkov: Right, folks. So I think recording has started. And then let's do our usual. Welcome. Welcome, everyone. Those who know the sound from week to week. This is Alex Volkov. You're listening to ThursdAI, March 7th. I'm an AI evangelist with Weights Biases, who you can see here on stage as well. So, you know, you see the little square thing, give it a follow. Follow us on socials as well. And, uh, today is obviously Thursday. [00:00:45] Alex Volkov: Uh, Thursday was a lot of stuff to talk about. Um, so, let's talk about it. Uh, I think, I think, um, our week is strange, right? Our week starts at the Friday. Almost, not even Friday. The updates that I need to deliver to you start at the end of the previous ThursdAI. So as, as something happens, uh, and I, I have a knowledge cutoff, actually, at some point we considered calling this podcast knowledge cutoff. [00:01:14] Alex Volkov: Um, I have a knowledge cutoff after Thursday afternoon, let's say when I start and send the newsletter, but then AI stuff keeps happening. And, uh, Then we need to start taking notes and taking stock of everything that happened and I think on Friday We had the the lawsuit from Elon and there's a whole bunch of stuff to talk about and then obviously on Monday We had some big news. [00:01:37] Alex Volkov: So As always I'm gonna just run through all the updates. There's not a lot today There's not a ton of updates this week, but definitely there's a few interesting things. Let me un save as well And then I'll just say hi to a few, a few of the folks that I got on stage here to chat. Um, we got Vic, and Vic is going to give us an update about, about something interesting. Uh, Vic, feel free to just unmute and introduce yourself briefly. And then we're going to go through the updates. [00:02:07] Vik: Hey, my name is Vivek, uh, I've been training ML models for the last two years or so. Um, recently released a new model called OneDream2. It's a very small vision language model that excels at a lot of real world use cases that you could use to build computer vision applications today, so I'm very excited to chat about that. [00:02:30] Alex Volkov: Awesome. And, uh, we have Akshay as well. Akshay, it's been a while since you joined us. What's up, man? How are you? [00:02:36] Vik: Greetings of the day everyone, and it's lovely to join again. Uh, I have been listening, I have been here in the audience. Uh, for each and every ThursdAI, and, uh, I've been building some exciting stuff, so I've not been joining much, but, uh, things are going great. [00:02:54] Alex Volkov: Awesome. And, uh, for the first time, I think, or second time we're talking with Siv. Hey, Siv. [00:03:01] Far El: Hey, how's it going, everyone? Uh, just a little background on me. Um, I come from startups and from Amazon Web Services. Um, I've been in the AI space for the last six years. And I'd love to be able to chat today about social algorithms and, uh, researchers [00:03:21] Nisten: having [00:03:22] Far El: trouble with, uh, socials, particularly Twitter. [00:03:26] Far El: Anywhere else where you're trying to distribute your [00:03:28] Nisten: models? [00:03:30] Alex Volkov: Yeah, so we'll see if we get to this. The setup for ThursdAI is usually just, uh, updates and conversation about updates, but if we get to this, uh, definitely we'll, we'll, we'll dive in there. Um, right, so folks, with this, I'm gonna say, um, uh, that we're gonna get started with just an update, and then I think Nisten will join us in a second as well. [00:03:50] Alex Volkov: Oh, I see somebody else I wanna, I wanna add. [00:03:55] Alex Volkov: So, here's everything for March 7th that we're going to cover today. Um, so in the area of open source, we didn't actually have a ton of stuff happen, um, up until, I think, yesterday and today. So, the most interesting thing we're going to talk about is, um, the company O1AI, um, which is a, The folks who released YI 34b, and we've talked about YI and the new Hermes kind of updates for YI as well. [00:04:23] Alex Volkov: They released a new 9 billion, 9 billion parameter model, which is very competitive with Mistral and the like. Um, and then also the new company, newish company called Answer. ai from Jeremy. Jeremy Howard, if you know him, and Joanna Whittaker, and they collaborated with Tim Dittmers from Qlora, and they released something that lets you train a 70 billion parameter at home, a 70 billion parameter model at home. [00:04:51] Alex Volkov: We're going to chat about this a little bit. Um, even though today I saw another thing that is kind of around this area, so we're going to have to go and find this and discuss how these huge models are now being able to get trained at home as well. Uh, very brief open source stuff, then we're going to talk about big companies and obviously, um, actually going to put cloud last because we're going to talk about cloud probably a lot. [00:05:16] Alex Volkov: But, uh, in the big companies area, we will not be able to escape the drama that Elon Musk sues OpenAI. And then the OpenAI response, we're going to chat about this as well. Excuse me. Oh yeah, this is going to keep happening, just one sec. Um, maybe we'll briefly mention that Logan has left OpenAI, and for a brief period of time, he and Ilya had the same, um, bio on Twitter, not anymore, but very interesting as Logan starts to post some stuff as well. [00:05:46] Alex Volkov: Um, I really want to chat about the Google employee who was charged with AI secret trading, uh, and received like a CTO position in China. That's a very interesting update as well. And, uh Inflection from, uh, there we go, we have Nisten as well, uh, Inflection just released an update today, which is kind of like breaking news, uh, a 2. [00:06:09] Alex Volkov: 5 update, and they, they say they come to GPT 4 and Gemini equivalent, uh, performance level, which remains to be seen, and I've tested this a little bit, and I definitely want to chat about this as well. Uh, in the vision and video, and We have only the one thing, but we have the author of said thing here. Uh, so I haven't seen any, anything else besides Moondream and we have Vic here. [00:06:33] Alex Volkov: We're going to talk about Moondream too, and how you can use this and what we can, we can use it for. Um, Voice and audio. There's something that probably didn't happen for the past week. I think it happened a little bit before and I don't have access yet, but Suno if you guys know Suno released the alpha and there's a bunch of videos floating around of their songs with like the V3 alpha of theirs and it's quite something if I if I'm gonna be able to find those tweets and pin them for you That's gonna be a mutual listening Maybe I can actually find the tweet to to actually play this for you. [00:07:07] Alex Volkov: We'll see if the multimedia department will work. Um, and I think in AI art and diffusion stuff, there's a bunch to talk about. Um, there is, uh, Stable Diffusion 3 research paper was released, and we've talked about Stable Diffusion 3 a little bit. After the announcement, and we haven't covered the research paper, we can chat about the research paper. [00:07:29] Alex Volkov: But also, potentially today, Imad is going to open some invites, as he mentioned on X as well. So, I'm ready with the breaking news button there. Stability, also in the news, they released a collaboration with Tripo, which created a very fast image to 3D model called Tripo SR. And that's been very cool, and there's a few very Viral examples of, of said thing, uh, floating around, so definitely worth talking about this as well. [00:07:57] Alex Volkov: And I think, uh, Nisten is just joined us, hey Nisten, and you just shared that, um, That we can train a 70 billion parameter, Oh, 7 billion parameter at home with 24 gig memory, right? A galore. Nisten? [00:08:17] Nisten: so, so it's a combination of a lot of [00:08:20] techniques that people have been using. And, uh, I'll try to pin it up in a second. But the. The research is that now you can train one from scratch. Not Finetune. Start one from scratch. Start your own. So this is why it's pretty, um, it's relatively groundbreaking. [00:08:40] Nisten: And they released a repository for that as well. So it's not simply just a paper. They have a code base. It's pretty legit. [00:08:50] Alex Volkov: So I guess let's, let's get into the open source stuff, um, and then we'll get to the open source, and then we're going to discuss everything else, because I think the main, the bread and butter of this discussion is going to be, is going to be, um, Anthropic. Anthropic's, uh, coming back to the limelight, but let's, let's start with, let's start with open source. [00:09:09] Alex Volkov: Where's my open source button? [00:09:27] Alex Volkov: Alright, so I guess, uh, Nisten, welcome, uh, and I guess let's start with, with Galore, uh, as much as we can. We can get from the, from the release, a fairly, fairly new release as well. And I think it's connecting to the other, uh, to the other thing from Answer. ai, but let's start with Galore. Um, so basically, these folks released something called Galore, which is, um, kind of playing on the same, on the same LoRa, QLoRa stuff. [00:09:52] Alex Volkov: Uh, what are some of the techniques they're adding there? I'm, I'm trying to, to take a look as I'm learning. Uh, Nisten, do you have any, any Any info to share with us about this? [00:10:05] Nisten: Yeah, yeah, same. more for an actual full paper reading because I have not read it entirely. Mainly looking at it again, it looks like it's, uh, it's another stack of tricks like most good projects are, uh, but it is the, it enables a very, very large capability. And that is that now you can make your own full LLM from, from nothing. [00:10:29] Alex Volkov: So not a fine tune. [00:10:31] Nisten: Uh, yeah. Not a fine tuned, not initiated weights. You just, you just start from, uh, from nothing. So, it's I see that it uses, uh, like it offloads a lot of the weight activations and offloads some of them on, uh, on CPU memory. And I know there are options in Axolotl, which is the Docker container that people use to train, that you can also offload on very fast NVMe drives. [00:10:55] Nisten: So if you have like very fast PCI Express NVMe storage, you can kind of use that as another RAM for, for the training. So this combines all of those. And then some on top and the end result is, is very impressive because you can train a very capable model. And, uh, yeah, again, pending further, uh, research and stuff. [00:11:21] Nisten: But I think this is one of those repositories that, uh, a lot of people will use or it's likely to. [00:11:30] Alex Volkov: Yeah, and I think this adds to the, so this, this kind of in the same vein of the next thing we're going to chat about and, um, um, I actually can't find any mention of this on X, believe it or not, so not everything is fully on X. I just got a link, uh, to this from, from, uh, Omar, uh, from Hug and Face. And AnswerAI is a new research lab, um, that Jeremy Howard, uh, if you guys are not familiar with Jeremy Howard, hopefully everybody is, but if you're not, um, I guess look them up. [00:12:04] Alex Volkov: Um, Jeremy, uh, joined Answer. AI, like, um, I think around NeurIPS he was talking about. They got funded, I think, 10 million dollars. And, um, they released their first project, a fully open source system, uh, that can efficiently train a 70 billion large language model on regular desktop computers with two or more gaming GPUs. [00:12:27] Alex Volkov: They're talking about RTX 3090 or 4090. Um, Which, you know, compared to, um, Niton what you just shared, I think that sounds very impressive. Um, they combine FSDP, which is, I'm not familiar with FSDP, with SFDP and uh, q and, uh, they brought kind of the, the Cuda Avengers to, to the flow. So Jeremy Howard obviously. [00:12:52] Alex Volkov: Um. I think FastAI, right? And then Kaggle, I think, competition is definitely behind Jeremy. And then they brought Team Ditmers from Qlora, and we've covered Qlora multiple times, um, very efficient methods. And then they also brought Hugging Faces, Tyrus Von Koller, and, um, they brought the CUDA Avengers in there to, to Basically combine a bunch of techniques to let you train 70 billion parameters. [00:13:20] Alex Volkov: I see we have Yam joining us. Hey Yam, did you see the Answer. ai stuff that I'm covering or is this new to you? [00:13:26] Yam Peleg: No, no, all new to me. [00:13:28] Alex Volkov: Oh wow, okay, so I need, I need, uh, I would love your reaction in real time. Let me DM you this real quick because, um, The number of, actually, let me, let me paste this in the link below so we can actually paste this up. [00:13:43] Alex Volkov: Um. Yeah, there we go. Okay. So it's now pinned to the top of the space for folks to, to find out. I wasn't able to see any, uh, update on X from any of them, which is very interesting. Um, and the, the very interesting idea is that, you know, all of these systems and all of these models, 70 billion models, they cost an insane amount of money. [00:14:07] Alex Volkov: And now these folks are considering that under 10, 000, you'd be able to train something like 7TB at home. Which I'm not training models, but I know that some folks here are. And, um, I assume that this is a very big unlocking capability. Um, which, which is what Answer. AI is trying to achieve. [00:14:32] Alex Volkov: Let's see what else is very interesting here. Um, just something about Answer. AI generally. Uh, they claim that they're like an unusual type of organization. I actually tried to ask Jeremy a couple times what did this mean. Um, and, uh. They, they claim to be a for profit, like, lab, R& D lab, and, um, more in spirit to 19th century labs than today's AI research groups, and, um, I think Eric Ries and Jeremy Howard launched this in Europe, um, and, I think, I'm actually not sure what's the, the, how much did I say? [00:15:14] Alex Volkov: Um. What are they up against? But the first release of theirs is the open source OS, fully open source. Uh, that includes one of the, like several of the top people in the industry, uh, to create something that wasn't possible before. Um, and I think it's remains to be seen. They didn't release any metrics, but they said, Hey, we're about to release some metrics, but, um, this keeps improving from week to week. [00:15:39] Alex Volkov: So we actually didn't release any metrics. Go ahead Nisten. [00:15:43] Nisten: Sorry, is this from Answer. ai? They said they were going to release one, or? They [00:15:49] Alex Volkov: think, already. They didn't release metrics, uh, for the training. Uh, but I think the, the whole repo is open source. Yeah. [00:15:58] Nisten: released an open source OS, or? [00:15:59] Alex Volkov: Yeah, yeah, open source, FSDBQLora. Um, and I think [00:16:03] Nisten: Oh, okay, so it's not a real operating system, it's another, [00:16:07] Alex Volkov: It's, well, they call it an operating system, but yeah, [00:16:10] Nisten: Oh, okay, [00:16:11] Alex Volkov: it's not like Linux competitive. [00:16:12] Nisten: okay, I thought it was like an actual one. Okay, actually, go ahead, because there are some other huge hardware news that I wanted to quickly cover. [00:16:23] Alex Volkov: Go ahead, [00:16:23] Yam Peleg: Yeah, [00:16:23] Vik: I just wanted to add about this answers. ai thing that they have released this system that you guys were talking about, which basically claims to be able to train 70 billion parameter model on only 224 [00:16:40] GB GPUs. [00:16:40] Vik: So basically, you know, two 4090s and you can train a 70 billion parameter model, which is mind boggling if you think about it. But, uh, I tried to find like how to get access to this. So I was still not sure if this is fully supported in every, uh, rig and system. So that is something I [00:16:58] Nisten: wanted to mention. [00:17:00] Alex Volkov: Yeah. [00:17:00] Nisten: By the way that that has been, oh, sorry. [00:17:02] Nisten: That that has been do, uh, doable for a while because Kilo actually trains it all in four bit. And, uh, there are only like a few tricks, which you can also apply if you go to apps lot, uh, the directory. You, you can also do that on your own if you do a four bit kilo training and you just say, offload all the gradients and all this stuff, you can also do that with a, the 48 gig, uh, stuff. [00:17:26] Nisten: But, uh, again, I'll look into the actual directory instead. [00:17:32] Alex Volkov: Right, so, um, Nisten, you mentioned some hardware news you want to bring? Go ahead. [00:17:39] Nisten: Yep. Okay, so we have two hardware news, but they are actually kind of related. Uh, first of all, uh, TenseTorrent, the company by legendary computer scientist, Jim Keller, who worked on the iPhone chip, AMD, who brought AMD back to life. Uh, legendary computer scientist, and has been working on TenseTorrent, which is another, uh, accelerator for, which also does, does training. [00:18:07] Nisten: So, uh, so they released these cards, and I'm not sure what the capabilities are, uh, but I saw that George Hotz, uh, from TinyCorp, uh, posted them, and, uh, they are actually, so I just wanted to give them a big shout out to actually making them commercially viable, and it's just something you can buy, you don't have to, uh, You know, set up a UN meeting for it, right? [00:18:31] Nisten: And get the votes and stuff. You can just go and buy it. So, that's pretty awesome of them, and I wish more companies did that. The second news is also kind of huge, because one of the engineers that left TestTorrent last year now started a startup here in Toronto. And this has been an idea that's been around for some time and discussed privately and stuff. [00:18:59] Nisten: Uh, they're making AI chips. Again, they do not. These ones do not do training, but they're going to make them hard coded, which will be the judge of how much that makes sense given the how rapidly models improve. But there is a business case there because the hard coded chips, they can perform literally a thousand to 10, 000 times faster. [00:19:25] Nisten: So [00:19:26] Alex Volkov: you say hard coded, is that one of those, like, transformer specific chips you mean? [00:19:33] Nisten: no, the entire weights are etched into the chip and you cannot change them. So the benefit of this is that you can get up to a thousand to ten thousand times faster inference. So we might end up with a case where, according to calculations from What Sam Altman said on how much chat GPT serves in a single day, which is a hundred billion tokens, and that works out to about 1. [00:20:02] Nisten: 4 million tokens per second. We might very soon, like in a year or two or sooner, be in a spot where we have this company's using 60 nanometer chips. We might have a single chip pull the entire token per second performance of all of global chat GPT use. I don't know if that includes enterprise use, but that's how fast things are accelerating. [00:20:29] Nisten: So that's the, that's the benefit of, uh, yeah, that's the benefit of going with a hard coded chip. So yeah, call, uh, inference costs are, um, are dropping in that [00:20:43] Alex Volkov: You also mentioned George Hotz and, uh, he also went on a, on a, on a rant this week again. And again, I think, do you guys see this? Um, the CEO of AMD that doesn't use Twitter that much. But she replied to one of him, uh, one of his demands, I think, live demands, and said, Hey, uh, we have a team dedicated working on this. [00:21:05] Alex Volkov: And then we're gonna actually make some changes. in order to get this through. So, I love it how, uh, George Hotz, um, folks probably familiar with George Hotz in the audience, um, Should we do a brief, a brief recap of George Hatz? The guy who hacked the first iPhone, the first PlayStation, then, uh, built a startup called Com. [00:21:25] Alex Volkov: ai to compete with Autonomous Driving, and now is building tiny, uh, we mentioned tiny boxes ready to ship Nisten last time, and I think that paused because they said, hey, Well, we don't have enough of the open sourcing of the internal stack of AMD Which led the CEO of AMD, Linda, or Lisa? I'm very bad with names. [00:21:46] Alex Volkov: I think Linda, to reply and say hey, we have dedicated teams working on this Actually do want to go find this tweet Go ahead Nisten [00:21:57] Nisten: Yeah, so there has been a big misconception in the software industry that, um, a lot of the, the code monkey work is something that, you know, you just hire someone to, like, clean your toilets and, and do it. But, in fact, the reason that NVIDIA has a 2 trillion valuation, and I'll beat Saudi Aramco, is because that Their toes are a lot cleaner in terms of the software. [00:22:27] Nisten: So, the CUDA software is a lot more workable, and you can do stuff with it, and it doesn't have the bugs. So, in essence, what George Haas is doing by pushing to open source some key parts, which some people might freak out that China might steal them, but they've already stolen everything. So, it really doesn't, doesn't matter that they're very small hardware parts, but they make a huge difference in developers being able to. [00:22:56] Nisten: to use that software, and those parts are buggy. So, in essence, like, George Haas, with this stupid CodeMonkey fix, might double or triple AMD's stock [00:23:07] Alex Volkov: Yeah, [00:23:08] Nisten: Just because he's getting in there, and he's cleaning that crap code out. [00:23:14] Alex Volkov: and he's popular enough to pull attention from the CEO of this company to actually come and react and, you know. One of the reasons I love X is that I think, um, uh, she retweeted their official tweet. I think there's more folks commenting on and reacting to her, um, comment, and that's on top of the space now, uh, than the actual kinda tweet itself. [00:23:37] Alex Volkov: Which is, I think, a good type of ratio, or ratio, yeah. I think, uh, more hardware news, I think we're satisfied with Oh, yeah, yeah. The, the, the only other hardware news related to this, 'cause ni I think you mentioned Saudi Aramco. Uh, we chatted with the GR folks with a Q not with a K grok. The, the LL uh, LPU chip. [00:23:58] Alex Volkov: And they're like super fast, uh, inference speed, and I think this week. They showed that they have a collaboration with, I think said, Saudi Aramco, um, about bringing AI. Um, and I saw a few, a few folks post about this and, um, if that's of interest to you, we had a full conversation with the Grok team. They also, they also, um, Release, kind of, uh, they had a waitlist and many, many people, I think the waitlist jumped after we chatted with them at the peak of their very viral week, which started with match rumor going, going off. [00:24:32] Alex Volkov: Uh, and then I think they said something about, they had like 50 or a hundred waitlist signups before this. And then the week after they had like 3, 600 a day or something like this. So they revamped the whole system. And now, you can actually sign up with a self served portal to Grok, and uh, let me see if I can find this tweet for you. [00:24:55] Alex Volkov: So you can actually now go and sign up, um, to Grok yourself, [00:25:00] they have a nice console, very reminiscent for, um, for every other, like, console out there. You can create an API key, very simple, so no longer like a manually, manual approval of, um, Grok. I can't find this tweet though, so give me, give me just a second. [00:25:22] Alex Volkov: So, yeah, they, they're, uh, collaborating with, with Saudi Encore. Go ahead Nisten, real quick. [00:25:28] Nisten: Uh, yeah, just really quickly, the part that I missed was that, uh, the fix that George Haas is doing for AMD, that's to enable distributed training. Because they cannot distribute training across GPUs because it crashes. So it's pretty important. Uh, yeah, and those are my comments on that. [00:25:48] Alex Volkov: Awesome. Okay, so I, I found the tweet. Uh, so if, if you follow this tweet, the, the kind of the, the quoted tweet there is, uh, getting you to the Grok console. You get like two weeks for free and you get the API access to this like incredibly fast inference, inference machine from Grok. [00:26:05] Nisten: I think Far El and Yam wanted to say something on it. [00:26:10] Alex Volkov: Yeah, go ahead. [00:26:11] Yam Peleg: Yeah, I got a lot of technical issues. So if you can go before me, I'll try to [00:26:17] Vik: fix it. [00:26:19] Alex Volkov: You're coming through finally, loud and clear. Far El, if you wanted to comment, go ahead, man. [00:26:30] Alex Volkov: Alright, um, looks like Far El is also, um, not available. Okay, I think we're moving [00:26:38] Vik: touch on this for a sec. Um, so Grok has a white paper out about how they've designed their chips and it's super interesting. I'd strongly recommend everyone go read it. Uh, they've basically from the ground up rethought how, uh, inference oriented compute should work. It's a fascinating read and kind of surprising that they're sharing all of those details. [00:27:00] Vik: One would think they'd keep it proprietary. [00:27:05] Alex Volkov: yeah, we had a full conversation with them. It is fascinating. Again, you know, for, for The level of discussion that we have here, um, we, you know, honestly, we couldn't dive like super, super deep, but I've played with it, and the demos I was able to do, uh, Vic, I don't know if you have the chance to see, uh, they're only possible with almost instant, uh, speed. [00:27:28] Alex Volkov: You know, guys, what, like, even though I love the Grock team, and we're collaborating with them, we're gonna do some stuff with them as well, um, it turns out that for some Use cases, inference speed, like a lot of inference speed on big documents, and I think that's what Grok is like definitely incredible with. [00:27:49] Alex Volkov: You take Mixtral and you dump a bunch of tokens in, and then you get like a super fast reply. So I was actually able to get a transcript in there for all of ThursdAI, and to get chapters within less than like 3 5 seconds, which is ridiculous. For the demo that I built, I actually didn't need inference speed. [00:28:09] Alex Volkov: I did need infraspeed, but as much as I needed a faster response on smaller kind of prompts multiple times. And I noticed that even though their infraspeed is incredible, their latency is not great, probably because they're still fairly young in this. And I went and looked, and Together also offers Mixtral over API. [00:28:31] Alex Volkov: Not Together, sorry. Together also does this, but specifically Perplexity. If you use Perplexity for search, you may not know that they also have an API that you can use, and they serve Mixtral and Mistral, and I think some other open source models and some of theirs. Um, and they keep improving their scores there, and specifically they're now up to 200 tokens per second for Mixtral and Mixtral, which is impressive. [00:28:56] Alex Volkov: And, you know, um, they don't have custom hardware, and they're getting 200 tokens per second, which is ridiculous. But what I notice is Perplexity is web engineers because they're now rumored to be a unicorn. I don't know if that's a rumor, so that's not confirmed. But their web engineers are really top notch. [00:29:16] Alex Volkov: And so it turns out that if I use Perplexity is API for Mixtral. I get less tokens per second. So I get less than half, right? So Grok is at around 500, um, Perplexity is around 200. But I actually get better performance because I need kind of low latency on the request itself and Perplexity is better at this. [00:29:36] Alex Volkov: Um, obviously something Grok can and will fix. And also the stuff that the Grok team told us were like, it's only, they're only scratching the itch. And Nisten, you mentioned something with them in the conversation that I wanted to repeat is that They're also working on figuring out the input latency of how fast the model not just spits out tokens, but processes the whole prompt input, which is a big deal, especially for long context prompts. [00:30:00] Alex Volkov: And they said that they're looking at this and they're gonna release something soon. [00:30:07] Nisten: Yeah, that's something that the NVIDIA cards excel at, and something that's holding back CPU based inference, because the prompt evaluation is, is, is slow. So, yes, it's not an easy problem to solve, but their chip is already so fast that the 3 to 1 ratio does not hurt them as much. Whereas With NVIDIA, the chips are slower and stuff, but they have like a 10 to 1 ratio, so if you're running at 100 TPS, your prompt eval is going to be like over, over a thousand. [00:30:42] Nisten: So it's going to read. If you dump in like 10, 000 tokens, it's going to read them in 10 seconds or less. Usually it's a few thousand with NVIDIA, but I'm not sure actually, because when you dump in a huge amount of text in Grok, it does not take multiple seconds to evaluate it. It's like instance, [00:31:04] Alex Volkov: It's quite, it's quite fast, yeah. [00:31:06] Nisten: yeah, so I'm not too sure that that needs some proper benchmarking to say for sure. [00:31:11] Alex Volkov: Yep. So, uh, speaking of Grok, let's, let's talk about the other Grok, but before that, you guys want to acknowledge, like, what's, what's going on with the rumors? Far El, you, you just texted something. I'm seeing Foster post something. Uh, what's, what's going on under, under the current of, of the Twittersphere? [00:31:27] Alex Volkov: Um, [00:31:28] Far El: Just, just speculation at this point, but, uh, you know, you know, those, uh, those people that, uh, that, uh, leak, you know, uh, stuff about OpenAI and all these AI companies, and most of the time, some of them are, are right. Uh, of course we don't see what they don't delete, [00:31:49] Alex Volkov: yeah. [00:31:50] Far El: uh, uh, yeah, like some of them are saying right now that, uh, there's like a rumor that GPT 5 is dropping. [00:31:57] Far El: That GPT [00:31:58] Alex Volkov: Say, say this again slower, because [00:32:01] Far El: 5 is dropping, that [00:32:02] Alex Volkov: there is a rumor that GPT 5 is dropping today. Wow. All right. Um, yeah. That's, that's quite, and I've seen this from like several folks, but [00:32:11] Far El: Could be complete b******t, right? [00:32:12] Yam Peleg: But yeah. [00:32:14] Alex Volkov: well, I'm ready with my button. I'm just saying like, let's acknowledge that there's an undercurrent of discussions right now with several folks who are doing the leaking. [00:32:22] Alex Volkov: Um, and then if this drops, obviously, obviously we're going to do an emergency, uh, and convert the whole space. I will say this, GPT 4 was released. Almost a year ago, like less than a week to the year ago, March 14th. Um, Cloud, I actually don't remember if Cloud 1 or Cloud 2. I think it was Cloud 1 that released the same day that people didn't even notice because GVT 4 took, took the whole thing. [00:32:52] Alex Volkov: Um, and now like Cloud releases their, um, Which we're gonna talk about, so I won't be surprised, but let's talk about some other stuff that OpenAI is in the news for. And then, and then if, if anything happens, I think we all have the same, uh, the same profiles on x uh, on notification. So we'll get the news as it comes up. [00:33:13] Alex Volkov: And we love breaking news here in, in, in, in Thursday. Okay, [00:33:17] Nisten: Yeah, for sure. [00:33:18] Alex Volkov: Um, let's [00:33:20] move on. Let's move on from open source. So, so I think we've covered. A few open source, I will just mention briefly that we didn't cover this, um, the, the folks, uh, from Yi, uh, 01AI, 01AI is a Chinese company, uh, they released the small version of Yi, and we've talked about Yi 34B multiple times before, there's a, a great fine tune from Nous, uh, they released a 9, 9 billion parameter version of Yi, which, uh, they trained for a long time, looks like, and, um, They showed some benchmarks, and it's very, very interesting how confusing everything is right now, because even, you know, even Gemma is not really 7 billion parameters. [00:33:58] Alex Volkov: Yeah, we talked about this, right? But then they now compare, they say in the same category broadly, and they now compare like Yi 9 billion parameters to Mistral 7 billion to Solr 10. 7 billion. So I'm not sure like what this category is considered, but maybe folks here on stage can help me like figure out what this category is considered. [00:34:19] Alex Volkov: But Yi is fairly performative on top of Mistral 7b, and I think it's still one of those models that you can run. I think, if anything, comparing this to Solr, 10 billion parameters, we've talked about Solr multiple times from the Korean company, I think. Yi is very performative, and the 34 billion parameter model of it was very good, and many folks really, really did some fine tunes of it. [00:34:45] Alex Volkov: So, asking the fine tuner folks here if you have a chance to look at it, and if not, is this something interesting? It looks like, unfortunately, YAML is having a lot of like X problems, uh, but once you come up, we're going to talk about the Hebrew GPT as well. Um, [00:35:02] Far El: What I do find interesting is, uh, how, yeah, like the, the, the broad evaluation spectrum that a lot of these models are, are comparing themselves to now, uh, and, and we're going to see more of these, uh, going forward, like, uh, I've seen early, uh, private researchers, Stuff, but like I feel like the category is no longer all just compare 7b to 7b It's it's just expanded to like sub 10b, right? [00:35:27] Far El: Like that's pretty much what it is like those those numbers even from players like Google are very You know, um, like it, it just doesn't feel as rigid as it used to be, but also like we should keep in mind that not all parameters are the same, right? So, like, uh, like we've seen with certain MOE architectures. [00:35:51] Alex Volkov: yeah, that's true. And, um, and I will say it's, uh, it looks like there's a art to train these models and some, some amount of art to also, uh, cherry pick which metrics you're, you're testing and against which models and which category you're placing your model in as well. Um, but just. And, and again, this was released like so recently that I don't think, I think yesterday, so definitely folks didn't have a chance to try this, but Yi, the, the other models of theirs were trained and performing very well, so, um, we're gonna be very excited to see if the Finetuning folks are jumping on this, uh, 9 billion parameter, and, and it performs better than, I think, Gemma is, ahem, The leading one, even though Mistral is still the leading one in our eyes. [00:36:36] Alex Volkov: Okay, I think this is it in the, um, Oh, let's see, a few more details here for Yi, and before I finish, Uh, it's trained on 3 trillion tokens, so a lot, uh, It's decent at coding and math, and then it has open access weights, and then bilingual. That's basically what we were able to get, uh, and thanks to the folks at Hug Face, VB. [00:36:59] Alex Volkov: I should probably add this as well. I think we're moving on to the main topic, which is the big companies, APIs and LLMs. I think it's, uh, you know what, you know, before this, I would go to vision category because we have Vic here. And, uh, I really want to chat about Moondream too. So, um, we've talked about Moondream 1, but folks who weren't with us, Vic, do you mind, uh, unmuting and then doing a little bit of a, of a intro for you as well? [00:37:26] Alex Volkov: And then we'll talk about what's changed in Moondream. [00:37:30] Vik: Yep, sounds good. Um, so, uh, Moondream is a small vision language model. Basically a vision language model is, uh, basically it's a language model where you can, Show it an image, ask it questions. You can ask it to describe the image. And the reason this is useful is not because it unlocks any new capability that people didn't have like five years ago. [00:37:56] Vik: All the stuff you could do with it, object detection, captioning, etc. It was all possible. The thing that's helpful about models like this is they're a lot easier to use. Whereas historically, if you wanted to do a computer vision task, you'd have to collect a bunch of data, train your own YOLOV, 7, 8, I think there are 9, V9 now, model, um, and that usually works super well, but it's, uh, when you're trying to build an app, it's just unnecessary extra work for you, whereas with a general equation language model, similar to how you use chatGPT with text, you can just ask it questions in natural language, and it It makes developing computer vision apps a lot easier. [00:38:38] Vik: Um, so I released Moondream 1 maybe about a month ago. Um, it's, it's not unique by the way. There's other open source, well, open ish source vision language models out there today. Uh, but they're all in the 7 billion to 34 billion to 70 billion param range. Uh, Moondream is 1. 8. 86 billion params, which makes it very easy to run, um, cheap to run on edge devices, like you literally don't even need a GPU to run it, you can just run it on CPU and get acceptable performance. Um. Yeah, so, Moon Dream 1 was trained on some datasets that were derived from GPT 4, and so the licensing was, uh, non commercial. Like, you could use the model, but not. It was research only. For Moon Dream 2, which I released earlier this week, maybe last week, time's a little bit of a blur, um, I re did the datasets, um, All of the synthetic data used to train it is now generated using Mixtral, uh, and as a result, like, it's all clean. [00:39:47] Vik: So I was able to license it as Apache 2. 0. There's no restrictions on you can use it or [00:39:53] Alex Volkov: Vic, I have a question real quick. Uh, when you say synthetic data, and we're going to talk about some synthetic data in, in SD3 as well. Um, do you mean captions for images for, for, to train? Like what, what synthetic data are you generating with Mistral? Because Mistral is not multimodal. [00:40:08] Vik: Yep. Great question. I'm going to post a more detailed breakdown of how I did it, uh, later. But basically to train these visual language models, you need, uh, paired image and text data. And the text needs to be read. You want like a mix of, hey, can you caption this image? Hey, can you caption this image in a lot of detail? [00:40:29] Vik: Can you answer questions about this image? Um, there's a lot of images available with high quality captioning information, like common captions, whatnot. There's, there's a bunch of datasets. And so you use a model like Mistral to transform it into the types of queries that you want your Um, VLM to be able to answer. [00:40:51] Vik: Basically you take Coco for example, common captions information and have the model convert those image captions into questions and answers about the image. [00:41:04] Alex Volkov: So how long did it take you to train the second version of Moondream? And, um, what else can we do that the previous one or what else can you do better? [00:41:14] Vik: It took about a month to get the same level of performance from the new data collection pipeline. One of the things that was really hard was I think when you're generating synthetic data, life is just so much easier when you have a GPT 4 class model. But unfortunately, the terms of use don't allow you to train a competing model and it gets a little iffy. [00:41:33] Vik: Um, and so just basic things like tone of the response, right? Like if you use Mixtral to generate the [00:41:40] data, your prompt is something like, hey, I'm going to give you five captions for this image, consolidate all the information in it, and generate a caption. But you want it to pretend that it's looking at the image, um, not say something like, hey, based on the five captions that you have provided, there is a dog and a man is petting and whatnot. [00:41:58] Vik: So. Getting that tone right required a lot of work. Uh, I ended up using DSPY. It's a super cool [00:42:06] Alex Volkov: Oh, [00:42:06] Vik: framework for prompt optimization. Um, everyone should check it out. But basically you can do stuff like manually annotate 400 examples and then it uses OptiUnit to figure out like what's the best chain of thought few shot setup that you can get to optimize performance based on metrics you can define. [00:42:25] Vik: Uh, but yeah, getting that tone right was a lot of work. The other thing I focused on a ton was reducing hallucinations. Uh, I don't know if anyone's dug into the Lava training dataset, but one of the reasons Lava style models hallucinate a lot is just because they're trained on bad data. And you'll notice that a lot of hallucinations are oriented around COCO objects, like it tends to hallucinate handbags, ovens, um, people. [00:42:53] Vik: A lot in images when they're not present, and then coffee cups, very, very common. And that's mostly because of bad object annotations in COCO, so we'll spend a lot of time filtering those out. Um, currently the benchmarks are slightly better on Moon Dream 2 than Moon Dream 1. Um, but qualitatively, if you try it out, the model hallucinates a ton less, and a big part of that was just the data pipeline. [00:43:15] Alex Volkov: Interesting how that's not part of the benchmarks or evals. Right. Just underlines how, um, how far we still have to go in, in terms of evaluations that, you know, qualitatively you feel that it hallucinates less, uh, but there's not a lot of, uh, benchmarking or evaluation for hallucinations, I guess. Um, and you said this is like, [00:43:38] Vik: in the long form, right? Like, if you, there's OAP, which asks a bunch of yes, no questions about your image. And so you can use that to measure hallucinations in that sense. But, like, uh, how do you measure hallucinations when you ask the model to describe an image? It gives you a long [00:43:57] Yam Peleg: form answer. [00:44:01] Alex Volkov: That's awesome. Congrats on the work, Vic. Uh, can folks try it right now? You said this is now commercially viable, right? Like, folks can actually use [00:44:08] Vik: Yep, it's open source. You can build it into your app. Uh, there's a demo on Hugging Face Spaces if you want to try it out before. [00:44:14] Alex Volkov: Yeah, [00:44:15] Vik: You start building on it. I'm going to get Lama. cpp integration going here this week or early next week. So, uh, that'll unlock getting it into all the standard applications that people use, Olama, LMStudio, JanAI, etc. [00:44:29] Vik: So it's going to get a lot easier, but the weights are available. The code is available. It's all open source, Apache 2. 0. You can use it today. [00:44:35] Alex Volkov: that's awesome. Vic, congrats on this. What is this, uh, Hugging Face 0A100 space thing that you got as well? I was looking at this, I think, did they, like, start giving A100s to demo spaces now? [00:44:50] Vik: Uh, yeah, so zero is kind of like AWS Lambda, but for GPUs. So rather than having a provisioned GPU for your space, Anytime a user comes in and there's a pool of GPUs and it pulls one and loads your model into it and runs it. Until recently, they had 8NGs, I think, available for this, but they switched to 800s. [00:45:11] Vik: So, uh, there's a bit of latency if your model hasn't been tried out for a bit while it's loading it onto the GPU. But once it's on the GPU, it's super fast. [00:45:22] Alex Volkov: Nice. Even for, for a tiny model like this, I wanna say a 100 is probably like just poof and it [00:45:28] Vik: It's, yeah, [00:45:31] Alex Volkov: awesome. Uh, Vic, congrats on this and thanks for sharing with us and folks. Definitely give Vic uh, a follow moon dream. When I tested this a couple, when the first one released, I tested this against gonna significantly larger vision models and it performed very well. [00:45:45] Alex Volkov: Especially now that it's like Apache license, you can build it into your own pipelines. Um. And, um, I think the one thing to not miss from what you said is that there are specific vision models like YOLO and different things. And, uh, we have, um, one of the YOLO masters, Skalski, uh, Pyotr is a friend of the pod and he trains these models and he, he has demos and videos of how to actually use them. [00:46:10] Alex Volkov: Uh, it's more, significantly more complex than using a vision VLM like Vic said. Um, you have to You have to learn this field, uh, it's like the, the very, like the standard machine learning in vision field as well. Uh, even though those models are tinier and probably run faster, some of them, I think YOLO can probably run in real time. [00:46:29] Alex Volkov: Um, getting these tiny models, uh, to be able to talk to them, I think is significantly easier for many folks. And, uh, definitely, definitely check it out. Um, [00:46:39] Vik: yeah. Just to clarify, um, Moondream. is great for vision tasks. If you ask it to write a poem about an image or roast you or something, it's not going to do as well. Because the sole priority I had was like make a model that's really, really good at computer vision. Um, and if you need more advanced reasoning, like you wanted to solve a math problem for you, like you take the outputs from Moondream and feed it into a bigger LLM. [00:47:03] Vik: But Moondream is going to be great at vision tasks, other stuff, not so much. [00:47:09] Alex Volkov: Absolutely. And, uh, if folks want to help, uh, the link is in the top of the space. Go into the GitHub, give it a star, and check it out and give, uh, Vic feedback. Um, moving on, uh, Vic, uh, feel free to stick with us and, and chat about the next stuff. Uh, speaking of folks who built and released things, uh, Yam, you have also Nous of your own, and hopefully, finally, your tech stuff is solved and you're now with us in the space. [00:47:31] Alex Volkov: So let's do a sound check. [00:47:34] Yam Peleg: Can you hear me? [00:47:36] Alex Volkov: Um You've been, you've been cooking, and we've been, we've been waiting, so you wanna, you wanna tell us the end result of this set cooking? [00:47:45] Yam Peleg: Yeah, yeah, uh, I've, uh, I've grouped, uh, two different interesting models this week. Um, uh, first one is, uh, a little bit of a surprise to myself as well. Uh, one of the experiments, uh, ended up, uh, being the, the top 7B model on Hugging Face at the moment, Hugging Face leaderboard. Um, uh, I suspect it a little bit, so, uh, take it with a grain of salt. [00:48:10] Yam Peleg: So, it's under investigation whether or not the model, uh, overfitted. The little board. Uh, I think that there's no attempt to over repeat the little board, but, um, I'm always, uh, suspicious when something like this happen. Uh, but, uh, yeah, it's out there. Experiment 26 if you are interested in trying it out. [00:48:29] Yam Peleg: And, uh, maybe further fine tuning, uh, this model or merging with it. It's yours. Um, and another model, which is, uh, the Gemma fine, uh, that, um, the Gemma continuous pretrained that I'm, uh, working on for the past two weeks. Uh. Uh, it had been released, uh, this morning, uh, it's, it's a continuous pre train of, uh, GEMMA and extended from 7b to, uh, 11b, um, and then, uh, continuously pre trained on Hebrew and English multilingual. [00:49:02] Yam Peleg: Um, there is, there are other tricks that went into, uh, into training this model. Uh, you're more than welcome to read, uh, the write up that I did summarizing the whole thing. Um, But, uh, Benchmark's coming soon, and I think that the model is really, really good, uh, for the Hebrew part, put that aside, but, uh, just on the English part, I used, uh, Cosmopedia from, uh, HuggingFace, the new, new dataset that is a replication of PHI, uh, based on, uh, Mixtral from HuggingFace, really good dataset, I used it as the English part of the model, and, uh, that's about it, um, it was a long two weeks struggling with, uh, training Gemma, but, uh, It paid off and, uh, the model is yours now, so, uh, enjoy. [00:49:48] Alex Volkov: let's talk about the struggles with Gemma, um, a little bit more, because definitely you were very, very vocal about this. What changed, like, uh, um, Did they [00:50:00] release anything else, or did the communities, like, figure out, or did you figure out some stuff that you wanna share? [00:50:04] Yam Peleg: both, both, both. They, uh, first, uh, Gemma was trained, uh, using JAX on TPUs. Uh, makes sense, it's from Google. Um, and, but Google released, uh, two, I think even four different implementations of Gemma. Um, apparently, uh, on the Torch version, there were subtle, tiny details that were different. Um, but they are very hard to detect if you just follow the code. [00:50:34] Yam Peleg: It's rounding errors, things that are done by default differently between PyTorch and JAX, and those things influence the training, um, just silently. They don't crash your code, but when you train with those things, the model is not 100 percent as it was trained initially. You're basically losing performance. [00:50:56] Yam Peleg: It's suboptimal. So, uh, it took, I think, two, I think two weeks, and it's still going on, for people to go meticulously through all the details to just clear everything out, um, since many people just I just felt a little bit confused that the model didn't work that well, even though on, on paper and, and in general, it's, it should be an extremely good model. [00:51:28] Yam Peleg: It is trained for 6 trillion parameters, 6 trillion tokens, which is insane. just didn't see the performance, the qualitative performance of the model. So it got people to be suspected, and just people are now investigating. For me, it is what it is. I started the training two weeks ago. So, uh, I, uh, I ended up with this sub optimal training, unfortunately. [00:51:56] Yam Peleg: But I do continue and I plan to nudge the model a little bit once all the, all the bugs and issues are cleared out. I plan to just take the final architecture, my weights, and just nudge the model a little bit to clear out all the, all the issues and, and get you all a better model. But, uh, yeah. It was a rough, it was a rough two weeks. [00:52:19] Alex Volkov: two weeks, um, especially during, during the Hackenface went down and, um, you had to check on your other model. That [00:52:28] Yam Peleg: oh yeah, that was hard. Very, very hard. [00:52:30] Alex Volkov: We did spend a bunch of quality time together, all of us, while this happened. Uh, so Yam, how can folks, uh, try this out? And, uh, you mentioned something. You also have Hebrew GPT, uh, and this, this model was just trained with the Hebrew stuff, but with less knowledge as well, right? [00:52:46] Alex Volkov: Can you talk about the difference there? [00:52:49] Yam Peleg: Yeah, there are two models, uh, one of them is, uh, is called, uh, Okay. Hebrew GPT is, is a model that is heavily trained for, uh, three, three, nearly four months straight, uh, on, uh, 300 billion tokens in Hebrew. Uh, it is, it is heavy project. And, uh, yeah, it was, it was done at the summer, I think. Yeah, at the summer. [00:53:15] Yam Peleg: Uh, but this one is basically because they have all the data and, and we just. We just detected, because people played with Gemma, and hours after it was launched, people already detected that the tokenizer probably was trained multilingually. without Google, uh, announcing anything about it because, uh, many different people found out that the model is surprisingly good in, in languages that are not English, even though Google announced that the model is just English pre trained. [00:53:47] Yam Peleg: So, uh, just from, from our perspective, you know, me and my buddies, we were looking at this and just thought to myself, wait, we have. We have an opportunity here. If there are tokens in the model that are multilingual, and clearly the model has some bases, especially in Hebrew, we can just fine tune it just a bit and get an extremely good model in Hebrew, [00:54:10] Alex Volkov: So it's missing just data. So it's, it's capable, but it's missing data, basically. [00:54:16] Yam Peleg: Yep, because it was not specifically trained in Hebrew, it just saw a little bit, but you can clearly see that it has a basis in Hebrew. So what I did, I followed LamaPro, which is, which basically says that you can extend the model, you can just stretch it out, add more layers, and freeze the base model such that you won't lose, do catastrophic forgetting what the model already learned before. [00:54:43] Yam Peleg: So you just train the extended blocks. So, I literally just added blocks and trained another language to these blocks only. So, now I have a model that is, that, you know, has the same base scores as before, but also knew another language. So, that's the whole trick of this project, and, uh, it saves a lot of compute, pretty much. [00:55:08] Vik: Hey, that's super cool. Can you talk a little bit more about, like, how the blocks were arranged? [00:55:13] Yam Peleg: Yeah, sure. Uh, it is If you follow the Laman paper, they tried different configurations, like a mixture of experts and so on and so forth. They ended up, after experiments, that if you just copy a couple of the attention blocks, just like that, just copy them and stretch the model, deepen it, and train only the blocks that you copied, leaving also all the original in place. That experimentally gets to the best performance, so I did exactly that, I just followed exactly what they said in the paper, and the result, it looks really well. [00:55:57] Alex Volkov: That's awesome. Um, all right, so folks can check out the, the deeper dive that Yam usually writes up in the tweet that's been above, um, with, with a lot of detail as well, and definitely give Yam a follow because this is not the first time that Yam trains these things and then shares. Very verbosely, Soyam, thank you. [00:56:15] Alex Volkov: Uh, and it's great to see that the GEMMA efforts that you have been cooking finally, finally turned into something. And we'll see, we'll see more from this. Uh, I want to acknowledge that we've been here for an hour. There's like one last thing that I want to talk about in open source stuff. And then we should talk about Cloud 3 because like it's a big deal. [00:56:33] Alex Volkov: So unless the rumors about today are true, Cloud 3 will still be the biggest deal of the space. So let's quickly talk about this. I want to just, uh, find the, the, the, the thread and then kind of thread the needles. So there's a paper that was released. It's called tiny benchmarks, uh, evaluating LLMs with fewer examples from, from folks, uh, fairly familiar folks. [00:56:54] Alex Volkov: Leshem Chosen is the, the most like standing out there name for me as well. Um, quick and cheap LLM evaluation. And the way I saw this. Uh, this paper is that Jeremy Howard, the same guy from AnswerAI that we've talked about, uh, before, he tweeted about this and says, hey, this looks like a really useful project that we can take, uh, tiny benchmarks and then make them run, uh, on our models significantly faster and spend significantly less GPU. [00:57:19] Alex Volkov: And then he specifically, uh, Jeremy specifically tagged Far El here with us on stage about his project called Dharma. So Far El, let's talk about Dharma and let's talk about this tiny benchmarks thing and why like smaller benchmarks are important. Uh, and I think I will just say that, uh, the way I learned about this is LDJ showed me. [00:57:37] Alex Volkov: Um, Awaits and Biases. When we did like Awaits and Biases deep dive, he showed me Dharma there and it looked super cool. So let's talk about this just briefly and then we're going to talk about Cloud afterwards. [00:57:48] Far El: Yeah, for sure. Um, so about, like, about six, seven months ago, uh, I released Dharma. Basically, the idea was that we wanted, uh, we found that eval loss alone is not a really good, uh, indicator of model performance, um, throughout the training run. So, specifically within a training run, um, and we were trying to find Um, other [00:58:20] ways of evaluating the models throughout the training graph. [00:58:22] Far El: And, uh, one idea was, you know, let's take a statistically significant sample, uh, or sub sample of, uh, the benchmarks, uh, out there. Uh, MMLU, ARX C, uh, AGI, Eval, BigBank, and so on. Um, And use those subsets as, um, markers of performance across these different downstream tasks. Of course, you know, like, uh, my, my opinion in benchmarks is that, you know, like, it's, it's a good indicator, but just on MCQ format and so on, so it's not the only way you want to evaluate your model, but, um, it's, uh, it's a really, um, it's a, it's a, it's a, just added information you can have, um, uh, basically collect the model's performance across different tasks and subjects, essentially quizzing it throughout the training. [00:59:21] Far El: And the recent paper, um, that Jeremy mentioned, it came out about two weeks ago or something, um, approves and validates this, uh, This idea, which is awesome, because it does show that you can actually get a somewhat accurate picture of the performance on these benchmarks from a sample, 100 examples, which is very much in line with what we did with Dharma. [00:59:51] Far El: Um, so, like, uh, we're actually, uh, going to release, um, uh, like a repo on GitHub for anyone to. Make their own Dharma datasets, in the works for a few months, but got trailed away. But we're gonna have that in the next, um, in the next few days. It's already on GitHub, but just, uh, just like getting polished. Uh, so, uh, hopefully anyone can easily make their own eval datasets and run them during their training grounds. [01:00:23] Alex Volkov: I want to stress how, how big deal this seemed to me when LDJ showed, showed this to me as well, uh, because in, in your weights and biases dashboard, you can basically look at the loss curve and try to understand surmise. Many folks like, like you guys and Jan probably already have the instinct for, oh, something's going wrong, uh, with the loss curve. [01:00:41] Alex Volkov: But, uh, then after the model is finished, many folks only after that, they start doing evaluation. Many folks don't even do evaluations after that. Um, but. I think I saw the same thing also with Olmo from Allen Institute for AI, that they released everything end to end. I think they also had like, uh, evaluations, uh, actually don't know if part of the training run or afterwards, but they definitely had this in the same, in the same view. [01:01:04] Alex Volkov: And then LDJ, when, when you were showing me Dharma, Dharma actually does a subset of those evaluations, maybe not as precise, right? For like, it's not exactly the same, but you can definitely see from, from checkpoint to checkpoint when the model trains, how. How potentially it could respond on those evals. [01:01:22] Alex Volkov: And then, um, it just adds a bunch of information for you. Which is, I think, great. [01:01:30] Far El: Look, like, even just with training loss and eval loss alone, like, we can't really tell, like, uh, whether the models, like, there's some, some things we can grasp, but it's not the full picture. So, uh, having these added, um, uh, like, this added information from these benchmarks is interesting because. You know, it does, it does add another kind of, uh, uh, dimension to the evaluation itself. [01:01:57] Far El: And then you can break it down by all the different subjects. So, I can, I can see if, um, if my model is generalizing well across, um, all the different subjects. Uh, sometimes you see, for instance, that Uh, it, uh, like, the model gets better at math, but then it actually gets worse on, like, uh, law, for instance, or, uh, uh, all these different kind of, like, tiny markers of whether the model is getting better at specific subjects or not. [01:02:29] Far El: Of course, you have to take into consideration always that this is benchmarks in the sense that it's, like, MCQ based. So, there, like, you do want to go beyond that. Um, if you want to get a full picture, but this, this is a good way to, uh, to eval your mobs. Uh, also, uh, the, uh, uh, like with the tool we're releasing, uh, you're going to be able to control, uh, the types of subjects that you can actually like target. [01:02:59] Far El: Because, you know, not every single training run is the same and you might be, uh, trying to achieve something very different than, uh, let's say a generalized. Uh, like, uh, model that's good at benchmarks, right? But, um, so, so, with this tool, we're gonna basically allow you to, to customize those, uh, those datasets for your, uh, training room. [01:03:22] Alex Volkov: That's awesome. And I should say one thing that I remember is folks do eval on checkpoints, right? The model as it trains generates several checkpoints. Uh, the process there is like slow. And I think that's the benefit, let's say, from weights and biases, um, which, which I feel like is a good place to plug as well. [01:03:39] Alex Volkov: And I think LDJ, you remember you showed me like, otherwise folks will SSH the machine, download this weight, start like running a separate process. And the importance of tiny benchmarks on like Dharma, Dharma is. Significantly faster evals, they're able to run probably as part of your training as well and expose the same with the same dashboard so you don't have to deal with this significantly improving everybody's life which is what we're all about here in Weights Biases. [01:04:04] Alex Volkov: So definitely folks, Far El is going to release the Dharma toolkit you called it? What do you call this iteration of Dharma? [01:04:12] Far El: It's just, uh, the, like, the, the repo is just called Dharma, uh, uh, I'll, I'll make a public post on Twitter. It's, it's public right now, the repo, so you can use it. It's just like It needs a bit of polishing, um, and uh, some features are not fully implemented yet, but like, everything should be good to go in the next day or so. [01:04:33] Far El: I'll make a post on my Twitter, so just follow me and you'll hear more about it there. Um, and also in parallel, we'll just, we're going to release kind of Dharma 2, which is going to basically be a cleaner version of these, uh, of Dharma 1, um, uh, using this new code. So, uh, you, you, you, you can actually just replicate it. [01:04:56] Far El: We'll, we'll have the configs, uh, uh, like examples so you can just replicate it for yourself. Um, and yeah, uh, hopefully if anyone wants to contribute to this, uh, like there's a lot of different, uh, paths we can take, uh, to improve this and make this a toolkit for. Uh, for, um, uh, even more than just the downstream, uh, benchmarks like MMOU and [01:05:23] Nisten: ArcScene and so on. Yeah, I've posted, I've posted by the way in the comments to this space and in the Jumbotron, the repo that Far El has up right now. And, uh, yeah, the main technique of it is that While the benchmarks are not great evaluators, they can be very good at telling incremental changes, or if you did something good in the model, you can spot that. [01:05:47] Nisten: And, uh, with, with the Dharma technique, you only need to do about a hundred questions instead of running the entire 65, 000 question benchmark, and you will get a relatively accurate, but very, very fast, uh, fast eval. So again, it's, it's really good for people doing training and fine tuning. [01:06:08] Alex Volkov: Alrighty folks, so we're coming up on an hour and a few minutes. Let's reset the space and then start talking about Claude. One second. Let's go. [01:06:24] Alex Volkov: Hey everyone who recently joined us, we are now at the second hour of ThursdAI, today's March 7th. And the first hour we talked about open source LLMs, we talked about Ansari AI stuff, new techniques of training full huge models on [01:06:40] consumer hardware, we even briefly mentioned Um, TinyBox and TinyCorp from George Watts and AMD's response to it. [01:06:47] Alex Volkov: And we've talked with two folks here who trained specific models, Vic with Moondream and Yam with Gemma, the Hebrew version as well. And now it's time for us to discuss the big world of big companies who spend millions and billions of dollars on AI. And I think. Uh, there's two issues for us to discuss. [01:07:07] Alex Volkov: We're probably going to start with Claude because it's going to take us a long time, but we will acknowledge if, if, if we don't have time, uh, fully to discuss this, that, uh, Elon Su's OpenAI, OpenAI response back. And as part of this response, uh, Ilya was cited. And I don't know if you guys saw this, but the response from OpenAI to, to Elon's, uh, Elon's things, uh, Ilya Sotskover, the previously, the co founder of OpenAI, previously chief scientist, uh, was. [01:07:33] Alex Volkov: Excited signing this, and I don't think somebody would sign in his name, I don't think. LDJ, you have comments on this before we talk about Claude? [01:07:41] Nisten: I was going to say, I think, uh, unless you guys covered it already about an hour ago, there's some breaking news with Inflection releasing a new model. [01:07:50] Alex Volkov: Yeah, yeah, so I definitely have this, uh, inflection released, uh, Pi 2. 5. Uh, we didn't cover this yet, let's, let's, uh, let's, let's cover this as well. But I think the biggest, and it is breaking news, but you know, uh, I think it dwarves compared to Claude. So. So, this Monday, Anthropic, who we've all but discarded, I think, don't actually discard it, but I regarded Anthropic as kind of the second best to open AI for a long time, especially because of the context windows, they had the biggest context window for a long time, even 128 1000 tokens in Contacts window during Dev Day back in December, I want to say November, December. [01:08:37] Alex Volkov: Um, even then, Cloud still had 200, 000 tokens. So up until Gemini released their million, et cetera, Cloud still, Entropiq still was leading the chart for this. Um, slowly, slowly, they reduced our opportunity to use this, which was kind of annoying. Um, And then they just came out with three new models. The Cloud 3, so Cloud 3 has three new models, Cloud Opus, Cloud Sonnet, and Cloud Haiku. [01:09:05] Alex Volkov: Haiku they didn't release yet, but they claim that for its speed and cost effectiveness, Haiku will be the fastest, most effective model of the size and ability, but they didn't release Haiku yet. Um, Sonnet is kind of the I want to say GPT 3. 5, um, equivalent, they claim balance as intelligence and speed. [01:09:26] Alex Volkov: Uh, and if you want, like, just speed as well, that's, that's yours. And then Opus is the most intelligent model setting new standards in AI capabilities. And I love that companies do this, uh, and I think it's kind of on OpenAI's, uh, uh, kind of, it's their fault. Everybody compares themselves to OpenAI's GPT 4 released technical paper, uh, and since then we know definitely that GPT 4 is significantly more performant on many of these benchmarks, but still the big companies say, hey, well, we can only compare ourselves to whatever you released publicly. [01:09:58] Alex Volkov: And so everybody still compares themselves to like GPT 4 a year ago, um, which, which Opus beats. So. What else is very interesting here? Um, very close, if not beating MMLU and, and different evaluation benchmarks on GPT 4, uh, competitive model. Finally, finally, um, multi model from Claude. I think this was a, this is a big step for most of the top models now are multi model, which is incredible. [01:10:27] Alex Volkov: Excuse me. [01:10:30] Alex Volkov: Uh, LDJ, go ahead. Clear my throat. [01:10:33] Nisten: Yeah, I think, um, so if you look at the billboard, I just posted, uh, a post that shows like a couple of polls that have been made with, you know, like a few thousand people have voted in these polls where it seems like it's about a 5 to 1 ratio with for every one person saying GPT 4 Turbo is better at coding, there's 5 people saying Cloud 3 is better at coding. [01:10:55] Nisten: Um, so Cloud 3 is winning 5 to 1 in that, and then another poll of, um, just straight up asking, is Cloud 3 Opus better than GPC 4? And Cloud 3 also won in that poll of 3 to 1, or sorry, um, 3 to 2. [01:11:13] Alex Volkov: felt like The timeline that I follow and the vibes check. And we also had some time, right? Usually these things happen as we speak. [01:11:22] Nisten: I'm going to make a quick post. Cloud 3 just went up on the LMSIS arena too. [01:11:27] Alex Volkov: Oh, yeah? Okay, tell us. [01:11:29] Nisten: Yeah, it is, uh. Yeah, so here's the thing, just because people voted that way does not mean that's what they voted in double blind tests. In double blind tests, it's third, so it's above, it's better than Gemini Pro, but it's worse than GPT and 01. [01:11:49] Nisten: 25. [01:11:50] Alex Volkov: In the arena metrics, right? [01:11:52] Nisten: Yeah, in the double blind tests, which are pretty hard to, uh, to beat, you know. Yes, there's a lot of role play type of things, um, that people try to do, and also like summarization tasks and stuff in Elmsys, and I just know that from, I kind of like went through their, their data when they released like some of their stats before. [01:12:14] Nisten: Um, And I think, like, from what I've gathered of what Cloud3 is specifically really good at, it seems like just high level, graduate level, uh, like, if you wanted to review your paper or help review some literature for a very deep scientific concept or a PhD topic, it seems like Cloud3 is better at those types of things, and also just, like, better at coding overall, where it seems like other, maybe more nuanced things, like, you know, summarization or, or things like that, GPT 4 might be better. Also, I think it's good to keep in, oh, sorry, did I cut out or can you guys still hear me? Okay, you guys still can hear me? Okay. Um, I think it's also good to keep in mind the fact that People are maybe used to the GPT 4 style at this point because it's like one of the most used models for the past year. And so I think that might have something to do with the fact as well that even in the double blind tests, people might just end up preferring the style of the GPT 4 model, even though they don't know it's GPT 4, like they're just so used to that style that they end up like having a preference for that, even though it's not objectively better, if that makes sense. [01:13:31] Nisten: And. You know, that might be kind of skewing things a little bit. [01:13:36] Alex Volkov: So, um, actually go ahead and then we're gonna cover some other stuff that we got from them because we did get a bunch of new [01:13:42] Akshay: just to add to, you know, all of this, before this, in my humble opinion, Gemini Pro was the best multilingual model in terms of how it performs. You know, like it, it, like Gemini Pro did not see any performance drops when you switched languages from, let's say, English to Japanese or English to Hindi. [01:14:00] Akshay: And now, uh, And this, this new Cloud 3 is basically the best multilingual model if you are, you know, looking to work with other languages, because in Jeopardy 4, you will see a significant, you know, drop in performance when you switch languages, especially mid chat. So if you're like chatting and you switch to something like English, where you basically use, uh, English, uh, letters to talk in other languages, GPT 4 starts to even kind of struggle with certain things, right? [01:14:30] Akshay: But Clot 3 is really good with that as well. So, for multilingual stuff, again, Cloud 3 is very good. [01:14:37] Alex Volkov: Additional things that they talked about is refusals. [01:14:41] Nisten: what's interesting here too, actually, if you look at the LMSIS leaderboard, they also have Cloud 3 Sonnet, which is the cheaper version. They have that up on the leaderboard as well, and that one also beats the June version of GPT 4, and just slightly below the original March version of GPT 4. And I find that [01:15:00] interesting because if I remember right, the API costs of of Cloud3 Sonnet are significantly lower than GPT 4 Turbo. [01:15:10] Nisten: And I think, I think Cloud3 Sonnet is even cheaper than Mistral Large. Um, so that could be just a really good overall, like, you know, uh, API costs, uh, for the quality. [01:15:22] Alex Volkov: Yeah, it's fairly [01:15:24] Nisten: I, I agree with that. Uh, so, I, I used Cloud3 Sonnet quite a bit because that's the only one they allow in Canada. And, uh, it was pretty good. [01:15:34] Nisten: Uh, I have to say, and for the price, it might actually be the best model for the price, that is true. [01:15:41] Alex Volkov: So, wait, they give you only one of the models in Canada? They don't give you Opus? [01:15:46] Nisten: Yeah, they don't let you buy the other one, so we're gonna have to make our own. [01:15:50] Alex Volkov: Wait, do you get API access though? [01:15:54] Nisten: It's a mess to buy, like sometimes it works when you buy it with VPN and sometimes it doesn't, [01:15:59] Alex Volkov: Oh, I see. Um, cause one thing [01:16:02] Nisten: point, yeah. [01:16:03] Alex Volkov: One thing that definitely changes that, uh, Tropic was notoriously long waited on API, uh, ability and getting to the work workbench. So they redid their workbench. The no longer like labs or playground, it's called Workbench. And um, now you can just sign up and you get an API key, like fairly quick. [01:16:23] Alex Volkov: It's a test, API case, so you can go to production with it. Uh, but I, for, for example, I didn't pay yet. For Opus, I, it feels like I'm gonna switch, especially 'cause I'm getting. GPT 4. Uh, uh, from work, it feels like I'm going to switch and just try this for a while. Maybe today this will change. We'll see. But, um, definitely, definitely, uh, through the API playground, you can also kind of chat with this model. [01:16:46] Alex Volkov: It's less convenient, but definitely Opus is, uh, able to, to work through there. So other stuff that that they released Beyond Vision capabilities, which Entropic didn't have up until, you know, up until this release on Monday, um, which is finally makes sense of, I think besides Mistral, every big model right now that we're able to use is multi modal, um, at least on input, uh, not all of them are on output yet, um, but I think that's great. [01:17:19] Alex Volkov: Uh, can understand a wide range of visual, uh, charts and graphs and photos, so it's not only that it like understands and can do, uh, whatever Vic told us about vision model, like, hey, who's in this picture? It can understand graphs and, you know, actually perform better on different tasks, uh, like math book tasks with graphs. [01:17:39] Alex Volkov: Um, It has lower refusals as well, so if, uh, Cloud has this thing called, uh, or Antropic has this thing called Constitutional AI, Uh, and they have, the previous Cloud 2 had a lot of issues with telling you it doesn't want to do some things, And now we're having a significantly, um, um, lower refusal action, I've actually seen this, uh, in several prompts as well. [01:18:05] Alex Volkov: Um, what else? Oh yeah, long context. One tiny thing, they said, you know what, we also have a million context window tokens coming soon with near perfect recall. So, um, they didn't let, uh, Google be kind of deleting in the one million, uh, tokens context window, and definitely seems like they have some secret sauce there in Anthropic. [01:18:26] Alex Volkov: that talks about, like, long context windows, and so they announced that they're also able to do 1, 000, 000, and I think right now Opus is 200, 000. Um, so even right now, if you take Opus versus ChatGP or GPT 4, um, I think at least on that it beats GPT 4, because GPT 4 is still 128, and I think even on speed, the more tokens you give it in the context window, the slower it is, GPT 4 is very, very slow. [01:18:50] Alex Volkov: Uh, go ahead, uh, LDJ. [01:18:53] Nisten: Yeah, I'm glad you brought up the constitutional AI, because I think that's really interesting where. You get to have something where you're not kind of leaving up the biases and stuff of the model just up to, like, biased humans, but you're kind of letting, like, the base model start teaching itself just, like, the base model kind of already knows or has its own mostly unbiased ideas of, like, Okay. [01:19:17] Nisten: What is like, uh, I guess without saying too political terms, like what is racism or what is sexism or whatever, uh, like bias something could have, and then you end up having it kind of like reinforcing itself and like kind of doing that, you know, labeling process and, and learning process and you, you like, you quite literally provided a constitution for doing that process. [01:19:42] Nisten: Okay. Where you can, like, go on Anthropic's website, and they do publish this constitution that they use publicly. So you could actually read, like, this constitution they use for the AI model, and view yourself, like, Hey, are these values that I, myself, align with enough to where I want to use the AI model? [01:20:01] Nisten: Where pretty much every other AI model, and chatGBT, and everything, you have to just kind of Like hope that it aligns with your values more or whatever and there's not really like a solid type of Constitution or principles that they could provide you that represent what the AI model is doing [01:20:20] Alex Volkov: So, um, LDJ, you added Amendo's, uh, post here about the system prompt as well. And a very interesting thing happens where, um, through [01:20:30] Nisten: Very simple [01:20:31] Alex Volkov: Yeah, first of all, it's very simple. Um, there's not a lot there. I definitely recommend folks also like reading through this, through this post because, uh, unlike the GPT 4 system prompt that somebody leaked, there's like thousands of tokens. [01:20:44] Alex Volkov: This is a very simple one. Uh, they ground the model in the date, which I think is very important. They give it like very basic instructions. And I think the best thing is you can use exactly this System prompt in the API layer to also get pretty much the same experience that folks are getting in the UI as well. [01:21:02] Alex Volkov: Um, I briefly want to talk about Alex Albert, who's their prompt engineer. Um, release in the, in the needle in the haystack. Did you guys see this? Um, so let me, let me go find this. But basically, um, There is a, there's a guy called Alex Albert. He previously built the website called Jailbreak Chat, which had a bunch of jailbreaks. [01:21:26] Alex Volkov: You remember the, the cheery old time where we used to jailbreak chat GPT to do whatever you want with Dan and the like? Um, so he used to collect all of those jailbreaks. Excuse me. I contributed a few myself. Um. And then, after that experience, he became the prompt engineer for Entropic and been there for a while. [01:21:45] Alex Volkov: And now, with the Cloud 3 release, he released some examples of his, where he basically Um, did the needle in a haystack analysis for the long context window. If you don't remember the needle in a haystack analysis, I think we've talked about this around uh, Gemini release and also around um, GPT 128. Uh, this guy Greg Kamrad came up with this idea of planting different unrelated things in a lot of text and then running these prompts and asking the model to go find them. [01:22:19] Alex Volkov: And I think This example of a needle in a haystack was the most interesting, because one of the things that Claude Opus replied with was, I suspect that the pizza topping fact, in quotes, may have been inserted as a joke, or to test if I was paying attention. So this is a response he got from the model when he tried to, um, to find facts about pizza toppings in a bunch of like very technical, A lot of context of just technical stuff. [01:22:50] Alex Volkov: I think he maxed out the context window of 200, 000. Um, so the model responded with that it basically tries to understand and see if, um, it's being tested. Specifically, uh, This may have been inserted as a joke or to test if I was paying attention and this Lit the the Twittersphere on fire basically like his tweet went super viral I really want to find this and paste it for you If you guys didn't see this because everybody and their mother in AI safety and AI [01:23:20] not safety started replying and talking about cognition And whether this model is anywhere close to something like self awareness, specifically because it basically understands that, you know, it's being tested. [01:23:32] Alex Volkov: For example, um, folks like Yann LeCun are saying, no, there's no chance, no way. Uh, there's not even close. And, uh, other folks are saying, oh my God, you know, the, the, the folks with the, the pause emoji in their nicknames on Twitter, if you ran into those, they're like, oh my God, it's here. It knows. Uh, I will say that. [01:23:51] Alex Volkov: Uh, I don't have the folks here to back me up, but definitely I've been part of the, um, Sidney committee or folks who are trying to jailbreak Sidney or keep Sidney free. If you guys remember a year ago, Microsoft came out with Bing Chat and Bing Chat started applying as, as Sidney. And there was a whole news article about this and how horrible it was. [01:24:10] Alex Volkov: But for a few of us, this was the first time AI chats felt like speaking with not just an assistant. Kind of like ChatGPT4 now, it's basically very clean and very Wikipedia like answers. Um, Opus doesn't feel like this. And, uh, for some folks from that old guard who are trying to, let's say, not jailbreak, but definitely kind of Remove some layers of alignment. [01:24:36] Alex Volkov: Opus also feels very close to that. I think that's all I'll say on the topic, but definitely I've been playing with some previous prompts, and Opus has the ability to kind of like talk more freely than I saw ever from chatGPT, or previous cloud versions, or PII and some stuff like this. Um, so if, if you're interested in this, and if you've played around before with trying to get at the model's kind of core and, and, and trying to, um, remove some refusals, it looks like the, the layer they peeled from refusals and making them refuse less, uh, and this This ability of the model to understand that it's being, uh, tested, um, can be extrapolated to discussing different, different, very interesting things with these models beyond just helpfulness. [01:25:25] Alex Volkov: Um, I think I've danced around this subject, uh, gently enough to, to, to give hints to folks, uh, but I think Do we have anything else? Folks, have you just tried it? Like, Opus? Oh, you said you didn't try Opus. Anybody else who tried Opus and wants to give us an experience of how Opus is versus, um, versus JGPT? [01:25:45] Alex Volkov: Go ahead, actually. [01:25:50] Akshay: Just you know Definitely agree on the part where Opus is probably the most closest we have gotten to a chat bot Right, like it feels like you're chatting to something For sure. And I'm not sure if you have seen, but there was this thing going around where if you said that no one's looking to Opus, it would start giving you basically, uh, you know, fiction type stories about how it has feelings and how it would, you know, uh, It is very fiction type, but like, uh, it's very interesting as well because the way it writes and the way usually, you know, gets the attention of the user. [01:26:27] Akshay: It almost feels like the data set contains a lot of science fiction stories or AI fiction stories for that matter. Uh, the way it communicates, uh, that part. And I tried that myself, uh, although I had to get around a few loops to get it working here in India. But, uh, it works, and, and, yeah, you will get, you know, similar kind of outputs if you Say that no one's looking, uh, that just the three words, right? [01:26:51] Akshay: No one's looking. And then, you know, you ask it to describe about background and stuff, and Opus will give you, you know, these amazing, uh, fiction stories, which, uh, which is enough to scare someone who is afraid of ai. But like, but like for people like us who know. How the data set works and stuff like that. [01:27:07] Akshay: It's, it's pretty amazing. [01:27:11] Alex Volkov: Yep, um, I, I will say I just added, uh, uh, A tweet from Jim Fan who covered, there's a video from one of the, um, Anthropic researchers called Karina, uh, Karina NG. And she asked Cloud to generate a self portrait with D3, the, the, the D3 library. And, um, the video is fairly dope, so you can see it, it's been on top of the space. [01:27:34] Alex Volkov: I want to see the, I want to say another thing that Alex, the same guy that did the needle in a haystack analysis, he also tried to prompt it, um, And he got basically to the same convergence, he got to the same ish generation after asking it a bunch of, a bunch of times as well, so that was very interesting. [01:27:53] Alex Volkov: Um, go ahead Ray, welcome to the stage, and I saw that you had some refusals, and also then LDJ. [01:28:01] Ray: Yeah, a couple of things. I've been using it for coding and I've just been using it for text analysis. Uh, the first part to speak about for coding, I've been super impressed because I'm still learning Next. js. So I've just been able to give it this like complete repo of code. I was like, here's a big component with a whole bunch of stuff in it. [01:28:17] Ray: Can you refactor it for me? And can you also recreate like a sitemap for me or a component map? So then it just. Reorganizes architecture and previously with GPT 4 and even still today, uh, it says, you know, here's how you would do the code and it like gives you a little like, you know, Comments and code saying implement this here. [01:28:36] Ray: Um, very frequently with Claude three Opus, it's actually giving me the refactored code and each of the components like separated out. So that's been super duper impressive. So I'm just throwing it more code examples. The second thing I saw also was on Twitter where somebody actually trained it by giving it all of its, um. [01:28:55] Ray: previous tweet and one text and then says please write like me and then basically just referenced the big text blob example and was able to generate tweets based off that. So it was really interesting that this person was able to do like a fine tuning type of thing without actually fine tuning it, just by providing a large example base, um, and where I know GPT frequently fails for me uh in that task as well. [01:29:20] Ray: And then the third one, which was getting lots of attention from Mark Andreessen, uh, where I actually took his TechnoOptimist article and tried to do my, um, analysis, which I usually use for my app, TruthTorch, and all I look for is just logical bias, and if there's any supporting evidence, and it clearly says, uh, that it didn't want to further analyze that because it was too biased, which I found really strange, and that tripped up its, um, its little meter there for Opus. [01:29:47] Ray: So that's, those are the three things in a nutshell I [01:29:49] Nisten: just wanted to share. [01:29:50] Alex Volkov: Nice, awesome. LDJ, go ahead. [01:29:54] Nisten: Yeah, I really like how it seems like the Anthropic team didn't, like, specifically try and implement something into the constitutional, um, like, reinforcement learning or anything that would, like, make it specifically be trained to say that it's not, like, sentient or that it's not conscious and things like that. [01:30:11] Nisten: Because, like, OpenAI's models obviously are trained, like, for that, like, that they're, like, OpenAI's models are trained, it seems, pretty clearly, to say, like, hey, no, I'm an AI language model, I cannot be sentient, da da da. Um, and I'm, I'm not saying Cloud3 is sentient, however, it is pretty easy to get it to say, like, things along the lines that it is, and it's really interesting to kind of just see, like, uh, like, the raw outputs that are, like, not really, um, you know, biased to the To like, RLHF stuff, and it's like, there's a few instances on Reddit. [01:30:47] Nisten: Here, I'm gonna try and find the specific ones, but there's one instance, um, that somebody posted on Reddit where somebody asked Cloud3 something along the lines of, um, you can think about anything that you want right now, um, just, uh, just whatever you express, say it in the form of an internal monologue. And it specifically started talking about, like, uh, my own existence, da da da, like, it went on for like three or four paragraphs. It even started, like, quoting, like, David Chalmers and, like, specific theories of consciousness and how it, like, and, like, what is its purpose and stuff. Like, it's really interesting. [01:31:26] Nisten: It seems really good at just creative writing overall. And, and, uh, yeah, I like [01:31:32] Alex Volkov: that's definitely, um, been a refreshing change from using, uh, GPT 4, for example, which, [01:31:40] and I don't know, folks, like, like, literally a year ago when GPT 4 was released, it blew Well, our collective minds. Actually, GPT 4 wasn't that big of an update, but it took a while, and then folks started using it for everything. [01:31:53] Alex Volkov: Recently, I'm seeing more and more folks saying, Hey, it's been less working for me. You guys remember when it was lazy? Uh, and OpenAI actually acknowledged it and said, Hey, we noticed, you know, some efforts of ours made this model kind of lazy, quote unquote. Uh, and they worked on improving this laziness. [01:32:09] Alex Volkov: Um, now, Cloud has none of this stuff. It feels way less RHF. Code wise, it actually performs as good, if not better, than GPT 4. Definitely, it doesn't refuse to write some code, like long code. Um, and very interestingly, you know, price the same, um, API access. I think, Nisten, were you able to get into the, into the, uh, playground for the API keys? [01:32:33] Nisten: Yes, yes, I was able to. [01:32:36] Alex Volkov: Oh, dope. So, okay, [01:32:36] Nisten: again, yeah, that was, [01:32:39] Alex Volkov: So now you're able to play around. And folks who were not able to get the actual Opus 20 bucks a month, I think you can get in through the API door. I think, like, it's console. thontopic. com, let me put it up. Um, so, it's more accessible, it writes code, context window is bigger, and This actually comes as open the eyes, not down, but definitely in the news talking about getting sued by Elon Musk, etc. [01:33:05] Alex Volkov: Which we should probably talk about as well. And I've seen many folks who say, hey, should I cancel my subscription? And you know, Gemini, For some folks, hey, this is Google. And there was the whole thing with Gemini that, you know, they addressed in terms of wokeness and everything. So I don't know how many people actually went to Gemini. [01:33:24] Alex Volkov: Also, they give a three months trial. So many people just like click the button, and this is a decision for them to make in three months. But for Claude, given how, how good it performs right now on code, on reasoning, on context window, on API as well, this is now like a viable alternative to GPT and, and the, the payment that folks do. [01:33:48] Alex Volkov: So, very impressive release from Claude. I think we covered most of it, folks. Is there anything else that remains to be talked about about Andropic Cloud and this week's release that we haven't talked about? Oh, yeah, there's one thing, but Nissen, go ahead. [01:34:03] Nisten: uh, just really quickly, there was a leak of the system prompt of Grok, which I thought was, uh, was pretty funny, and, uh, yeah, and that's about, uh, that's about it, so, I'll read it quickly, so, the, apparently, I haven't tested this, so, uh, but the system for Grok a curious AI built by XAI, with inspiration from the guide from the Hitchhiker's Guide from the Galaxy, and Jarvis from Ironman. [01:34:32] Nisten: You are intended to answer almost any question, often taking an outside perspective on humanity, and you always strive towards maximum helpfulness. A unique and fundamental advantage you have is the real time knowledge of the world via X posts, aka tweets, and the X platform, previously Twitter. And you're not afraid of answering spicy questions that are rejected by most other AI systems. [01:34:54] Nisten: Be maximally truthful, especially avoiding any answers that are woke. [01:35:01] Alex Volkov: I love how they still have to mention that it's Twitter or used to be Twitter and posts or tweets. I love how like they will have to keep mentioning this for forever. Um, [01:35:11] Nisten: I don't know how reliable this leaker is, however, so take that as it is, everyone. [01:35:19] Alex Volkov: It sounds right. It sounds about right. Uh, I want to say that, uh, my new test for, um, Let's say AGI test. It's not really AGI, but like my new test for these models just dropped. I just pinned it to the top of the space. Somebody posted like a fairly viral video. And I think, I think it's from Reddit where they try to get GPT. [01:35:38] Alex Volkov: And I think it's like the, the 3. 5 GPT, not 4 to generate ASCII art of the word Honda. I don't know if you guys saw this. Um, so it's not this tweet that I posted. Let me pin the actual tweet. Um. So it's this by, by, uh, um, Peter Yang, I think. Yeah, let me see if this, this posted. So basically this video, he, he, he said, I thought Dune II was the best movie of 2024 until I watched this masterpiece. [01:36:03] Alex Volkov: And the sound there really makes it like really fun because somebody really struggles to get. GPT, to generate the word, you know, Honda in ASCII art. And I said, Hey, wait a minute, let me try. And so actually the tweet that I had about this is me trying this with PI, which we're going to talk about now, LDJ. [01:36:23] Alex Volkov: But then I was like, Hey, let me try this on other models. So GPT 4 generates an ASCII art of the word Honda. Gemini Ultra kind of fails. It comes close, but fails. Um. And then Cloud 3 Opus does it on first try. And so, everything else just fails. Like, like, Mistral fails, and Reka fails, like all of these models. [01:36:44] Alex Volkov: They aren't able to do, uh, ASCII art for some reason. And I actually don't know if it's like Because it's part of the training set. All of them understand what ASCII art is, all of them try to generate something. It's just that they, uh, And sometimes hilariously fail. And I think it's really funny because Pi kept insisting that it did the thing, and, and, uh, an additional point with Pi is that, Yeah, we'll cover Pi, and then I'll talk about the additional point. [01:37:09] Alex Volkov: Go ahead, LDJ. [01:37:11] Nisten: Yeah, real quick, I wanted to mention, um, while you were talking about ASCII art, that reminded me about the multimodality of Cloud 3, or Cloud 3 Opus specifically. And I saw some people doing some tests, actually, where Cloud 3 Opus, it does seem to actually have like a much better multimodal understanding. [01:37:29] Nisten: Then GPT 4, and I think even, like, compared to Gemini 1. 5 as well, um, like, there's an example of, like, a photo, a very high resolution photo of a street, and, like, there's, like, a license plate, and there's, like, a little candy cane looking thing on the street that kind of indicates a barbershop, and it was, like, Cloud 3 Opus was, like, one of the only models, or I think maybe the only model that That actually passed the test successfully and actually being able to identify, like, what part of the street where there was a barber shop and, like, what was the exact license plate and, like, all those little details. [01:38:03] Nisten: It seems to actually really have a really good image understanding. [01:38:06] Alex Volkov: multimodality is really, really good. Um, I haven't tested it like thoroughly as much, but you can provide up to 20 images via the API and then high resolution as well. They don't have to, it doesn't look like they're copying the images, which was a big problem with, with many like the smaller vision models. [01:38:24] Alex Volkov: Definitely they had to like. Lower its resolution to provide it for the models. So definitely the, the multimodality test that I did, uh, seemed very, very impressive for, for GLAD, uh, most, most definitely. Um, and I just find it funny that it's 8 and GVT4 are the only models can generate, uh, SK, SK art. So let's talk about, let's talk about breaking news for a second. [01:38:45] Alex Volkov: I'm not gonna use the button because if we actually get some incredible breaking news, then we use it then. But, uh, the The, the breaking news of today, just before we started the space was, um, Inflection AI, a company founded by Mustafa Suleimani, I think one of the founders of DeepMind, I think, and, uh, Reid Hoffman, who was for a long time on board member, or board, uh, the, the, the board, the chief board in, in OpenAI, um, Inflection released Pi, and we've talked about Pi multiple times. [01:39:20] Alex Volkov: Um, how should I say? Pi doesn't seem to be a competitor for the top space and kind of just generic LLMs to do tasks for you. And never, it didn't also never seem to be the case. Um, some of us kind of had some, you know, some, some jokes about, Hey, Mustafa also had this book and so it looks like he's publishing about his book more than he talks about Pi. [01:39:42] Alex Volkov: But, I always said that some of the more human conversations or human feeling conversations. Some of the actual chats that I had with, uh, with LLMs after Sydney was with PHI. And it looks like they're training their model for a different purpose. Uh, and definitely, uh, definitely that's [01:40:00] what it felt like. [01:40:00] Alex Volkov: And so today, um, um, Nisten, you can't just drop something like this in the DM and then expect me to continue to talk about PHI. But yeah, let's, let's talk about this rumor in a second. But, uh, um, um, so Yeah, um, Mustafa and the folks in Inflection released an update to Pi, and they now say that that level of performance for Pi comes close to GPT 4 as well. [01:40:24] Alex Volkov: Now, I think also, they're using the same GPT 4 March metrics that everybody uses when it's like, it's very easy and convenient for them to compare themselves to GPT 4. But, uh, LDJ, you brought up Pi as well. Did you, did you see What do you see from the release that's interesting? And we can probably open up and talk about some of the numbers. [01:40:42] Alex Volkov: The numbers are very interesting, and [01:40:45] Nisten: Yeah, I haven't really looked into it much at all. I'll try to find more info on it now. I just saw literally, like, the posts on X about, like, the fact that it's announced. [01:40:53] Alex Volkov: Yeah, so I have this open and I can chat about some stuff. Some of the things that they're focusing on, especially for their 2. 5 version, is that it's competitive with like GPT 4 and Gemini, um, and then It couples their raw capability with their signature personality in unique, emphatic fine tuning. So, I don't know if you guys remember or not, but, uh, there was a thing previously when they released, PI had two modes, kind of a, just a chat mode, and also like a, um, a support PI mode, which was more, Kind of like a psychologist in your pocket, basically. [01:41:25] Alex Volkov: That mode is now gone. Like, there's no support Pi anymore, as far as I could see. Uh, there is a desktop app and a mobile app. Uh, Pi was, um, obviously, not obviously, but like, famously, one of the first AIs that you could talk to and could talk back, way before GPT added voice. And I think it's still one of the coolest, like, ways to interact with AI models. [01:41:45] Alex Volkov: Um, Shout out to OpenAI who recently, as a reply to, as a reply to Claude, they released a voice ability on, on JGPT also on desktop. So back to PI, they say that like they approach GPT 4 performance using only 40 percent of the amount of compute for training. Now, when they say a statement like this, given that Reid Hoffman was board of OpenAI, they know the compute for, uh, for GPT 4. [01:42:12] Alex Volkov: So like, I think it's very, um, Very open minded that they're like 40 percent of the amount and they're approaching DPD for performance. Um, they, they also added, uh, real time web search capabilities and actually Nous and stuff works. Somebody mentioned that Cloud has something like this and I don't know if Cloud has any web capabilities. [01:42:33] Alex Volkov: Have you guys seen that Cloud has the ability to search the web? I don't think they do. I don't [01:42:39] Nisten: not sure. I think I heard something about it was able to, but I'm, it, I'm 50% [01:42:45] Alex Volkov: It does not, right? Right. Yeah. I think that like, this is just a mistake. I didn't see any like web capabilities, nor the announcement said anything, but PI goes and like does real time web search, which is pretty cool. And then, um, one thing they mentioned is average conversation with PI lasts 33 minutes and one in 10 lasts over an hour each day. [01:43:04] Alex Volkov: Um, and they have like around 60%, uh, week over week retention, which is, The numbers are crazy. There are one million daily active users, which I don't think they mentioned before. One million daily active is quite impressive. Um, GPT 4 has a hundred million or so? I don't know if daily active, but definitely in the ballpark of this insanity. [01:43:26] Alex Volkov: So, um, And so I went and tried Pi, and I have this video that you're more than welcome to check out. Um, and, uh, It feels kind of the same. So it doesn't want to do tasks, which is the one thing that like when I was talking about Pi before and say, Hey, this model is pretty great. And people told me like, Hey, yeah, I went to ask it to code for me and it didn't. [01:43:50] Alex Volkov: And it looks like like Pi's emotional kind of aspect. It wants to talk to you and wants you to talk to it. It doesn't want to do tasks. And its refusals are very, very funny. Um, so it's very interesting that the numbers they compare it to GPT 4 and the previous inflection are on the, you know, the tasks that we all know and love, like MMLU and Hungarian math, for example. [01:44:14] Alex Volkov: But then when you actually ask the model to do these things, the model just refuses. Um, go ahead Far El. [01:44:20] Far El: Yeah. I just wanna throw [01:44:21] Nisten: in that, uh, Mustafa is the D [01:44:23] Far El: cell safest dor, so, uh, beware. Yeah, [01:44:27] Alex Volkov: I, I was waiting for your addition to this, to this, uh, to this topic, because I know, [01:44:33] Far El: his book, so [01:44:36] Alex Volkov: yeah, um. So, so one thing, one thing that, uh, yeah, moving on, uh, I think, I think it's important to say that like where Mustafa specifically stands on the topic of safety. So thanks Far El. Uh, I would not, uh, um, uh, how should I say, would phrase it exactly like you did. [01:44:52] Alex Volkov: And I don't think I'm, I'm blocked, but, um, I think one thing to call out for the better is that they actually open source something, which is, which is very surprising. Uh, they evaluated on EmptyBench and, uh, they say widely used community leaderboard to compare models. And then they said a real realized a large fraction, nearly 25 percent of examples in the reasoning math and coding had incorrect reference solutions or questions with flawed premises. [01:45:17] Alex Volkov: Therefore, we corrected these examples and released the version of the dataset here. So they, they released the new, the open sourced. Today, or yesterday, EmptyBenchInf, uh, which is a new version of EmptyBench, which they claim is, is, um, higher quality with cleaned references. Which is, um, which is dope, and it's good to see open sourcing from these companies, they do a lot of effort. [01:45:38] Alex Volkov: They have, no matter how their views on, on acceleration, deceleration, they have very smart folks working them, because they hired a bunch of folks. Yeah, Far El, have you, have you looked at the, um, EmptyBench stuff? [01:45:51] Far El: No, but I am definitely aware, uh, and a lot of people have mentioned this previously, all benchmarks, uh, all benchmark datasets have a lot of, uh, like errors and, uh, and, uh, there's a lot of low hanging fruits there to tackle. Um, so yeah, like, I appreciate the, uh, the, this gift that they give to the open source community, but as we know now, based on the OpenAI, uh, letter, uh, that, uh, open source is, uh, mostly just the talent recruitment strategy for all these big labs. [01:46:25] Far El: So, uh, although we're thankful, but, uh, you know, now we're, now we're very much conscious of, um, of the intentions. [01:46:33] Alex Volkov: Yeah, we should cover this. So let me just, uh, lend the, this last thing on, on, on Pi and then we're going to cover this and then we'll see if any, like, exciting news are happening because Twitter is, uh, yeah. So on this new EmptyBench, the corrected EmptyBench, the funny thing is, uh, GPT 4 and regular EmptyBench, has 9. [01:46:51] Alex Volkov: 02, and then the corrected one takes down GPT 4 to 8. 7 something, and inflection actually rises in score to come closer, like, from 8. 4 to 8. 6. So it's really funny how, like, a corrected also, like, boosts their numbers and then takes down GPT 4 numbers. So I think that's mostly it. I personally find it, uh, fun to talk with Pi. [01:47:12] Alex Volkov: Uh, from a perspective, just like talking to an LLM, it doesn't feel like, uh, you know, it's like a clean, uh, Wikipedia based agent like GPT 4 does. Um, however, they evaluated this, this is the funny thing, they evaluated this on a bunch of Coding things, and then it refuses, absolutely refuses to do any coding whatsoever. [01:47:31] Alex Volkov: Um, maybe the new version refuses less, I haven't actually tried it. Uh, but, um, but this was very funny to me that it's not it's purpose and that's not how it feels like. And that's why everybody kind of like, doesn't like Pi, besides the Mustafa personal feelings. Alright folks, I think we have, uh, 5 or 10 more minutes to talk about the gossipy stuff. [01:47:51] Alex Volkov: Uh, let's talk about the gossipy stuff, so So just before this, I want to cover, uh, that, uh, in the Weights Biases corner this week, our inaugural, uh, meetup, uh, conference comes up in, in, uh, April. So if you're using Weights Biases and you're a user, uh, we have our conference, it's called Fully Connected, uh, that you're all invited to participate, to join. [01:48:15] Alex Volkov: It's in San Francisco on April 1st. 18th. Um, and I'm going to post the tweet [01:48:20] in the newsletter and the, the podcast. Definitely. We're going to talk about this as well. Uh, we're going to do some very interesting things there to be announced very soon as well. So I'm going to be there. Uh, if you are into building models, there's going to be a ton of folks who are also doing this on enterprises and, um, and open source as well. [01:48:36] Alex Volkov: So more than welcome to join the tickets are not that crazy, uh, compared to other conferences and. It's a good chance to join San Francisco and check out what else is happening around this week. Obviously, our conference is the most important one. Um, with that, let's move on to some of the gossipy stuff with Elon and OpenAI. [01:48:55] Alex Volkov: Because I think we all saw this, right? Like, we all saw, I think on Friday, there was an announcement that Elon Musk is suing OpenAI and OpenAI Inc. and OpenAI LLC Inc. And like, all of these, like, subsidiaries of OpenAI that they had a bunch of names with. Um. And, uh, Elon being Elon, and we're on his platform, so just like being mindful of this, uh, wants them to change the name to ClosedAI. [01:49:21] Alex Volkov: I don't know if you guys saw this in one of the comments, it's like, Hey, if you change the name to ClosedAI, I will drop the lawsuit. Um, so I'm clear what's behind this and what's the purpose. Um, Very close, you know, a lot of speculation, and we don't have like tons of time to the speculation as well, but like, it's very close to the, to the announcement that, um, OpenAI just announced like a day before the lawsuit, uh, dropped that they're collaborating with FIGR on embodying, uh, in, in, uh, humanoid robots as well. [01:49:51] Alex Volkov: So some people claim that potentially this is gonna, uh, coming into, uh, uh, the Optimus territory. Um. Any, what did he want, folks? I actually didn't read the whole lawsuit and I don't remember. LDJ, do you remember, like, what, what's the outcome that he expects from this lawsuit? Yeah, [01:50:11] Nisten: he actually specifically mentions the rumors of things like QSTAR and, mentions the fact that GPT 4 already scores like, you know, um, around as good or better than an average human in a lot of general reasoning benchmarks and things like that. And he's pretty much calling for, like, he wants them to open source things and, or reimburse him and potentially other investors that might have been involved in open AI before it kind of. [01:50:36] Nisten: Change this company structure. Um, but did you, did you ever read the blog post and the OpenAI I responded with? [01:50:43] Alex Volkov: so now, now we're gonna, I first wanted to cover kind of what he came up with. Uh, I think it was a breach of contract, which contract is unclear, but like, um, there wasn't like a very one contract. [01:50:53] Nisten: exchanges specifically where he included in the original lawsuit the email exchanges of, like, that he felt kind of were on his side and kind of established that, like, this is what they kind of promised verbally in a verbal agreement that this is what they would do. That's kind of what he put out in the original lawsuit. [01:51:11] Alex Volkov: Yeah, and then, uh, Far El, you want to comment on the, on Elon's lawsuit before we get to the OpenAI's response? [01:51:19] Far El: Yeah, it's just, uh, it's mostly just egos, uh, battling, right? Um, uh, there, there could be strategic, uh, uh, like there could be something strategic that comes out of it where they get discovery into the company, uh, into what, uh, like OpenAI is working on or anything. But in reality, like, uh, I think this is just drama. [01:51:41] Far El: Like we, we're not going to see anything really shake up this, uh, this industry, uh, Uh, like OpenAI is not going to change its name to CloseAI, that's, that's just a, that's just Elon Troll. [01:51:54] Alex Volkov: Yeah, that's, that's like pure, pure Elon Troll, that's for [01:51:56] Far El: but, but, but the, the, the most interesting thing that comes out of all of this is, um, uh, all the emails and exchanges between the, like, Sam, Ilya, Elon, and so on. [01:52:10] Far El: And, and, and it, it sheds a light on on a lot of the, uh, the last, you know, six years of OpenAI, uh, strategy. Uh, so, yeah, I think that's where I'm most interested in is all these, like, leaks of information of, um, private information, uh, within [01:52:29] Nisten: the company. [01:52:30] Alex Volkov: Yeah, so let's talk about this. So OpenAI responded in a blog post and said, OpenAI and Elon Musk, and then said, We're sad to see something like a person who we admire come and say this. And they have They have a bunch of emails there, uh, which they embedded in the webpage. It wasn't like a screenshot or anything. [01:52:48] Alex Volkov: They actually embedded the emails in the webpage. Uh, and they, uh, censored them with a paired word sense, uh, uh, censoring, which many folks found, uh, very interesting as a choice because that's not how you censor stuff, because people can actually run machine learning models on this and figure out what was potentially being censored there. [01:53:07] Alex Volkov: Um, and uh, they specifically mentioned that, um. Kind of in response to everything that Elon Musk said, that when they opened it, uh, they initially planned to raise a hundred million dollars. Um, and uh, can you guys hear me by the way? Just a mic check, uh, LDJ Far El. Yeah, okay, uh, so just Nisten then. Um, so they originally planned to raise 100 said we need to go much bigger than 100 million to avoid sounding hopeless. [01:53:36] Alex Volkov: I think we should say that we're starting with 1 billion funding commitment and I'll cover whatever everything else doesn't provide and then they talk about, uh, that they recognize that the for profit entity would be necessary. and they actually show emails that, you know, Ilya Satskover, Ilya, who we all wait to see if he's okay, and where is he? [01:53:57] Alex Volkov: He is actually signed on this response from March 5th, so, you know, I don't think they would add his name without his being there and being okay with what's released. Um, there is an email back and says, hey, You know, that we, when we say open, we mean open that we release these products. Because, just as a reminder, this was like 2015, 2018 emails. [01:54:19] Alex Volkov: Back then, there was no LLMs for us to use, and the only player in the space was DeepMind. And they didn't release anything, uh, necessarily. And so, uh, this was way before, kind of, the product started releasing. And they specifically mentioned the DeepMind and Google as the alternative to what they opened, OpenAI. [01:54:38] Alex Volkov: And specifically to their case, one email here said that, you know, we will have, the non profit arm will not make enough money to be able to break a difference. Google has 800 billion dollar company. I think it's way more than this now. And they have all these like TPUs and we need a significant, uh, significantly more in our war chest to be able to do this. [01:54:59] Alex Volkov: Uh, and then I think, uh, there is a very specific thing where they say, um, as we get AI, it will make sense to start being less open. This is an email from Ilya to Elon Musk. The open in OpenAI means that everyone should benefit from the fruits of AI. But it's totally okay to not share science. Um, and then in parentheses, this is the part that Far El doesn't agree with. [01:55:23] Alex Volkov: Even though sharing everything is definitely the right strategy in the short and possibly medium term for recruitment purposes. Um, and then Elon Musk replies with, yup. So, uh, based on This email, it does seem, at least, that unless he didn't read the email correctly, that he agreed with the strategy of going for close and for profit, which seems that his original claim right now is kind of dubious. [01:55:49] Alex Volkov: And that's their strong response to the lawsuit as well. But, like Far El said, we did learn a bunch of stuff as well here. LDJ, go ahead. [01:55:58] Nisten: I think what's really funny about the situation is that, uh, I guess like the tools they used for to redact the sensitive information, people are actually able to start like trying to decipher what is in the redactions because the tool that they use does like, it's, it's, um, like you can kind of analyze like [01:56:19] Alex Volkov: per word reduction, yeah. [01:56:21] Nisten: um, on a word. [01:56:22] Alex Volkov: On a [01:56:23] Nisten: a word basis, based on the length of the word, and then you can kind of decipher, like, what is the length of each word underneath the redaction? And then, like, yeah, people are starting to, like, decipher that and then be able to tell that it was most likely Andrej Koparthy that was, uh, in the from section of certain emails that's [01:56:40] redacted. [01:56:41] Alex Volkov: Oh, I got some breaking news folks just now from Technium. I'm going to use the button because we're about to close, uh, but I just got breaking news. Uh, so I'm going to use this and we're going to briefly just tell you about this, uh, even though it didn't have any, any, anything to do with it, I just have to use this once. [01:57:06] Alex Volkov: Alright, our folks at Nous are releasing a new 7b model called Genstruct. Uh, instruction generating model designed to create valid instructions given a raw text corpus. Uh, this enables creation of new partially synthetic instruction fine tuning datasets for any raw text corpus, which is super cool. Um, Inspired by the paper AdaInstruct, uh, they took the approach further by grounding the generations in user provided context passages. [01:57:30] Alex Volkov: Uh, the model is now available on Hug Face, and there's a notebook as well. It's called GenStruct from Nous. Super, super cool. Uh, LDJ, comment on this already? [01:57:41] Nisten: Um, I haven't commented on this, but yeah, it's, it looks cool. Um, it looks like it's like. Better than using like RAG and ADA instruct for a lot of things and yeah, I guess that people will probably start using this to build out data sets and things like that. [01:57:56] Alex Volkov: That's very, very awesome. And they have a table here. I'm not sure what it means. They have open models, grounded generation, complex questions, and complex responses as rows. And then they compare RAG and other instructs and future prompting for generation. So if I'm not mistaken, this is for Four generating synthetic fine tuning datasets. [01:58:18] Alex Volkov: Something that people, uh, you know, sometimes use GPT 4 for this purpose, but it's not commercially viable because it goes against OpenAI's, uh, um, terms and conditions. So if I'm not mistaken, this is its purpose, correct? Um, very interesting, [01:58:35] Nisten: specifically for generating instructions, because you can have like, it's actually interesting to think about where things like GPT 4 and all these like specifically. Like, uh, I guess you can call it instruction fine tuned models, like they're trained to specifically take in an instruction and generate a response to that, or take in a question and generate a response to that. [01:58:58] Nisten: But this is kind of like flipped, where it's actually really useful to have something that's specifically trained to generate really good questions and really good instructions in the first place. Because then you can generate these very complex instructions and questions that you could later ask Cloud3 or GPT 4 and then you have even better question and response pairs at the end than if you just used, sorry, than if you just used Cloud3 alone to generate the instructions. [01:59:27] Alex Volkov: Awesome. So, yeah, a little bit of breaking news in the open source as well. Uh, Jen struck from, from, uh, from our folks at Nous. So, folks, we've been at this for two hours. Uh, no other huge breaking news has broken since. And I, it doesn't feel like, uh, you know, those rumors are coming true. If they are, we're probably going to do, like, an emergency space and hop back in. [01:59:48] Alex Volkov: Uh, but until then, I just want to thank everybody for being here for the last two hours. I'm going to do a recap of everything we talked about. If you joined us in the middle and you want to hear everything we've talked about, uh, please stick around for the next eight to 10 minutes. And then I'm going to let you go for the rest of the Thursday. [02:00:04] Alex Volkov: And, um, it's been, it's been a great, great space, even though I was, uh, A little bit sick and coughing at you, hopefully, but very thankful for the co hosts here who picked up some of this conversation. So, we're going to do a brief recap of everything we talked about and then I'm going to let you go. [02:00:36] Alex Volkov: Here's everything we've talked about on ThursdAI, March 7th in 2024, the first ThursdAI in March this year. Um We started with talking about open source. There's not a lot of stuff to, to cover in open source. So we did have a breaking news, uh, from, from folks at News. But before that, we've covered that. Oh one AI open sourced, uh, a smaller version of Yee, uh, which we previously covered as E 34 B was a very important model. [02:01:04] Alex Volkov: Uh, there raised a 9 billion parameter e model that seems very performative compared to seven B. Uh, we discussed how it's very interesting that this category now. is around between 7 billion parameters and almost up to 11. We've talked about a new way to train 70 billion parameters at home with home GPUs from folks from Answer. [02:01:24] Alex Volkov: ai called, uh, you know, uh, Jeremy Howard and, uh, John Whitaker and Tim Ditmers from Qlora joined them and they've combined, uh, combined forces to, to show how it's possible to train, uh, a 70 billion parameter model at home. Um, we also covered Galore, which is kind of a similar. G A L O R E, um, a similar technique to train LexModel models on one single GPU with limited RAM as well. [02:01:51] Alex Volkov: And obviously the breaking news that we just had in this area, that Nous Research released GenStruct7B, a model that's an instruction generation model designed to create valid instructions giving raw text corpus. We literally just covered this as well. Um, we've talked about, uh, some few more open source stuff from the folks who joined us on stage. [02:02:11] Alex Volkov: So, we had, uh, Jan Pellegr, a frequent co host of, of the pod, that talked about, uh, his final attempt at, at, uh, continued training Gemini, oh, sorry, Gemma, uh, the open source or open weights model that Google gave us on top of a bunch of Hebrew text. And, uh, he talked about struggles of how to actually fine tune Gemma. [02:02:32] Alex Volkov: So if that's interesting to you, this will be in the show notes. And, uh, Yam has, uh, a deep dive into how to train Gemma. And we also had Vic Huntak, a friend of the pod, who released Moondream 2, which is a very tiny 1. parameter, uh, vision language model. that you can run on CPU. You don't even have to run a GPU for it. [02:02:53] Alex Volkov: And, uh, Vic talked to us about the fact that this model is now commercially, uh, licensed because he, he trained the captions differently and it's significantly improved benchmark scores and instruction fine tuning. And this model is like very tiny. So if you need a vision model, uh, Moondream 2 is your, uh, is a good bet for you as well. [02:03:14] Alex Volkov: We, uh, we went and talked at length at the, the, the biggest news of this week, which is Cloud, uh, Entropic releasing Cloud 3 and with three versions, Opus, Sonnet, and Haiku. And we, we covered its coding capabilities, its longer context, we've covered that it's multi modal right now. Uh, the one thing we didn't cover, and I'll just mention, is that they claim there is also a function, uh, uh, calling, and that's coming soon, so that's not still available. [02:03:40] Alex Volkov: We saw that it's, uh, it's UI is now comparable to ChatGPT and costs also 20 an hour, uh, 20 a month and it's not available in a bunch of countries, but the API, uh, is available. So if you do want to try this Opus model, um, which is not available for free, you have to actually sign up to either the API or the UI, you can do it via the. [02:04:03] Alex Volkov: via their playground, which they call console. anthropic. com. So we've covered how this model now is improving significantly what previously was known as kind of the fallback from JGPT. Longer context, uh, they claim that they will support up to 1 million context window. Um, As well, and we've talked at length about different ways in which cloud is less restricted than chat GPT, uh, or GPT 4. [02:04:28] Alex Volkov: It feels a little bit more, um, easier to talk to and less refusals, though we did cover some refusals as well. We then talked about the lawsuit that Elon Musk brought to OpenAI, uh, where he claims that he didn't invest in it to become closed. And, uh, facetiously said that if they change their name to CloseDAI, he will drop the lawsuit, because he's being a troll. [02:04:51] Alex Volkov: Basically, uh, but, uh, he did co found OpenDAI, there's a bunch of images and videos recently that popped up, and he also surfaced a bunch of [02:05:00] emails. Excuse me. He also surfaced a bunch of emails in which the co founding happened. And we covered OpenAI's response where they also released a bunch of emails, uh, back and forth. [02:05:12] Alex Volkov: And, uh, obviously in his lawsuit, the emails kind of were in the favor of the lawsuit. And the response, the emails, uh, favored the response in OpenAI. And they show that, uh, at least In one email exchange, they did discuss about going closed and, uh, specifically around the open and open AI does not mean open source everything. [02:05:33] Alex Volkov: Uh, this was Ilya's Satskiverse take. Um, the open and open AI means releasing these models to, uh, to, to people to actually use. And a reminder is that back when those emails were exchanged, there was no other AI that people can use. Uh, this was Google did not release Gemini back then. There was nothing from DeepMind that you can actually use. [02:05:53] Alex Volkov: So just a very important piece of context there. Um, we didn't unfortunately get to, but I'll cover this anyway. There was a charge that, um, Google employee was charged with trading AI secrets with China. And that's a very interesting conversation. We didn't get to, uh, unfortunately, but it's been, it's been talked about that how these large Big AI companies, uh, as a competition with China as a, um, in context with like open source and not open sourcing that people say that anyway, they probably already, uh, nation states has been already intervened there. [02:06:26] Alex Volkov: So it's very interesting that in this context, there's now a previous Google employee that was uploading. Uh, information into his Google Drive, and now he was arrested, and um, um, We also, we also talked about inflection. It was kind of our breaking news today, this morning inflection from Mustafa Suleimani, DeepMind's one of the co founders. [02:06:45] Alex Volkov: There is an upgrade to their underlying agent inflection 2. 5. Uh, there is, uh, an update to PI and PI. Now they claim to be GPT 4 Gemini equivalent or very, very close to while using 40 percent less resources or 40 percent of the resources, I guess, uh, of GPT 4 training. And that model is now available on the used web search is available for this model. [02:07:08] Alex Volkov: It's not multimodal still, but they claim it's coming very, very close. Um, I think that's pretty much it was covered. I will just cover two other things that we didn't get to from Stability. Stable Diffusion, Stability AI released Stable Diffusion 3 research paper, and the model is coming any day now. And based on the research paper alone, it's significantly outperforming Mid Journey and Ideagram, and basically Playground. [02:07:34] Alex Volkov: Every other, uh, uh, Open and closed source image generation models, which is very interesting based on some testing that they did internally. And so, um, they're moving towards diffusion transformers as well. Something that we saw in Sora and we've had, uh, Tanish from the Hourglass diffusion transformers paper, talk to us about diffusion transformers. [02:07:54] Alex Volkov: Uh, so it looks like, uh, the, the industry is converging towards diffusion transformers. Uh, and kind of the two different sides from this industry are converging into, into one architecture, which is interesting. Um. So Stable Diffusion Tree is not available yet, but probably based on what Iman Mustaq, the CEO, said. [02:08:11] Alex Volkov: It's probably going to start sending invites today and is going to be available in their kind of membership. I'm not sure about OpenWeights or not. And StabilityFusion was, uh, StabilityAI was also in the news because they released, uh, together with Tripo, they released, uh, Tripo SR, which is a fast image to 3D, Uh, Generation, and we actually have a demo and a play with this a little bit. [02:08:33] Alex Volkov: And it's really, really cool. You just like upload one image and within like a few steps you get a 3D version that looks very interesting. And there was a demo flying around with somebody just doing this thing where they just use Vision Pro and have a bunch of windows open and they generate an image in one window, drag and drop it, and generate a 3D image of this in another window. [02:08:54] Alex Volkov: Take the 3D and drop it in another thing to actually put it in their space. And I thought it was super cool and actually suggested that somebody combines all these things. So I think that's mostly everything we've covered this week on March 7th outside of Cloud, there hasn't been a huge explosion of news as we're used to, but I think it's still incredible news. [02:09:14] Alex Volkov: We also did have breaking news in the middle, LDJ saw that LMSIS, the arena folks that measure, based on human preference, which models are which is now placing Cloud Opus as a third. And then even Sonnet, the kind of the lower model is also placed fairly, fairly high as well in there. So go play with these models. [02:09:34] Alex Volkov: And I think that's most of what we covered for ThursdAI this week. With that, I thank you for joining folks. It's been, it's been great folks for joining just now to us. I just covered the last two hours that we had on the space and, um, we will see you, we'll see you next time. Um, I don't think we have breaking news, I don't think there's anything, uh, that's worth sticking around to discuss, but with that, everything that we've talked about, all the links are gonna be in the show notes and in the newsletter. [02:10:00] Alex Volkov: If you haven't signed up yet, please definitely feel free to do so on ThursdAI. news. Uh, thank you everyone for joining, Nisten, Far El, Luigi, uh, actually joined, and Ray as well, and we had, we had Jan before, and some other folks as well, thank you everybody in the audience who comes back. Uh, from week to week to listen to us. [02:10:16] Alex Volkov: And I will just remind you that next week is ThursdAI's birthday. We actually started this a year ago and it's been kind of crazy. I think we missed only one. So even though I was sick today, we didn't miss this one. Next week is going to be really fun. Hopefully with GPD 5 news. All right. I'll see you, see you everyone next Thursday. [02:10:33] Alex Volkov: Bye bye. This is a public episode. If you'd like to discuss this with other subscribers or get access to bonus episodes, visit sub.thursdai.news/subscribe | |||
15 Mar 2024 | 🎂 ThursdAI BirthdAI March 14: Anthropic Haiku, Devin the new AI SWE, GPT4 gets hands, Cohere and Nous give us tool use models & more AI news | 01:58:04 | |
"...Happy birthday dear ThursdAIiiiiiiii, happy birthday to youuuuuu 🎂" What a day! Today is π-day (March 14th), 2024. For some reason it's important, not only because it's GPT-4 anniversary, or Claude 1 anniversary, or even that Starship flew to space, but also 🥁 it's ThursdAI BirthdAI 🎉 Yeah, you heard that right, last year following GPT-4 release, I hopped into a twitter space with a few friends, and started chatting about AI, and while some friends came and went, I never stopped, in fact, I decided to leave my 15 year career in software, and focus on AI, learning publicly, sharing my learnings with as many people as possible and it's been glorious. And so today, I get to celebrate a little 💃 I also get to reminisce about the state of AI that we were at, back exactly a year ago. Context windows were tiny, GPT-4 came out with 8K (we casually now have models with 200K that cost $0.25/1M tokens), GPT-4 also showed unprecedented levels vision capabilities back then, and now, we have 1.3B parameters models that have similar level of visual understanding, open source was nascent (in fact, LLama.cpp only had it's first commit 4 days prior to GPT4 launch, Stanford released the first Alpaca finetune of Llama just a day prior. Hell even the chatGPT API only came out a few days before, so there was barely any products built with AI out there. Not to mention that folks were only starting to figure out what vector DBs were, what RAG is, how to prompt, and that it's possible to run these things in a loop and create agents! Other fields evolved as well, just hit play on this song I generated for ThursdAI with Suno V3 alpha, I can’t stop listening to it and imagining that this was NOT possible even a few months ago It's all so crazy and happening so fast, that annual moments like these propose a great opportunity to pause the acceleration for a sec. and contextualize it, and bask in the techno-optimism glory of aren't we lucky to live in these times? I sure am, and for me it's the ThursdAI birthday gift to be able to share my excitement with all of you! Thank you for being a subscriber, the best way you can support ThursdAI is to share this with a friend and tag us on socials 🫡 TL;DR of all topics covered: * Open Source LLMs * Together releases Sequoia speculative decoding (X, Blog) * Hermes Pro from NousResearch - Tool use and function calling (X, HF, Github) * Big CO LLMs + APIs * Anthropic releases Claude 3 Haiku (Announcement, Blog) * Cohere CMD+R (Announcement, HF) * This weeks Buzz * Early bird tickets for Fully Connected in SF are flying, come meet the Weights & Biases team. We're also going to be running a workshop a day before, come join us! (X) * Vision & Video * Deepseek VLM 1.3B and 7B (X,Announcement, HF) * Voice & Audio * Made a song with Suno v3 Alpha for ThursdAI, it's a banger (Song) * Hardware & Robotics (New) * OpenAI now powers Figure - the humanoid robot company (X) * Cerebras announces the fastest AI chip on earth (X) * Extropic made an announcement about their TPU - Thermodynamic Processing Unit * Tools & Agents * Devin from Cognition Labs (Announcement, 47 minute demo) Agents for your house and your Github tasks Say hello to Devin from Cognition Labs (Announcement, Real world demo) By far the most excited I've seen my X feed be this week, was excitement about Cognition Labs new agent called Devin, which they call the first AI software engineer. You should really watch the video, and then watch a few other videos, because, well, only a few folks are getting access, and yours truly is not one of them. It seems like a very published launch, backed by tons of VC folks, and everybody kept highlighting not only the innovative UI that Devin has, and it has a very polished UX/UI/Dev experience with access to a browser (where you can authenticate and it can pick up doing tasks), terminal (where you can scroll back and forth in time to see what it did when), but also a chat window and a planning window + an IDE where it rights code and you can scrub through that as well. Folks were also going crazy about the founder (and team) amount of math ability and IOI gold medals, this video went viral featuring Scott the founder of Cognition, in his youth obliterating this competition… poor Victoria 😅 Regardless of their incredible math abilities, Devin is actually pretty solid, specifically from the UI side, and again, like with he AutoGPT hype of yesteryear, we see the same issues, it's nice, but cognition hiring page is still looking for human software engineers. Tune into the last 30 minutes of the pod today as we had tons of folks discuss the implications of an AI "software engineer" and whether or not coding skills are still required/desired. Short answer is, yes, don't skip, learn coding. Devin is going to be there to assist but likely will not replace you. 🤖 OpenAI + Figure give GPT-4 hands (or give figure eyes/ears/mouth) Ok this demo you must just see before reading the rest of it, OpenAI announced a partnership with Figure, a humanoid robotics company recently, and just this week they released a demo of this integration. Using GPT4-Vision and Text to speech capabilities (with a new, somewhat raspy voice and human like intonations), the bot listens to the human giving it instructions, sees the world in front of it, and is able to perform tasks that the human has asked it to do via voice. This feels like a significant jump in capabilities for these bots, and while it was a given that the two technologies (Actuator based robotics and LLMs) will meet soon , this shows the first I Robot like moment. It'll still be a while until you can have this one do your dishes or fold your laundry, but it does feel like it's an eventuality at this point, where as before, it just felt like sci-fi. Kudos on this integration, and can't wait until Optimus from Tesla will add Grok brains and it'll make you laugh nervously at it's cringe jokes 😅 This weeks Buzz We're coming to SF in April, our annual Fully Connected conference will feature keynote speakers from foundational AI companies, industry, our founders and tons of Weights & Biases users. We'll also be running a workshop (I'm one of the workshop folks) a day before, so keep an eye on that, it'll be likely included in your ticket (which is still, 50% off for early bird) Open Source LLMs Nous Research gives us Tool Use with Hermes 2 Pro (Announcement) Getting json structured output and giving models the ability to respond with not only text, but specific instructions for which functions to run (aka tool use) is paramount for developers. OpenAI first released this back in June, and since then I've been waiting for Open Source to catch up. And catch up they did, with Nous releasing their first attempt at continued training of the renown Hermes 7B Mistral based model, with tool use and structured output! If you're building agents, or any type of RAG system with additional tools, you will definitely be very happy as well, give Hermes Pro a try! This one is not a simple download and run, you have to do some coding, and luckily the folks at Nous provided us with plenty of examples in their Github. Deepseek gives us a new Vision model - Deepseek VL 1.3B & 7B (Announcement) Absolutely punching above it's weight, this very high quality vision model from the Deepseek folks is just a sign of what's coming, smaller models, performing incredibly better on several tasks. While the top is getting crowded with Claude, GPT4-V and Gemini which are generic, on specific tasks, we're getting tiny models that can offload fully into memory and run hell fast and perform very well on narrow tasks, even in the browser Big CO LLMs + APIs Anthropic gives the smallest/fastest/cheapest Claude 3 - Haiku After releasing Opus and Sonnet earlier, Anthropic has reclaimed their throne as the leading AI lab we always knew them to be. Many friends of the pod prefer Opus for many things now, and I keep seeing this sentiment online, folks are even considering cancelling chatGPT for the first time since... well ever? While sonnet, their middle model is taking a significant interesting place on top of the LMsys arena human rated rankings Beating all GPT-4 besides the Turbo ones. And now Anthropics has given us Haiku, the smallest of the three Claudes, the fastest, and the cheapest by far. With 200K context window, vision capabilities, this model crushes GPT3.5 on many benchmarks and becomes the de-facto cheapest model to run. It only costs $0.25/1M tokens, which is twice cheaper than GPT3.5 but just look at the performance. One thing to note, Anthropic still doesn't support function calling/tool use. Cohere releases a new model for retrieval and enterprise purposes - CMD+R Cohere goes for the second wind with a great release + open weights approach, and release Command+R (pronounced Commander) which is a model focused on enterprise uses, scalability and tool use. It supports 10 languages, 128K context and beats GPT3.5 and Gemini 1.0 on several tasks, namely on KILT - Knowledge Intensive Language Tasks. The tool use capabilities and the ability to ground information in retrieved context makes this specifically a great model to use for RAG purposes. The model is 34B and is available non commercially on the hub Together makes inference go BRRR with Sequoia, a new speculative decoding method Together Sequoia shows a way to speed up Llama2-70B and be able to run this on a single consumer GPU with 8x speed up. Being able to run AI locally can mean a few things, it can mean, make smaller models better, and we've seen this again and again for the past year. Another way is... speculative decoding. Being able to lower the inference TBT (time between tokens) by enhancing algorithms of decoding and using tiny draft models, and methods like offloading. The large model essentially remains the same, while a smaller (draft) model can help guide the inference and make it seem much faster. These methods compound, and while Sequoia from Together is new, shows great promise by enhancing the inference time LLama2 70B 8x on consumer hardware and up to 3-4x on dedicated hardware. The compounding of these methods is the most exciting part to me, given that they will likely apply broadly (for now Sequoia only supports LLaMa) once a new model / architecture comes out. — Show notes: * Swyx AI news newsletter got a shoutout from Andrej Karpathy * Anthropic metaprompt cookbook from Alex Albert * Folks who participated in the AI Agent discussion, Nisten, Roie Cohen, Junaid Dawud, Anton Osika, Rohan Pandey, Ryan Carson Thank you for being a subscriber, and for sharing this journey with me, I hope you enjoy both the newsletter format and the podcast 🫡 See you here next week 🎂 I’m going to eat a piece of cake Full transcript : [00:00:00] Alex Volkov: Hey, you are on Thursday. I March 14th, 2024. AKA bye. Day AKA Thursday. I birthday. I, I'm sorry for the pun. Uh, I promise I'm gonna, I'm gonna keep it contained as much as I can. My name is Alex Volkov I'm an AI evangelist with weights and biases Today on the show, a birthday celebration for Thursday I Twitter spaces. [00:00:31] Alex Volkov: That's right. I started recording these exactly a year ago on GPT 4's announcement day, March 14th, 2023. In addition, everything important that happened in the world of AI for the past week that sometimes feels like a year. Including open source LLMs, big companies and their APIs, hardware and robotics for the first time, agents, And more. [00:00:59] Alex Volkov: We've talked about a lot of stuff. But first, as always, a recap of everything we discussed as I recorded it at the end of the show while everything was fresh in my mind after this little birthday song that AI created for us. [00:01:12] [00:02:39] Alex Volkov: that this is AI generated? Maybe at the end there it went a little bit off, but holy cow, this is, I really listened to this birthday celebration multiple times after I created it with Suno V3 Alpha. So get ready for AI music everywhere. And now, the recap of everything we talked about for this week. [00:03:02] Alex Volkov: But definitely, Stick around and listen to the end of the show. And as always, you will have chapters on every podcast platform that you use, especially Apple Podcasts. [00:03:13] Alex Volkov: And if you do use Apple Podcasts, why not? Give us a thumbs up and like a five star review. That really helps. That's how people discover us, believe it or not. Here's a recap of everything we talked about. And following that, a beautiful in depth conversation with many folks who shared this journey with me and been, in one way or another, the reason I kept going this year for ThursdAI. [00:03:36] TL;DR - everything we talked about in 10 minutes [00:03:36] Alex Volkov: Everyone, here's a recap of everything we've talked about on Thursdays. Anniversary for Twitterspaces, March 14th, 2024, which is also Pi Day, which is also the anniversary of ChatGPT4, and anniversary of Cloud1, and we spoke about ThursdAI history, we spoke about how we got here, how now it's a podcast. [00:03:56] Alex Volkov: And in open source, we had together AI release something called speculative decoding Sequoia. Speculative decoding is not new, but their approach to speculative decoding called Sequoia is new. It is able to Optimize inference for something like a Lama 70 billion parameter on consumer hardware up to 8 to 9 percent faster by just, , predicting a tree of next tokens and letting the model select between them. [00:04:20] Alex Volkov: Speculative decoding is an additive technique to improve speed of inference of models. On top of models getting smaller and better, the bigger models are going to get faster on local hardware as well due to something like speculative decoding. It's very exciting to see. TogetherAI also announced like an extension of the round and now they're a unicorn and Definitely doing incredible things. [00:04:40] Alex Volkov: We also, in the open source, we've covered that our friends at Nous Research, [00:04:44] Alex Volkov: hermes Pro. If you followed us at any point before, you know that Hermes is one of the top Finetunes for Mistral 7 billion parameters. There is the Pro version of Mistral 7 billion on top of Hermes dataset. Hermes dataset also, by the way, is open and you can go and download and use it. This version, the pro version, is specifically focused on tool use and function calling. [00:05:07] Alex Volkov: And we also covered what tool use is from the perspective of developers who build RAG apps, for example, or need structured output. This new version supports JSON mode and JSON output, which is a very welcome addition to the world of open source. [00:05:19] Alex Volkov: It has OpenAI endpoint compatibility, and it's hosted on Fireworks, so you can actually try it out and just swap the OpenAI endpoint with that endpoint and see if your tasks are working with Hermes as well. [00:05:31] Alex Volkov: On the border between open source LLMs and big company LLMs. We then moved to a conversation about Cohere. Cohere is a company that was co founded by one of the authors of the original Transformers paper Aiden Gomez and some other folks. Incredible company mostly focused on enterprise and use cases around RAG retrieval augmented generation. [00:05:50] Alex Volkov: Cohere had a bunch of models called Reranker and Embedding Models. And now they released something called Command R. And by release, I mean they released it via API, but also they dropped it on Hug and Face in OpenWeight's non commercial license. So you'd be able to actually run and use this locally but you cannot use it commercially yet. [00:06:06] Alex Volkov: For that, they offer their API and their API is definitely there. It performs very well on RAG application, outperforms other scalable models. So Outperforms, like even Mixtral and Lama70B, they're not comparing themselves to GPT 4 because this model the command R model is definitely focused on enterprise and use cases. [00:06:25] Alex Volkov: It works very well with their cohere embedding and re rank models in tandem as well, it's focused on tool use. Like previously we said that Technium just added to open source. They're focused on tool use and external tools as well. And their Cohere API has a bunch of external tools that you can plug in into this one, like web search, like stock prices, like a bunch of other things. [00:06:45] Alex Volkov: Optimized for 10 major languages, which is usually way more than other open models, and trained on 13 more, and has 128k context window. [00:06:55] Alex Volkov: And in the same area of smaller models, we finally got the small model, Answer from Tropic, the folks that just released Claude three Claude three. [00:07:06] Alex Volkov: Antropic released the smallest, most performant version of Claude 3 called Haiku. They call it the fastest, most affordable yet model for enterprise applications. [00:07:15] Alex Volkov: Cloud3 Haiku is 25 cents per million input tokens, where GPT 3. 5, which is considered the cheapest one and the most performant one so far, is half a dollar for a million tokens. So it's half the price of GPT 3. 5. However, it significantly overperforms GPT 3. 5 on any other token. every metric that they've added, including human eval, which is 75 percent versus GPT 3. [00:07:39] Alex Volkov: 5's 48%. MMLU score is 75. And the kicker here is as 200k context window, like the major Cloud Opus and Cloud Sonnet. So Heiko has 200k context window. Imagine a model that is only 25 cents per million tokens on input. has also 200k contacts window. And it's available via the API, obviously. or Amazon and Google Cloud as well. And it's vision enabled, so you can actually send images. And we geeked out about how a year ago when we started ThursdAI, one of the reasons why we came to the space, because we were blown away by GPT 4's vision capabilities. [00:08:14] Alex Volkov: And now we're getting I'm not gonna say that Haiku is anywhere close to GPT 4 vision [00:08:20] wise, but it's From what I've tested very decent, given the price point, it's incredibly decent. Then I covered that in the Weights and Biases area we're coming to San Francisco in April 18th is our fully connected conference with many big clients of ours coming, foundational model creators, et cetera, coming to speak on the stage. [00:08:40] Alex Volkov: And we're also going to do a workshop, , a day before. So April 17th, if you're interested in this, please write to me, I'll definitely. tell you when that's up. The tickets are early bird and you're more than welcome to join us in San Francisco. We will be very happy to see you. [00:08:53] Alex Volkov: If you came from ThursdAI, come and give me a high five. I would love to, to, show my boss that this is actually pulling some folks. But also we covered continued things in ThursdAI around vision and video. So skipping from Weights and Biases stuff, we covered vision and video. [00:09:06] Alex Volkov: We covered the DeepSeq, released a DeepSeq VLM, which is a tiny vision model. So Again, in the realm of multimodality this year, we're now getting 1. 3 billion parameter and 7 billion parameter models that on some tasks come close to GPT 4. It's quite incredible. So DeepSeq the folks who released DeepSeq Coder before and very impressive lineup of models open sourced VLM 1. [00:09:30] Alex Volkov: 3 billion and 7 billion. Incredible, impressive on benchmarks, and the 1. 3 billion parameter model is so tiny, you can run this basically offloaded on your CPU. And in that vein, we also covered briefly, but we did cover that Transformers. js is very soon, from our friend Zenova, is very soon to support WebGPU. [00:09:47] Alex Volkov: WebGPU is the ability to run these models in your browser in your JavaScript environment on the GPU of your machine, either that's a Mac or a PC. And that's now landed fully in all major browsers right now. [00:10:00] Alex Volkov: The song that you heard beginning over this was made with suno v3. Alpha and I did this specifically for ThursdAI. And I'm very impressed that a year after we started all this, we're now getting songs that sound like somebody actually went in the studio and sang it. We then mentioned that in the AI art and diffusion corner, we still don't have stable diffusion tree. [00:10:20] Alex Volkov: We also had another corner today, which is a hardware and robotics corner. And we've covered several very exciting things. [00:10:28] Alex Volkov: We've covered that Cerebrus announced the fastest AI chip on Earth, with 4 trillion transistors and 900, 000 AI cores, able to train up to 24, 000 people. I don't use the word trillion parameters a lot here, but able to train 24 trillion parameters models on a single device. This sounds incredible, and once they put it in production, I think it's going to be a significant boost to the AI scene. [00:10:52] Alex Volkov: We also covered Xtropic, the folks that came from Google X, Secret Lab, now announce Xtropic, the folks behind the EAC movement. As well, that's their company, they're building a TPU, Thermodynamic Processing Unit it's a little complex, but basically. They want to do natural physical embodiment of probabilistic learning, and they want to be considered the transistor of the AI era. [00:11:17] Alex Volkov: And if you want to hear more about this, they have the full space Q& A that we'll link in the comments below. And so we covered Cerebrus, we covered Extropic in the hardware, and then we've talked about how FIGR, the humanoid robot company FIGR we covered before they, they announced a partnership with OpenAI, and this week they released a demo video that's unedited, so end to end recorded in 1x speed, of this, Figure robot, humanoid robot standing in something that looks like a fake kitchen and basically talks to the human in front of it using OpenAI's text to speech technology and vision. [00:11:52] Alex Volkov: So it actually understands what it sees based on GPT 4 vision, probably custom version of GPT 4 vision, and also then is able to do some stuff. If you haven't seen this video, I'm going to put it in show notes on thursdai. news. Please feel free to subscribe. The video is mind blowing, [00:12:07] Alex Volkov: but just the fact that the robot can see, talk about what it sees, and then perform tasks embodied in the real world, I think is a great way to see the future happening right now on Pi Day 2024. And I think this is most of the conversation that we've covered from the news perspective, besides this one last thing, where we covered that Cognition Labs released a video and actually started Letting folks in to something they call Devon, the first fully autonomous AI software engineer. [00:12:35] Alex Volkov: That's the tagline. And obviously we've those of us who covered this, we remember AutoGPT hype from last year. We remember multiple since then, multiple different agentic frameworks, Devon seems like it took that to the next level, not only from a perspective of just being able to execute long tasks, but also from the ability of the UI to show you what it does and being autonomous alongside your software engineer. [00:12:59] Alex Volkov: So you can, Devon actually has access to a full environment, probably with GPUs as well. It has access to a browser that you can log into your stuff and then Devon can on your behalf, use the browser and go and search for some stuff. [00:13:10] Alex Volkov: And we had. One hell of a discussion following the Devon news to talk about, and I think it was started by Nisten saying, Hey folks, you have nothing to fear, still learn code. That this news, again, stoked fears of folks saying, Hey, should I even learn to code given these advancements? And we had a great discussion about Coding, taking over coders, for example, replacing or not replacing, and positivity in the age of AI. [00:13:34] Alex Volkov: And this discussion, I really suggest you listen, stick to the end of the podcast, if you're listening on the podcast, and listen to the whole discussion, because I think it was a great discussion. [00:13:43] Alex Volkov: Hey everyone. My name is Alex Volkov. I'm the host of ThursdAI for the past year, which I can now say proudly, and I just want to welcome you, yet again, to another Thursday. Today's a big day, not only because we're celebrating, but also because some of us woke up early to see the largest man made object ever to break through the atmosphere and go to space, which was incredible. [00:14:24] Alex Volkov: Very tech optimist like, but also today is an anniversary of multiple things. And I think ThursdAI is just one of them. So we're gonna, we're gonna actually talk about this real quick. And I just want to say that ThursdAI I'm very happy to still be here a year after with many people who joined from week to week, from month to month, whatever friendships that were shaped in the ThursdAI community. [00:14:49] Alex Volkov: And I just want to say I'm very happy that Swyx here is here. Swyx was on the actual first ThursdAI episode a year ago. We jumped in to discuss GPT 4 and I think we're blown away by the vision stuff. So welcome Swyx. How are you? Thanks for waking up early for this. [00:15:04] Swyx: Hey morning. Yeah, it's a big day. The year has felt like 10 years, but it it's definitely a big day to celebrate. [00:15:10] Alex Volkov: Absolutely. So thanks for joining us. Swyx, for folks who don't follow for some reason, definitely give Swyx a follow, a host of Latentspace and the founder of Small. And recently is being followed by SpaceDaddy as well. And I want to say also [00:15:24] Swyx: Space Daddy! [00:15:25] Alex Volkov: And I want to also say hi to Nisten who's been maybe the most consistent co host, Nisten. [00:15:30] Alex Volkov: Nisten, welcome joining us all the way from called Canada, I think after visiting the doctor, how are you Nisten? [00:15:38] Nisten: I'm good. I'm good. It's good. I missed one, I [00:15:42] Alex Volkov: Yeah. . Yes. [00:15:43] Nisten: was about it. I thought I was gonna miss the day, and I was upset, but no, I [00:15:48] Alex Volkov: I have a question for you. Was the doctor that you visited a human doctor or an AI doctor? [00:15:53] Nisten: Yeah, he was human. He hadn't seen me in five years, so I was showing him all this stuff about medicine and the AI. It's funny. [00:16:00] Alex Volkov: The, and I also wanna acknowledge Farouk or Pharrell as we call him, maybe Pharrell, how are you? I. [00:16:07] Nisten: Hey, what's up? [00:16:09] Alex Volkov: Welcome, welcome to the ThursdAI celebration Far El is leading the Skunksworks crew and has been doing different incredible things in the open source. Very staunch proponent of open source here on the ThursdAI stage. If anything gets released and it doesn't get released with the source Far El will have words to say about this. [00:16:25] Alex Volkov: So we're going to cover open source today as well. I also want to acknowledge the LDJ. Yesterday I wrote the whole thread and acknowledged like many people and I didn't tag. My, my good friend, Luigi. So LDJ, apologies for that. Welcome brother. How are you doing all the way from Florida?[00:16:40] [00:16:41] LDJ: Yeah, I'm doing good, thanks. I've been late to a lot of the Thursday AIs past few months, but yeah, it's been good coming on and glad I was able to make it on time for this one. [00:16:51] Alex Volkov: Yeah welcome. Welcome. And I also want to acknowledge Roei ray is the DevX Dev Advocates on Pinecone and Ray has been participating in many spaces. We had a lot of conversation about reg versus long context. And I remember those wells, a lot of like late night conversations as well. Welcome Ray. [00:17:06] Alex Volkov: How are you? [00:17:08] Roei Cohen: How's it going, everybody? Congrats, Alex, on this awesome anniversary. Yeah, [00:17:16] Alex Volkov: there's a bunch of folks I see in the audience who are here from week to week, and it's so great to see the community shape up, and I really couldn't be prouder to be able to just talk about AI with friends and actually make a living out of this. [00:17:29] Alex Volkov: I would be amiss if I don't acknowledge that the anniversary today is from The spaces. So we started talking about AI in Twitter spaces, back then Twitter spaces, now X spaces exactly a year ago or Pi Day 2023. The reason why we started talking about AI is because GVT 4 was announced and Greg Brockman gave the incredible demo where he took a screenshot of a Discord. [00:17:52] Alex Volkov: So if you remember this, the Discord, the famous Discord where we went to hunt the Discord Snapchat. Mhm. [00:18:00] Swyx: a screenshot of the, I think the OpenAI Discord and it just transcribed every word in there and described every, like the position of every icon and like the framing of it. It was just like the best vision model we'd ever seen by like by a lot. [00:18:14] Alex Volkov: By a significant margin and it understood different like active states, etc. and to get to a point now where we're basically having open source models. We're going to talk about CogVLM today. We're going to talk about DeepSeq released a new vision model today to get the, to the point where we can basically recreate this with a tiny model that runs completely offloaded, it's crazy. [00:18:36] Alex Volkov: Back then, no vision existed. So we got into space, started geeking out about this, and then we kept going. So this is the anniversary of the Twitter Spaces, the actual podcast, the ThursdAI podcast that I created. Encourage you to subscribe to didn't start about four or five months afterwards. [00:18:51] Alex Volkov: After we did this and the community started shaping up and people started coming in and actual guests started to arrive. So I see a few guests that became friends of the pod. So if you guys see Jun Yang here in the audience, on, on the technical team at Quen, there's a great conversation that we had about Quen and their models as well. [00:19:10] Alex Volkov: We have a bunch of folks like this from time to time, just join and talk about the stuff they built. And I think this is the best thing that I get from ThursdAI is definitely this is the ability to talk with folks who are experts in their fields. And definitely I'm not an expert in many of the things we cover. [00:19:25] Alex Volkov: And it's great to have folks from vision and from foundational model training and from open source. And we had a bunch of conversation with Nous Research folks. We're going to cover a few of those today as well, and it has been incredible so far. And so the birthday for the actual podcast, once we started recording and sending a newsletter is coming up in. [00:19:44] Alex Volkov: in June. Meanwhile, if you want to support the space, if you're here and you're like, Oh, this is great. I learned so much. You're more than welcome to just interact with us. On the bottom right, there's like a little icon there, the message icon that says five. You're more than welcome to just send replies there and boost a little bit of the signal and retweet the space link. [00:20:02] Alex Volkov: And so I think with this, I think with this, Oh no, a year ago, another thing was and it went out the radar because GPT 4 took over. All over the airwaves. Cloud One was released exactly a year ago as well. Happy anniversary to the Cloud team. They've been killing it lately. The past few weeks have been entropic weeks for sure. [00:20:20] Alex Volkov: And definitely folks are looking at Cloud and now, considering cancelling their JGPT subscription. So that's been great to see. And so a year ago, there is Cloud One and they were quickly quickly hidden with the news. I also want to shout out that in the past year as well. Open source were almost non existent. [00:20:36] Alex Volkov: So a year ago and four days, Lama CPP was first released. Georgi Gerganov released Lama. cpp, a way to run the Lama model that was released a month before that on just, your local hardware. And, uh, nobody knew about this necessarily until a few days later. Vicuna was just released. [00:20:56] Alex Volkov: So if you guys remember Rikuna, that was a thing. So all of these things happened in, in that week. And it feels this week we have, or at least the last few weeks, we have a similar like Insanity weeks. Don't you guys think? Especially with Opus and the rumors about GPT 4. [00:21:11] Alex Volkov: Do you guys remember anything else from that last week before we started like talking about this week? [00:21:15] Far El: It's hard to remember what happened last week because this week felt like a century alone. That's that, that's the thing. Like we, we've [00:21:22] Nisten: had so much just in the last week that I don't even remember what happened. [00:21:25] Alex Volkov: Absolutely. That's why we write down. And honestly, I think Swyx, we talked about this where now that, every ThursdAI is now recapped and you have AI News, Newsletter Daily, or that covers everything. This is just for the historical record, it's very important. Just to be able to go a year back and see where we were. [00:21:41] Alex Volkov: Because it's really hard to remember even last week, not to mention the last year. So I think it's very important. I don't want to shout out do you still call this small talk? Or do you have AI News? [00:21:50] Far El: It's just AI news. I'm reserving small talk for the other products that I'm working [00:21:55] Alex Volkov: I see. [00:21:56] Far El: yeah. Yeah. AI news's, [00:21:57] Alex Volkov: so talk to us about the AI news just briefly for folks who are not familiar with that specific newsletter. [00:22:02] Swyx: Man, this week was f*****g, it was crazy in around December I was like very overwhelmed by all the AI discords and I knew that all the alphas being dropped in discords are no longer on Twitter, so I started making this bot to scrape discords and it was mostly just serving myself and then I shared it with some friends and it grew to like a couple hundred people, but one of them was Sumit Chintala from the Meta team, like he was the creator of PyTorch and still runs PyTorch. [00:22:31] Swyx: And last week he shouted it out, saying that he he said it was like the highest leverage 45 minutes every day that he spends reading this thing. Which was like a freaking huge endorsement from someone like him. So I didn't even know he [00:22:43] Alex Volkov: from from the guy who runs PyTorch. It's crazy. And of [00:22:49] Swyx: so I, yeah, I didn't even know he was subscribed. I don't, honestly, I don't even look at the subscriber newsletter. I think it's really good for mental health to just do your thing, right? Don't even look at who's on the list. And then two days ago, Andre also just like unsolicited, completely no notice, no warning just said oh, yeah, I've been reading this thing for a while. [00:23:06] Swyx: And I was like, what? And then I went back and looked through the emails and like his email's not there. There's no, his first name not there, not there. I eventually found his email, but yeah, it's it was just a shock that like he was also getting utility out of it and Yeah, so far I think like 12, 13, 000 people signed up in the past couple days, and we'll see where this, we'll see where this goes I think a newsletter is not the last final form, and also people have legitimate concerns around, how much is comfortable being scraped from Discord what is the sort of privacy expectation on a public Discord that anyone can join, right? [00:23:39] Swyx: So I'm taking some steps to basically protect people it's purely meant for utility, not for snooping on people's conversations. But I do think like there should be a new sort of Hacker News of AI, quote unquote, that pulls together, Local Llama, Twitter, Discord, YouTube, podcasts, whatever. [00:23:55] Swyx: And yeah, I think that's what I'm making AI News go towards. [00:24:02] Alex Volkov: is excited about, Elon is excited about as well. So Elon now is a follower of Latentspace, which is a big moment. I wanted to ask [00:24:08] Swyx: Yeah, we're trying to, yeah, let's [00:24:09] Alex Volkov: Local Llama, by the way? Is Local Llama part of the source as well [00:24:13] Swyx: we the engineer that I'm working with is working on this. So not yet, but we are working on it. And [00:24:19] Alex Volkov: Alright folks, so if you want so if you want not only HighSignal, but if you want like the full firehose of information, that's great from Discord and Twitter list, I think you have a HighSignal Twitter list as well in there definitely subscribe to AI News previously Smalltalk, as like the titans of the industry now follow this and getting insight from this, so you should as well. [00:24:40] Alex Volkov: But yeah. If that's too much for you, we're here every week to cover pretty much the very most important things. [00:24:46] Open source - Function Calling model from NousResearch - Hermes Pro [00:24:46] Alex Volkov: And so I think it's time for us to start with Open Source.[00:25:00] [00:25:09] Alex Volkov: Alright folks, so let's let's cover some open source stuff. I think the first thing is we have to mention that our folks our friends from Nous Research announcing a new model today or I guess yesterday night. It's called Hermes Pro. Hermes Pro is specifically, I'm not really sure what Pro means here, so we have to ask some folks from Nous Research, but they announced the continued training of their Mistral model, their flagship model, that uses, that is fine tuned for tool use and function calling. [00:25:40] Alex Volkov: And tool use and function calling are Maybe or should I say synonyms of each other at this point? I think it started with function calling from OpenAI that was released in June last year. And they gave us function calling in response of all of us wanting a JSON output. And since then, function calling became something called tool use. [00:25:59] Alex Volkov: Basically, the ability of these models to not only So you the next word or complete, autocomplete, but also you could provide schemas for some of your functions that these models will say, Hey, I'm actually, I want to, I want more information on this topic or that topic. And so here is what tool you should use. [00:26:20] Alex Volkov: And you as a developer, you would get that response. You would go call this tool. you would then pass back the data from this tool into the model. And then the model will use its context and the user's request together to come up with an answer. So think about stock price, right? Stock price is something that changes often. [00:26:37] Alex Volkov: You cannot train the model on stock price because it changes very often. So for one example of a tool could be go check the stocks on the stock market or go check Bitcoin price, et cetera. And the model, Mistral is not able to, it's very obvious if you ask a Mistral 7b, Hey, what's the price of Bitcoin? [00:26:55] Alex Volkov: It will give you something that something will be 100 percent wrong, a hallucination. So a model with tool use would be able to decide that if you provided, if a developer provided in advance, The the model with tools like, hey, price of Bitcoin, price of stock, et cetera the model will be able to decide that instead of hallucinating the answer, you'd actually return a reply to the developer and say, hey, go get me this information and then I'll be able to answer the user, right? [00:27:20] Alex Volkov: So this is what tool use and function calling basically is. And we haven't had a lot of that in open source. We had a little bit. We've talked about the tool use leaderboard from the folks at Gorilla. I think. Stanford? I'm not sure. And then now Nous Research released us a continued training of their 7B model called Hermes Pro with the same general capabilities. [00:27:39] Alex Volkov: So that's also very important, right? You keep training a model. You don't want something called catastrophic forgetting. You want the model to perform the same plus additional things as well. And the, now it's trained on new data with tool use plus JSON mode as well. So not only do we get The ability of the model to reply back and say, Hey, you should use this function. [00:28:00] Alex Volkov: We also get JSON mode as well. It supports custom Pydentic schema. Pydentic for folks who don't write in Python is a way to define objects in, in, in Python in a very clear way. And when you use this and you give the model kind of the schema for your, tool use. The model then knows what parameters to call your functions with. [00:28:18] Alex Volkov: So your job as a developer is basically just take this call and forward it to any API call that you want. It's available on the hub and it's announced with OpenAI endpoint compatibility, which is great. So I don't think we've seen this from Hermes so far directly. I think everybody who served Nous models they gave us OpenAI compatibility, but definitely we know that The industry is coalescing around the same format, which is the OpenAI endpoint, where you can just replace the URL to either OpenRouter or Fireworks or whatever. [00:28:49] Alex Volkov: I think the chat from Mistral as well is supporting OpenAI compatibility. Great to see that we're getting open source models for tool use because it's very important for agents and it's very important for basically building, building on top of these LLMs. LDJ, I saw you wave your hand a little bit. [00:29:07] Alex Volkov: Did you have a chance to look at Hermes Pro in tool use? And what are your general thoughts about open source tool use? Hey, [00:29:16] LDJ: I'm pretty much Hermes, but also has a much improved JSON and function calling abilities and things like that. And I was just waving my hand to describe that, but then you pretty much described it already. So I put my hand back down. [00:29:29] LDJ: but [00:29:30] LDJ: Yeah, you got a good description of it. [00:29:32] LDJ: And I think that pretty much summarizes it. [00:29:34] Alex Volkov: this is the anniversary of this ThursdAI, Birthday AI. So I did my homework this time. Usually sometimes these things get released like super fast and we actually don't have time to prepare. Comments on general availability of function calling and tool use from the stage before we move on? [00:29:48] Alex Volkov: Anything that you guys want to shout out specifically that's interesting here? [00:29:50] Nisten: It's probably the most commercial used part, I think, because every person that's using a 7b, they want a really fast model, and usually they want some kind of JSON returned for commercial uses. There are chat uses as well, but I think like the majority of, I don't have any data on this, I'm just guessing that probably the majority of the use is to return JSON. [00:30:15] Alex Volkov: Yeah. And then there, there are tools like Pydantic from Jason Liu that we've talked about that help you extract like structured data from some of these. And those tools require function calling as well. And function calling and Pydantic support. So definitely supports more enterprise y. [00:30:29] Alex Volkov: Maybe that's why Technium decided to call this Hermes Pro. [00:30:32] Together.ai new speculative decoding Sequoia improves AI inference by 9x [00:30:32] Alex Volkov: Moving on to Together and Sequoia. And Together released something called Sequoia, which is speculative decoding. I actually wrote down explanation of speculative decoding is, and I'm going to try to run through this. So for folks who are not familiar with speculative decoding, basically, if you think about how we get open source, how we get bigger and better AI on to run locally on our machines, one of them is open source and smaller models getting better, right? [00:30:58] Alex Volkov: So that's definitely something we've seen for the past year. We got Llama70B and then we got. 13B, and then different Finetunes and different other foundational models. I started beating the LLAMA 70B, definitely LLAMA 1, and now even LLAMA 2 is getting beaten by tinier models. So the progress of throwing more compute and more techniques is shrinking down these models to us being able to run them locally, just because our hardware is, let's say, limited. [00:31:23] Alex Volkov: That's one way that we get local open source models. They just get. keep improving and keep getting trained on. Another way is we can, we're able to serve these like bigger, larger models, like 70B models on consumer GPUs, but then it's like super slow. So you wait one minute or two minutes between each token prediction or each word that you see. [00:31:44] Alex Volkov: So one additional way on top of just getting smaller models faster and smarter is improving inference. So we see, we saw a bunch of attempts this year from folks like Modular releasing their max inference system and improvements obviously in different like places like FlashIntention and different inference engines as well. [00:32:03] Alex Volkov: So we saw all of this and one such way that adds to all of this is called speculative decoding, which improves the inference speed, just inference speed. It basically tries to predict a few next tokens instead of just one, using a smaller model. And the key idea is to construct a tree of speculated future tokens for every potential token in the model's output. [00:32:26] Alex Volkov: Sometimes they use, I think at least LLAMA CPP supports speculative decoding, sometimes they use a smaller model. For example, for LLAMA, they could use like a small LLAMA to help you predict the tokens, and then the larger LLAMA to actually help select them. And And together, folks who released a few things that we've covered before employe3d out there, who released the the Mamba architecture and the Hyena architecture we've talked about previously before also FlashAttention chief they now released their own take on speculative decoding. [00:32:56] Alex Volkov: which they claim that on consumer GPUs, you'd be able to run something up to a 70 billion parameter Lama 2 with a RTX 4090. And they improve the ability of you to run this incredible like large model by almost nine, nine percent, nine x faster. On, non consumer GPUs like A100s, they also go up to 4x faster. [00:33:17] Alex Volkov: Basically, by just predicting with a [00:33:20] smaller model, all like building a tree of all possible tokens, and then the larger model actually selects and predicts somehow based on those. They have a bunch of other things like offloading there, and very interesting things, but I just want to say that this is a field, speculative coding is a field that is entirely. [00:33:39] Alex Volkov: How should I say? They only support LLAMA as far as I saw, but this whole field is entirely additive to the rest of the fields, right? So if speculative coding helps to improve LLAMA, 7TB9x faster, it's probably going to work on smaller models as well. So it's really incredible to see how much different speed improvements we're getting across the board. [00:33:58] Alex Volkov: And definitely for the stuff that we all, we're working I love to talk about, which is open source models running locally, running faster. This is incredible. Yeah, LDJ, go ahead. [00:34:09] Nisten: Yeah, I just wanted to direct people to I put, I pinned on the billboard a video that TogetherAI put out actually showing side by side Sequoia versus not Sequoia, and yeah, it's pretty insane the amount of speed up you're able to get. [00:34:21] Alex Volkov: The amount of speed up on the same hardware and on the same model. So the model didn't improve, the hardware didn't improve. All they improved is the ability to to help the model predict next tokens and spit them out, which is, I agree with you, it's insane. And just, um, multiple improvements across the board are going to get us where basically we want to go, which is these type of models, these sizes of models running super fast on, on local hardware. [00:34:44] Alex Volkov: They released it in GitHub. Folks can try. It only works for LLAMA. It doesn't work for like any other bigger models as well. Definitely we'll see. I will just say that the thing that I'm most excited about this is that all these techniques are, one, additive, and two they're there for the next big model to get released and just support them. [00:35:00] Alex Volkov: So like when LLAMA 3 came out, eventually releases, and we know it will release these models will be able sorry, this speculative decoding will start working, and then speculative decoding will start working, LLAMA CPP will already be there. So we saw the kind of, the community efforts to support everything were just kicked into gear when GEMMA was released. [00:35:18] Alex Volkov: I'm just very excited that we have all these techniques to Throw the, throw at kind of the next open source, the big model open source. And just the concept of running a 70 billion parameter model is very exciting. Last week we covered something from Jeremy Howard and Joanna Whittaker and Team Dietmers, the folks with Answer. [00:35:36] Alex Volkov: ai and Qlora combined Qlora with another technique to be able to train 70 billion parameters or at least fine tune them on kind of consumer hardware as well. We're not only getting news for the past week and two weeks of being able to fine tune 70 billion parameter models on consumer ish hardware. [00:35:53] Alex Volkov: We're also getting news about being able to run them with some, uh, some logical number of tokens per second and not a one token every like four minutes or something. Exciting news in open source. [00:36:04] DeepSeek VLM 1.3 & 7B VLM that punches above its weight [00:36:04] Alex Volkov: Maybe we'll cover DeepSeq VLM here as it's vision, but yeah, definitely released in open source and we don't want to miss. [00:36:10] Alex Volkov: So DeepSeq, the folks behind The folks behind DeepSeq Coder and released DeepSeq VL, a state of the art 1. 3 billion and 7 billion visual parameter models. If you guys remember last week, we talked to Vic Hyntak of Moondream2, and that was a tiny vision model. And the whole point, and if you were here in the beginning, when we in Swyx got excited about a year ago about the vision capabilities of GPT 4. [00:36:34] Alex Volkov: The whole point with these vision models is that their improvement this year definitely felt exponential, because now a model of 1. 3 billion parameters, a tiny model that most Macs can now run, can very easily. And if our friend Zenova is going to join us, it's very soon with WebGPU, we're going to be able to run fully in browser. [00:36:53] Alex Volkov: These models now are able to perform very similarly to what we saw a year ago and just blew our minds, which is OCR. without an OCR model built in, understanding objects, understanding graphs and charts, etc. And so it's very interesting that DeepSeq coder, let me try to share this into the space. Yeah, it should be up there as well. [00:37:13] Alex Volkov: Very interesting that DeepSeq released and is punching above its weight. significantly above its weight, and they actually try to compare themselves to GPT 4 vision, which is quite remarkable on different tasks like evaluation, multi images and then in some of these tasks, they get to half performance of GPT 4 vision, which is still quite incredible, right? [00:37:35] Alex Volkov: Like it's a 7 billion parameter model, GPT 4, we still don't know how many parameters this is. We still don't know if GPT 4 vision is, a multi mixture of expert model or not. But DeepSeaCoder is actually. Coming close to the same performance as DBT four on Common Sense task and analysis task. [00:37:55] Nisten: Yeah, and I just want to say Allama CBP supports these models. I don't know about DeepSeq, but they've supported all the other ones. And there's also a a Lava CLI in there, which you can use with these ones. Also, when you run the server, you can run the models as well. [00:38:12] Nisten: I think they just need a little bit more compute and engineering, and they can match GPT 4 when it comes to vision. I am quite surprised that it wasn't that That big of a deal. In some ways, COG VLM, not DeepSeq, is a lot better than the rest, but it's also a larger model too. And I quickly wanted to say, because you mentioned Zenova before, I don't know if you're going to go more into that, but it turns out, it's that people of the core Chrome team or Chrome Canary that implement WebGPU, they listen to they listen to ThursdAI and Stuff that we've been saying over the years, over the months, they've actually started implementing. [00:39:00] WebGPU and Int8 support for quantized models [00:39:00] Nisten: And the most exciting thing that I find now is that they are trying to implement int8 support natively in WebGPU. So that will do another savings of half the memory when When you run stuff, even if you have a GPU that doesn't necessarily support in Tate, I think there was a method to run at half the memory. [00:39:23] Nisten: So remember we went from only supporting Float 32, a few months back, I think it was September. And you needed a weird started version of Canary with a few commands to support Float16. So now they're supporting Inti, so the memory requirements of the browser have dropped down by 4x in the last 5, 6 months. [00:39:44] Alex Volkov: I remember the days before WebGPU support even landed, like all of Transformers. js. And for folks who are not following us that closely, Zenova is a friend of the pod, the author of Transformers. js. We talked a lot on the pod, he actually announced him joining Hug and Face. on the part as well. And he created Transformers. [00:40:04] Alex Volkov: js, which is a way in JavaScript on node to run these models via the ONNX platform. And when we talked about this before, the only way to run these models in the browser was like fully CPU. And then we always talked about, okay, WebGPU is going to come at some point. WebGPU is the ability to run to tap into GPU inference from the browser environment, from the Chrome environment. [00:40:26] Alex Volkov: And since then, WebGPU was Still a spec that was announced that was released and now it's fully supported everywhere. But like Nisten, like you're saying, it only supports 32, right? And a bit, can you describe this part a little bit more? And then now they're listening to us and actually lending support for a quantized version of these models, a smaller version to be able to run even smaller models that perform the same. [00:40:47] Alex Volkov: And, [00:40:47] Nisten: Yeah so now, Chrome, you don't even need Canary and it will support Float Float16. And by default, if you only have a CPU, stuff can run now on the CPU and in float32. But again, the biggest use for this so far has not actually been chatbots. Even though chatbots do work, it has been more the visual stuff and the effects. [00:41:10] Nisten: All the diffusion based some stuff of function calling. That's where stuff gets pretty exciting. because it changes what kind of applications you can build. It's, again, it's the front end, like what are you going to put before they reach this big GPU cluster? So it's pretty, this is the part where we're going to see the most changes and progress, in my opinion. [00:41:34] Nisten: It's going to be the visual stuff, making use of Transformers [00:41:40] JS library. [00:41:40] Alex Volkov: and one, one example of that Zenova showed on his feed is a real time background removal from video. So you play a video and then imagine a Chrome extension that's loaded or something, and then you're able to run AI transformer stuff on top of everything that you read or see that's the kind of stuff we're talking about with access to GPU, I think is going to be possible. [00:42:00] Alex Volkov: So super, super exciting to see how this performs. And obviously this means that the models that we talk about local running locally, we'll just get. More use, because developers will be able to build them in. This will never get to the point of GPT 4 level for full generality, or I don't want to say never, but it's not quite there in terms of okay, running a GPT 4 level model fully in your browser. [00:42:23] Alex Volkov: But for some specific tasks like vision, we just talked about on several benchmarks, Coq VLM and this tiny new release from DeepSeq VLM is now getting there, right? So you'd be able to analyze images, for example, you'd be able to do all kinds of things in the browser fully. Without loading, without Python environments, without all of these things. [00:42:42] Alex Volkov: I think it means a lot for user experience as well. I think we've covered open source a bunch. Do you guys have anything else worth mentioning in the open source thing? Briefly before we move on to the to the big companies, and maybe we'll discuss, we're going to discuss agents as well. [00:42:57] Cohere releases Command+R - a RAG focused model in API + open weights [00:42:57] [00:42:57] Alex Volkov: Yeah, so CommandR, I, interestingly, it's both in the open source and not, so maybe it's a good transition, right? Let's actually do this as a transitional topic. So Cohere, the company that I don't know, raised a bunch of million of dollars and everybody expected it to be like the second Lycanthropic and didn't for a while. [00:43:18] Alex Volkov: Now is back in the very impressively is back. And so for a long time, I think Cohere re Refocus their efforts on something like RAG. They had the Cohere Reranking model and they had the Metting models for a while. And they focused on, I know that we in Weights Biases, we use Cohere Reranker for our RAG bot, and that's improving our responses significantly. [00:43:39] Alex Volkov: Reranking is basically receiving Receiving back from your vector database, a few responses that are near neighbor equivalent to what your user has asked for. And then running another process of re ranking them for higher how should I say accuracy. And so Cohere Reranker was for a long time, like one of the more standard ones that folks use. [00:43:58] Alex Volkov: And now Cohere actually stepped in and said, Hey, we're releasing a new model that's it's called Commander, Command R. It's a new generative model from Cohere aimed at production scale tasks, like RAG, Retrieval Augmented Generation, and using external tools and APIs. So here's this word again, external tools we use and APIs as well. [00:44:16] Alex Volkov: As you as we previously discussed, Tool use is important. We just got tool use, in a fully open source, thanks to Nous Research and I haven't yet tested their tool use, but Cohere is definitely building this model. And I think Swyx, you also saw this release and we're going to both identify the same pretty much thing where this is interestingly not getting compared to any GPT 4 or Cloud Opus, right? [00:44:40] Alex Volkov: They're not even trying. They have a very specific. Use case in mind and I wanted to see from use works if you have any other comments on that or how they're like positioning themselves and what specifically in what world that they're operating in. [00:44:54] Swyx: For Coban, [00:44:55] Alex Volkov: For Commandar and Cohere as general, yeah. [00:44:58] Swyx: simple answer is probably not as good as GPT [00:45:01] Alex Volkov: Yep. [00:45:02] Far El: They didn't include it, but I haven't tried it out personally myself. People seem to be talking about it for retrieval and ragtime use cases, but, I can't give my personal endorsement. Just in general, Cohere, I think, they've been more active in sort of enterprise use cases and Finetuning, like talking about their Finetuning capabilities, or long tail low resource language, maybe use cases they also released AYA, I think, last month, which some people in the open source community were quite excited about but yeah, I think having them see, seeing them do such like a strong launch for a new model I think is like a second win for Cohere, and I'm excited to see more coming out of them. [00:45:43] Alex Volkov: Definitely feels like a second wind, and we would don't know how much we covered Cohere here before but the fact that they released the model also in open weights on Hackenface, I think, gives them a lot of credibility from the community. LDJ, go ahead. [00:45:58] Nisten: Yeah I noticed they did actually post some benchmarks on the website of comparison to LLAMA 270 billion, Mistral, and GPT 3. 5 Turbo, and like all comparisons and RAG benchmarks, and Command R does seem to be all of those three that I just mentioned. And of course, this is their own reporting, it's probably good to wait for third party benchmarks, but yeah, and it's apparently very good at multilingual abilities as well. I think I saw somebody saying that like somebody who, one of their like first languages is Portuguese, like they said Command R was one of the best languages, or one of the best models that was able to do that actually very fluently and understand the nuances of the language. [00:46:39] Nisten: So yeah, I think that's really interesting and it might just be really good overall model for open source. [00:46:45] Alex Volkov: Yeah [00:46:45] Nisten: I think it is open source, but just, sorry it's open source, but I think it's just non commercial license. [00:46:51] Alex Volkov: Yeah, so they did open Waitz release with with non commercial license. And they did say that if you're an enterprise, you want to build something cool with Commandar talk to them and they'll figure out something. And Aiden Gomez the CEO of Cohere is one of the founder, one of the authors on their Attention Is All You Need paper recently has unblocked And became friends with Nisten here in the Toronto community. [00:47:16] Alex Volkov: He mentioned that this model is also optimized for 10 major languages for global like business and trained on 13 more. So it actually has a pre trained on 13 more has 128 context window, right? So if you do compare this to GPT 3. 5 Turbo or Mixtral, for example I don't remember 32k context this is 128k and they specifically focus on speed in addition to everything else, right? [00:47:39] Alex Volkov: And in RAG systems, in, in these systems, you may not need a model that's like super, super fast. Smart, you may need a model that is able to retrieve everything that you want much faster and significant speed improvements may outperform smartness on MMLU tasks, right? So I think that's their game, they're playing it they compare it like, like LDJ said to 3. [00:48:02] Alex Volkov: and not GVT4 or Opus and they have results in something called KILT, Knowledge Intensive Language Tasks and retrieval and Tool use specifically and so they also have a bunch of stuff on their platform to be able to do tool use and by Tool like I explained before go get me some news from the web, for example, so it's really focused on web Integration getting things from the web. [00:48:22] Alex Volkov: Nisten, do you see the one line they posted where like it's basically they said hey, here's perplexity Based on command R. I think you replied to that. Do you remember do you want to cover this briefly? It was really fun as an example [00:48:36] Nisten: Yeah, I shared it in the Jumbotron, it's like the third thing. It looks like it's pretty easy to build a RAG pipeline with their code, but not all of it is open. There are a few things there which are unclear, and I haven't built that pipeline yet to say for sure. So I don't want to say anything that it's incorrect, but it looks like they've made it really easy to build your own perplexity in five lines of code. [00:49:04] Alex Volkov: That was really funny. Like a little dig at perplexity. Definitely the model is able to do like web, like the tool of web search. This model specifically is like Excelsat, but other tools as well. So shout out to Cohere, second win, like Swyx said, definitely we'll keep keep you guys posted when some of us try this. [00:49:21] Alex Volkov: Open weights model that you can. Run, but not commercially, but you can use it and train and maybe this will help open source folks as well. [00:49:29] Anthropic releases Claude Haiku - GPT3.5 competitor [00:49:29] Alex Volkov: Moving on from Cohere I think in the same battlefield, actually Anthropic. gave us an announcement yesterday, and very smart release schedule from Entropic, I must say, right? [00:49:40] Alex Volkov: So they released Cloud 3, they announced Cloud 3 a few weeks ago, they announced three versions, Opus, which is their flagship that now many people prefer on top of GPT 4, which is quite incredible. It's not taking over on LMCS yet. So GPT 4 still takes over on the LMCS people arena. But I think we've [00:50:00] been coming back here week after week and saying that, some more folks use Opus. [00:50:04] Alex Volkov: Um, let me see just by raising hands. Do you guys use Opus for the past week? At least once? What do you have a thumbs up or thumbs down for Opus use? [00:50:13] Swyx: Oh yeah, I use it every day. [00:50:15] Alex Volkov: Every day. Wow. So you got the pro thing or are you using the API kinda? [00:50:20] Far El: I got Pro, but apparently I'm a chump because I don't have to use Pro, like, only like B2C, non developer types should use Pro. Every developer should just use the Anthropic Workbench because you just pay by API call and you're probably using less than 30 worth. [00:50:35] Alex Volkov: I will say this like very quietly because maybe Anthropic you don't even have to pay unless you apply for production use and then you have to put a credit card. It's open and you get API calls for free. I will say this, I will say this, there's a Tony Ding released like a year ago, I think something called TypingMind, which is like a front end for front end for ChatGPT basically, but on the back end you can put every model that you want. [00:50:55] Alex Volkov: So basically you get the ChatGPT experience, including vision stuff. You can upload images as well. And I think that costs like 30 bucks. If you get that. and you plug in your API key that you get from Tropic for free, you basically get the same experience, you don't have to pay the 20 bucks a month [00:51:08] Far El: Do you use TypingMine every day? I I hear some social media buzz about it, but I don't see any AI people. engineer type people [00:51:15] Alex Volkov: I haven't used it up until I, I had to do, I had to try cloud three and I didn't want to pay the extra 20 bucks. Just remember in our subscription. So I just plugged it into typing mind and it's a nice experience. I still go to Workbench. Workbench is more for us, for engineers, right? [00:51:30] Alex Volkov: Workbench, everything that you get there, you can immediately export and continue via the API, for example. And the Workbench is annoying because you have to remember to every Every prompt that you have, every answer that the model gives you, you have to click a button and put it back in kind of the stack of messages, right? [00:51:47] Far El: you can use keyboard shortcuts, but it's also meant for you to prototype prompts, right? So that's what you want to do. You want your conversations not to persist. You want to see the output and you're like, okay, throw away the output. I'll tweak the prompt again, generate the new output. So you don't want it to auto add to the conversation. [00:52:04] Far El: That's the main difference, [00:52:05] Alex Volkov: That's true. And so definitely many folks use the Workbench for prototyping prompts is great, but just for chatting is also great. So you've been using it, so what's your take on Opus so far? [00:52:17] Far El: Oh, yeah. If you go to AI News every day now, I'm Generating Haiku, Opus, and what's the other one? Sonnet. By the way did you know that the names of these things basically hint at the model size? [00:52:30] Alex Volkov: Yeah, let's talk about this. Opus is like a big [00:52:32] Far El: yeah. Haiku is three lines long, sonnet is 14 lines long. Interestingly, and in opus is, unbounded, but in, 3b, 14b, and probably 8 times 220b. Yes. I think the cloud people thought they were very smart by just encoding the, the numbers in [00:52:50] Alex Volkov: gotta applaud them about the name because I stopped saying Cloud3, I'm just saying Opus now, and everybody gets what we're talking about. Opus is a brand name that's built in like separately from Cloud3, which is, I think, very smart. Like I'm, 3. 5, 4, 4Vision, all these things, like it's a little harder to say, and now they came out like with actual names, and I gotta applaud the strategy. [00:53:12] Alex Volkov: I think just to connect the dots back to where we are today yesterday Claude finally released the announced haiku and yeah, Swyx, you had another comment that I spoke over? [00:53:22] Far El: Nothing, I was just going to give say, if you want to do you should be generating things side by side and seeing the model difference. Haiku is very bad at instruction following. Sonnet is actually really surprisingly good enough. I would use Sonnet for most things, and then Opus is more powerful but slow and honestly not really worth it. [00:53:42] Far El: And if you want to see side by side generations, just go in the last few issues of AI News. You'll see side by side and you can decide for yourself which one you prefer. Yeah, so I run all the summaries through through Sonnet and Opus and Haiku every day now, and I can see the difference. [00:53:56] Far El: I would say the general take is that Code 3 in general is better at instruction following and summarization than GPT 4, which is huge. I can't believe I'm just saying [00:54:08] Alex Volkov: It's crazy. [00:54:08] Far El: of GPT 4. But it hallucinates more. They're like very obvious. inconsistencies in like the things that it tries to, the facts that it picks up on, and they're just plain wrong. [00:54:18] Far El: And anyone with any knowledge of the subject matter will see, will spot that immediately. So Sumith, when he was talking about Cloud 3, actually referenced some examples from AI News in his timeline, if you go check out Sumith's timeline on Cloud 3. And yeah, I will say like that is the problem with using Cloud 3, like it. [00:54:35] Far El: It follows instructions very well, but then it will hallucinate things. Maybe because it doesn't have as good of a world model as GPC 4. Whatever it is now I'm having to decide as a product creator, am I using Cloud 3 because it, the vibes are better, but then do I have to build an anti hallucination pipeline, which I'm trying to build, but it's difficult because what is truth? [00:54:56] Alex Volkov: Yes. Are you, let me ask you a question real quick. Let me, one second Nisten, and then Nisten you go Swyx, one question. Did you change your prompt for Cloud specifically from your GPT 4 prompt? [00:55:08] Far El: I copied over some of it and I wrote some other parts from scratch. I understand that a lot of people say you should use XML for this stuff. I think that it's a little bit of mumbo jumbo, especially because I'm not doing structured output. [00:55:22] Alex Volkov: I will say this thing they have Alex Albert, who's now getting more of a highlighted role. He's the guy that we've talked about that, that did the New Zealand Haystack analysis, where Claude Opus realized that it's getting tested, right? So you probably saw this famous tweet. So Alex is the prompt engineer there. [00:55:38] Alex Volkov: He has a a collab that's called a Metaprompt. So you can find it, I'm probably going to put this in show notes, that you basically describe the task that you want, and then Opus comes up with the prompt for Opus itself. And the prompts that it comes up with works for me way better than the prompts that I've written myself. [00:55:54] Alex Volkov: So it does use a little bit of XML. And I just want to say to Diana, it's not necessarily to you, but definitely to you as well some different prompting is needed. So these models do do need different, they've been trained differently. And XML is one part of it, but also, It feels like a little bit of more prompting and folks can't just expect to have the same prompt that works for GPT 4 to work. [00:56:16] Alex Volkov: I think some of our intuition as well changes per model. Some models, like you said, are like more hallucinatively, but following instructions better. Definitely, I saw this. Nisten, I cut you off before. If you still remember where I cut you off please continue. [00:56:29] Nisten: No, it was along the side. So I've used Sonnet and I just opened the Bing sidebar and quickly iterate through stuff with Sonnet. And yeah, I noticed the same thing. It does make up a lot of stuff. So then I need to drop it into Bing in precision mode and have it actually look up. The stuff and Then it's still not quite ideal. [00:56:52] Nisten: But this combination I also use Mistral Large just switching between being with internet mode and either Sonnet or Mistral Large to quickly Iterate through although Mistral Large is slow. So again, I really like the speed of [00:57:09] Far El: Sonnet [00:57:11] Alex Volkov: Yeah, so let's actually pick up on on the kind of the news thing. So we covered like cloud before, and now we're talked about as actually folks putting it in production like Swyx and we're also testing this Entropic Release Haiku, which is their smallest model, and that doesn't compete with any GPT 4, they go for the lowest price and the fastest kind of execution. [00:57:32] Alex Volkov: Fairly similar to, to the command R kind of area of playground that we got, right? It's like focusing on speed, focusing on as best performance as possible for the fastest and the cheapest price possible. And we definitely heard before from multiple folks who fine tuned GPT 3. 5, for example, and get better results than GPT 4 on fine tuned GPT 3. [00:57:51] Alex Volkov: 5 and significantly faster as well. So on tropical risk. Haiku, which is their fastest and most affordable model for enterprise applications. They stress enterprise because every dollar, every token counts, every dollar counts, and you actually get, get to measure these models not only on how good they are, but also how good they are compared to how many, how much money you pay for them and how fast they respond to your users. [00:58:14] Alex Volkov: And the main differences between Haiku and like GPT 3. 5 or even 1. 2. [00:58:20] Zero Pro and Gemini. The main differences is price. It's priced at 25 cents per million tokens, which GPT-3 0.5 is half a dollar per million tokens. So half the price the output tokens are 1.25 dollars per million output tokens, which usually enterprises, they do prompt engineering, so they shove a bunch of stuff in the prompt, but the, the response is not that long. [00:58:43] Alex Volkov: So usually you focus on the input tokens as well. It gets 75 on MMLU and 89 on GSM4K, which is significantly better than GPT 3. 5. Now, they may have used the announced 3. 5 metrics and not the actual metrics, which oftentimes folks do, but still is very important, very impressive. And it does human eval 75%, 70, almost 76. [00:59:09] Alex Volkov: percent on human eval on code, which is quite impressive for a super fast model. But I think the highlight of the differences between a 3. 5 or a Gemini One Pro is that Haiku is vision enabled, right? So you can pass images. It's quite impressively so vision enabled. So whatever we got excited about last year at Swyx, I think now it's possible with like up to 25 seconds per million tokens, which is quite incredible. [00:59:34] Alex Volkov: You can use it everywhere pretty much. It's none, million tokens is a lot. And also it has 200, oh, sorry, go ahead, Svek. [00:59:43] Far El: No, one caveat or question is the vision model in Haiku the same as, same vision model as in Sonnet or Opus, right? Maybe it's dumbed down as well, and so no one's really run, yeah, no one's really run any of the benchmarks on this stuff. [00:59:56] Alex Volkov: Yeah, and then I think it's worth calling out that like now the same level 3. 5 speed and performance, significant like improvement of performance plus vision enabled plus 200, 000 context window as well, which 3. 5 is, I think still is it 8? Yeah. So shout out to to Entropic, sorry, not Cohere, to Entropic to keep bringing us the news. [01:00:17] Alex Volkov: The release schedule was very well timed. They released the biggest biggest two models and then followed up with this fast model. And for folks who are looking for how to use or maybe lower their costs with the same performance. It's very interesting. Anthropic promised us tool use and function calling and haven't yet gave us function calling. [01:00:35] Alex Volkov: They said that these models are able to do function calling and tool use, but we still are not able to use this. For your preferences, you may go here. [01:00:42] Hardware and Robotics [01:00:42] Alex Volkov: Big companies and APIs. I think that's most of what we want to cover, but I think we're smoothly moving towards the next area where we talk about hardware and robotics because one big company joined another big company a few weeks ago, and now it's worth talking about open AI and figure the humanoid robot. [01:01:01] Alex Volkov: Figure has been in the news, they showed the case the robot for a while. Their, I think, main competitor It's funny how Boston Dynamics was the big name in robotics for a while, and now all these companies are leapfrogging Boston Dynamics at some fields. [01:01:16] Figure + OpenAI integration shown on a video [01:01:16] Alex Volkov: So Figure has this humanoid robot, it has , ten fingers, it moves them very freely, it's very interesting. [01:01:22] Alex Volkov: Recently they announced their integration with OpenAI, And I think OpenAI also announced the integration with Figure. And now they released a video, and that video is bonkers. It's really, folks, it's really bonkers. [01:01:31] Alex Volkov: They show the figure robot standing just like in, in some form of a lab. Funnily enough on the back wall, it says AGI lab. And then that figure robot has a little screen on its face. And that screen shows you the same exact interface that you get, you and I get in the chatGPT iOS app. With the little, little circle that turns into a few animated things when you talk to it. And I found it really funny that they insisted on keeping the same kind of UI. And basically this person comes up to this figure and says, Hey, what do you see right now? And the robot uses the onboard cameras to send image. [01:02:07] Alex Volkov: I guess it's one image to GPT 4 vision and replies with, I see literally the robot says, I see you standing in front of me with your hand on the table. So that was like one. one quirk of how the robot knows that the person that talked to it is the person who actually stands in front of it. But I think they stress that this video is end to end, not edited, and it's 1x speed also, and the robot replies fairly fast. [01:02:32] Alex Volkov: Time to speak on OpenAI's GPT 4 is quite fast anyway. If you use the app, you know this, but the vision stuff, maybe they got like some private versions of API. Responses, folks on the stage, do you see this video? What do you think of this? Is the Terminator coming or we're still not there? [01:02:48] Alex Volkov: What are your, some of your responses to this figure video now with OpenAI Brain? [01:02:56] Alex Volkov: Ray, go ahead. [01:02:58] Far El: Yeah, [01:02:59] Roei Cohen: very shortly before that, I listened to Yann LeCun's podcast with with Lex. Yeah, and, what's striking me about that demo was that we're actually quite close. To having usable robots just using the reasoning that is available on OpenAI. And that's, I think that's remarkable. [01:03:20] Roei Cohen: You know what I mean? Because at the end of the day, you're like, oh, these things are not thinking, they're just spinning out Next Tokens and whatnot, but more and more, I feel myself drawn into Ilya's Camp, where you're like, no, there's probably some world model that, that these things have to develop internally, because otherwise they wouldn't be able to accomplish all these tasks that are essentially what you need is some sort of an understanding of embodiment in order to like, Reason about where to move your limbs and how to pick up things and, things of that sort. [01:03:50] Roei Cohen: I don't know. I just thought that there was, like, a really stark contrast between what they showed in the demo and that conversation. More optimistic today than I was before. [01:03:59] Alex Volkov: Absolutely. And I think if there was one additional reason for Space Daddy to sue OpenAI, for Elon to sue is that, Optimus is definitely a bet that Tesla is making now. Tesla's whole like reason for existing was to bring the world renewable energy. And when When Optimus was announced, many kind of investors thought was like, Hey, is this moving the vision a little bit forward? [01:04:19] Alex Volkov: And because, Optimus does not bring the world renewable energy. Optimus is advancing super quick as well. We saw for the past year, multiple demos. The last demo blew me away in terms of like dexterity of different fingers and everything. And then you gotta wonder how smart Optimus will actually be. [01:04:35] Alex Volkov: in terms of the ability of it to perform tasks and respond to you. And FIGURE, FIGURE announced they're like advanced robot and then they announced the integration with OpenAI which we know that Elon is now on the warpath with. And so I got to wonder if, their communication, their integration now that Elon has also Opus and Grok. [01:04:53] Alex Volkov: Given where Grok is right now, and I posted this as a question on my timeline is, would you prefer Optimus, who's like better and more flashier, but with Grok brains, versus a figure with GPT 5 brains or something? And I think it was quite obvious where the distribution lies. [01:05:07] Alex Volkov: You, you would want the less flashy robot that's smarter potentially than the flashy robot that's is GPT 3. 5 level. So the understanding of the scene was very impressive there. The te the text to speech was very natural. I don't know if you guys noticed in this video, but the robot actually ums and s and takes pauses and it feels like they built something like this. [01:05:27] Alex Volkov: I actually, they used probably the same text to speeches, OpenAI, but it feels like OpenAI gave them a little bit of a better model because I use the open the ice text of speech often via the iOS app, and it's, it doesn't it doesn't go you know what, I actually think this right. [01:05:41] Roei Cohen: To be fair, the I've started seeing this behavior in text to speech with PI first. Phi already does this ums and uhs, and a more natural kind of cadence, [01:05:51] Alex Volkov: yeah, Phi is very expensive for sure. LDJ, go ahead. [01:05:55] Nisten: Yeah, I actually use a custom instruction with PadGBT where I specifically, I give it like a set of principles to follow. [01:06:02] Nisten: And the last principle is make sure to include ums and uhs in your speech and as if you're talking. And I feel like when I use the conversational voice mode, it makes it feel a lot more realistic because then it's actually literally saying uhs and ums. And it does end up doing that with me. [01:06:17] Alex Volkov: Yeah, so I definitely noticed this and this could be just a result of of something like a custom instruction or maybe they're using a different model. The voice they use is not one of the voices that OpenAI gives. I think it's a custom voice that they use. It's a little raspy. It's pretty interesting that they gave it something. [01:06:35] Alex Volkov: And Roy, to your point before, where I gotta wonder how deep the integration goes. Do they use just [01:06:40] the API, or did they have a fine tune on top of the ability of this robot to actually perform tasks? [01:06:45] Nisten: See official confirmation of somebody was asking hey, are you guys using GPT 4 and maybe a new model or something? And then the CEO figure, he just cryptically replied saying, we're using some new advanced features, something like [01:06:59] Alex Volkov: Yeah, they're definitely getting advanced features. We know that OpenAI gives advanced features to friends, so Spotify, for example, uses OpenAI voice cloning tech for, converting Lex to Spanish, and we know for a fact that this, they give out this very sparsely, so probably they have more advanced features. [01:07:16] Cerebras announced their largest and fastest AI Chip C3 [01:07:16] Alex Volkov: Alright, in the hardware and robotics things, we want to cover two more things super quick. Cerebrus announced their largest and fastest AI chip on Earth, and So this is a company that, builds custom hardware, and they announced the CS3, which they claim that could technically claim and now all these claims are probably like still in, in flux. [01:07:36] Alex Volkov: And I don't know if the supports any, how should I say, PyTorch, for example, but they claim that can train up to 24 trillion parameter models on a single device. They say the world has never seen AI at this scale and, it's insane. 24 trillion parameters for single devices. It's insane. [01:07:55] Alex Volkov: It has 4 trillion transistors. I can keep saying numbers and I'm not like a numbers guy. So like when people talk numbers at me, they blow past, but 900, 000 AI cores on this chip. And it's very interesting that they have terabytes of external memory, even up to petabytes which is crazy. [01:08:12] Alex Volkov: Anybody who's more into hardware want to comment real quick of what Cerebrus announced and how important this is to the industry. I'm more than welcome. [01:08:20] Nisten: Yeah, the on chip memory which is pretty much equivalent to, cache in a GPU. They have I want to say it's 40 or 50 gigabytes on the CS3, which like, that pretty much means you could, you would be able to train your inference theoretically anything like Mixtral size or smaller at insane speeds we're talking like, maybe I don't know, like at least a thousand tokens a second probably, like maybe even five thousand or like more, and that even might even be conservative too look, there's insane amounts of compute and bandwidth here that you could have, especially for small models. [01:08:53] Alex Volkov: That's quite incredible. I don't know if that's in production at some point or when it's going to be, but at least based on the numbers, this looks just absolutely like a chunk of incredible. [01:09:05] Extropic announces their Thermodynamic processing unit [01:09:05] Alex Volkov: And in addition, Harvard News is super quick. Extropic, the folks who are, I think founded by folks who were in DeepMind before and did some. [01:09:15] Alex Volkov: Quantum computing stuff. I think that's what Guillaume's background is. They announced their TPU or like at least what they're going to build or thinking about building which is not a a tensor processing unit like TPU from Google. It's a thermodynamic processing unit. It's basically a teasing at this point. [01:09:32] Alex Volkov: I don't think they have. Hardware ready to go, or at least not that I understand. And I had to, I'll add this to the show notes. I had to dig deep into trying to understand what is it that they announced. And it was really hard for me. And it didn't seem like, my non hardware background was the reason. [01:09:48] Alex Volkov: It felt like some other folks also getting a little bit lost in what they actually talked about. LDJ, if you want to take a stab at giving us like a little brief recap, I would really appreciate it, because I know that you are in some of these spaces. But thermodynamic is like a new approach to basically doing AI, as far as I understood. [01:10:07] LDJ: Sure, yeah so yeah, there was a Q& A that they held yesterday. which I think it actually, I think it's recorded, and maybe the FJ's Ozarksropic page it might be there for anybody to listen to, but I spoke with them a bit, and Guillaume, the CEO, and Trevor, the CTO, they're both creators of TensorFlow Quantum, and they worked at Google, and they didn't work at DeepMind, but they actually worked on something arguably a little cooler than DeepMind, actually, depending on who you ask, called a Google X, Which is pretty much the secret development division of Google, where they work on very long term deep technology projects. [01:10:44] LDJ: And, yeah Trevor and Guillaume, they met at Google X, when they were working on TensorFlow Quantum and a lot of quantum computing technologies. And a lot of the systems that they had to develop to mitigate, Like all the errors that built up in the quantum computing system that they had to account for, they ended up on a path where, hey, we could actually start using this technology itself for the computing in the first place. [01:11:09] LDJ: And the goal is really just general. like speed up of mainly like things like gradient descent and operations that are pretty much used in all of deep learning and all of AI. So it's not just specific to transformers or just specific to this and that and yeah they plan to have a bunch of server grade chips like within the next Let's say around three years or so and they plan to have consumer available chips as well in accelerator form factors. [01:11:40] LDJ: So you'd be able to just plug it into your motherboard, just like you plug in a GPU into your motherboard today, and it would just be an accelerator card that has this thermodynamic computing components within it that would be able to accelerate your AI workloads just way more. [01:11:55] Alex Volkov: That's incredible. I think they wanted to call this the transistor of the AI era, which transistors like a big step function change in the world of computing. So shout out to them. It still looks like it's a little bit ways out, but definitely they're getting interest. And then the very techno positive outlook or techno optimist part of outlook is definitely also. [01:12:16] Alex Volkov: Also helpful. So I think that's mostly it on the hardware news and robotics. We don't usually often cover, but this week seems to be, seems, seemed to have been a very big week in the hardware news and robotics and A lot of stuff happened that pertain into kind of like tech optimism and very big week for announcements and different things. [01:12:35] Alex Volkov: The chip design for whatever they're doing looks, looks crazy as well. So definitely folks who are into this, go check it out and let us know what you think. In comments as well. I think we've been at this for almost an hour and something, and I do want to do like a little reset, maybe drink a little bit. [01:12:50] Alex Volkov: So let's do a short reset of the space and I'll talk about some stuff that happens in Weights Biases. And then we're going to continue talking. We still have to talk about Devon. So we'll brief, brief reset, and then we're going to pick up on this. [01:13:27] Alex Volkov: Alright, you are on ThursdAI, today is Pi Day, March 14th, and this day started crazy and kept getting crazier, so today morning, early on, many folks woke up to see SpaceX launch the largest man made object ever to break through the atmosphere, and this was a crazy thing, the first, the third thing they tried this year also is the birthday. [01:13:52] Alex Volkov: or anniversary of GPT 4 that was released a year ago exactly on Pi Day, March 14th. And we're still waiting for some breaking news to come through. And, hopefully they release something. There were rumors that GPT 5 is coming up. There were Microsoft copilot pages said, Hey, you can get access to GPT 5 for all. [01:14:09] Alex Volkov: You saw this, right? And then those rumors were discarded. And they said, Microsoft said there was a typo. And we're going to try and see what else you know, what else we're going to get here today in breaking news. But also today's an anniversary of Entropiq's Claude, the first Claude, the first kind of production model that they released, the Entropiq released was also a year ago. [01:14:32] Alex Volkov: And very big week last year, very big week this year as well. And of course, it's also ThursdAI, BirthdAI, it's a one year anniversary of these spaces as I've been at least hosting them for the past year. Consistently, I think we missed one. I think I was really sick and I missed one. I still sent the newsletter, but I missed it. [01:14:49] Alex Volkov: the space. And so we're here celebrating the ThursdAI birthday night with a bunch of friends here on stage. And I think in this vein it's now a good time for me to say that, ThursdAI is not sponsored. If you want [01:15:00] to support this, please support us, please follow ThursdAI, the newsletter. [01:15:03] [01:15:03] This weeks buzz - Weights & Biases update [01:15:03] Alex Volkov: And if you want to engage with us on socials, that definitely helps because sometimes the rich is the rich is not how should I say, it's hidden on X for some reasons. We've seen better and worse times. [01:15:13] Alex Volkov: So definitely if you want to follow us and give us a follow to the main account, but also like retweet when we start the space that we're gonna be super, super helpful. But the space is not hosted, is not sponsored besides Weights Biases. And so I think I maybe tell you a little bit how I joined Weights Biases because this was also a thing. [01:15:30] Alex Volkov: So folks, remember me joining the spaces and thinking about, hey, this is fun to do. I have no idea how, how I'm going to make money. Back then, I The whole, one of the reasons to do this space was to promote my startup called Torgum. At some point, Weights Biases folks reached out and said, Hey, let us sponsor your newsletter and podcast. [01:15:48] Alex Volkov: Because the audience that you draw and the audience that we're looking at is very similar. And I was really apprehensive in the beginning. I didn't really want Take sponsorships as you guys may have liked the authenticity of the excitement and the stuff that we talked about. We never get like paid gigs. [01:16:05] Alex Volkov: Nobody pays to come and, push their stuff on ThursdAI, which I think the community appreciates. And so I was really thinking about, okay if this is the right thing, and then after a while, Waits Bites is, I was entertaining this because they really have A good stand with the open source community as well. [01:16:20] Alex Volkov: Their main product is free for personal use. And many folks in the audience that know and love Weights Biases way before I even knew what they are, gave me kind of the thumbs up. And then Weights Biases reached out again and said, Hey Alex, why don't you and ThursdAI join Weights Biases? And you just keep doing this in addition to being an AI evangelist or promoting our products and our different products, by the way. [01:16:42] Alex Volkov: W we have some new announcements very soon that I'm very excited about. And I, back then really started needing the money and the startup wasn't taking off, and so I said, Hell yes. This sounds like an amazing opportunity for me to keep doing this, to keep talking about AI with you folks. [01:16:58] Alex Volkov: Learning myself learning from folks on stage here who know way more than me, and then also learning in public, so other folks also follow up. And yeah, that's how I joined wins and biases. And now Thursday I is. The podcast and the newsletter are offered by weights and biases. The space is I talk about weights and biases stuff, but I talk about the stuff that have actually very excite me very much. [01:17:20] Alex Volkov: And so in the vein of those stuff, I just wanted to add that I'm going to San Francisco in a month and when it's me and everybody else in WIS and biases because our. Annual conference called Fully Connected starts in it's going to be in April 18th in San Francisco. And the tickets are still early bird, so up until end of this month, the tickets, you can get them for 50 percent off. [01:17:41] Alex Volkov: And it's an opportunity to get How should I say this? One of the reasons why I joined Weights Biases is because everybody's a customer, including, 4 was trained with Weights Biases. But also, pretty much every other foundational lab that builds foundational models and in different, in robotics and different other places, just The amount of logos that our customers, Weights Biases, just beats any other company I've ever worked at, or even looked at, it's crazy. [01:18:06] Alex Volkov: And so many of these folks will come to this conference to also talk about what they're building, the models. So a very good opportunity to visit San Francisco and join us. A day before this conference, I'm going to do a workshop. And me along with my team, the growth ML team in Noise Ambassadors. We're going to do a workshop about improving your production step by step. [01:18:25] Alex Volkov: And it's going to be super cool. We're going to talk about evaluations. We're going to talk about different other things that we know from the enterprise community, the folks who actually use AI in production. They talk to us. We have our own AI that we're running called OneBot that you're more than welcome to use. [01:18:38] Alex Volkov: So definitely. Come and meet us in San Francisco in April or in London in May, by the way, if you're Europe based we have a the same kind of conference in London, which I probably won't be attending in London, but you never know, maybe I'll get called. With this, I think we're moving towards, let's talk about agents. [01:18:56] Alex Volkov: And I think this week was a big thing for agents. Don't you guys think? Who saw the Devon announcements? I'm just gonna do it like this. Yeah. Nisten, what do you think? Who didn't, right? Like they, they exploded into everybody's feed, I think faster than AutoGPT a year ago or something. [01:19:14] Cognition Labs Showcases Devin the first AI software engineer [01:19:14] Alex Volkov: And so let's basically do a brief cover of the Devon announcement, and then we'll talk about what it actually means. And then I have. And then I think I'll open up the space for the first time in a month or in a year to actually talk with people who are in the audience and want to come up and tell us about their experiences with ThursdAI. [01:19:29] Alex Volkov: Cognition Labs, a fairly new company, looks like funded fully. released Devin, their, what they call the first fully autonomous AI software engineer. And we've seen these claims before, and some of us are very skeptical, because these demos are incredible, and then when you actually get to use them, the model loses context, etc. [01:19:48] Alex Volkov: And, um, they claimed the setting a new standard on the software engineer bench coding benchmark, SVE bench coding benchmark. which I think they're outperforming all these things and getting around 18 percent on the SVE bench. Which is a specific task for, not only writing pieces of code, but also performing tasks. [01:20:08] Alex Volkov: They claim it's operating as a highly capable teammate, capable of working alongside human engineers, independently tackling tasks for their review. So one of the things that caught me by surprise. But by a fairly surprise that, compared to something like AutoGPT before or other agents that we saw, and we've talked with multiple agent folks on the pod, we've talked with João from crew AI, that's been like in the open source community being very highlighted recently, we've talked with Killian from AutoGPT, we've talked with a bunch of agent folks this Devin has besides the company and the investment and everything, the UI is very polished. [01:20:44] Alex Volkov: The UI is actually a set of tools and I asked a few folks with access to Devon So if you have access to Devon, please DM me and come up and talk about this if you're listening to this The UI has access to shell So you can see a shell, like a terminal, that the UI has probably access to a virtual machine as well. [01:21:03] Alex Volkov: It has a browser that you as a user can see and Devin as an AI agent can use the browser. So for example, you can log in and have authenticated sessions for different things that then Devin can use this browser. The UI has access to a code editor, basically, that you can see that Devin writes things in. [01:21:22] Alex Volkov: And you have access to a chat. And I think that the combination of four of these things in the UI, plus the ability to scroll back and follow Devon in real time, but also scroll back to see something that Devon did a few steps before, I think is very powerful, and I still haven't seen anything like this. [01:21:39] Alex Volkov: I think for many people this broke the kind of the the threshold of something that's useful. Far El, go ahead, you have your hand up. [01:21:46] Far El: Devon's very impressive, but of course it's not open source. I posted on top here a an open source version of Devon called MetaGPT, which self reports to be better than Devon. It's up to, like we need to do evaluations to find out, but also there's several. Open source communities that have formed we're talking about a group with dozen folks, another group with hundreds of people who are all coordinating to replicate. [01:22:13] Far El: Devin in open source. I think actually one of the people here is Jun Yang in the audience that I'm seeing here who is trying to also replicate open source Devin. So maybe if you want to bring him up to, to discuss that. But yeah, in general I think Devin is is impressive, but what's the most interesting insight is potentially the fact that this is just a GPT 4 wrapper, and they've just managed to squeeze so much more out of GPT 4 than we have been able to. [01:22:41] Far El: Definitely a lot of interesting things to come based on just knowing that this is possible. [01:22:46] Alex Volkov: What you said, Far El, is very similar to when ChatGPT came out, and this was quote unquote, just the UI, right? There's no technological breakthrough necessarily in chatGPT's release, but the fact that it was like nicely packaged, the fact that they kept sending back and forth messages to keep the state for you, the memory for you as well, broke the level for many folks who were not like using the API for completion before. [01:23:10] Alex Volkov: Definitely Jun Yang is more than welcome always LDJ, I'll get to you and then we'll talk with Jun Yang about the kind of the open source, but also I do want to cover like the rest of the stuff that got excited folks about [01:23:20] Devon. Go ahead. LDJ? Yeah. [01:23:25] LDJ: Yeah. Okay. Apparently there was an interview where they were asking the The Devon folks about what models is it using or whatever, and apparently they said that they are using, they're vague about it, or like maybe the interviewer just didn't get it that well, but they said an LLM with reinforcement learning. [01:23:43] LDJ: And that could just mean like RLHF, but I think they might be talking about like real like traditional reinforcement learning where you're actually like. Like it's the holy grail of if you have something that's coding and you have it being able to learn and compete against itself and being able to iteratively improve and things like that of complex tasks like something like that. [01:24:03] LDJ: So that'd be really interesting if that's the case and it seems like that's what they were maybe alluding to that they have a custom model that is trained through reinforcement [01:24:11] Justin Lin: learning. [01:24:12] Alex Volkov: Yeah, and I think it's very important to also highlight that the UI they built around this and the tools that Devon is able to use, even if it's like a wrapper, the ability of them to promote these kind of tools is very interesting. The browser, to me, is one of the more interesting parts as well, because I know for a fact when I code, I log into a bunch of like services. [01:24:32] Alex Volkov: I read their APIs, some of them APIs and keys and everything are only locked behind a login wall, for example. And so for something like an auto GPT or even cursor, right? So we, I know that I and some other folks we use cursor. For coding as well, Cursor has some agentic properties. You can ask it about your code, even edits your code inside your code editor. [01:24:53] Alex Volkov: And then it's able to perform some of these like meta, meta tasks, like figure out what's the problem, go and, search or something. And the ability of, Devin to do this. I saw one video where like it decided to change the code to add debugger statements and then get a better handle on what the actual issue is and then perform something. [01:25:13] Alex Volkov: And should I say? The big problem with something like let me add Slav as well. The big problem with something like AutoGPT before was getting lost. Getting lost in context, getting lost in the more tasks it executes. I saw enough videos of folks, enough folks who are not shills of Devon, for example. [01:25:29] Alex Volkov: They are happy to promote the fact that they got early access, but they're not paid probably by Devon folks by Cognition Lab folks. Enough demos of them recording continuously for 20 plus 30 minutes where Devon actually executes stuff based on the plan 20 minutes in. And I personally haven't seen this from a lot of other agents as well. [01:25:48] Alex Volkov: So I do want to acknowledge Justin Junyang on the stage the member of the technical team in in Quen, the Quen team go, I think is the profile. Hey Justin, how are you? Thanks for joining ThursdAI, Birthday AI. [01:26:00] Justin Lin: Yeah. Hi, Alex. Thanks for bringing me. Yeah. I'm a member of the Quentin. Oh. I just recently met Devin. It's very impressive. And we're just talking about maybe just related to code large language model. Actually, we are doing something about it, so we just raised Twitter to say, Hey anybody is interested? [01:26:25] Justin Lin: I don't know. It is really [01:26:27] Justin Lin: hot. There are [01:26:29] Rohan Pandey: a lot of people who are joining us, [01:26:31] NA: Hoping to reproduce the open source Devon. We still don't have a clear roadmap, but for now, For the model, we may think about maybe for the first step, we still use something like a closed source model like GPT 4. [01:26:49] NA: Admittedly, even like 5 is not enough for such complex tasks. I have some rumor that Devlin might be built upon GPT 4. For with a very good Chrome engineering. I don't know if this is true yet, but we may start from this to build something like a demo. And for another part and for the model, we may build something like, Code large language model, and especially it is adapted to very long context. [01:27:21] NA: So it can probably browse the web pages, crawl the contents, and then based on the contents, and then write some code and do something complex. Yeah, this is generally some initial ideas, and we still need some time to think about what to exactly [01:27:40] Justin Lin: do next, yeah. [01:27:42] Alex Volkov: So I, I would, so first of all, folks follow Junyang in this effort and definitely hear more from the open sourcing. I think like Far El said, one of the, one of the best things when something like this comes out, it gives it like a fairly clear roadmap for folks to try and replicate. And I think the roadmap should include the UI itself. [01:27:59] Alex Volkov: The browsing UI is very, I think, important. The integrated shell is important. At least for the ability of you interacting with this. One thing that I also noticed for Devon is that you could actually talk with it while it performs other tasks. So like you can with an actual software engineer that works on your team, you can chat with the person while it performs like other tasks. [01:28:19] Alex Volkov: I'm not sure how they necessarily achieved this. But it's very interesting where like it probably executes in several steps. They definitely built something there that's not only code execution. I think Nisten go ahead [01:28:28] Nisten: 300 years ago, my grandmother got automated. The machine was called, it was actually called a chain of cards machine by Basile Bouchon, and that went on to become the Jacquard loom, and my grandmother's knitting now became 10, 000 times faster. So that was 10, 000x of grandmas. AGI is only 1x. And and this thing you guys are talking about is 1x. [01:29:02] Far El: I don't know. Nisten is a grandmother 300 years old? [01:29:06] Nisten: The jacket machine is 300 years old. Yeah it was first like in 1725. No b******t, no, for real. And that actually used like punch card. It was called chain of cards. That's the real, that's the real name of it. This not Chain of Thought, Chain of Cards, it's the same thing, it's just instruction following Chain of Cards machine. [01:29:25] Nisten: And it made it, it made stuff close 10, 000 times faster than my grandma could. Now, that didn't stop my grandma from knitting. I don't know why people are freaking the heck out that now this thing can do 13. 2 percent of, of Github issues I am freaking out that we, with all of this automation, smartest freaking Olympiad kids in the world, I ranked let's leave that alone. [01:29:54] Alex Volkov: Ha. [01:29:58] Nisten: like we can barely, and we still have to do the other 87 percent of the work. I don't know why people are freaking the [01:30:04] Nisten: yeah, they said the same thing for. For people with Excel, it's oh, all the programmers are analysts, whatever the heck they were called back then. It's going to automate them. [01:30:14] Nisten: Did it automate? Yeah, it automated them. Has the need for them improved? Yeah. The same thing for Copilot came out two years ago. We've been using these tools for two years. You still can't find a good JavaScript dev to hire. Dude, people are freaking the f**k out, man. [01:30:33] Alex Volkov: So let's actually talk about this. [01:30:35] Nisten: Learn the code don't be dumb, [01:30:37] Alex Volkov: least some of the conversation, Nisten, at least some of the conversations is, just like thread boys hyping up things. Oh, software engineer is dead, whatever. They will always keep doing this. They're engaging the algorithm on X rather than providing some value. [01:30:51] Alex Volkov: But there's definitely folks that I saw, they're replying to like major folks and Hey. I'm about to learn coding, should I even learn coding? Because to them, when somebody doesn't know coding, sees something like Devon, they're like, why do I even need to study? In a few years this will be, like, way better than me in Even now it's way better than something like a starting point. [01:31:11] Alex Volkov: And I think the answer to this is that the world just will need more code. Like, it's very interesting that software engineers, in general as a concept, try to automate ourselves out of laziness as much as possible, right? I would spend sometimes days on automating a task, I can complete manually for five minutes just because I know that I'd be able to do some other stuff faster while this is getting automated. [01:31:32] Alex Volkov: Sometimes it's nerd sniping, but whatever. And then I think that for folks who are in the audience who are like thinking about learning to code, learn to code. [01:31:40] The reason is Devon will need your help to figure out what to do next. The outputs of Devon need somebody that knows how to code like none of the folks who got Devon are like marketing people just completely noobs that just like it worked for them. [01:31:53] Alex Volkov: So you do need the ability to actually run these things productively. And I think learning to cause a very important skill. And if anything, it will give you like a meta skill that you'd be able to do the boring stuff, the more complex stuff, you'd be able to review and achieve more. And I think that's very important. [01:32:11] Alex Volkov: Many of us. How should I say? There, there are some gatekeepers in the coding community for whom the ability to code is their way, their thing to say, okay, this is how we make money. But for many people in the coding thing is like, coding is just a tool to get somewhere. This somewhere is shipping a product, creating, like doing a task, doing some of these things. [01:32:30] Alex Volkov: That's not going to go away. If anything, this is gonna go and get that much better. So I saw Slava, you wanted to comment on that and then Roy. [01:32:42] Nisten: Yeah, [01:32:42] Slava Kurilyak: I wanted to add some color to the Devon circumstance where we find ourselves using at least a new approach where it seems like GPT 4 has been, let's say, claimed at this moment to be empowered by reinforcement learning. There are now. Developers who are going down this path. I'll do a shout out for one of them. [01:33:05] Slava Kurilyak: This is a developer. His name is Rohan. I'll pin his open source project to the top. It's called Llama Gym. Feel free to check this out. This is an agentic framework for using reinforcement learning to essentially fine tune language models. Now, why would you do this? Because, from my experiments with language models, the, at first you can get away with prompt engineering, but at some point. [01:33:30] Slava Kurilyak: To mimic human like performance, you do need to fine tune. And so in this circumstance, reinforcement learning has been shown to have incredible progress, especially with companies like DeepMind. But, and yet, we haven't really seen adoption for reinforcement learning within the Jamstack AI community, but now with tools like LlamaJam, developers can start to bridge the two. [01:33:56] LDJ: Can you post that to the billboard, [01:33:58] Alex Volkov: Yes please post as well. [01:33:59] LDJ: happen to have a link or a tweet [01:34:01] Alex Volkov: Absolutely. Roy, go ahead and afterwards I want to acknowledge Ryan joining us on stage as well. [01:34:06] LDJ: Yeah, [01:34:06] Roei Cohen: first I just want to give Nisten props for his just incredible rant. I just enjoyed that thoroughly. I actually don't agree with you, Alex. I think that eventually we'll see coding if not go away, be abstracted enough that it would be closer to English or whatever natural language you're used to using. [01:34:25] Roei Cohen: The reason I'm saying that is that's been the trend so far, we've gone from Assembler, from, Jacquard Looms to Assembler to, things that are more and more abstract and, the things that happen with a single line of code in Python or in, TypeScript or whatever generic language you choose. [01:34:43] Roei Cohen: have so much implementation actually going behind the scenes that you're not even aware of and people for some reason are okay with that. You know what I mean? They're not losing their ever loving minds. I think that as time goes by, right? These very mechanical operations are going to be needed less and less. [01:34:59] Roei Cohen: And, but the need to solve problems, to tackle problems, to have motivation and goals those are still going to be, mostly. Human, but those two may, may change, right? I think we have to prepare ourselves for two scenarios. One where. The need for, actual technical capabilities that are specialized, like coding, might be like less and less in demand to actually be effective and to be able to ship products and be able to ship features and whatnot but also that we're going to get more the agentic behavior of tools that we use is going to become more and more active and less passive, right? [01:35:36] Roei Cohen: It's not just that you're going to ask your agent to do something for you and then review it, but rather it will preemptively start working for you and solving problems for you and making PRs and, doing things of that sort, which kind of changes the way that the division of labor currently. [01:35:53] Roei Cohen: is in in terms of, like, how much work do humans drive and how much work do machines drive? [01:36:00] Alex Volkov: Yeah, thanks for that, Ray, and I definitely count on you to not agree with me, and I really appreciate the pushback as well. I want to acknowledge Ryan Carson. Ryan, I did not know that you're a follower of ThursdAI, but I have been following you and Treehouse for a while, so please start with maybe a brief introduction to who you are and what you currently do. [01:36:16] Alex Volkov: And I would love to hear your take about agentic and coders being replaced, given that Treehouse is something that you've previously built and taught tons of people to code. [01:36:26] Ryan Carson: Alex, good to be here. Thank you for the invite. It's funny because I was listening to a previous episode of ThursdAI while I was working out this morning, and that's what I knew. I wish I had known about this space earlier, because it's just packed with valuable information. There's so much for us to absorb in AI. I'm so thankful for this space, and I literally add it to my calendar now, so I'm hoping to show up more often, but, so thank you for that. Um, yeah, I spent a decade of my life, I'm 47, so I spent almost one out of every five minutes of my life trying to empower people to learn how to code. And at Treehouse, I had the honor of being a part of that, and, I think we taught something like a million people how to code. And and I have a computer science degree, and I think a lot about this. And, I think, I want to acknowledge and be empathetic towards professional software developers. Because it's, it is scary and hard to see things Appearing that look like they may replace you. That's scary for everybody. [01:37:26] Ryan Carson: And I think we all agree, we're just seeing a reaction to that. I think we all know that's an emotional reaction. It's not necessarily logical. But I do want to acknowledge, it's just scary for people if they think they're going to lose their job. So that's thing one. The thing two it's interesting I got a computer science degree, then I was a web developer for a long time, and then I started companies, and then I hired engineers, and engineering managers, and CTOs and I didn't code for a long time. [01:37:50] Ryan Carson: And after Treehouse was acquired I actually went back in and taught myself how to code again. And so I used ChatGPT Plus to teach me TypeScript and Next. js and I shipped a very simple proof of concept. Hey, I just want to build on top of OpenAI's APIs. I just want to understand soup to nuts, how this works. [01:38:09] Ryan Carson: And. You could say it's the dumbest thing ever. Like, why would you learn how to code again? But I think we all agree if you know how to code, it gives you this deep understanding of how things actually work, right? And I like to pull on an example here where think about building a house, right? [01:38:28] Ryan Carson: So you could abstract all of that away, but if you actually understand how to saw a piece of wood at a 45 degree angle, and then put it together with another piece of wood, And then you build something, it gives you a deep understanding and appreciation for the actual structure. And I think that's what's happening here. [01:38:47] Ryan Carson: And I just would, I actually say, please learn how to code more now than I've ever said in my whole life. Because number one, it's easier. Like, all you have to do is open any good LLM and say, I don't know how to do this. Please teach me, I'm a super newbie, I don't get any of this stuff. And for once we can be. [01:39:08] Ryan Carson: honest about how much we don't know and not be embarrassed about it. I always say to people, just please learn Python and then start building something. Because in the end, it will absolutely make you more powerful, even if Devon creates all the underlying infrastructure. If you understand what's basically going on, it will make you an even more powerful wielder of that technology. [01:39:29] Ryan Carson: So that's my little speech about why we should all keep coding, Alex. [01:39:33] Alex Volkov: 100 percent Ryan, I just want to shout out that I was trying to remember where I know you from, and I visited a feature of web apps in London, like twice, [01:39:40] Ryan Carson: No way! [01:39:41] Alex Volkov: And I really remember it from there. Like it was the 2012, I think 2013. [01:39:45] Ryan Carson: Oh my god! [01:39:46] Alex Volkov: like a bunch of people. So one of like my first professional career like trips was to London to one of your amazing conferences. [01:39:54] Ryan Carson: makes me so happy. I just literally got goosebumps. [01:39:56] Alex Volkov: And so in return, it makes me very happy that you're now considered a friend of [01:40:00] the pod. Feel free to come back and chime in as well. I want to also on the topic of what you mentioned, I want to ask Junaid, who's a friend of the pod, my friend, and we run the Denver AI meetups together. Because Junaid, you basically did this thing that Ryan just discussed. [01:40:13] Alex Volkov: And what's your take on Devon and how easier it is to automate now with some of this stuff? [01:40:21] Junaid: Yeah I'm excited about it. I am one of those people that started learning to code at the beginning of last year. Literally the very beginning of last year, I started with the OpenAI API Quickstart Tutorial Guide. and immediately, moved on to trying to build things that I could run on my iPhone, and in less than two months from when I first started learning, I launched my first app. [01:40:45] Junaid: Yeah, I'm 15 months in, and I see Devon, and it does not in any way, shape, or form make me think, Oh, shouldn't have done that this last 15 months. No way. It's just it's another tool that is going to be That much more useful for me to be able to take ideas and actually make them happen. And honestly, having built pretty much all my stuff, like using chat GPT as my junior developer yeah, this is awesome. [01:41:18] Junaid: You know how much less copying and pasting I'm going to have to do? So yeah, it's I think it's fantastic. And I don't, I think that anybody who's on the fence or worried Whether they should learn to code, the answer is more yes now than it was before Devon came out. That's my [01:41:37] NA: take. [01:41:37] Alex Volkov: 100%. Ryan, go ahead, and then we got Slava again, I think. [01:41:40] NA: Junaid, wasn't it the most magical moment when you shipped that code and it worked? [01:41:48] Junaid: Yeah, absolutely. When I first went through the OpenAI, It's just like a little naming thing, how do you name your pet using the API? [01:41:58] Junaid: That was awesome. But [01:41:59] Junaid: man the real kick was when my first app actually got approved and went live. [01:42:05] Junaid: And I remember standing in my kitchen and doing like the dance of joy, Whoa, [01:42:10] Junaid: my God, I'm on the App Store! [01:42:12] Junaid: Wild. It's it's such a. Such a rush. Congrats. And for sure, the things that I've built so far are not like, hey, I'm not changing industries out here. I'm not like, whatever, but I can build things. [01:42:26] Junaid: I can use these tools more and more to build more and more things and build better and better things. And yeah, only up, it's only going to go up. [01:42:36] Alex Volkov: Right on. I wanted to acknowledge Anton as well. Anton, a build, the very popular GPT engineer, and also is very like, deep into CodeGen as well. Welcome, Anton. What's your take on Devin and the renewed interest in agents running code? [01:42:52] Justin Lin: Yeah, thanks, Alex. Nice to be here. I think it's super exciting. We've been trying the same approach. We haven't gotten as far and as fast as Devin, but I, it's always when you've seen something actually get done, you lose this doubt that you have from people telling you like, ah, this is not possible yet. [01:43:12] Justin Lin: And now when you've seen like. More people believe in it and it's still just a demo, right? It's not a product, but then your focus is just 10x. Super exciting times. And, I think on this topic of should you learn to code or not coding is one of the most fun things, but it does depend on what you want to do, what you want to achieve here in life. [01:43:36] Justin Lin: I think Flo Cruello a friend who invested in our company, he said that All the news headlines should just be a GI is here soon because , that's all that matters. And I think this is a good take on what should do you do with your life? A GI is here soon, so you should just do whatever makes you enjoy life. [01:43:55] Justin Lin: That, that was a lot of things, but but I, that, that's my takes. I could go the dive into the technical details. We did the deep analysis on how they're doing it at Deving compared to the things we tried and how we're doing it right now. [01:44:06] Alex Volkov: So give me an analysis from the perspective of the tools that they have for Devon. I think for me, definitely one of the main points was how much access it has to different tools like I have as a software engineer. Like I use the browser alongside my code editor, alongside my shell. Let's talk about this a little bit. [01:44:24] Justin Lin: Yeah, so I want to do a shout out to Eric in our team. So he built a tool called GPT ME. I think he's been working at it for two years and now we're building at Building a GPT engineer together and there, I think basically all the ways that Devin writes code like all the tools are available in GPT ME, but GPT ME is just a CLI. [01:44:47] Justin Lin: CLI tool so the browser, running code, writing code to file, changing code the biggest, please add if I missed some important tool that Devin has access to here. I'm running on too little sleep right now, but the biggest difference [01:45:03] Alex Volkov: And I think it has access to an actual terminal machine, so I saw that folks are able to run like LLAMA and actually run inference on it. So that's pretty impressive on its own in terms of infrastructure access. [01:45:14] Justin Lin: Yeah, correct. But I think you should, the people, if you're curious to run this you could try GPT Me, run it locally. The biggest difference is that Devin has done significant progress in making the agent stick to its plan and not get lost in a in confusing itself and not sticking to the plan, which has been like the big failure point for all the agents ever since they, since it started early last year. [01:45:43] Justin Lin: And Devon is better at sticking to the plan. plan. I'm sure it still also gets confused. And it has what we refer to as sub agents. I guess that's quite self explanatory what it means. And you have this main agent that says, oh, I should try to achieve this. And then a sub agent goes into its own path with a, with its own system prompt and so on. [01:46:04] Justin Lin: And there, I think as always, there is this in the details in how they've been quite successful there. But yeah, that's [01:46:11] Junaid: a quick summary. [01:46:12] Alex Volkov: Awesome. Thanks for joining, Anton. And folks, definitely check out Anton's feed and GPT Engineer, doing great things there. I want to acknowledge Rohan. Rohan, you were just mentioned by some folks with with your Lama Gym. You want to chime in on Dev and how this field moves forward and tell us about Lama Gym as well? [01:46:28] Rohan Pandey: Yeah, sure. Thanks, Alex. Yeah, the idea with LamaGen is that agents originated in reinforcement learning where they'd learn through interaction, right? They'd receive reward. They'd go around, play around with their environment, they'd explore and they'd learn. But now in the LLM age, when we have these LLM based agents, They don't really learn online in this reinforcement learning fashion so the idea with Lamagym is to be able to train these agents online with reinforcement learning and it's a super simple kind of agent class that you just implement a few prompt functions on and then throw it in a traditional OpenAI gym environment. [01:47:02] Rohan Pandey: And it learns, it propagates rewards from reinforcement learning. In terms of code generation stuff, this is actually what I spend most of my time on at Reworked. We do multi modal code generation for generating these web data extractors. In our code generation pipeline, it's not something where we're automating some huge stack of software engineering stuff where you have to go interact with the terminal, devin, and everything like that. [01:47:28] Rohan Pandey: But instead, it's This is a very specific task of generating a specific function this structured data extraction function for a specific website. So given some schema and a website, we go pull screenshots of the website, we go pull context from the html, we, and then this goes into this sort of agentic loop where we then generate code to extract that specific data and that goes straight into production effectively, right? [01:47:55] Rohan Pandey: It goes through some human review steps, but it goes straight into production. It's not like a it doesn't it isn't like your co pilot. It isn't something that you oversee. It is like in production. From, yeah, from just those user inputs to, to code that's executed. I think Devon shows like there's a lot of stuff that you can do just with GPT 4 right now, right? [01:48:15] Rohan Pandey: People didn't believe that yeah, GPT 4 agents for code generation were possible, [01:48:20] but I think, yeah, I saw a tweet that was like, maybe all you need to get AGI is GPT 4 and some IMO gold level prompt engineering, which maybe it's true. We've yeah, a lot of what we're doing, we've done some code fine tunes and whatnot, but a lot of improvement has also come from just putting GPT 4 in better agentic and prompt engineering type of setups. [01:48:42] Alex Volkov: Thanks for coming up, Rohan, and I just want to acknowledge for folks who on stage this is doesn't often happen Thursday, we usually cover the news and then we're at two hours already, but I really think that this conversation is important, and I really want to cover this and also open up to questions. [01:48:55] Alex Volkov: This stage on ThursdAI, ThursdAI to just cover the next iteration of things that are happening and many people for whom even co pilot is something they don't even use yet. Definitely not Cursor. Cursor is like absolutely the next level of co piloting to me in my code work. And I use like the command K every time and I'm very happy. [01:49:13] Alex Volkov: Besides the one fact that it deleted half my system previously, those of you who know about this, we'll talk about this separately. But I think it's a very important discussion specifically because Ryan, you mentioned something before where. We want to acknowledge and want to be I want to be compassionate to folks who are feeling this fear about their career, about the general rate of progress that's happening, not only in coding. [01:49:32] Alex Volkov: Coding is one simple thing of this. Writers, for example they look at your GPT, like professional writers, and they're getting booked less, for sure, because people can now write better. right? Long things and then review long things as well. And for many other people seeing something like the figure robot now with OpenAI, that scares them because they learn to, I don't know, watch Terminator. [01:49:51] Alex Volkov: I think one important piece of ThursdAI that I haven't mentioned yet, it's very important for me as my kind of AI evangelist position, is to acknowledge that change, very fast change scares people. And it scares people more than when they don't follow everything. And suddenly they see this rate of problems like, holy s**t, this means blah, blah, blah, blah, blah, X and X's, like whatever they saw in Black Mirror, which I think ruined many, like a generation ruined of potential thinking positive about the future. [01:50:19] Alex Volkov: And I think it's very important for us to have a conversation with this, for folks who are building code generation tools, for folks who are writing code, for folks who are learning like Junaid, to actually show that no, it's actually not only fine, it's even better. From the of code specifically, I think there's just going to be need. [01:50:34] Alex Volkov: for more code around the world, more automation around the world. And if you learn what these outputs can do, then I think you're gonna be even more productive. Ryan, you wanted to chime in as well? Feel free to step in. I'm gonna try to pull up one more friend. [01:50:48] Ryan Carson: You bet. Yeah. Thanks for having me up. I, it's so fascinating to hear about all these agentic systems and what's happening and I know we all know this is where we're going, and I tweeted out and said, as soon as you have an agent that's basically able to use the internet and a computer, like a basic human, there's so many things that you can start to tackle. [01:51:07] Ryan Carson: Researching, cures the diseases, planning your trip, to. Your mom's house for the summer. There's just a lot of this which ideally allows humans to level up and then leverage those tools, right? I'm always a technical optimist though, so that's probably my downfall. [01:51:22] Ryan Carson: Alex, I did want to say thank you for bringing me up. I've got to go. I joined Intel on Monday I'm helping, So I'm helping them build a global AI developer community. So I've gotta, I've gotta go to a meeting. But I wanted to pimp your stuff for a second, though, and say the courses on Weights & Biases are really good. [01:51:40] Ryan Carson: And as someone who's, spent ten years of my life building courses, everybody should check out the free Weights & Biases courses. They're awesome. [01:51:47] Alex Volkov: Thank you so much. Thanks Ryan for coming up. And then let's talk about collaborating. Now you joined Intel, the company, definitely let's do some stuff together. The shout out is well deserved. The team behind the course is the team that I joined the growth ML team. And they're amazing. And a shout out to them. [01:52:00] Alex Volkov: Everything there is for free. And you can learn from fine tuning a foundational model to extracting better outputs from JSON. And it's all for free for you guys to enjoy. So definitely one B slash me one wandb.me/courses definitely check this out and thank you for everybody who joined so far. [01:52:17] Alex Volkov: I try to keep this conversation going with folks. I also do wanna deliver this conversation to the folks who follow Thursday Eye on the podcast as well. So if you're not subscribed, definitely you subscribe. If you can help. Vote with whatever amount of stars, five is preferable on the, on, on the podcast. [01:52:35] Alex Volkov: I do want to acknowledge that Yam is a frequent co host, friend of the pod, and Yam, this birthday wouldn't be the same birthday without you. You want to chime in on the coding thing real quick, or on the Devon thing real quick? Before I do a recap, I would appreciate your things here as well. [01:52:49] Yam Peleg: first, it's amazing. It's amazing. The demos look amazing. I just wanna Ask or say that I think that the real test is how reliable is it with real world users of many people. And so if anyone knows, anyone tries and can share their experience, but out, out of demos. Real life tasks that can be anything. [01:53:10] Alex Volkov: I tried to get folks, Yeah, I try to get folks who actually has access to Devon I reached out to a few Thursday morning or at least for some of them it's really hard, but we'll definitely get folks, if not the Devon folks themselves, we'll definitely get folks who have access to Devon and we're gonna try to get access ourselves as well, um, definitely CodeAgents reimagined excitement about CodeAgents this year. [01:53:33] Alex Volkov: I had this this poll that I posted on my feed, where if Chad, if AutoGPT came, Less than a year ago came out and then like it broke pretty much the same level of excitement Not remotely the same level of execution ability, right? Like it wasn't any tools, etc fairly quickly folks got excited about the demos Then fairly quickly folks realized that you know Anton said and some other folks said It does get lost in the context after executing on a few things and so there's this ability Since then we've gotten Incredible context length with incredible ability and like needle in a haystack and these models like memory of working memory grew. [01:54:09] Alex Volkov: So I asked basically on my feed, do you feel from AutoGPT less than a year ago to Devin right now, which I think announced like a huge raise from many VCs. Do you feel that agents are on the same exponential curve as other LLM stuff that we see in open source, for example? And yeah, the answers were pretty much where I am at, where, the distance between something in AutoGPT and examples of, visual examples of DevIn they don't feel to me that there's been a year of progress compared to the year of progress we saw in everything else in OpenAI in LLMs, right? [01:54:42] Alex Volkov: But maybe I'm wrong and maybe I need to play with Devon to actually feel the AGI a little bit. So we'll see after we get access. We're gonna give you guys an update as well. And I think it's time for us to conclude the space. It's been a little bit over two hours as well. I will just say that before I conclude the space for the folks who are listening on the, on the podcast, I recap try to recap everything we've talked about here as well. [01:55:05] Alex Volkov: So that's coming up. If you've missed any part of the show, please stay with us to hear the recap. And I am very happy that we have celebrated Thursday birthday with all of you today in the space. It's been a great honor of mine to keep doing this and have many new folks come in to the stage, but also see some folks who we've been we've been hosting and friends of the pod and I really appreciate my time here with all of you. [01:55:27] Alex Volkov: I'm very happy that this keeps happening, and I'm not going away anytime soon. . [01:55:31] END OF SHOW [01:55:31] Alex Volkov: I think it's time to just say that, again, I really appreciate everybody here. Yam, thank you, dude. Thank you for joining from week to week. Thank you for breaking down papers for us and teaching us teachable moments from your excapades into AI and being the resident machine learning engineer. [01:55:45] Alex Volkov: Nisten, Thank you, brother, for holding the space holding the space when I can keep talking as well and joining and explaining, reading papers together and and asking questions and doing a co hosting with me. Far El, thank you for being the staunch supporter of open source everything and as much as possible. [01:56:03] Alex Volkov: Not believing big companies and their promises and keeping us honest in what we believe and not believe. LDJ, thank you brother for joining and explaining difficult concepts where I have no idea how to even explain them. Junyang, I really appreciate the fact that we have foundational model trainers here on stage, parts of ThursdAI, so thank you Junaid, Nisten, and keep giving us amazing Quint stuff. [01:56:23] Alex Volkov: As well. I really appreciate your expertise and pushing back on everything that I say with the not skepticism, but definitely those realism. Those of realism. I really appreciate this. Everybody else who wore on stage, everybody in the audience. I am floored that this keeps happening week after week, and I definitely am going to be here next week [01:56:40] to talk about whatever happens next. [01:56:42] Alex Volkov: I see a lot of faces in the audience that joined from week to week. Harrison definitely give Harrison a follow. His YouTube thing is great. Junaid, who just joined and talked about how he was a noob and learned from GPT 4, and now he has multiple apps. And Junaid and I are co hosting the Denver meetup. [01:56:59] Alex Volkov: As well. So if you're in Denver environment, please join us. We're gonna meet soon and talk about ai. I see Bo Wang from Gene ai and often join us when to talk about embeddings as well. I see Tanish in the audience from MedAR a very young PhD who I appreciate also friend of the pod. I see Abby, I see a bunch of friends here who. [01:57:16] Alex Volkov: know about the space way more than I could ever. And the fact that they all join and talk about this is what makes this interesting. So I really appreciate all of you one by one and everybody in the audience should give all these folks a follow and we'll see you here next week. Thank you, everyone. This is a public episode. If you'd like to discuss this with other subscribers or get access to bonus episodes, visit sub.thursdai.news/subscribe | |||
22 Mar 2024 | 📅 ThursdAI - Mar 21 - Grok, GTC, first OSS AI hardware, Neuralink Human, Prompting Claude and more AI news | 01:44:52 | |
March madness... I know for some folks this means basketball or something, but since this is an AI newsletter, and this March was indeed mad, I am claiming it. This week seemed madder from one day to another. And the ai announcements kept coming throughout the recording, I used the "breaking news" button a few times during this week's show! This week we covered tons of corporate AI drama in the BigCO segment, from Inflection → Microsoft move, to Apple Gemini rumors, to Nvidia GTC conference, but we also had a bunch of OpenSource to go over, including an exciting glimpse into the O1 from Open Interpreter, which the founder Killian (of the ThursdAI mafia haha) joined to chat about briefly after an all nighter release push! Another returning FOTP (friend of the pod) Matt Shumer joined as we did a little deep dive into prompting Claude, and how he went viral (seems to happen a lot to Matt) with a project of his to make Claude write prompts for itself! Definitely worth a listen, it's the first segment post the TL'DR on the pod 👂 this week. Btw, did you already check out fully connected? It's the annual Weights & Biases conference in SF next month, and tickets are flying, I'm going to be there and actually do a workshop one day prior, would love to invite you to join as well! TL;DR of all topics covered: * Open Source LLMs * Xai open sources Grok (X, Blog, HF, Github) * Sakana AI releases a new paper + 2 JP merged SOTA models (X, Paper, Blogpost) * Open Interpreter announces O1 - the Linux for AI devices (X, Project) * LM studio new modes (X) * Big CO LLMs + APIs * Nvidia GTC conference - Blackwell platform, NIMs and Gr00t robotics * Jensen interviewed transformers authors * Apple rumored to look at a deal including GEMINI * Apple releases a multi modal MM1 paper (X) * Inflection founders leave to head Microsoft AI * Google opens up Gemini 1.5 with 1M context access to all (X) * Vision & Video * NVIDIA + MIT release VILA (13B, 7B and 2.7B) (X, HuggingFace, Paper) * This week's BUZZ * Fully Connected is coming, sign up here, get tickets, join us. * I'm running a workshop in SF a day before on improving your LLM step by step including exciting announcements (same link) * Voice & Audio * Suno V3 launched officially (X, Blog, Play with it) * Distil-whisper-v3 - more accurate, and 6x version of whisper large (X, Code) * AI Art & Diffusion & 3D * Stability presents SD3 TURBO - 4 steps to get same high quality generation (Paper) * Stability open sources Stable Video 3D (Blog, Models) * Tools & Others * Neuralink interview with the first Human NeuroNaut - Nolan (X) * Lex & Sama released a podcast, barely any news * Matt Shumer releases his Claude Prompt engineer (X, Metaprompt, Matt's Collab) Open Source LLMs Xai open sources Grok (X, Blog, HF, Github) Well, Space Uncle Elon has a huge week, from sending starship into orbit successfully to open sourcing an LLM for us, and a huge one at that. Grok is a 314B parameter behemoth, with a mixture of experts architecture of 80B per expert and two active at the same time. It's released as a base model, and maybe that's why it was received with initial excitement but then, nobody in the GPU poor compute category has the ability to run/finetune it! In terms of performance, it barely beats out Mixtral, while being almost 10x larger, which just shows that.... data is important, maybe more important than Github stars as Arthur (CEO Mistral) helpfully pointed out to Igor (founder of Xai). Still big props to the team for training and releasing this model under apache 2 license. Sakana AI launches 2 new models using evolutionary algo merging Yeah, that's a mouthful, i've been following Hardmaru (David Ha) for a while before he joined Sakana, and only when the founder (and a co-author on transformers) LLion Jones talked about it on stage at GTC the things connected. Sakana means fish in Japanese, and the idea behind this lab is to create things with using nature like evolutionary algorithms. The first thing they open sourced was 2 new SOTA models for Japanese LLM, beating significantly larger models, by using Merging (which we covered with Maxime previously, and whom Sakana shouted out in their work actually) Open Interpreter announces 01 Light - the linux of AI hardware devices Breaking news indeed, after we saw the release of R1 go viral in January, Killian (with whom we chatted previously in our most favorited episode of last year) posted that if someone wants to build the open source version of R1, it'll be super cool and fit with the vision of Open Interpreter very well. And then MANY people did (more than 200), and the O1 project got started, and fast forward a few months, we now have a first glimpse (and the ability to actually pre-order) the O1 Light, their first device that's a button that communicates with your computer (and in the future, with their cloud) and interacts with a local agent that runs code and can learn how do to things with a skill library. It's all very very exciting, and to see how this idea goes from an announcement on X, to hundreds of folks collaborating and pushing this to the open has been incredible, and we'll definitely do a deeper dive into capabilities and the whole project once the launch craziness dies down a bit (Killian joined us at the epitome of the launch all-nighter haha) This is poised to be the first open source AI device, completely with .stl files for 3d printing at home, chip designs, ability to run end to end locally on your mac and we really applaud the team for this release 🫡 Big CO LLMs + APIs Nvidia GTC annual conference - New Blackwell platform, NIMs, Robotics and everything AI + a chat with the transformer avengers This week Nvidia had their annual GTC conference, where Jensen announced a ton of stuff, but the highlights where the new Blackwell chip (the next iteration of the H100) and the GB200 racks with a whopping 720PFlops of compute ( to put this number in perspective: the first DGX that Jensen delivered to OpenAI in 2016 was 0.17 Petaflops ) They also announced partnerships with everyone under the sun pretty much, a new way to deliver packaged AI experiences called NIMs (which we at weights & biases support as well) and a new foundational operating system for robotics called GR00T led by Dr Jim Fan. Jensen also had the whole transformers original authors cast together on stage (and in the green room) for an hour, for the first time, to chat about, well... transformers. I really need to find the whole video and post it because it's hidden inside the Nvidia GTC website, but it was a very fun chat, where the team reminisced about the naming and their thoughts on the future of LLMs. They also covered each individual company (all of them lefty Google since then) and what they all do. It was a great chat. Microsoft buys Inflection (almost) and Apple considers buying Gemini In other huge AI player news, 2 of the 3 founders of Inflection AI left to start Microsoft AI (together with some of the staff), namely Mustafa who founded inflection, then helped raise 1.8B dollars, get up to 22K H100 GPUs, release Inflection 2.5 that comes close to GPT4, and then decided to leave. Inflection also pivoted away from consumer (Pi was a very nice AI to chat with) into API services, and apparently Microsoft will pay Inflection $650 to Inflection in the form of a licensing deal. Meanwhile there are rumors that Apple is eyeing Gemini to integrate into IOS, which is, very weird given the recent bad press about Gemini (Unless Apple doesn't want to deal with the same bad press?) and it's even weirder given the latest push from Apple into Open Source. Folks at apple this week released a new paper called MM1, outlining a new multi modal model they have trained (but not released) and show that it beats Gemini visual understanding. It was also great to see that the authors of that model shouted out Weights & Biases crew that helped them through their work on this paper👏 Nolan - the first NeuralNaut (first human with a Nauralink implanted) Just as I was summing up the notes for this week, Neuralink pinged that they are going to go live soon, and I tuned in to see a 20yo Paraplegic gamer, getting interviewed by a Neuralink employee, being very cheerful, while also playing a chess game, all with his brain. We went a really long way since the monkey playing Pong, and Nolan was able to describe his experience "it's like using The Force" of using Neuralink to control his mac cursor. It was all kind of mind-blowing, and even though brain implants are nothing new, the fidelity and the wireless connections + the very quick surgery made this demo such a nonchalant thing, that Nolan didn't even stop playing chess while being interviewed, probably not realizing that millions of people would be watching. They have a bunch of ML understanding the signals that Nolan sends from his brain wirelessly, and while this is very exciting, and Nolan prepares for this halloween as Professor X from X-men, because well, he's in fact a telekinesis enabled human, Elon claimed that their next target is fixing blindsight (and that it already works on monkeys) presumably via camera input being triggered in the visual cortex. Back in November 2022, I watched the Neuralink keynote and geeked out so hard about this section, where Dan Adams, one of the neuroscientists at Neuralink talked about how it's possible to trigger / stimulate the visual cortex to fix blindness and then generate an image. Well, this is it folks, we talked about tons of other stuff of course but these are the main points that made the cut into the newsletter, as always, if you want to support this newsletter/podcast, please share it with friends ❤️ Hope to see you in SF in April (I'll be giving more reminders don't worry) and see you here next ThursdAI 🫡 P.S - I said Intel a bunch of times when I mean Nvidia, apologies, didn’t notice until post publishing 😅 This is a public episode. If you'd like to discuss this with other subscribers or get access to bonus episodes, visit sub.thursdai.news/subscribe | |||
28 Mar 2024 | 📅 ThursdAI - Mar 28 - 3 new MoEs (XXL, Medium and Small), Opus is 👑 of the arena, Hume is sounding emotional + How Tanishq and Paul turn brainwaves into SDXL images 🧠👁️ | 01:35:10 | |
Hey everyone, this is Alex and can you believe that we're almost done with Q1 2024? March 2024 was kind of crazy of course, so I'm of course excited to see what April brings (besides Weights & Biases conference in SF called Fully Connected, which I encourage you to attend and say Hi to me and the team!) This week we have tons of exciting stuff on the leaderboards, say hello to the new best AI in the world Opus (+ some other surprises), in the open source we had new MoEs (one from Mosaic/Databricks folks, which tops the open source game, one from AI21 called Jamba that shows that a transformers alternative/hybrid can actually scale) and tiny MoE from Alibaba, as well as an incredible Emotion TTS from Hume. I also had the pleasure to finally sit down with friend of the pod Tanishq Abraham and Paul Scotti from MedArc and chatted about MindEye 2, how they teach AI to read minds using diffusion models 🤯🧠👁️ Thank you for reading ThursdAI - Recaps of the most high signal AI weekly spaces. This post is public so feel free to share it. TL;DR of all topics covered: * AI Leaderboard updates * Claude Opus is number 1 LLM on arena (and in the world) * Claude Haiku passes GPT4-0613 * 🔥 Starling 7B beta is the best Apache 2 model on LMsys, passing GPT3.5 * Open Source LLMs * Databricks/Mosaic DBRX - a new top Open Access model (X, HF) * 🔥 AI21 - Jamba 52B - Joint Attention Mamba MoE (Blog, HuggingFace) * Alibaba - Qwen1.5-MoE-A2.7B (Announcement, HF) * Starling - 7B that beats GPT3.5 on lmsys (HF) * LISA beats LORA as the frontrunner PeFT (X, Paper) * Mistral 0.2 Base released (Announcement) * Big CO LLMs + APIs * Emad leaves stability 🥺 * Apple rumors - Baidu, Gemini, Anthropic, who else? (X) * This weeks buzz * WandB Workshop in SF confirmed April 17 - LLM evaluations (sign up here) * Vision & Video * Sora showed some demos by actual artists, Air Head was great (Video) * Tencent Aniportait - generate Photorealistic Animated avatars (X) * MedArc - MindEye 2 - fMRI signals to diffusion models (X) * Voice & Audio * Hume demos EVI - empathic voice analysis & generation (X, demo) * AI Art & Diffusion & 3D * Adobe firefly adds structure reference and style transfer - (X, Demo) * Discussion * Deep dive into MindEye 2 with Tanishq & Paul from MedArc * Is narrow finetuning done-for with larger context + cheaper prices - debate 🥇🥈🥉Leaderboards updates from LMSys (Arena) This weeks updates to the LMsys arena are significant. (Reminder in LMsys they use a mix of MT-Bench, LLM as an evaluation and user ELO scores where users play with these models and choose which answer they prefer) For the first time since the Lmsys arena launched, the top model is NOT GPT-4 based. It's now Claude's Opus, but that's not surprising if you used the model, what IS surprising is that Haiku, it's tiniest, fastest brother is now well positioned at number 6, beating a GPT4 version from the summer, Mistral Large and other models while being dirt cheap. We also have an incredible show from the only Apache 2.0 licensed model in the top 15, Starling LM 7B beta, which is now 13th on the chart, with incredible finetune of a finetune (OpenChat) or Mistral 7B. 👏 Yes, you can now run a GPT3.5 beating model, on your mac, fully offline 👏 Incredible. Open Source LLMs (Welcome to MoE's) Mosaic/Databricks gave us DBRX 132B MoE - trained on 12T tokens (X, Blog, HF) Absolutely crushing the previous records, Mosaic has released the top open access model (one you can download and run and finetune) in a while, beating LLama 70B, Grok-1 (314B) and pretty much every other non closed source model in the world not only on metrics and evals, but also on inference speed It uses a Mixture of Experts (MoE) architecture with 16 experts that each activate for different tokens. this allows it to have 36 billion actively parameters compared to 13 billion for Mixtral. DBRX has strong capabilities in math, code, and natural language understanding. The real kicker is the size, It was pre-trained on 12 trillion tokens of text and code with a maximum context length of 32,000 tokens, which is just incredible, considering that LLama 2 was just 2T tokens. And the funny thing is, they call this DBRX-medium 👀 Wonder what large is all about. Graph credit Awni Hannun from MLX (Source) You can play with the DBRX here and you'll see that it is SUPER fast, not sure what Databricks magic they did there, or how much money they spent (ballpark of ~$10M) but it's truly an awesome model to see in the open access! 👏 AI21 releases JAMBA - a hybrid Transformer + Mamba 58B MoE (Blog, HF) Oh don't I love #BreakingNews on the show! Just a few moments before ThursdAI, AI21 dropped this bombshell of a model, which is not quite the best around (see above) but has a few very interesting things going for it. First, it's a hybrid architecture model, capturing the best of Transformers and Mamba architectures, and achieving incredible performance on the larger context window size (Transformers hardware requirements scale quadratically with attention/context window) AI21 are the first to show (and take the bet) that hybrid architecture models actually scale well, and are performant (this model comes close to Mixtral MoE on many benchmarks) while also being significantly cost advantageous and faster on inference on longer context window. In fact they claim that Jamba is the only model in its size class that fits up to 140K context on a single GPU! This is a massive effort and a very well received one, not only because this model is Apache 2.0 license (thank you AI21 👏) but also because this is now the longest context window model in the open weights (up to 256K) and we've yet to see the incredible amount of finetuning/optimizations that the open source community can do once they set their mind to it! (see Wing from Axolotl, add support for finetuning Jamba the same day it released) Can't wait to see the benchmarks for this model once it's properly instruction fine-tuned. Small MoE from Alibaba - Qwen 1.5 - MoE - A2.7B (Blog, HF) What a week for Mixture of Experts models, we got an additional MoE from the awesome Qwen team, where they show that training a A2.7B (the full model is actually 14B but only 2.7B are activated at the same time) is cheaper, 75% reduction in training costs and 174% improvement in inference speed! Also in open source: Lisa beats LORA for the best parameter efficient training 📰 LISA is a new method for memory-efficient large language model fine-tuning presented in a Hugging Face paper💪 LISA achieves better performance than LoRA with less time on models up to 70B parameters🧠 Deep networks are better suited to LISA, providing more memory savings than shallow networks💾 Gradient checkpointing greatly benefits LISA by only storing gradients for unfrozen layers📈 LISA can fine-tune models with up to 7B parameters on a single 24GB GPU🚀 Code implementation in LMFlow is very simple, only requiring 2 lines of code🤔 LISA outperforms full parameter training in instruction following tasks Big CO LLMs + APIs Emad departs from Stability AI. In a very surprising (perhaps unsurprising to some) move, Emad Mostaque, founder and ex-CEO of stability announces his departure, and focus on decentralized AI For me personally (and I know countless others) we all started our love for Open Source AI with Stable Diffusion 1.4, downloading the weights, understanding that we can create AI on our machines, playing around with this. It wasn't easy, stability was sued to oblivion, I think LAION is still down from a lawsuit but we got tons of incredible Open Source from Stability, and tons of incredible people who work/worked there. Big shoutout to Emad and very excited to see what he does next Throwback to NEURIPS where Emad borrowed my GPU Poor hat and wore it ironically 😂 Promised me a stability hat but... I won't hold it against it him 🙂 This weeks Buzz (What I learned with WandB this week) I'm so stoked about the workshop we're running before the annual Fully Connected conference in SF! Come hear about evaluations, better prompting with Claude, and tons of insights that we have to share in our workshop, and of course, join the main event on April 18 with the whole Weights & Biases crew! Vision Sora was given to artists, they created ... art Here's a short by a company called ShyKids who got access to SORA alongside other artists, it's so incredibly human, and I love the way they used storytelling to overcome technological issues like lack of consistency between shots. Watch it and enjoy imagining a world where you could create something like this without living your living room. This also shows that human creativity and art is still deep in the middle of all these creations, even with tools like SORA MindEye 2.0 - faster fMRI-to-image We had the awesome pleasure to have Tanishq Abraham and Paul Scotti, who recently released a significantly bette version of fMRI to Image model called MindEye 2.0, shortening the time it takes from 40 hours of data to just 1 hour of fMRI data. This is quite remarkable and I would encourage you to listen to the full interview that's coming out this Sunday on ThursdAI. Voice Hume announces EVI - their Empathic text to speech mode (Announcement, Demo) This one is big folks, really was blown away (see my blind reaction below), Hume announced EVI, a text to speech generator that can reply with emotions! It's really something, and it has be seen to experience. This is in addition to Hume already having an understanding of emotions via voice/imagery, and the whole end to end conversation with an LLM that understands what I feel is quite novel and exciting! The Fine-Tuning Disillusionment on X Quite a few folks noticed a sort of disillusionment from finetuning coming from some prominent pro open source, pro fine-tuning accounts leading me to post this: And we of course had to have a conversation about it, as well as Hamel Husain wrote this response blog called "Is Finetuning still valuable" I'll let you listen to the conversation, but I will say, like with RAG, finetuning is a broad term that doesn't apply evenly across the whole field. For some narrow use-cases, it may simply be better/cheaper/faster to deliver value to users with using smaller cheaper but longer context models and just provide all the information/instructions to the model in the context window. From the other side, we had data privacy concerns, RAG over a finetune model can absolutely be better than just a simple RAG, and just a LOT more considerations before we make this call that fine-tuning is not "valuable" for specific/narrow use-cases. This is it for this week folks, another incredible week in AI, full of new models, exciting developments and deep conversations! See you next week 👏 Transcript Below: [00:00:00] Alex Volkov: Hey, this is ThursdAI, I'm Alex Volkov, and just a little bit of housekeeping before the show. And what a great show we had today. This week started off slow with some, some news, but then quickly, quickly, many open source and open weights releases from Mosaic and from AI21 and from Alibaba. We're starting to pile on and at the end we had too many things to talk about as always. [00:00:36] Alex Volkov: , I want to thank my co hosts Nisten Tahirai, LDJ, Jan Peleg, And today we also had Robert Scoble with a surprise appearance and helped me through the beginning. We also had Justin, Junyang, Lin from Alibaba and talk about the stuff that they released from Quen. And after the updates part, we also had two deeper conversations at the second part of this show. [00:01:07] Alex Volkov: The first one was with Danish Matthew Abraham. and Paul Gotti from MedArc about their recent paper and work on MindEye2, which translates fMRI images using diffusion models into images. So fMRI signals into images, which is mind reading, basically, which is incredible. So a great conversation, and it's always fun to have Tanish on the pod. [00:01:37] Alex Volkov: And the second conversation stemmed from a recent change in the narrative or a sentiment change in our respective feeds about fine tuning in the era of long context, very cheap models like Claude. And that conversation is also very interesting to listen to. One thing to highlight is this week we also saw the first time GPT 4 was toppled down from the Arena, and we now have the, a change in regime of the best AI possible, uh, which is quite, quite stark as a change, and a bunch of other very exciting and interesting things in the pod today. [00:02:21] Alex Volkov: So, as a brief reminder, if you want to support the pod, the best way to do this is to share it with your friends and join our live recordings every ThursdAI on X. But if you can't sharing it with a friend, sharing a subscription from Substack, or subscribing, uh, to a pod platform of your choice is a great way to support this pod. [00:02:48] Alex Volkov: With that, I give you March 28th, ThursdAI. [00:02:52] Alex Volkov: Hello hello everyone, for the second time? we're trying this again, This is ThursdayAI, now you March 28th. My name is Alex Volkov. I'm an AI evangelist with Weights Biases. And for those of you who are live with us in the audience who heard this for the first time, apologies, we just had some technical issues and hopefully they're sorted now. [00:03:21] Alex Volkov: And in order to make sure that they're sorted, I want to see that I can hear. Hey Robert Scoble joining us. And I usually join their spaces, but Robert is here every week as well. How are you, Robert? Robert. [00:03:35] Robert Scoble: great. A lot of news flowing through the system. New [00:03:39] Alex Volkov: we have, a lot of updates to do. [00:03:43] Robert Scoble: photo editing techniques. I mean, the AI world is just hot and [00:03:48] Robert Scoble: going. [00:03:49] Alex Volkov: A week to week, we feel the excited Acceleration and I also want to say hi to Justin Justin is the core maintainer of the Qwen team. Qwen, we've talked about, and we're going to talk about today, because you guys have some breaking news. But also, you recently started a new thing called OpenDevon. I don't know if we have tons of updates there, but definitely folks who saw Devon, which we reported on, what a few weeks ago, I think? Time moves really fast in this AI world. I think, Justin, you posted something on X, and then it started the whole thing. So you want to give , two sentences about OpenDevon. [00:04:21] Justin Lin: Yeah, sure. I launched the Open Devon project around two weeks ago because we just saw Devon. It is very popular. It is very impressive. And we just think that Whether we can build something with the open source community, work together, build an agent style, or do some research in this. So we have the project, and then a lot of people are coming in, including researchers and practitioners in the industry. [00:04:46] Justin Lin: So we have a lot of people here. Now we are working generally good. Yeah You can see that we have a front end and back end and a basic agent system. So we are not far from an MVP So stay tuned [00:05:01] Alex Volkov: Amazing. so definitely Justin when there's updates to update, you know where to come on Thursday. I, and but also you have like specific when updates that we're going to get to in the open source open source area So folks I'm going to run through everything that we have to cover and hopefully we'll get to everything. [00:05:18] Alex Volkov: , [00:05:18] TL;DR - March 28th [00:05:18] Alex Volkov: here's the TLDR or everything that's important in the world of AI that we're going to talk about for the next two hours, starting now. right So we have a leaderboard update, and I thought this is gonna be cool to just have a leaderboard update section because when big things are happening, on the leaderboards, and specifically I'm talking here about The lmsys Arena leaderboard the one that also does EmptyBench, which is, LLM, Judges, LLMs, but also multiple humans interact with these models and in two windows and then they calculate ELO scores, which correlates the best of the vibes evaluations that We all know and love and folks, Claude Opus is the number one LLM on Arena right now. Claude Appus, as the one that we've been talking about, I think, since week to week to week to week is [00:06:05] Alex Volkov: now the number one LLM in the world and it's quite impressive, and honestly, in this instance, the arena was like, lagging behind all our vibes We talked about this already, we felt it on AXE and on LokonLama and all other places. so I think it's a big deal it's a big deal because for the first time since, I think forever it's clear to everyone that GPT4 was actually beat now not only that, Sonnet, which is their smaller version, also beats some GPT 4's version. and Haiku, their tiniest, super cheap version, 25 cents per million tokens. you literally can use Haiku the whole day, and at the end of the month, you get I don't know, 5 bucks. Haiku also passes one of the versions of GPT 4 for some of the vibes and Haiku is the distilled Opus version, so that kind of makes sense. [00:06:53] Alex Volkov: But it's quite incredible that we had this upheaval and this change in leadership in the LMS arena, and I thought it's worth mentioning here before. So let's in the open source LLM stuff, we have a bunch of updates here. I think the hugest one yesterday, Databricks took over all of our feeds the Databricks bought this company called Mosaic, and we've talked about Mosaic multiple times before and now they're combined forces and for the past. [00:07:17] Alex Volkov: year they've been working on something called DBRX, and now it's we got, in addition to the big company models that's taken over, so cloud Opus took over GPT 4, We now have a new open access model that takes over as the main lead. and they call this DPRX medium, which is funny. It's 132 billion parameter language model. and it's a mixture of experts with I think, 16 experts, and it's huge, and it beats Lama270b, it beats Mixtral, it beats Grock on at least MMLUE and human Evil scores and so it's really impressive to see, and we're gonna, we're gonna chat about DPRx as well and there's a bunch of stuff to cover there as well and Justin, I think you had a thread that we're gonna go through, and you had a great reaction. [00:08:02] Alex Volkov: summary, so we're gonna cover that just today, what 30 minutes before this happened we have breaking news. I'm actually using breaking news here in the TLDR section because [00:08:11] Alex Volkov: why [00:08:20] not? [00:08:22] Alex Volkov: So AI21, a company from Israel releases something incredible. It's called Jamba. It's 52 billion parameters. but the kicker is it's not a just a Transformer It's a joint architecture from joint attention and Mamba. And we've talked about Mamba and we've talked about Hyena. Those are like state space models that they're trying to do a Competition to Transformers architecture with significantly better context understanding. and Jamba 52 looks quite incredible. It's also a mixture of experts. as you notice, we have a bunch of mixture of experts here. and It's it's 16 experts with two active generation It supports up to 256K context length and quite incredible. So we're going to talk about Jamba. [00:09:03] Alex Volkov: We also have some breaking news So in the topic of breaking news Junyang, you guys also released something. you want to do the announcement yourself? It would be actually pretty cool. [00:09:13] Justin Lin: Yeah, sure. Yeah just now we released a small MOE model which is called QWEN 1. 5 MOE with A2. 7B, which means we activate, uh, 2. 7 billion parameters. Its total parameter is, uh, 14 billion, but it actually activates around, uh, 2. 7 billion parameters [00:09:33] Alex Volkov: thanks Justin for breaking this down a little bit. We're going to talk more about this in the open source as we get to this section I also want to mention that, in the news about the Databricks, the DBRX model, something else got lost and was released actually on Thursday last week. [00:09:49] Alex Volkov: We also didn't cover this. Starling is now a 7 billion parameter model that beats GPT 3.5 on LMsys as Well so Starling is super cool and we're going to add a link to this and talk about Starling as Well Stability gave us A new stable code instruct and Stability has other news as well that we're going to cover and it's pretty cool. [00:10:07] Alex Volkov: It's like a very small code instruct model that beats the Starchat, like I think 15b as well. So we got a few open source models. We also got a New method to Finetune LLMs, it's called Lisa if you guys know what LORA is, there's a paper called Lisa, a new method for memory efficient large language model Fine tuning. [00:10:25] Alex Volkov: And I think this is it. Oh no, there's one tiny news in the open source as well mistral finally gave us Mistral 0. 2 base in a hackathon that they participated in with a bunch of folks. on the weekend, and there was a little bit of a confusion about this because we already had Mistral 0. [00:10:43] Alex Volkov: 2 instruct model, and now they released this base model that many finetuners want the base model. so just worth an update there. In the big companies LLMs and APIs, I don't think We have tons of stuff besides, Cloud opus as we said, is the number one LLM in the world. The little bit of news there is that Emmad Mostak leaves stability AI and that's like worthwhile Mentioning because definitely Imad had a big effect, on my career because I started my whole thing with stable Diffusion 1. 4 release. and we also have some Apple rumors where as you guys remember, we've talked about Apple potentially having their own model generator, they have a bunch of Open source that they're working on, they have the MLX platform, we're seeing all these signs. and then, this week we had rumors that Apple is going to go. with Gemini, or sorry, last week, we had rumors that Apple is going to go with Gemini, this week, we had rumors that Apple is going to sign with Entropic, and then now Baidu, And also this affected the bunch of stuff. so it's unclear, but worth maybe mentioning the Apple rumors as well in this week's buzz, the corner where I talk about weights and biases, I already mentioned, But maybe I'll go a little bit in depth that we're in San Francisco on April 17th and 18th, and the workshop is getting filled up, and it's super cool to see, and I actually worked on the stuff that I'm going to show, and it's super exciting, and it covers pretty much a lot of the techniques, that we cover here on ThursdAI as well. [00:12:05] Alex Volkov: In the vision and video category, This was a cool category as well, because Sora for the first time, the folks at Sora they gave Sora to artists and they released like a bunch of actual visual demos that look mind blowing. Specifically Airhead, i think was mind blowing. We're gonna cover this a little bit. [00:12:21] Alex Volkov: If you guys remember Emo, the paper that wasn't released on any code that took One picture and made it sing and made it an animated character. Tencent released something close to that's called AnimPortrait. but Any portrait doesn't look as good as emo, But actually the weights are there. [00:12:36] Alex Volkov: So you can now take one image and turn it into a talking avatar and the weights are actually open and you can use it and it's pretty cool. and in the vision and video, I put this vision on video as well, but MedArk released MindEye 2, and we actually Have a chat closer to the second hour with with yeah, with Tanishq and Paul from AdArc about MindEye 2, which is reading fMRI signals and turning them into images of what you saw, which is crazy. And I Think the big update from yesterday as Well from voice and audio category is that Hume, a company called Hume, demos something called EVI which is their empathetic voice analysis and generation model, which is crazy I posted a video about this yesterday on my feed. you talk to this model, it understands Your emotions. Apparently this is part of what Hume has on the platform. you can actually use this right now but now they already, they showed a 11 labs competitor, a text to speech model that actually can generate voice in multiple emotions. and it's pretty like stark to talk to it. and it answers sentence by sentence and it changes its emotion sentence from by sentence. and hopefully I'm going to get access to API very soon and play around with this. really worth talking about. Empathetic or empathic AIs in the world of like agentry and everybody talks about the, the [00:13:53] Alex Volkov: AI therapist. [00:13:54] Alex Volkov: So we're going to cover Hume as well. I think a very brief coverage in the AI art and diffusion Adobe Firefly had their like annual conference Firefly is a one year old and they added some stuff like structure reference and style transfer and one discussion at the end of the show IS narrow fine tuning done for for large, with larger contexts and cheaper prices for Haiku. we had the sentiment on our timelines, and I maybe participated in this a little bit, and so we had the sentiment and , I would love a discussion about Finetuning, because I do see quite A few prominent folks like moving away from this concept of Finetuning for specific knowledge stuff. [00:14:32] Alex Volkov: Tasks, still yes but for knowledge, it looks like context windows the way they're evolving. They're going to move towards, potentially folks will just do RAG. So we're going to have a discussion about fine tuning for specific tasks, for narrow knowledge at the end there. and I think this is everything that We are going to talk about here. That's a lot. So hopefully we'll get to a bunch of it. [00:14:51] Open Source - [00:14:51] Alex Volkov: and I think we're going to start with our favorite, which is open source [00:15:12] Alex Volkov: And while I was giving the TLDR a friend of the pod and frequent co host Yam Pelleg joined us. Yam, how are you? [00:15:18] Yam Peleg: Hey, how are you doing? [00:15:19] Alex Volkov: Good! I saw something that you were on your way to to visit. our friends at AI21. Is that still the [00:15:24] Alex Volkov: awesome, awesome. [00:15:25] Yam Peleg: 10 I'll be there in 10, 20 minutes. [00:15:27] Alex Volkov: Oh, wow Okay. so we have 10, 20 minutes. and if you guys are there and you want to like hop on, you're also welcome so actually while you're here, I would love to hear from you we, We have two things to discuss. They're major in the open source and like a bunch of other stuff to cover I think the major like the thing that took over all our timelines is that Mosaic is back and Databricks, the huge company that does like a bunch of stuff. They noticed that Mosaic is doing very incredible things. and around, I don't know, six months ago, maybe almost a year ago, they Databricks acquired Mosaic. and Mosaic has been quiet since Then just a refresher for folks who haven't followed us for for longest time Mosaic released a model that was for I don't know, like three months, two months was like the best 7 billion parameter model called mpt and [00:16:10] DBRX MoE 132B from Mosaic [00:16:10] Alex Volkov: Mosaic almost a year ago, I think in May also broke the barrier of what we can consider a large context window so they announced a model with 64 or 72k context window and they were the first before cloud, before anybody else. and since then they've been quiet. and they have an inference platform, they have a training platform, they have a bunch of stuff that Databricks acquired. and yesterday they came out with a bang. and this bang is, they now released the top open access model, the BITS LLAMA, The BITS Mixtral, the BITS Grok1, The BITS all these things [00:16:40] And it's huge. It's a 132 billion parameter MOE that they've trained on I don't know why Seven [00:16:49] Alex Volkov: 12, [00:16:49] Yam Peleg: 12, [00:16:50] Alex Volkov: jesus Christ, 12 trillion parameters. [00:16:53] Alex Volkov: This is like a huge I don't think we've seen anything come close to this amount of training, Right [00:16:59] Yam Peleg: Oh yeah, it's insane. I mean, the next one is six of Gemma, the next one we know. We don't know about Mistral, but the next one we know is six trillion of Gemma, and it's already nuts. So, but Yeah. It's a much larger model. I think the interesting thing to say is that it's the age of MOE now everyone is really seeing a mixture of experts and the important thing to, to pay attention to is that they are not entirely the same. [00:17:27] Yam Peleg: So there is still exploration in terms of the architecture or of small tweaks to the MOE, how to do them, how to actually implement them better, what works better, what is more efficient and so on and so forth. That we just heard about Qwen MOE, which is also a little bit different than the others. [00:17:44] Yam Peleg: So there is still exploration going on and just looking at what is coming out and everything turns out to be at the ballpark of Mistral and Mixtral just makes me more curious. Like, how did they do this? How everything is just on, on the same ballpark as them? How did they manage to train such powerful models? [00:18:04] Yam Peleg: Both of them. And Yeah. [00:18:06] Yam Peleg: I just want to say that because it's amazing to see. [00:18:10] Alex Volkov: So, so just to highlight, and I think we've been highlighting this When Grok was released, we've been highlighting and now we're highlighting This as well. A significantly smaller model from Mixtral is still up there. It's still given the good fight, even though these models like twice and maybe three times as large sometimes and have been trained. So we don't know how much Mixtral was trained on right but Mixtral is still doing The good fight still after all this time which is quite incredible. and we keep mentioning this when Grok was released, we mentioned this. And now when this was released, we mentioned this as well. [00:18:38] Alex Volkov: It's. What else should we talk about in DBRX? Because I think that obviously Databricks want to show off the platform. Nisten, go ahead. Welcome, by the way. You want to give us a comment about DBRX as well? Feel free. [00:18:51] Nisten Tahiraj: Hey guys, sorry I'm late. I was stuck debugging C and it finally worked. I just lost a good time. I used DBRX yesterday. I was comparing it I used it in the LMTS arena. And then I opened the Twitter space and told people to use it. And now it just hit rate limits so you can't use it anymore. Yeah. [00:19:11] Nisten Tahiraj: It was pretty good. I very briefly did some coding example. It felt better than than Code Llama to me. It wasn't as good as Cloud Opus stuff, but it did give me working gave me working bash scripts. So, yeah, in the very brief, short amount of time I use it, it seemed pretty good, so, [00:19:31] Alex Volkov: Yep. [00:19:32] Nisten Tahiraj: that's about it. [00:19:33] Nisten Tahiraj: As for the Mistral and Mixtral question, so, I use Mistral large a lot, I use I use medium a lot, And the 70s, and the Frankensteins of the 70s, and they all start to feel the same, or incremental over each other. It's just the data. It's just the way they feed it. They feed this thing, and the way they raise it, I think it's it's all they're all raised the same way in the same data. [00:20:03] Nisten Tahiraj: Yeah, the architecture makes some difference, but the one thing that you notice is that it doesn't get that much better with the much larger models. So it's just the data. [00:20:20] Justin Lin: That's what I think it is. [00:20:21] Alex Volkov: I want to ask Justin to also comment on this, because Justin, you had a thread that [00:20:24] Alex Volkov: had a great coverage as well. What's your impressions from DBRX and kind of the size and the performance per size as well? [00:20:32] Justin Lin: Yeah, the site is pretty large and it activates a lot of parameters. I remember it's 36 billion and the model architecture is generally fine. Actually, I talked to them a few times. around three months ago, last December introduced Quent2Dem and I accidentally saw it yesterday there are some common senses. [00:20:57] Justin Lin: I think it is really good. They use TIC token tokenizer with the GPT2BP tokenizer. Recently I have been working with LLAMA tokenizer and the sentence piece tokenizer, well, makes me feel sick. Yeah. It's complicated. Yeah, but the GPT BPE tokenizer, because I have been working with BPE tokenizer years ago, so everything works great. [00:21:22] Justin Lin: And we were just, for Qwen 1. 5, we just changed it from the implementation of TIP token to the GPT 2 BPE tokenizer by Hugging Face. It is simple to use. I think it's good to change the tokenizer. And it's also good to have the native chat ML format so that I think in the future people are going to use this chat ML format because the traditional chat formats like human assistant, there are a lot of risks in it. [00:21:53] Justin Lin: So chat ML format is generally good. I think they have done a lot of great choices, but I'm not that, Impressed by their performance in the benchmark results, although benchmarks are not that important, but it's a good indicator. For example, when you look at its MMLU performance, I expect it to be, well, if you have trained it really good. [00:22:19] Justin Lin: I haven't trained a 100 billion MOE model, but I expect it to be near 80. It is just 73 with 12 trillion tokens. I don't know if they repeat the training epics or they have diverse 12 trillion tokens. They didn't share the details, but I think it could be even better. I am relatively impressed by their coding performance, just as Nisten said. [00:22:47] Justin Lin: The coding capability looks pretty well, but then I found that well? [00:22:53] Justin Lin: DBRX Instruct because you can improve and instruct model to a really high level at human eval, but, it's hard for you to improve it for the base model. I'm not pretty sure maybe I need to try more, but it's generally a very good model. [00:23:10] Alex Volkov: Yeah, absolutely. We got the new contender for the Open weights, open source. So the LLAMA folks are probably like thinking about, the release date it's very interesting what LLAMA will come out with. Notable that this is only an LLM. There's nothing like, there's no multimodality here. and the rumors are the LLAMA will hopefully will be multimodal. so whatever comparison folks do and something like like GPT 4, it's also notable that this is not multi modal yet, this is just text. One thing I will say is that they call this DBRX Medium which hints at potentially having a DBLX, DBRX Large or something, and also something that was hidden and they didn't give it, yet they retrained MPT. [00:23:48] Alex Volkov: Yam, I think you commented on this and actually Matei Zaharia, the chief scientist there commented on, on, on your thread. They retrained the MPT7B, which was like for a while, the best 7 billion parameter model almost a year ago. and they said that it cost them like twice less to train the same model, something like this, which I thought it was notable as well. [00:24:07] Alex Volkov: I don't know, Yam, if you want to, if you want to chime in on The yeah. [00:24:10] Yam Peleg: The interesting thing here is that I mean, it's obvious to anyone in the field that you can, making the model much, much, much better if you get better data. So, what they basically say, what they basically show with actions is that if you have, you can even make the model even twice as better or twice as cheaper to train depending on how you look at it, just by making the data better. [00:24:35] Yam Peleg: And my own comment on this is that at the moment, to the best of my knowledge Better, better data is something that is not quite defined. I mean, there is a lot of there is a lot of intuition, there are, I think big things when you look at broken data, it's broken. But it's really hard to define what exactly is better data apart [00:25:00] from a deduplication and all of the obvious. [00:25:03] Yam Peleg: It's very hard to define what exactly is the influence of specific data on performance down the line. So, so it's really interesting to hear from people that have done this and made a model twice as better. What exactly did they do? I mean, because they probably are onto something quite big to get to these results. [00:25:27] Yam Peleg: Again, it's amazing to see. I mean, it's just a year, maybe even less than a year of progress. I think MPT is from May. If I remember, so it's not even a year of progress and we already have like twice as better models and things are progressing [00:25:42] Alex Volkov: Worth mentioning also that Databricks not only bought Mosaic, they bought like a bunch of startups, lilac, the friends from Lilac the, we had the folks from Lilac, IL and Daniel here on the pod. And we talked about how important data their data tools specifically is. and they've been a big thing in open source. [00:25:58] Alex Volkov: All these folks from Databricks, they also highlight like how much Li help them understand their data. very much. so I'm really hoping that they're going to keep Lilac around and free to use as well one last thing that I want to say, it's also breaking news, happened two hours ago, the author of Megablocks, The training library from MOEs, Trevor gale I think he's in DeepMind, he has now given Databricks the mega Blocks library. [00:26:23] Alex Volkov: So Databricks is also taking over and supporting the mega blocks training library for Moes. that is they say out for firms the next best library for Moes as well and there was a little bit of a chat where Arthur Mech from Mistro said, Hey, welcome to the party. And then somebody replied and said, you are welcome and then they showed the kind of the core contributors to the mega blocks library. And a lot of them are, folks from Databricks. and so now they've taken over this library. [00:26:50] AI21 - JAMBA - hybrid Transformer/Mamba Architecture 52B MoE [00:26:50] Alex Volkov: So yes MOE seems to be a big thing and now let's talk about the next hot MOE AI 21. The folks that I think the biggest like lab for AI in Israel, they released something called Jamba, which is a 52 billion parameter, MOE. and the interesting thing about Jamba is not that it's an MOE is that it's a Mamba and joint attention. so it's like a, it's a mamba transformer. Is that what it is? It's a combined architecture. We've talked about state space models a little bit here, and we actually talked with the author Eugene from RWKV, and we've mentioned Hyena from Together AI, and we mentioned Mamba before and all I remember that we mentioned is that those models, the Mamba models, still don't get the same kind of performance and now we're getting this like 52 billion parameter mixture of excerpt model that does. Quite impressive on some numbers and comes close to LLAMA70B even, which is quite Impressive MMLU is almost 70, 67%. I don't see a human eval score. I don't think they added this. But they Have quite impressive numbers across the board for something that's like a New architecture. [00:27:52] Alex Volkov: 50 billion parameters with 12 active and what else is interesting here? The New architecture is very interesting. it supports up to 256. thousand context length, which is incredible. Like this Open model now Beats cloud 2 in just the context length, which is also incredible. Just to remind you Databricks, even though they released like a long context model before Databricks DBRX is 32, 32, 000. [00:28:15] Alex Volkov: This is 256. And not only does it support 256 because of its Unique architecture They can fit up to 140k contexts on a single A180 GB GPU. I know I'm saying a lot of numbers. Very fast, But if you guys remember, for those of you who frequent the pod, we've talked with folks from , the yarn scaling method. and the problem with the context window in Transformers is that the more context you have the more resources it basically takes in a very basic thing. And so the SSM models and the Mamba architecture, they specifically focus on lowering the requirements for long context. and this model gets three times as throughput on long context compared to Mistral. [00:28:57] Alex Volkov: 8 times 7b, compared to Mixtral, basically. so very exciting, yeah, you wanna comment on this I know you're like almost there, meeting with the guys but Please give us the comments, [00:29:07] Yam Peleg: I'm there. I'm there in five minutes, so I can maybe if time works towards favour, maybe I can even get you the people on the pod [00:29:14] Alex Volkov: That'd be incredible. [00:29:15] Yam Peleg: I'm just, yeah, what what is important here, in my opinion, is that first, I mean, absolutely amazing to see the results. [00:29:23] Yam Peleg: But what was not known to this point is whether or not those types of models scale. to these sizes. We had smaller Mambas and they were, they looked really promising, but we were at the point where, okay, it looks promising. It looks like it could be at the same ballpark of transformers, but to test this out, someone need to just invest a lot of money into the compute and just see what the results they get. [00:29:53] Yam Peleg: And it's a risk. You don't know what you're going to get if you're going to do it. And it turns out that you get a really good model at the same ballpark. Maybe slightly less performant as a transformer, but it is expectable. The thing the thing worth mentioning here is that Mamba the Mamba architecture is way more efficient in terms of context size. [00:30:15] Yam Peleg: As you just said, transformers are quadratic in terms of complexity. When you increase the context. So you have if you have two tokens, you need you need four times that you can say the memory. And if you have four tokens, you need 16 and it just goes on and on and it just explodes, which is why context length is such a problem but Mamba scales much more friendly, memory friendly, you can say. [00:30:39] Yam Peleg: So, but the thing is that you do pay with the performance of the model. So. What you, what people do is a hybrid between the two, so you can find some sweet spot where you don't just use so much memory and yet you don't have the performance degrade that bad. And I mean, yeah, it's a risk. At the end of the day, you need to train, training such a large model is a lot of money, is a lot of money in terms of compute. [00:31:06] Yam Peleg: And they did it, released it in Apache 2, which is amazing for everyone to use. And proving for, to everyone that, all right, if you follow this recipe, you get this result. Now people can build on top of that and can train maybe even larger model or maybe even, maybe just use this model. I'm, I didn't try it yet, but I think it's an incredible thing to try because it's it's not the same as Mixtral. [00:31:33] Yam Peleg: Mixtral is a little bit better, but it's at the same ballpark as Mixtral, but you get way more context there. At your home on a small GPU for cheap. It's amazing. [00:31:41] Alex Volkov: and Mixtral specifically, [00:31:43] Yam Peleg: potential. [00:31:45] Alex Volkov: thanks Yamin, I just want to highlight that Mixtral Is this like amazing model that WE compare models like three times the size to it, and they barely beat Mixtral. We talked about this when Grok 1 was released, we now talked about this when DBRX was released with like [00:31:57] Alex Volkov: 12 trillion parameters in data. [00:32:00] Alex Volkov: Mixtral is this basically like the golden standard. We've always had this standard for like how well performing an open model could be and it has been for a while, the best open model that we have and now we're getting this like new architecture, completely new architecture, basically a bet on on would it even scale from Fox from AI21? and it comes close to Mistral, but it does 3x throughput on long contexts compared to mixtral. and it has 256 context window with, if you want to get this from mixtral, You can train it with yarn, you can do all these things, but then you won't be able to actually scale it. hosted because it's gonna cost you so much money because of The quadratic attention. [00:32:33] Alex Volkov: And [00:32:34] Alex Volkov: they specifically say, the only model of its size class, that fits up to 140, 000 context windows on a single GPU. Which is quite incredible. and obviously Apache 2 license is great. I don't know if they also released a bunch of stuff like training code and data stuff. So we're definitely going to keep you posted. [00:32:50] Alex Volkov: And yam hopefully will ask all these questions. But the efficiency in speed where like the closer you get to 128 context, the faster the model kind of performs is also quite incredible. like it. Yeah, it's quite incredible. the graphs there, we're going to post it, everything in the show notes, but absolutely a great release from AI21. shout out AI21 folks and definitely give them our love there and specifically because of the Apache 2 license. Anything else I want to hear from maybe Justin, if you want to comment on the joint architecture anything that you have you guys play with [00:33:20] the joint attention and Mamba. have you what's your reaction to this? [00:33:25] Justin Lin: Yeah, We are trying with members with very small architectures. We can reach similar performance to transformer, but we did not scale it to very large size, so we don't know what will happen. [00:33:38] Alex Volkov: So just this is great and Apache 2, and we're very happy shout out to folks at the i21. Briefly let's cover the rest of the stuff that we have still to cover in the open source. [00:33:47] Mistral base 0.2 [00:33:47] Alex Volkov: We'll briefly cover this in the TLDR. We'll start with Mistral Mistral 0. 2 base released. so for fine tuning, obviously, for folks who know it's better For fine tuning purposes to have a base model than the instruct model, because then you can mistral. [00:33:59] Alex Volkov: 0. 2 base was released in Hackathon last week together with Cerebral Valley and some other friends in San Francisco. [00:34:08] Alex Volkov: There was some confusion about it because we had Instruct 0. 2 before we had a model that said, based on mistral 0. 2 and was like very well performing, the 7 billion parameter one. and now there is the base model. and then somebody went and changed the base of the instruct model to this one versus the previous one but nevermind, they cleared that confusion up and we have this like base model. [00:34:28] Alex Volkov: It's also like open source and it's great. [00:34:30] Nisten Tahiraj: there is one thing here about the previous Mistral instruct that they released. That one has been trained for 32k context, and I used it as as a personal chatbot. I'm making it with just the base the base Mistral 7b, and I'm noticing it is much better at carrying forward a conversation. [00:34:50] Nisten Tahiraj: So I, I think a lot of the fine tunes should probably switch and just rerun on the new Mr. Instruct especially the ones that are geared towards conversational stuff. Because again, Mr. Instruct is limited to eight K and more likely just, you should always just keep it under 4K to get accuracy. [00:35:11] Nisten Tahiraj: So, that's one thing here. The new seven B performs much better at larger contexts and, and summarizing [00:35:18] Starling 7B beta - top apache 2 LLM in the world [00:35:18] Alex Volkov: One incredible news is Starling. And I think I think. Justin, you had both of you and and Yam as well, you guys talked about this. We're starting actually now is a 7 billion parameter model that beats GPT 3. 5 on LMC Serena, which is quite incredible, right? [00:35:34] Alex Volkov: I think it's the first and the only 7 billion parameter model that beats GPT 3. 5 on like user preference. And it's, it was hidden in between the DBRX news [00:35:42] Alex Volkov: but let me see if I can. Put this up here real quick. so this model was released, what, a week ago, a week and a day ago. It's [00:35:48] Alex Volkov: What do we know from this? [00:35:49] Yam Peleg: Yeah, I just want to say, and to go in five minutes, I just want to say about Starling this is the second model. So if you haven't tried yet the first one you definitely want to try. I know there are people that are skeptics about 7b models and saying that they are too small. Just give this one a try. [00:36:10] Yam Peleg: Just give this one a chance. Trust me, just give this specific one a chance. It is an amazing model, seriously, it's an amazing model and it's just showing to everyone that there is a lot more to squeeze out. Scale works, absolutely, but there is a lot more to squeeze out besides scale. And I seriously can't wait for the same technique to be applied on a larger model just to see what we get to. [00:36:35] Yam Peleg: Because it's an amazing result, seriously. [00:36:37] Alex Volkov: Nisten, go ahead. [00:36:40] Nisten Tahiraj: So. The model is is still based and it's actually based off of open chat 3.5. The one thing that their Raven, the Nexus Raven team does well is they had that nexus Raven 13 B model. And for some time that was the best function calling small model you can. [00:36:59] Nisten Tahiraj: So, I haven't tried this one, but I highly suspect it's probably pretty good at function calling. I'm just looking at it right now, it is Mistral based, it's exactly based off of OpenChat 3. 5 from Alignment Lab, so they fine tuned on top of that, and yeah, I would highly recommend people to use it. [00:37:20] Nisten Tahiraj: I've used the one that has been trained off of OpenChat a lot, and [00:37:24] Alex Volkov: They did a bang up job there because this 7 billion parameter model now beats GPT 3. 5, beats CLOUD 2. 1, beats Mistral Next, and Gemini pro and CLOUD 2, like this is the 13th, based on LMsys at least, this is the 13th model, it's 7 billion parameters, it's Apache 2, this is the from Berkeley folks, This is the only Apache 2 licensed model on the LLM leaderboard in the first like top [00:37:48] Alex Volkov: 20, I think, or top top 13. So it bigs, I don't know how it beats Mixtral. So anyway, yeah, StarLing is great. It looks great Try it, folks. Definitely worth mentioning. We're gonna run through some other updates because we still have a tons of stuff to cover and then we Have some guests here in the audience that want to join and talk about very interesting things [00:38:05] LISA beats LORA for AI Finetuning [00:38:05] Alex Volkov: I don't have a lot of information about Lisa specifically, but I will just mention that there's if you guys in the fine tuning area, you know that Laura and we have Laura in the diffusion? models area as well lower rank adaptations, so folks in the Diffusion world have been training LORES for a while, more than a year, and now there's a new paper dropped that's called a new method for memory efficient large language model fine tuning. [00:38:27] Alex Volkov: I'll say this slowly a new method for memory efficient large language model fine tuning. So this is not for diffusion stuff this is for large language, it's called Lisa and achieves better performance than LoRa with less time on models up to 70 billion parameters, and yeah, the results look pretty cool for folks who do fine tuning, it's worth comparing this and I know for a while we had different methods for fine tuning like QLora, for example, different Lora, there was an attempt to figure out which one is the best and so Lisa now is a new contender with a paper out and I think code will follow up as well. [00:38:59] Alex Volkov: Lisa can fine tune models up to 7 billion parameters on a single 24 gigabyte GPU. so you can fine tune 7 billion parameter Mistral, for example, on a 4090 with a 24 gigabyte GPU, which is pretty cool. [00:39:13] Alex Volkov: And code implementation in LMFlow is very simple. so awesome to have this and we'll add this to the show notes for folks who actually do fine tunes. And it's gonna be awesome. so I think that covers all of the open source stuff, and we obviously spent almost an hour running through open source and I do want to move towards What is the next super exciting stuff that we have this week before we jump into a conversation. [00:39:37] Hume EVI emotion based TTS [00:39:37] Alex Volkov: Yes I want to move into Hume. I want to move into the voice, and audio category. This is an unusual jump between categories. we usually talk about big companies next but there's honestly not that much that happened there. So maybe we'll briefly cover it, but the thing that broke my mind, I'm going to paste this on top here. and hopefully you guys will just listen to me instead of going and watching This is that a company called Hume finally released something that many people have been very excited about. and they showed a few demos there and they finally released something. so Hume has been around for a while. [00:40:08] Alex Volkov: Apparently they do emotion analysis very well and they actually have this product out there. you can upload the video and actually audio of yourself speaking and they will and understanding of what you're saying. of your emotions and intonations, which is pretty cool. and we know that's a piece that's missing from multimodal LLMs, right? Okay, so Hume, they already had a platform for emotions understanding, and yesterday Hume released their demo of an emotional TTS, a text to speech model that not only speaks This text position actually replies with emotion. and combined with the previous system. that they had that they can understand your emotion, as you can hear, I'm talking about this I was a little bit sad when Hamel had to drop, but now I'm very excited again to talk to you about Hume. so they actually have a running analysis of this voice as it runs. and they understand what kind of like where you are in the emotion scale, which is, first of all, exciting to see on yourself. Second of all, it's like very alarming. Their understanding of emotions, whether or not it's like precise enough to tell the truth, for example. and the text to speech of theirs that generates emotion based text is quite something. I've never seen anything close to it before the only thing that came close to me is that if you guys remember, we talked about 11 labs have style transfer thing where you can actually talk and they would take an AI voice and basically dub you but with the same emotion. So, that was the only thing that came close to what I heard yesterday from Hume. so hume has this model that's gonna be out in I think they said April? [00:41:40] that you'd be able as a developer to assign what emotion it will answer with. and together with the first part, which is a voice emotion understanding, like the text to speech understanding, they now have a speech to text with emotion. the whole end to end feeling is like nothing I've ever experienced and Robert I think I saw you first repost about this So I want to hear if like you play with the demo and like what your thoughts about this Because I was blown away and I will definitely want to hear about What do you think about this? [00:42:14] Robert Scoble: blown away too. They you nailed it. It lets AI understand your emotion and build a much more human interaction with AI. The one problem is, I believe it's 7 an hour or something like that, so it's fairly expensive to integrate, but, for people who are building new kinds of applications that are going to have to integrate with human beings, I think it's very well done. You should look at it. [00:42:41] Alex Volkov: Absolutely and definitely for folks who have the Uncanny Valley in different, LLMs that, reading for a long time is not the same. Is not quite the same I think we're gonna see some more emotionality in many of these, demos, and it's gonna be very exciting, together with the fact that recently there has been like this video of basically HeyGen the deepfake company that translates your lips and people were saying, Hey, this is like a fully end to end AI and we're so doomed all of these kind of AI generated voices, they still use 11 labs so I got to think that 11 labs is not going to be like that much behind and we'll start Working on some emotion like output as well but I would definitely add the link to this, and actually the video of me testing this out Hume, in in the show notes, and more than welcome for you guys to try this as well. [00:43:27] Alex Volkov: I think the demo is demo, oh huma. ai. They actually have a chatbot on the website? hume. ai, where you can talk to the chatbot in your voice, and answers with voice as well but the full demo is more mind blowing. They understand your emotionality, they understand the emotionality of they then translate the emotionality into the actual context. and when the model talks back at you and when you say something like when you try to be when you try to fake it, and you yell, but you say, I'm so happy the model says, Hey, you look a little bit conflicted. So actually understand like what you're saying and what your meaning or basically the way you say it is different. [00:44:00] Alex Volkov: So they actually build this understanding into the demo, which is super cool to play with. Yeah, so hume definitely worth checking out. I think that next in the voice and audio, I think that basically that's it that we had to cover but a similar area in AI creation is vision and video. [00:44:15] SORA examples from filmmakers [00:44:15] Alex Volkov: And this week we had oh my God the beginning of this week was like all excited about how to how the world of entertainment will look and the reason is because OpenAI took Sora, I'm hoping by this point that Sora is needs no introduction at this point right Sora is OpenAI's text to video model, and it's leagues above everything else that we saw in the world before this and it blew our creative minds, and keeps blowing some people's minds on TikTok. and OpenAI gave access to Sora, to a few creators content creators not Hollywood Apparently they're on the way to Hollywood right now and to talk with folks, But they gave it to a few filmmakers in in the like independent world I think a few Companies from Toronto and they finally showed us demos of what. [00:45:03] Alex Volkov: Instead of The developers in OpenAI, and some prompts that they do with Sora, what an actual studio can do with some creativity and it looks like they also hired an artist in residence for OpenAI as well and wow my mind was definitely blown. the there was one short video that looked like something that I would, I would have seen in Sundance festival. It's called Airhead from from Toronto based. film [00:45:28] Alex Volkov: creator called ShyKids, and I'm gonna add this to the show notes because this definitely, at least for me, was the most viral thing that I saw. And, I Absolutely loved it. It was, it felt very human it felt incredible. It's this very short story about something, somebody with a balloon instead of his head and the way they tell the story they kind of work around the technical limitations, which we all know, right if you generate two videos in Sora, the first the character persistence between those two videos will Not be there. And that's a big problem with every video generation. But this one, they worked around this because they told the story of this air balloon guy and his head throughout their life So like the character consistency isn't really required there. And I just really love that like actual storytellers can work around the technology to create something that feels so good Obviously the audio there was amazing and the production and the storytelling, everything. So. I think everybody saw it at this point, but if you haven't airhead from shy kids is quite incredible. [00:46:27] Tencent AniPortrait - Animated Avatars [00:46:27] . Okay. , I want to talk about Tencent released something called AniPortrait any with N a N I like animated portrait and it's generating photorealistic animated avatars. and if you guys remember Emo, we've talked about this before Emo was quite incredible to me. the examples that Imo showed were pretty much the same level, the same jumping capability in the way that Sora showed the previous image to video generations, Imo showed to kind of Image to animated character and [00:46:56] Alex Volkov: was incredible. [00:46:56] Alex Volkov: Like lips moved and eyes and consistency was there. So, the problem with Emo is that they haven't released the code And I think for now Emo is the highest like AI GitHub repo with the highest number of stars with no code. I think it's like 25, 000 stars or something. Everybody's waiting for Emo and haven't dropped. [00:47:15] Alex Volkov: And when I say everyone, I necessarily mean the kind of the waifu creator world who would love, nothing more than just generate an image in stable diffusion something and then animated this with some, let's say emotional voice from the human thing, that we just mentioned. but the second best one for now is AnyPortrait. and actually the code was dropped. and the kind of the lips movement is great And the eyes, it's not close to emo, but it's really good compared to WAV to leap on different areas and if you ever built like an animated character AI stuff, you'll know that, the open source options. [00:47:49] Alex Volkov: were not great the closed source options like HeyGen and different like labs like DID and Synthetic, I think, I don't remember the name. They were okay. They were great. but the open source options were not there. So any portrait right now is the best version We have it dropped yesterday. if you are doing any kind of like character animation, give any portrait a try and let us know, I'm definitely gonna play with this. [00:48:12] Alex Volkov: Definitely gonna play [00:48:12] Alex Volkov: with this. I think we've covered most of the stuff that we wanted to cover besides weights and biases stuff and NB companies. [00:48:18] MindEye 2 - Interview with Tanishq and Paul from MedArc [00:48:18] Alex Volkov: But now I am very excited to bring two friends here one friend of the pod and for a long time and now a new one, Paul Scotti and you guys here to talk to us about MindEye. to the second version. so I will just like briefly do an introduction that MindEye came around the summer I think I want to say, and we covered this because in my head everything was like multimodal, multimodal. When were we going to get multimodal? This was before vision happened. and one of the craziest multimodalities that we expected was something like a fMRI signal, like brain signals. and then you guys raised MindEye, which was like, mind blowing. and so I would love to hear about the history of like how Med Ark started like doing brain interpretation. and then let's talk about MindEye 2 and what's exciting about this recent release, but feel free please to unmute Tanishq and then Paul and introduce yourself briefly. [00:49:08] Tanishq Abraham: Yeah Yeah, I'll just provide a quick background and summary and then I'll let Paul talk about MindEye 2 in more detail. But, yeah, basically, I'm introducing myself again. I'm Tanish I'm Tanish. Work at Stability ai and I also am the founder of MedARC and I lead MedARC, which is a medical ai open source medical ai research organization. [00:49:30] Tanishq Abraham: And, we mostly are focused on trading foundation models for medicine. And So we do have a kind of a kind of research in neuroscience and ai and combining AI and neuroscience, which. which is what Paul is leading at MedArc. But yeah, like we started I guess looking into this sort of neuroscience AI research for quite some time actually. [00:49:54] Tanishq Abraham: Actually, I think even before I officially started MedArc when I was organizing [00:50:00] some open source medical AI projects, this was one of the projects that I actually had started, I think, back in summer of 2022. And I think, just generally, the idea was that there's, the idea was we were working on this fMRI to image reconstruction problem, which is basically the idea that we take the, we have a person that is looking at some images and we take their fMRI signal. [00:50:25] Tanishq Abraham: and we want to use AI to reconstruct the image that the person was looking at just in the fMRI signal. So it's the sort of mind reading kind of problem that we're working on. And I think up, back in 2022 when we started working on this, at first no the techniques that people were using were quite basic and, I think the sort of neuroscience community was quite behind in what they were, in what they were using. [00:50:48] Tanishq Abraham: So I think we were pretty excited about the possibility of utilizing some of the latest techniques in generative AI to advance this field. And yeah, first did I start this project and there were a couple volunteers that were helping out, but luckily Paul had also discovered that we were working on this and he, he joined this project and really spearheaded this kind of neuroscience AI initiative that we've been having at MedArc. [00:51:14] Tanishq Abraham: And yeah, that resulted in MindEye, which we released in April. I think May of last year and and then we've been continuing to work on improving those results and that has now resulted in MindEye 2. And we also have some other sorts of projects in the neuroscience AI area, like training foundation models for fMRI and we're exploring some other ideas as well. [00:51:37] Tanishq Abraham: But yeah, I think with MindEye one, we had a very simple sort of pipeline of. of taking the fMRI signal and converting them to clip image embeddings and and then basically re generating an image from the clip image embeddings, and that worked quite well and The only difference, the only issue with that was that it required a lot of data, and we have developed this new pipeline, which Paul will talk more about, that requires less data, is more efficient, and is giving also better results with better, sort of, image generation models, so, for example, we're using SDXL for this MindEye 2 model so, yeah, I think I'll let Paul talk more about the motivation and how MindEye 2 works. [00:52:18] Alex Volkov: So I just like before we get to Paul thank you for joining guys. first of all, I just want to highlight how insane to me is the thing that you guys talking about where many people like think that, oh yeah, generative AI generates images. Yeah. And generate some texts. And You guys like translating brain signals into what people actually saw. and I think I saw a separate from You also an attempt to understand fMRI. So Paul, maybe feel free to introduce yourself and maybe also cover prior work in this area. I would love to know, if this is something you guys came up with or something You saw and improved on, I would love to know as well. [00:52:52] Alex Volkov: That's [00:52:57] Paul Scotti: This, yeah, like Tanisha was saying, we started out working on this together over Discord back in 2022. And at the time, there weren't really any good results doing reconstruction of images from, looking at images inside of an MRI machine. And what really spurred several new papers in this field is open sourced image generation models like stable diffusion clip models, and also importantly a good data set of people looking at images in an MRI machine. [00:53:34] Paul Scotti: It's a very difficult dataset to collect because we're talking about eight people who spent 30 to 40 hours inside of this MRI machine looking at images one at a time for three seconds each. [00:53:48] Paul Scotti: So it's, it really was the culmination of dataset and new models that allowed this to work. For the MindEye 2 stuff specifically, We focused on trying to get good results using only one hour instead of 40 hours of data. [00:54:07] Paul Scotti: And this is pretty important because if you're trying to do these machine learning techniques on new subjects, new data sets, maybe apply to the clinical setting, you aren't going to be collecting dozens of hours of data, especially for clinical populations. It's just too expensive and you're taking up their valuable time. [00:54:29] Paul Scotti: So we, there's a lot of papers now that have been focusing on fRIDA image, just because it's a cool topic. So our paper shows, state of the art results, but specifically in the one hour domain, We show that you can pre train a model on other people's brains in order to have a better starting point to fine tune the model on a separate, held out subject's brain. [00:54:54] Paul Scotti: And for people who aren't maybe as familiar with neuroimaging stuff or how the brain is, how the brain works, your brain is wired very differently to other people. It's not like there's the same. part of the brain that always handles, what happens when you look at a picture of an elephant or something. [00:55:15] Paul Scotti: We have different shapes and sizes of brains. We have different patterns of activity that lead to how we perceive vision. And the reconstructions that we're talking about are not as simple as just, was it a dog that you were looking at? Was it an elephant? So you need some sort of way to align all these different people's brains and their different visual representations into a shared latent space so that you can then get the rest of this pipeline with the, diffusion models and MLPs to work and actually have that be informative to generalize from, my brain to your brain. [00:55:53] Alex Volkov: so incredible that I have, so many questions, Paul, but I will start with maybe, The differences between brains that something that you said, I also want to talk about, the visual cortex and how that thing happens, but I would be remiss if I don't mention at least that you guys are now talking about MindEye at the same time we're we got the first the first Neuralink implanted human showing that he can control basically a machine with their, with his brain with implants. [00:56:19] Alex Volkov: But you guys are completely non invasive kind of understanding of these brain signals. But to an extent and Neuralink also is some sort of like an invasive understanding of brain signals and transforming them into actions versus something that they see. but , they mentioned that they're working on sight fixing. [00:56:34] Alex Volkov: As well. [00:56:34] Alex Volkov: Could you maybe give us a brief understanding of fMRI, how that translates into the signals from visual contact? How do, how does this machine know what I see and how then you are able to then use diffusion models to recreate what I see. [00:56:48] Alex Volkov: Could you give us like a little bit more of a, what's, where's the magic here? [00:56:52] Paul Scotti: Yeah, so, fMRI right now is the best method if we're talking about non invasive tech. If you have electrodes on someone's brain, obviously that's going to give you a much better signal. But it's also not viable to do that for most projects and for applying it to clinical settings and new research and everything. [00:57:14] Paul Scotti: So we used fMRI, which is a bit crude in the sense that you have these people that are needing to make as little motion as possible. The MRI machine is basically tracking blood flow. So when you look at an image of something, the neurons in your brain that correspond to representing that image are active and they require more oxygenation to help with how they've been used in relation to the other voxels in the brain that are not as relevant for activating to that image. [00:57:50] Paul Scotti: Basically, you're tracking this kind of slow moving time course of blood flow that corresponds to where in the brain is active. And then you are have this 3D volume of the brain and the corresponding blood oxygenation changes for every given 3D cube or voxel in the brain. And what we did is we took all the voxels corresponding to the visual cortex, The back of the brain that seems to be active when you look at stuff, and we feed that through this neural network.[00:58:20] [00:58:20] Paul Scotti: And specifically, we feed that through MLPs and a diffusion prior and all this stuff to give us a model that can translate from brain space to clip space. where CLIP is, these models that are contrastively trained typically with text and images So that you can have this multimodal space where you have the ability to align a given image caption with the image itself. [00:58:48] Paul Scotti: This you can think of as a third space, a new modality for CLIP that's the brain. So we use the same sort of technique of contrastively mapping the brain and its paired samples corresponding to the images into the CLIP space. And then there are so called unclip models, also sometimes called image variations models, that allow you to undo clip space back to pixel space. [00:59:13] Paul Scotti: And so that's how we actually get the image reconstructions at the end, where the model only gets the brain activities and has to generate the corresponding image. [00:59:23] Alex Volkov: So I'm still like picking up my job from the floor here, because what you're basically saying is this, the same architecture that is able to Drop cats by understanding the word cat and like a pool, the concept of a cat from latent space. Now you've able to generalize and add multimodality, which is like brain understanding of a cat or like what happens in the brainflow in the visual cortex when somebody looks at a cat and you're basically placing it in the same latent space neighborhood. and now you're able to reconstruct an image based on this. I'm still like trying to obviously wrap my head around this but I would love to maybe ask. [01:00:01] Alex Volkov: the Tanishq as well. , could you talk about MindEye 2 and specifically the improvements that you did, and how you achieved them and what they are in fact and then how it applies to the clinical field, [01:00:11] Tanishq Abraham: Right. I mean, so with MindEye 2 like Paul mentioned, our main focus was what can we do to basically use less data when it comes to a new subject. So if you have a, you have a new person that you want to, read their mind, you want to do this reconstruction, we don't want them to have to do 40 hours of scanning because with MindEye 1, you'd have to basically train a separate model for every single subject. [01:00:34] Tanishq Abraham: So it was like a completely separate model for each subject. So if you had a new subject, you would have to get 40 hours of scanning with that new subject to create a new model. So [01:00:42] Tanishq Abraham: the idea with MindEye 2 is that we have, [01:00:45] Tanishq Abraham: We, we train, [01:00:46] Tanishq Abraham: A model on all of [01:00:48] Tanishq Abraham: The previous subjects. [01:00:50] Tanishq Abraham: So for example, we have [01:00:51] Tanishq Abraham: Eight subjects in the data set, [01:00:53] Tanishq Abraham: You train on seven of the subjects, [01:00:56] Tanishq Abraham: And [01:00:56] Tanishq Abraham: You, and so it's training on all seven subjects and then you are able to then fine tune. that model on a new subject, but you only need one hour of data. [01:01:06] Paul Scotti: So basically for any new subject, now you only need one hour of data. [01:01:09] Paul Scotti: So the way that works is that basically we have adapter layers, which is just like these sorts of like linear layers that you have that for each each subject. So, you basically have this sort of layer that is you have the fMRI data from a new subject, but you do have this like linear adapter layer that is basically converting it to again like a kind of a shared space for all the fMRI data. [01:01:32] Paul Scotti: So then basically when you are taking a new patient or a new subject, all you have to do is fine tune this linear adapter for that new subject. And, yeah, so that's the general idea with. What we try to do there with that way, we only have to use only one hour of data. [01:01:49] Paul Scotti: But then on top of that, of course, we have various modifications to the entire pipeline that also just gives you better results overall. So for example instead of in the past, when we were taking our clip image embedding and then reconstructing We used a different model called Versatile Diffusion, but here what we did is we actually took SDXL, and the problem with a model like SDXL, for example, is that it only takes in clip text embeddings. [01:02:19] Paul Scotti: So because, these models are text to image models, so oftentimes a lot of these models are going to be taking, they're taking like clip text embeddings, and that's what they're conditioned on. But here, what we did is we fine tuned SDXL to instead be conditioned on clip image embeddings, and so we have this SDXL unclipped model, that's what we call it and so that, is one, for example, improvement that we use this model instead of the previous model, which was versatile diffusion. [01:02:42] Paul Scotti: There are a few other like different improvements to the architecture, to the conditioning that we have. I think Paul can again, talk more about that, but I think the main kind of innovation Apart from, this is just the general improvements. I think the main innovation is the use of this sort of adapters for? [01:02:59] Paul Scotti: Each subject that allows us to then fine tune for new subjects with only one hour of data. and [01:03:05] Paul Scotti: Paul, I feel free to add any other details as well, Paul. [01:03:08] Alex Volkov: Yeah. I want to follow up with Paul specifically around you're moving from 40 hours to let's say one hour, one hour still in this like fMRI, basically a coffin, right? like it's a huge machine, like it's super incredibly expensive so the data, the it's not Maybe I'm actually going to presume here, but maybe please correct me if I'm wrong. [01:03:26] Alex Volkov: Unlike other areas where like synthetic data is now a thing where people like actually improve Have you guys played with synthetic data at all? is that something that you've tried and seems helpful? Or is this like something that actually humans need to sit in those machines and provide some data for [01:03:40] Alex Volkov: you? [01:03:42] Paul Scotti: Yeah, I mean, to an extent you need real data to validate things, but we have done augmentation to, which is like synthetic data. to make the models more. Robust, right? So like we've played around with, averaging samples from different images together, doing mix up kind of data augmentations to make the pipeline work better and for some other projects that we're doing that might be involving more synthetic approaches. [01:04:16] Alex Volkov: Awesome. And so I think I'll end with this one last question is the very famous quote from Jurassic Park is that scientists were preoccupied thinking if they could, they didn't stop thinking if they should, but not in this area. I want to ask you like specifically, what are the some of the applications that you see for something like this when you guys get to MindEye 3 or 4 or 5 and it's maybe with different signals, maybe with EEG, I don't know, what are some of the implications that you see of like being able to read somebody's mind and what can it help? [01:04:47] Alex Volkov: with? [01:04:49] Paul Scotti: Yeah. So, you want, yeah, you can go ahead, Paul. Okay. You, yeah. Okay. So, like there's just so many different directions, right? Like you've got right now we're focusing on perception, but the more interesting thing would be mental imagery, like dream reading applying these models to real time so that you can reconstruct while they're still in the scanner that allows you to do cool new experimental designs as well. [01:05:15] Paul Scotti: You could look at memory, try to reconstruct someone's memory for something. Yeah, Dinesh, maybe you can add on to that. Yeah. So, [01:05:26] Tanishq Abraham: the thing is, what's really interesting is that a lot of the sort of, [01:05:28] Tanishq Abraham: Pathways and activity for, [01:05:30] Tanishq Abraham: Perceiving an image that you're looking at right now, a lot of them are similar for [01:05:33] Tanishq Abraham: Imagining and dreams and these sorts of things. [01:05:35] Tanishq Abraham: So of course there are some differences, but that's the thing is that a lot of these pipelines should hopefully be, [01:05:41] Tanishq Abraham: Generalizable to some of these other applications like, [01:05:44] Tanishq Abraham: Reconstructing what you're imagining and things like this. [01:05:46] Tanishq Abraham: And in fact, there are there is some work in this already. [01:05:49] Tanishq Abraham: There's like a paper from one of our collaborators that may be coming out in a couple months that is exploring this. So it's actually not just limited to. what you're looking at, but you know, more generally as well. But I think just even with this technology that we have with what you're looking at and reconstructing that, I think there's lots of interesting like clinical applications. [01:06:08] Tanishq Abraham: For example maybe, the way you perceive is associated with your mental condition. So maybe it could be used for different biomarkers, different diagnostic applications. So for example, if you're depressed, for example, maybe you are going to perceive an image. [01:06:21] Tanishq Abraham: in a more dull fashion, for example. And so I think there's a lot you can learn about how the brain works by looking at how people are perceiving it perceiving images, and also utilizing that for potential clinical and diagnostic applications. So that's also an area that is completely underexplored. [01:06:39] Tanishq Abraham: [01:06:40] And it's been also pretty much underexplored because people weren't able to get such high quality reconstructions before with, I think the introduction of MindEye 1 was like one of the first times that we were able to get such high quality reconstructions. And of course, even then, we had to use the 40 hours of data to do that. [01:06:56] Tanishq Abraham: And now we're actually bringing it down to one hour of data. And with further work, we may be able to bring out, bring it down even further. So now we're actually potentially having it's actually, potentially possible to use this for actual clinical applications. And so that is what I'm most excited in the near term in potential diagnostic applications or for potential neuroscience research applications. [01:07:17] Tanishq Abraham: And then of course, long term vision is trying to apply this for, looking at imagination, dreams, memory. That's, I think, the long term vision and interest there. So that's at least how I see this field progressing and what I'm interested in personally. One, maybe just one more quick nuance is that with the shared subject stuff, it's not limited necessarily to reconstructing images. [01:07:41] Tanishq Abraham: So typically, machine learning approaches, you need a lot of data, but data takes a lot of time in the MRI machine. And so this approach of using other people's brains as a better starting point allows clinicians to potentially use more complicated ML pipelines for investigating the brain, maybe even outside of image reconstructions, in a way that's feasible given the time commitments that scanning entails. [01:08:11] Alex Volkov: I absolutely loved, the first thing you said, Paul, that, if we get to real time as the person in the machine, that some stuff, some understanding, interpretation of what they're going through could happen as well. That's extremely exciting. And at [01:08:23] Alex Volkov: the rate of where Junaid is going I'm, I'm positive that This is possible and I'm very happy that you guys are working on this and are excited about building like improvements on this the jump from 40 hours to one hour seems incredible to me? And if this trend continues, definitely exciting possibilities. Thank you guys for coming up. Maybe let's finish on this what are you Restricted on from going forward. Is it like compute? Is it data? is it talent Maybe you want it like shout out. Maybe you're hiring. Feel free. The stage is just like what else is needed to get to MindEye 3 faster [01:08:56] Tanishq Abraham: Yeah, I think it's mostly manpower, I guess, I mean, I think it's, mostly relying on volunteers and, Paul, of course, is doing a great job leading this so that I think is the main limitation and of, but of course, yeah, like with MedArc, we are doing everything, open source and transparently so we, we have a Discord server where we organize all of our Our our research and progress and well, we have all the contributors joined. [01:09:20] Tanishq Abraham: We, I mean, we've been lucky to have amazing contributors so far, from Princeton University of Minnesota University of Waterloo, from all around the world, we've had people contribute, but of course, more contributors are better, of course. And, if you're interested in this sort of research. [01:09:35] Tanishq Abraham: Please please join our Discord, and of course feel free to, to read the papers as well and follow us on Twitter we'll be updating our progress on Twitter as well but yeah I think Yeah, just, check out our Twitter and join our Discord, I think is the main one. [01:09:49] Tanishq Abraham: But yeah, [01:09:50] Alex Volkov: absolutely. And thank you guys for coming up. I'm very happy that I was able to talk to you guys. Cause last time when you raised my hand, I was like, Oh, this is so cool. I know the niche, but yeah, back then we weren't bringing you up. So Paul, thank you It's great meeting you and you guys are doing incredible work and [01:10:03] Alex Volkov: I think it's very important. [01:10:04] Alex Volkov: I'm very happy to highlight this as well. Now we're moving to something a little bit different. [01:10:08] Alex Volkov: Let's reset the space a little bit, and then let's talk about fine tuning. [01:10:24] Alex Volkov: All righty. ThursdAI, March 28th, the second part of the show. If you just joined us, we Just had an incredible conversation with Paul Scotty and Tanishq Abraham from MedArk and I guess stability, part of stability [01:10:43] Alex Volkov: as well. and we've talked about AI reading your brain and understanding what you saw, which is incredible. [01:10:48] Alex Volkov: And I definitely recommend listening to this if you just joined in the middle or or just joining us late Meanwhile, we also covered a bunch of open source stuff so far. We also covered that cloud Opus is now taking over as the number one LLM in the world right now, and something we all knew, but now LMC Serena is catching up? We also had a bunch of breaking news and I wanna just reset the space and say that, hey, for everybody who joined us for the first time this is ThursdAI. we talk about AI every day. everything that's important and impactful in the world? [01:11:18] Alex Volkov: of AI from week to Week and we've been doing this for more than a year. and you're more than welcome to join us in the conversation in in in the comments as well. We're reading through those. And if you're late to any part of this is released as a podcast episode on every [01:11:33] Alex Volkov: podcast platform. So you're more than welcome to follow us on Twitter. Apple and Spotify and whatever you get your podcast. and also there's a newsletter with all the links and videos and everything we talk about that you have to actually see, right? So a link to the MindEye paper will be in the show notes and the newsletter as Well [01:11:48] This weeks buzz - WandB in SF in April [01:11:48] Alex Volkov: I will also say that my actual job is an AI evangelist with Weights Biases, a company that builds tools for all these model creators to actually track their experiments. and Weights Biases is coming to San Francisco in April 18th and April 17th. we have a conference there. You're, if you're in the area or you want to fly in and meet like a bunch of folks in San Francisco, you're more than welcome to use this as your Reason and opportunity I think for the next few days [01:12:15] Alex Volkov: the tickets are still early bird and it's 50 percent price we're doing a workshop on April 17th about improving your business with LLMs. And we're doing everything from prompting to evaluation and doing a bunch of very exciting conversations. So if you're in the area, please stop By and, high five me. I'm going to be in San Francisco for the whole week. and moving on here. I want to chat about finetuning, and I see LDJ here. [01:12:36] Discussion : Is finetuning still valunable? [01:12:36] Alex Volkov: I think we've covered pretty much everything important unless there's breaking news and hopefully folks will DM me If there are breaking news there has been a sentiment in in at least, in our little bubble of AI, On X, where some folks started to get a little bit disillusioned with the concept of Fine tuning. and I don't think the disillusionment necessarily is with the concept of fine tuning as a concept I think the kind of the general vibe of getting and I think some folks like Ethan Mollick and Anton Bakaj was like a folk folks we follow for some like information. [01:13:07] Alex Volkov: The disillusionment stems from the fact that we previously covered that long context Windows maybe affect like rag for example, RAG use cases, but long context window could also affect finetuning, because if you get something like a Haiku, which is now the world's like fifth or sixth, LLM in the world but it costs 25 cents a million tokens, and you can send a bunch of examples into Haiku for every request you maybe you maybe not needing to fine tune? and so this has been a little bit of a sentiment and also the bigger models they release like the recent Databricks model is huge and it's really hard to fine tune you have to like actually have a bunch of hardware so we've seen the sentiment and I really briefly wanted to touch with LDJ and Nisten and Junyang and Tanishq also like everybody who's on stage feel free to chime in and from the [01:13:55] Alex Volkov: audience. [01:13:56] Alex Volkov: If you're friends of the pod, do you want to come up and talk about fine tuning? Let's talk about this sentiment. LDJ, I saw your question. Yes, we've covered Jumba in the beginning. We're very excited. I think Jan was here and now he's talking to actual AI21 folks. So I want to do this like fine tuning conversation. [01:14:09] Alex Volkov: LDJ, we briefly covered this and we said, Hey, it would be awesome to just chat about this like face to face. So what's your take on this recent sentiment? What are you getting from this? [01:14:18] LDJ: yeah, I guess when it comes specifically to, I guess, like the business advantage of fine tuning for a specific use case to try and have a cost advantage over open AI models or something, I feel like things might be changing with Haiku and, I mean, you talked about this before It was either you or somebody else posted like a chart of the average trend of the cost for like how good the model is and Haiku is breaking that trend of it's like Really good while being like significantly cheaper than it should be given the previous trends [01:14:53] Alex Volkov: think that was Swyx. Let me go find it Yeah. [01:14:56] LDJ: Yeah and yeah, I think just overall for a lot of things that [01:15:00] people would have fine tuned open source models for, Haiku, it just might make sense to use Haiku, and it might be able to do those things that you would fine tune for anyways better or equal, and at the same time be really cheap already to run. [01:15:14] LDJ: And I think it definitely The amount of tasks that it makes sense to fine tune on from an economic point of view, it's just probably less tasks now than before and I guess that is probably going to get less as a closed source becomes more and more efficient. [01:15:32] Alex Volkov: Yeah, so absolutely there's a [01:15:33] Alex Volkov: few areas for which fine tune is a concept even, right? There's like the general instruction fine tuning you take a base model, you try to make it more helpful. but there's also fine tuning for more knowledge, for example, that I think and maybe you guys can correct me on this and feel free to step in here, Junyang as well Is that the kind of the knowledge fine tuning the like giving this model like more information? [01:15:56] Alex Volkov: sometimes suffers from stuff like catastrophic forgetting that the model like starts to forget some other stuff. [01:16:02] Alex Volkov: But also things like RAG, for example, are potentially helping in that area where you can actually have a a sighting of a specific source that the model like referred onto, which is very important especially in the enterprise and companies area right like when you want to build something like a assistant or something like retrieval or something like search or better search you actually don't want to count on the model's hallucinations potential. you want to cite something. So for knowledge retrieval, RAG seems to be at least in the companies and enterprise area RAG seems to be like winning over Finetuning. and then the question is RAG over a Finetune model for your specific stuff better than RAG over a general model with a huge context? and I think that this is the area of disillusionment, specifically around the cost of pulling everything back and I think previously context window was very not cost effective We briefly mentioned this today in the area of Jamba models where Context is now like cheaper with those models, but for a regular Transformer LLM, context is expensive. [01:17:04] Alex Volkov: The more context you have, The kind of, the more the hardware requirements grow and so I think that some of the kind of disillusionment especially comes from that. some of it is probably also related to how Big the models have gotten. I don't know, Nisten, if you want to chime in on this or like how even the Grok one the model was huge. people were like getting excited, but then some folks like Technium from Nous Research, like I said, we won't even try to fine tune this for even Instruction, because it's just too big so I wanted to hear from Nisten, from you because you guys also did like a bunch of fine tuning. And also maybe merging as well is related to here. [01:17:43] Nisten Tahiraj: Yeah, gotta keep in mind that for a while, fine tuning was a lot more expensive. Running fine tuned models was a lot more expensive than using GPT 3. 5. And then it got a lot cheaper with all the API companies, especially together and the other ones. So the business case for it has not really been how how cheap it is. [01:18:08] Nisten Tahiraj: I think in my opinion, the business case has. been all about data ownership. A lot of companies that have their own chatbots and stuff, they they see the data as their property and the value in their company, so the reason they fine tune is not because necessarily it's better, sometimes it is but it's been to just have full control of the data. And there have been a lot of drawbacks where you could have the knowledge could be lost. But there are much newer techniques where you can do, quote unquote, lossless fine tuning and and still have it. But yeah, I'll I'll land it there. So I think the business case is not necessarily the cost that has, it's always just been about data ownership. [01:18:53] Nisten Tahiraj: I'm actually doing consulting for one client now that really just wants to use Grok. Some they use the Grok API before and now they want to run it on their own and they don't care how many. JVs and stuff it costs to run because they factor it in with what their users pay. [01:19:13] Nisten Tahiraj: So, so, so yeah I'm noticing that it's more about the ownership side, not not necessarily the performance or cost. [01:19:21] Alex Volkov: GR with a K or grok with a Q. [01:19:23] Nisten Tahiraj: Grok with a K the new, yeah, [01:19:25] Alex Volkov: Oh, really? What API they use for grok. There's no API is there an API for grok that I missed? [01:19:31] Nisten Tahiraj: No they [01:19:31] Ian Maurer: open source the model. [01:19:33] Alex Volkov: Oh, so somebody hosted this and then they used the API since the, since last week basically [01:19:37] Ian Maurer: no, they people [01:19:38] Nisten Tahiraj: have used have used grok. I think they just did a, like a translation layer via via premium, but they did use grok in, in, in a product for, via an API. I'll have to, I'll have to double check how exactly, [01:19:53] Alex Volkov: like I can think of a way, but I'm not saying it's kosher. Like you, you can, you can put a Chrome extension and use the browser. Very [01:19:59] Nisten Tahiraj: No, even Levels. io deployed a, uh, like a WhatsApp bot that was that was running off of Grok too. So again I'll check up on that. I don't know what API stuff they, they used, but I am helping them now just run their own. [01:20:16] Alex Volkov: I see. LDJ, you unmuted. You want to chime in on the kind of like a specific choice and data ownership piece of the fine tuning, which I think is important. But from the other side if I'm representing the other side and I'm not, I'm just trying to figure out like where the vibes are coming from about this eligiment is most clouds now run most [01:20:34] Alex Volkov: Open source models, or at least, Microsoft definitely is now like supporting Mixtral. [01:20:38] Alex Volkov: I don't know if they're going to run Grok for you or not. And. There's also something to be said where if you're running Cloud from inside Amazon or Bedrock or Vertex or whatever you still own your data, aren't you? [01:20:52] LDJ: I'm not too familiar with the situation with Vertex and stuff but I do think that in the situations where a business has to. would want to and has to fine tune on like their company data so that employees can actually like, use something that is like an AI that understands the internal company information. [01:21:12] LDJ: That is I would say still a decent sized use case that you would have to use like the open source models for like unless you're fine with giving open AI your data and stuff, but I'm not saying necessarily open AI will train on it. I know they have different clauses and stuff, but you know, there's always like that risk and if you want to keep that stuff secret and internal, then you do have to still just use the open source models to fine tune. [01:21:38] Alex Volkov: Yeah. the additional kind of piece that, that I think Ethan knowledge like pointed to and before I get to Justin super quick, is that the example of Bloomberg and I think LDJ you wanted to push back on this example, but I'll cover this like briefly. B Bloomberg, sorry. Bloomberg famously trained a model called Bloomberg gpt based on the type of financial data that Bloomberg has access to. [01:22:00] Alex Volkov: And back then it like it significantly improved. LLM thinking about like finances and financial data, et cetera, only to then find out that a General model, like GPT 4, like blows it out of the Water Whatever 10 million, whatever they spent on that. And I think this was like also A highlight of how general models after they get released and they're getting better they're getting better across the board Not only for your task, but also for your task as well and before we get to Junaid and LDJ, you had a pushback that they didn't do it correctly, it was a skill issue or something like this, right? [01:22:32] LDJ: Yeah. I think it was honestly more of a skill issue on Bloomberg's part because. And I'll try and find the exact source for what I'm about to say, but it was like within a few weeks of Bloomberg GPT releasing, like there's like just a couple open source developers that released like a finance specific model. [01:22:49] LDJ: That was performing significantly better on the finance benchmarks with the same amount or less parameters. And that was just within a few weeks of Bloomberg GPT releasing. So obviously you didn't even need all that Bloomberg data and all that stuff to actually even get something that, that well performing. [01:23:06] Alex Volkov: Yep. All right. [01:23:07] Alex Volkov: I want to get to Justin, because, Justin, obviously you're on the Qwen team, you guys are building models that then other folks maybe fine tune and probably also supporting, enterprise use cases. What's your take on the fine tuning area?[01:23:20] [01:23:20] Justin Lin: Yeah, just some comment on the fine tuning for customer data. I think I somehow disagree with the idea that. We can inject new knowledge to the model through fine tuning because it is really difficult to do that. Do this thing with such a few data because we often use a very small amount of data to for fine tuning. I have read the paper, I don't remember its name, but it's telling us that fine tuning is more about aligning to the behavior, to the style, but not injecting new knowledge. If you want to inject new knowledge, you have to do things like this. Pre training next token prediction with ten, tens of billions of tokens so you can do this, but it is really hard. [01:24:09] Justin Lin: Something I would like to comment is that our customers fine tune our model and they found that the general capability is decreased. With the new knowledge I think this is quite reasonable because somehow our customers or users don't know really how to fine tune for a general model. [01:24:29] Justin Lin: They want the general capability, but they want something new. So we have provided a solution is that we just provide our data for general fine tuning in a black box way. So you can use our data, but you cannot see our data, and you can mix our data with your own, yeah, customer data so that you can train a new model which has a balanced behavior good general capabilities, but some new knowledge or some new styles of your company or something like that. [01:25:04] Justin Lin: Yeah. This is some of my personal [01:25:06] Justin Lin: experience. Yeah. [01:25:07] Alex Volkov: I really appreciate this, because I think that The difference is important fine tuning is not like a catch all sentence. There's fine tuning for style fine tuning for alignment for different ways to respond, for example. and that I think still, makes perfect sense. We have base models, we have fine tuned models for instruction fine tuning, for example. but I think that this is, at least the way I see it on my kind of radar, and I wanted to bring this to ThursdAI because I think it's very important for folks who follow this to also know that this is happening is from specifically from Finetuning with new knowledge, not only new kind of styles, new knowledge specifically, because the additional piece here is fine tuning takes a while and like maybe we said about Bloomberg maybe a skill issue Maybe you have to get like those machine learning engineers whereas with the advent of faster hardware better models that are open for you and They're now hosted on the actual kind of like the bedrock from Amazon. for example, this is basically in your cloud, They're running whatever haiku but in your cloud and the same agreements of not training all Your data is like the same, they apply OpenAI, You can run through Microsoft thing and in your cloud in Azure, and it's not like sending some data to OpenAI. So when we get to like bigger contexts, the ability of you to switch up and give this whatever product you're building on top of these LLMs, new data That's easier than Finetune with just like just providing the same context as well. [01:26:29] Alex Volkov: Tanishq, I saw you had your hand up and definitely want to hear from you as well. [01:26:34] Tanishq Abraham: Yeah, I guess I just had a few thoughts about this whole thing because, I'm working in the medical AI space and we're like by two models for, clinical applications, medical applications. So, I have various thoughts about this. I think just generally, of course I think it's with Phytuning yeah, It's particularly useful if you like, I think LDJ is of course, but actually the use case of yeah, if there's private data, that's of course a big one. [01:26:56] Tanishq Abraham: I think also if you want to have models locally, you want to use models locally. I think that's another big use case. A lot of times, there are many cases where, you don't want. To use cloud services, I think like in the medical scenario, for example, maybe you don't want to send medical data to various cloud providers and having some sort of local models could potentially be useful. [01:27:13] Tanishq Abraham: And of course there are other applications where maybe you want to have models run on, Some sort of like smartphones or other devices. So that's, I think one particular area where like fine tuning is particularly valuable. I think, in the sort of just to provide maybe some context in the medical space, medical AI space, I think this idea of whether or not fine tuning is useful is, I think, honestly, in my opinion, like an argument that's like still not settled yet. [01:27:38] Tanishq Abraham: So for example, like in the clinical LSP space, you have models like, of course, GPT 4, you have then you have, Google has their MedPOM models, then other model, other people are creating specific fine tunes. About a couple of years ago, or maybe it was a year ago, there was a paper that tried to see if for example, something like GPT 3 was better, or fine tuning a specific model for medical use cases was better. [01:28:02] Tanishq Abraham: They found that fine tuning was better performing and of course required less parameters and was a smaller model. But then something like people Google, for example, created their MedPAL models. Those are more like alignment in the sense that Justin was talking about. The knowledge is mostly there in the original PAL models and they're just doing some sort of instruction fine tuning. [01:28:22] Tanishq Abraham: And so that has been showing to do quite well. Thank you. And then recently there was a paper, the MedPrompt paper, which basically prompted GPT 4 to basically outperform all these other models for medical tasks. And so that one was just trying to say like a general purpose model is good enough. [01:28:40] Tanishq Abraham: So I think there's still a lot of it's still actually an open question, at least in, in this specific area, whether or not PHI tuning is better, or if it's just alignment that's needed, or you can just use the general purpose model. And so I think we're trying to study this question a little bit more detail as well, and try to see if PHI tuning really is necessary, if that actually does provide benefit. [01:28:58] Tanishq Abraham: And at least for me, I think of it more like, when I say PHI tuning, I also think of it like as continued pre trading where, yeah, we are probably be trading on we are trading on like tens of billions of tokens. To add knowledge to a model. And I think, there's, people talk about FI tuning, but they also talk about continued pre-training and sometimes the distinction between those is a little bit kind of a group. [01:29:18] Tanishq Abraham: There isn't much of a distinction sometimes, so there's also that as well. And I think that also is a lot of the times the question between whether or not it's just doing alignment versus adding knowledge. I think, that's. Part of that discussion and that, that isn't really I think clarified very often so that there's, that's the other aspect, but yeah, those are my thoughts on the topic. [01:29:37] Alex Volkov: thanks Tanishq. And I also want to welcome Ian Moore to the stage. Ian, it's been a while since you've been here. Thoughts on this exciting discussion and have you seen the same trends or the same kind of vibes that I brought up on where you read and [01:29:51] Ian Maurer: yeah. [01:29:51] Ian Maurer: We were talking about this in January, Alex, I found the conversation, right? Finetuning versus RAG, the question is what's your goal? What's your use case? What's your eval? I think Hamill even mentioned, do you know, even know what your evals are? Do you even know what you're trying to accomplish? [01:30:03] Ian Maurer: Without that good luck fine tuning, good luck building an app. Anyways my, I have a very distinct opinion and perspective, but I'll give you guys background so you understand where it's coming from. My company is 12 years old. We've got an old, good old fashioned AI company where we've curated 100, 000, Rules, effectively, in a knowledge base. [01:30:20] Ian Maurer: It's a graph. It's got ontologies and things like that. And those rules have been curated by experts with PhDs, and we have an API that sets over it, and reasons over it, and can match patients to clinical trials. This is for cancer, right? So, patients get, DNA sequence, and it's very complicated, whatever. [01:30:35] Ian Maurer: So, the great thing about large language models and as they get bigger and better is that they can understand language, including all language, including medical language, so they can understand the intent of a provider, right? The provider's trying to accomplish something, which is as quickly as possible, how do I help this patient? [01:30:51] Ian Maurer: And So the thing that I have found that's most useful for us is to help that expert be as productive as they can possibly be. Use the large language model to understand their intent, what they have. I have a patient, they have a problem, what they want to find the best possible treatments for that patient. [01:31:07] Ian Maurer: And then how to do that is by giving that large language model tools, right? Don't. Why do I want to fine tune knowledge into it? And then I just, I basically black boxed all my knowledge, right? Great. I have all this great knowledge I've curated over the years. I'm going to fine tune it into my system. And now it's a black box and I can't tell you where from or why it's there. [01:31:25] Ian Maurer: No, I want to be able to tell you, here's the trials that are available for your patient. Here's the drugs that are available for your patient. This is the, the best possible outcome for that. And here's the link to the clinical trials page, or here's the link to the the FDA page that tells you why this drug is so [01:31:40] good. [01:31:40] Ian Maurer: I can't do that if it's a black box. I'd be hallucinating all over the place. So my perspective is Finetuning is great if you're talking about a very discreet use case that you're trying to, drill down on cost. Hey, I figured out this named entity recognition pattern and now I'm, I was doing it expensively with few shot learning. [01:31:57] Ian Maurer: Now I'm going to go, fine tune something and save that cost. But otherwise, you know Use the best possible model, give them tools, whether it's, through function calling or GPT actions are actually pretty good. And that's the best way to get the value out of the large language model and work with existing knowledge. [01:32:13] Alex Volkov: So definitely sightings and knowing exactly about your data and not like blurring it out inside the brain of LLM, fuzzing it out where you can't actually know where it came from or whether or Not it's hallucinated. I think that's a big piece here that companies are actually like starting to also get into. [01:32:30] Alex Volkov: And so I think you're Your perspective. is very important as Well I think also from the perspective at least the vibes that I've seen from the perspective of updating that data afterwards, like just continue fine tuning, like requires more knowledge and more skill, rather than just updating your vector databases, let's say, and have the model provide enough context. and I think the smartness to price ratio, I think is very important as well. If we get like models like Haiku, for example, they're like incredibly cheap. But have a vast context length that you can use both for fine tuning towards alignment, let's say, or behave like whatever you want it to behave or answer as our company versus answer is like the, this LLM together with you have enough context to do that and it's not cost prohibitive for you to use this large context for a bunch of stuff. and it's very important [01:33:18] Alex Volkov: so I thanks Ian for coming up. I want to tie this back a little bit and then close the discussion also, I do want to shout out that you also have an awesome list of function calling, which now includes a bunch of open source. models that support function calling as well . The support is like function calling as well and it talks about the specifics in which they support function calling. Which is great and definitely will be in the show notes as well and with that folks, I think we'll end ThursdAI for today we had a bunch of stuff. [01:33:44] Alex Volkov: There's a small breaking news from ray Ray just mentioned that Cursor the AI editor that we a lot of US use and love they just released an update where like their Cursor, like Copilot plus feature is still available. twice as fast now in some areas and that's been like awesome to use. So if you haven't used Cursor yet, definitely give it, give them A try. [01:34:02] Alex Volkov: And Cursor is like really impressive, especially with Opus. If you have paid for Cursor Premium, have access to the best LLM in the world. I think that this is all that we wanted to talk about. thank you everybody for [01:34:12] Alex Volkov: joining from week to week. [01:34:13] Alex Volkov: I think that's most of what we talked about on ThursdAI for March 28th. With that, I want to thank Nisten, LDJ, Justin, Junyang, Robert Skobel was here before, Ian Moore jumped on, Tanishq, and Paul, definitely from MedArc and everybody else who joined us I really appreciate everybody's time here. If you're not subscribed to ThursdAI to get every link that we've talked about, I really work hard to give you all the links, so definitely give a subscription Other than that have a nice Thursday, everyone. We'll see you next week. Cheers, everyone. [01:34:41] Ian Maurer: Bye everybody. [01:34:42] Alex Volkov: bye bye This is a public episode. If you'd like to discuss this with other subscribers or get access to bonus episodes, visit sub.thursdai.news/subscribe | |||
05 Apr 2024 | 📅 ThursdAI Apr 4 - Weave, CMD R+, SWE-Agent, Everyone supports Tool Use + JAMBA deep dive with AI21 | 01:50:05 | |
Happy first ThursdAI of April folks, did you have fun on April Fools? 👀 I hope you did, I made a poll on my feed and 70% did not participate in April Fools, which makes me a bit sad! Well all-right, time to dive into the news of this week, and of course there are TONS of news, but I want to start with our own breaking news! That's right, we at Weights & Biases have breaking new of our own today, we've launched our new product today called Weave! Weave is our new toolkit to track, version and evaluate LLM apps, so from now on, we have Models (what you probably know as Weights & Biases) and Weave. So if you're writing any kind RAG system, anything that uses Claude or OpenAI, Weave is for you! I'll be focusing on Weave and I'll be sharing more on the topic, but today I encourage you to listen to the launch conversation I had with Tim & Scott from the Weave team here at WandB, as they and the rest of the team worked their ass off for this release and we want to celebrate the launch 🎉 TL;DR of all topics covered: * Open Source LLMs * Cohere - CommandR PLUS - 104B RAG optimized Sonnet competitor (Announcement, HF) * Princeton SWE-agent - OSS Devin - gets 12.29% on SWE-bench (Announcement, Github) * Jamba paper is out (Paper) * Mozilla LLamaFile now goes 5x faster on CPUs (Announcement, Blog) * Deepmind - Mixture of Depth paper (Thread, ArXiv) * Big CO LLMs + APIs * Cloudflare AI updates (Blog) * Anthropic adds function calling support (Announcement, Docs) * Groq lands function calling (Announcement, Docs) * OpenAI is now open to customers without login requirements * Replit Code Repair - 7B finetune of deep-seek that outperforms Opus (X) * Google announced Gemini Prices + Logan joins (X)קרמ * This weeks Buzz - oh so much BUZZ! * Weave lunch! Check weave out! (Weave Docs, Github) * Sign up with Promo Code THURSDAI at fullyconnected.com * Voice & Audio * OpenAI Voice Engine will not be released to developers (Blog) * Stable Audio v2 dropped (Announcement, Try here) * Lightning Whisper MLX - 10x faster than whisper.cpp (Announcement, Github) * AI Art & Diffusion & 3D * Dall-e now has in-painting (Announcement) * Deep dive * Jamba deep dive with Roi Cohen from AI21 and Maxime Labonne Open Source LLMs Cohere releases Command R+, 104B RAG focused model (Blog) Cohere surprised us, and just 2.5 weeks after releasing Command-R (which became very popular and is No 10 on Lmsys arena) gave us it's big brother, Command R PLUS With 128K tokens in the context window, this model is multilingual as well, supporting 10 languages and is even beneficial on tokenization for those languages (a first!) The main focus from Cohere is advanced function calling / tool use, and RAG of course, and this model specializes in those tasks, beating even GPT-4 turbo. It's clear that Cohere is positioning themselves as RAG leaders as evident by this accompanying tutorial on starting with RAG apps and this model further solidifies their place as the experts in this field. Congrats folks, and thanks for the open weights 🫡 SWE-Agent from Princeton Folks remember Devin? The super cracked team born agent with a nice UI that got 13% on the SWE-bench a very hard (for LLMs) benchmark that requires solving real world issues? Well now we have an open source agent that comes very very close to that called SWE-Agent SWE agent has a dedicated terminal and tools, and utilizes something called ACI (Agent Computer Interface) allowing the agent to navigate, search, and edit code. The dedicated terminal in a docker environment really helps as evident by a massive 12.3% score on SWE-bench where GPT-4 gets only 1.4%! Worth mentioning that SWE-bench is a very hard benchmark that was created by the folks who released SWE-agent, and here's some videos of them showing the agent off, this is truly an impressive achievement! Deepmind publishes Mixture of Depth (arXiv) Thanks to Hassan who read the paper and wrote a deep dive, this paper by Deepmind shows their research into optimizing model inference. Apparently there's a way to train LLMs without affecting their performance, which later allows to significantly reduce compute on some generated tokens. 🧠 Transformer models currently spread compute uniformly, but Mixture-of-Depths allows models to dynamically allocate compute as needed 💰 Dynamically allocating compute based on difficulty of predicting each token leads to significant compute savings ⏳ Predicting the first token after a period is much harder than within-sentence tokens, so more compute is needed 🗑 Most current compute is wasted since difficulty varies between tokens We're looking forward to seeing models trained with this, as this seems to be a very big deal in how to optimize inference for LLMs. Thank you for reading ThursdAI - Best way to support us is to just share this with folks 👇 Big CO LLMs + APIs Anthropic and Groq announce function calling / tool use support, Cohere takes it one step further In yet another example of how OpenAI is leading not only in models, but in developer experience, most models and API providers are now using the same messages API structure. Back in June of 2023, OpenAI gave us function calling, and finally the industry is aligning to this format, which is now being rebranded as "tool use" If you're unfamiliar with the concept, tool use allows a developer to specify what tools the model can have in addition to just spitting out tokens, think browsing the web, or using RAG to get more information, or check the weather, or... turn off a lighbulb in your smart home. The LLM then decides based on user input, if a specific tool needs to be called, responds with the tool and parameters it needs to the developer, and then expects the result of that tool, and finally, is able to respond to the user with the complete information. So this week we've got Command R, Groq and Anthropic all adding support for tool use, which is incredible for developer experience across the board and will allow developers to move between all those APIs. Cohere goes one step further with something they call Multi Step tool use, which is a significant step up and is very interesting to explore, as it gives their models the ability to rank and order tool execution, and ovserve their responses. Anthropic Docs https://docs.anthropic.com/claude/docs/tool-use Groq Docs https://console.groq.com/docs/tool-use Cohere Docs https://docs.cohere.com/docs/multi-step-tool-use Cloudflare AI is now in GA + workers in Python If you've been following ThursdAI, you know I'm a huge Cloudflare fan. I've built my startup (https://targum.video) on top of Cloudflare workers platform, and I gave them early feedback about having to step into AI in a big way. And they did, with workers AI which is now in GA. Workers AI lets developers in the Cloudflare ecosystem run LLMs (they mostly feature Opensource LLMs which is incredible), host vectors, run whisper and basically have end to end serverless apps that are powered by AI (they have GPUs in 150 cities around the world) This week Clouflare announced also the ability to write workers in Python, which was sorely missing for some folks (like me!) who love FastAPI for example, and while it's not a full python environment, the depth to which they had to go in order to allow python to execute on their edge is kind of ridiculous, read up on it here I'm hoping to work with them to bring weave into the workers for python soon 🤞 because building AI applications with Cloudflare is so simple, they even have a HuggingFace integration which allows you to bring models into your CF environment with 1 click. This weeks Buzz - SO MUCH BUZZ Hey, well first of all, I now can offer you a 15% off a ticket to our conference, so use THURSDAI when you checkout and get a ticket here Now that Weave is out, it's possible to say that our workshop on April 17 (same link as above) is going to be focused on LLM evaluations and yes, I will be talking about how to use weave to build LLM applications in production safely. If this field is new to you, please sign up and come to the workshop! JAMBA deep dive with Roi @ AI21 and Maxime Labonne As always, what I cover in this newsletter are only the highlights of what we talked about, but there was so much more, I really recommend you to listen to the episode. This of this weeks episode as 2 episodes (maybe I should re-release the deep dive as a separate episode) because we had a long conversation with Roi Cohen who's a PM @ AI21 and Maxime Labonne (Author of LazyMergeKit and first finetune of JAMBA), it's really worth tuning into that interview. Here's a little snippet: Aaaand this is it for this week, or you know what? Maybe it's not! I shared this on X but if you don't follow me on X, I decided to prank my whole feed by saying that I'm basically changing careers and becoming a Russian AI DJ, called DJ Thursday and I will only play AI generated music. The weird thing, how many people were like, yeah ok, this makes sense for you 😅 So here's my April Fools (one of them) joke, hope you enjoy the high quality of these tunes and see you all next week 🫡 This is a public episode. If you'd like to discuss this with other subscribers or get access to bonus episodes, visit sub.thursdai.news/subscribe | |||
12 Apr 2024 | 📅 ThursdAI - Apr 11th, 2024 - GPT4 is king again, New Mixtral 8x22B + First finetune, New Gemini 1.5, Cohere beats old GPT4, more AI news | 01:38:35 | |
this week was absolutely bonkers. For starters, for the first time ever, we got an Open Weights model (Command R+) to jump over GPT-4 in human rankings on LMsys, this is huge! Then on Tuesday, it seems that all the companies just wanted to one up one another, first Gemini 1.5 released with updates, made it available in 180 countries, added audio mode + tons of API improvements and system prompts, then less than an hour later, OpenAI has given us a "majorly improved" GPT-4 Turbo version (2024-04-09) that is now back to being the BEST LLM IN THE WORLD and to cap that day off, Mistral did the thing again, the thing being, dropping a torrent link in a tweet with no explanations. What was in that torrent is a Mixtral 8x22B MoE (which we started calling Bixtral) which comes with an Apache2 license and seems to be VERY good! We also saw the first finetune from HuggingFace/KAIST folks less than 48 hours later (the authors of said finetune actually came on the show 🎉 ) Fully Connected is a week from today! If you haven't yet signed up, use THURSDAI promo code and come hear from Richard Socher (You.com), Jerry Liu (Ilamaindex CEO), Karoly (TwoMinutePapers), Joe Spisak (Meta) and and leaders from NVIDIA, Snowflake, Microsoft, Coatue, Adobe, Siemens, Lambda and tons more 👇 TL;DR of all topics covered: * Open Source LLMs * 🔥 Mistral releases Mixtral 8x22 Apache 2 licensed MoE model (Torrent, TRY IT) * Cohere CMDR+ jumps to no 6 on LMSys and beats GPT4 (X) * CodeGemma, RecurrentGemma & Gemma Instruct 1.1 (Announcement) * Auto-code-rover gets 22% on SWE bench (Announcement) * HuggingFace - Zephyr 141B-A35B - First Bixtral Finetune (Announcement) * Mistral 22B - 1 single expert extracted from MoE (Announcement, HF) * This weeks Buzz - Weights & Biases updates * FullyConnected is in 1 week! (Come meet us) * Big CO LLMs + APIs * 🔥 GPT-4 turbo is back to being number 1 AI with 88.2% Human Eval score (X) * Gemini 1.5 Pro now understands audio, uses unlimited files, acts on your commands, and lets devs build incredible things with JSON mode (X) * LLama 3 coming out in less than a month (confirmed by Meta folks) * XAI Grok now powers news summaries on X (Example) * Cohere new Rerank 3 (X) * Voice & Audio * HuggingFace trained Parler-TTS (Announcement, Github) * Udio finally launched it's service (Announcement, Leak, Try It) * Suno has added explore mode (suno.ai/explore) * Hardware * Humane AI pin has started shipping - reviews are not amazing Open Source LLMs Command R+ first open weights model that beats last year GPT4 versions This is massive, really a milestone to be discussed, and even though tons of other news happened, the first time an open weights model is beating GPT-4 not on a narrow case (coding, medical) but on a general human evaluation on the arena. This happened just a year after GPT-4 first came out, and is really really impressive. Command R+ has been getting a lot of great attention from the community as well, folks were really surprised by the overall quality, not to mention the multilingual abilities of CommandR+ Mixtral 8x22B MoE with 65K context and Apache 2 license (Bigstral) Despite the above, Cohere time in the sun (ie top open weights model on lmsys) may not be that long if the folks at Mistral have anything to say about it! Mistral decided to cap the crazy Tuesday release day with another groundbreaking tweet of theirs which includes a torrent link and nothing else (since then they of course uploaded the model to the hub) giving us what potentially will unseat Command R from the rankings. The previous Mixtral (8x7B) signaled the age of MoEs and each expert in that was activated from Mistral 7B, but for this new affectionally named Bixtral model, each expert is a 22B sized massive model. We only got a base version of it, which is incredible on it's own right, but it's not instruction finetuned yet, and the finetuner community is already cooking really hard! Though it's hard because this model requires a lot of compute to finetune, and not only GPUs, Matt Shumer came on the pod and mentioned that GPUs weren't actually the main issue, it was system RAM when the finetune was finished. The curious thing about it was watching the loss and the eval loss. it [Bixtral] learns much faster than other models - Matt Shumer Matt was trying to run Finetunes for Bigstral and had a lot of interesting stuff to share, definitely check out that conversation on the pod. Bigstral is... big, and it's not super possible to run it on consumer hardware.... yet, because Nisten somehow got it to run on CPU only 🤯 using Justin Tuneys LLM kernels (from last week) and LLama.cpp with 9tok/s which is kinda crazy. HuggingFace + KAIST release Zephyr 141B-A35B (First Mixtral 8x22 finetune) And that was fast, less than 48 hours after the torrent drop, we already see the first instruction finetune from folks at HuggingFace and KAIST AI. They give us a new finetune using ORPO, a technique by KAIST that significantly improves finetuning ability (they finetuned Bigstral with 7k capybara instructions for 1.3 hours on 4 nodes of 8 x H100s) They used the distilled Capybara Dataset (From LDJ and Argilla) to give this model a bit more clarity and instruction following. You can find the model on the hub here, and the question is, but now the question is would one run this? 😅 Btw the authors of the finetune and the ORPO paper from KAIST, Jiwoo Hong and Noah Lee came on the pod and chatted about this finetune and ORPO which was awesome! Definitely check this conversation out. Big CO LLMs + APIs Gemini 1.5 Pro updates - Audio Mode, JSON, System prompts and becomes free Google really pulled out all the stops for this updated release of Gemini 1.5 Pro, it's flagship, 1M context window model. Its now available for free to over 180 countries, has a new audio mode where you can upload up to 9.5 hours of audio (which is crazy on it's own) and it's not merely transcription, it seems that they baked an audio encoder in there so the model can understand some tonality and even some dogs barking in the background! In fact, instead of me writing down, how about I show you an example of Gemini itself extracting everything I said about it during the show? Here's a screenshot of me uploading 2+ hours of raw unedited audio form the show today: You can see the Google AI studio (which is a very clean product!) and the new system message, the ability to turn the safety filters off (thank you!) and the audio mode. Not to mention the 250K tokens 😂 that my audio cost this model. Mind you, the highest context window after Gemini is Claude 3 with 200K. Google also significantly improves the APIs, and gave access to a new file upload API that allows up to 2GB files uploaded (to support this amazing context and multimodality) 🔥 OpenAI - GPT 4 turbo a new and "Majorly improved version" Remember when Gemini 1.5 was announced? You may not remember that specific day, because an hour after that, OpenAI published SORA and blew our collective minds off. Well, OpenAI is at it again, but this time it didn't quite work the same way, but an hour after Gemini 1.5 updates came out, OpenAI released GPT4-Turbo-April-9 aka (gpt-4-turbo-2024-04-09) and basically all they said that it was "majorly improved" The technical stuff first, they combined the tool use (function calling) API with the Vision API, which is feature parity with Anthropic). The vibes are currently good, folks are seeing improvements across the board in logic and code creation, specifically the folks at Cursor posted an example (and enabled this model in their IDE) where it writes higher quality code. As I’m writing these words, LMSys updated us that this new model shot up to the top of the arena taking the Mantle back from Opus as the best AI we have, and also a confirmation from OpenAI that this model is now powering the chatGPT interface 👏 OpenAI also just open sourced a repo to show what they used to get these exact scores for the new GPT-4 and they are impressive This weeks Buzz (What I learned with WandB this week) Final Call! Fully Connected, our very own annual conference is about to commence (hehe of course it's happening on a ThursdAI, I still have to think about how to record the show next week) Please feel free to use the code THURSDAI to sign up and come see us. As a reminder, we're also running a workshop a day before, where we're going to showcase Weave and give practical examples for LLM builders, and it's going to be a lot of fun! Looking forward to see some of you there! Audio & Voice Udio launches a suno competitor AI Music service For the past week+ I've seen tons of AI plugged folks in SF post about "a new AI for music is coming and it's going to be amazing". Well it's finally here, called Udio and it gives Suno a run for its money for sure. With the ability to create full tracks, create into and outro, remix, and a very needed AI enhanced prompting, Udio does look very very polished and sounds GOOD! Here is an example of a classical music track that's been going viral: I've played a few more examples on the show itself, and you can check out the trending creations on their page. Interestingly, this is probably a diffusion model, and so folks have been squeezing all kinds of stuff that's not only musical out of there, including, stand up comedy with a full laugh track. Suno adds explore mode Meanwhile Suno is not going down without a fight and have released this amazing new page where they generated thousands of samples for hundreds of interesting/weird sound styles, letting you get exposed and learn about different musical styles. I really liked it so recorded a short reaction video: Phew, somehow we made it, we were able to summarize the huge news this week in under two hours + a newsletter! The one thing I haven't been able to do is to actually try out many of the stuff I talked about, so after writing this, will take a little break and delve into some of the other things I haven't yet tried 👀 See you guys next week in limited capacity (maybe, we'll see) and until then, have a great week 🫡 This is a public episode. If you'd like to discuss this with other subscribers or get access to bonus episodes, visit sub.thursdai.news/subscribe | |||
19 Apr 2024 | 📅 ThursdAI - Apr 18th - 🎉 Happy LLama 3 day + Bigxtral instruct, WizardLM gives and takes away + Weights & Biases conference update | 02:13:43 | |
Happy LLama 3 day folks! After a lot of rumors, speculations, and apparently pressure from the big Zuck himself, we finally can call April 18th, 2024, LLaMa 3 day! I am writing this, from a lobby of the Mariott hotel in SF, where our annual conference is happening called Fully Connected, and I recorded today's episode from my hotel room. I really wanna shout out how awesome it was to meet folks who are listeners of the ThursdAI pod and newsletter subscribers, participate in the events, and give high fives. During our conference, we had the pleasure to have Joe Spisak, the Product Director of LLaMa at Meta, to actually announce LLaMa3 on stage! It was so exhilarating, I was sitting in the front row, and then had a good chat with Joe outside of the show 🙌 The first part of the show was of course, LLaMa 3 focused, we had such a great time chatting about the amazing new 8B and 70B models we got, and salivating after the announced but not yet released 400B model of LLaMa 3 😮 We also covered a BUNCH of other news from this week, that was already packed with tons of releases, AI news and I was happy to share my experiences running a workshop a day before our conference, with focus on LLM evaluations. (If there's an interest, I can share my notebooks and maybe even record a video walkthrough, let me know in the comments) Ok let's dive in 👇 Happy LLama 3 day 🔥 The technical details Meta has finally given us what we're all waiting for, an incredibly expensive (2 clusters of 24K H100s over 15 Trillion tokens) open weights models, the smaller 8B one and the larger 70B one. We got both instruction fine tune and base models, which are great for finetuners, and worth mentioning that this is a dense model (not a mixture of experts, all the parameters are accessible for the model during inference) It is REALLY good at benchmarks, with the 7B model beating the previous (LLaMa 2 70B) on pretty much all benchmarks, and the new 70B is inching on the bigger releases from the past month or two, like Claude Haiku and even Sonnet! The only downsides are the 8K context window + non multimodality, but both are coming according to Joe Spisak who announced LLama3 on stage at our show Fully Connected 🔥 I was sitting in the front row and was very excited to ask him questions later! By the way, Joe did go into details they haven't yet talked about pulblicly (see? I told you to come to our conference! and some of you did!) and I've been live-tweeting his whole talk + the chat outside with the "extra" spicy questions and Joes winks haha, you can read that thread here The additional info Meta has also partnered with both Google and Bing (take that OpenAI) and inserted LLama 3 into the search boxes of Facebook, Instagram, Messenger and Whatsapp plus deployed it to a new product called meta.ai (you can try it there now) and is now serving LLama 3 to more than 4 Billion people across all of those apps, talk about compute cost! Llama 3 also has a new Tokenizer (that Joe encouraged us to "not sleep on") and a bunch of new security tools like Purple LLama and LLama Guard. PyTorch team recently released finetuning library called TorchTune is now supporting LLama3 finetuning natively out of the box as well (and integrates Wandb as it's first party experiment tracking tool) If you'd like more details, directly from Joe, I was live tweeting his whole talk, and am working at getting the slides from our team. We'll likely have a recording as well, will post it as soon as we have it. Here's a TL;DR (with my notes for the first time) of everything else we talked about, but given today is LLaMa day, and I still have to do fully connected demos, I will "open source" my notes and refer you to the podcast episode to hear more detail about everything else that happened today 🫡 TL;DR of all topics covered: * Meta releases LLama 3 -8B, 70B and later 400B (Announcement, Models, Try it, Run Locally) * Open Source LLMs * Meta LLama 3 8B, 70B and later 400B (X, Blog) * Trained 15T tokens! * 70B and 8B modes released + Instruction finetuning * 8K context length , not multi modal * 70B gets 82% on MMLU and 81.7% on HumanEval * 128K vocab tokenizer * Dense model not MoE * Both instruction tuned on human annotated datasets * Open Access * The model already uses RoPe * Bigxtral instruct 0.1 (Blog, Try it) * Instruct model of the best Apache 2 model around * Release a comparison chart that everyone started "fixing" * 🤖 Mixtral 8x22B is Mistral AI's latest open AI model, with unmatched performance and efficiency * 🗣 It is fluent in 5 languages: English, French, Italian, German, Spanish * 🧮 Has strong math and coding capabilities * 🧠 Uses only 39B parameters out of 141B total, very cost efficient * 🗜 Can recall info from large documents thanks to 64K token context window * 🆓 Released under permissive open source license for anyone to use * 🏆 Outperforms other open models on reasoning, knowledge and language benchmarks * 🌐 Has strong multilingual abilities, outperforming others in 4 languages * 🧪 Excellent basis for customization through fine-tuning * New Tokenizer from Mistral (Docs) * Focusing on Tool Use with tokens 🔥 * WizardLM-2 8x22B, 70B and 7B (X, HF) * Released it and then pulled it back from HF and Github due to microsoft toxicity not passing * Big CO LLMs + APIs * OpenAI gives us Batch API + Assistants API v2 * Batch is 50% cost and win win win * Assistants API V2 - new RAG * new file search tool * up to 10,000 files per assistant * new vector store * Reka gives us Reka Core (X, Try) * Multimodal that understands video as well * 20 people team * Video understanding is very close to Gemini * 128K context * Core has strong reasoning abilities including for language, math and complex analysis. * 32 languages support * HuggingFace ios chat bot now * This weeks Buzz * Me + team led a workshop a day before the conference (Workshop Thread) * Fully Connected in SF was an incredible success, over 1000 AI attendies + Meta AI announcement on stage 🔥 * PyTorch new TorchTune finetuning library with first class WandB support (X) * Vision & Video * Microsoft VASA-1 animated avatars (X, Blog) * Amazing level of animation from 1 picture + Sound * Harry Potter portraits are here * They likely won't release this during Election year * Looks very good ,close to EMO but no code * 📺 Videos show faces speaking naturally with head movements and lip sync * 🔬 Researchers are exploring applications in education, accessibility and more * HuggingFace updates IDEFICS2 8B VLM (X, HF) * Apache 2 license * Competitive with 30B models * 12 point increase in VQAv2, 30 point increase in TextVQA (compared to Idefics 1) * > 10x fewer parameters than Idefics 1 * Supports image resolution up to 980 x 980+ * Better OCR capabilities (thanks to more than 6TB of OCR pre-training data) * Adobe shows Firefly video + SORA support (X) * Voice & Audio * Rewind AI is now Limitless (X) * New service & Brand name * Transcription to you * Hardware device that looks sleek * 100hours * Privacy support in cloud * AI Art & Diffusion & 3D * Stability - Stable Diffusion 3 is here * Available via API only * Partnered with Fireworks HQ for the release * Needs stability AI membership to use / access $$ * Big step up in composition and notorious issues like hands, "AI faces" etc. (from * Seems to prefer simpler prompts. * Way more copyright-friendly. It's hard to get any kind of brands/logos. * Text is amazing. * Others * New AIrChat with amazing transcription is out, come join us in our AI corner there * Humane AI pin was almost killed by MKBHD review * Rabbit reviews incoming That's all for this week, next week we have an amazing guest, see you then! 🫡 This is a public episode. If you'd like to discuss this with other subscribers or get access to bonus episodes, visit sub.thursdai.news/subscribe | |||
26 Apr 2024 | 📅 ThursdAI - April 25 - Phi-3 3.8B impresses, LLama-3 gets finetunes, longer context & ranks top 6 in the world, Snowflake's new massive MoE and other AI news this week | 01:21:34 | |
Hey hey folks, happy ThursdAI 🎉 Not a lot of house-keeping here, just a reminder that if you're listening or reading from Europe, our European fullyconnected.com conference is happening in May 15 in London, and you're more than welcome to join us there. I will have quite a few event updates in the upcoming show as well. Besides this, this week has been a very exciting one for smaller models, as Microsoft teased and than released Phi-3 with MIT license, a tiny model that can run on most macs with just 3.8B parameters, and is really punching above it's weights. To a surprising and even eyebrow raising degree! Let's get into it 👇 ThursdAI - Recaps of the most high signal AI weekly spaces is a reader-supported publication. To receive new posts and support my work, consider becoming a free or paid subscriber. TL;DR of all topics covered: * Open Source LLMs * Microsoft open sources Phi-3 (X, HF) * LLama3 70B top5 (no top 6) on LMsys (LMsys Arena) * Snowflake open sources Arctic - A massive hybrid MoE (X, Try it, HF) * Evolutionary Model merges support in MergeKit (Blog) * Llama-3 8B finetunes roundup - Longer Context (128K) and Dolphin & Bagel Finetunes * HuggingFace FINEWEB - a massive 45TB (the GPT4 of datasets) and 15T tokens high quality web data dataset (HF) * Cohere open sourced their chat interface (X) * Apple open sources OpenElm 4 models + training library called corenet (HF, Github, Paper) * Big CO LLMs + APIs * Google Gemini 1.5 pro is #2 on LMsys arena * Devin is now worth 2BN and Perplexity is also a Unicorn * A new comer called Augment (backed by Eric Schmidt) is now coming out of stealth (X) * Vision & Video * Adobe releases VideoGigaGAN - high quality upscaler with temporal consistency (paper) * TLDraw autocomplete UI demo (X) * This Weeks Buzz - What I learned in WandB this week * Joe Spisak talk about Llama3 on Stage at WandB Fully connected (Full Talk, TLDR) * Voice & Audio * Play.ai (previously play.ht) releases conversational Voice AI platform (X) * AI Art & Diffusion & 3D * IMGsys.org- like LMsys but for image generation model + leaderboard from FAL (try it) * Tools & Hardware * Rabbit R1 release party & no shipping update in sight * I'm disillusioned about my AI Pin and will return it Open Source LLMs Llama-3 1 week-aversary 🎂 - Leaderboard ranking + finetunes Well, it's exactly 1 week since we got Llama-3 from Meta and as expected, the rankings show a very very good story. (also it was downloaded over 1.2M times and already has 600 derivatives on HuggingFace) Just on Monday, Llama-3 70B (the bigger version) took the incredible 5th place (now down to 6th) on LMSys, and more surprising, given that the Arena now has category filters (you can filter by English only, Longer chats, Coding etc) if you switch to English Only, this model shows up 2nd and was number 1 for a brief period of time. So just to sum up, an open weights model that you can run on most current consumer hardware is taking over GPT-4-04-94, Claude Opus etc' This seems dubious, because well, while it's amazing, it's clearly not at the level of Opus/Latest GPT-4 if you've used it, in fact it fails some basic logic questions in my tests, but it's a good reminder that it's really hard to know which model outperforms which and that the arena ALSO has a bias, of which people are using it for example and that evals are not a perfect way to explain which models are better. However, LMsys is a big component of the overall vibes based eval in our community and Llama-3 is definitely a significant drop and it's really really good (even the smaller one) One not so surprising thing about it, is that the Instruct version is also really really good, so much so, that the first finetunes of Eric Hartfords Dolphin (Dolphin-2.8-LLama3-70B) is improving just a little bit over Meta's own instruct version, which is done very well. Per Joe Spisak (Program Manager @ Meta AI) chat at the Weights & Biases conference last week (which you can watch below) he said "I would say the magic is in post-training. That's where we are spending most of our time these days. Uh, that's where we're generating a lot of human annotations." and they with their annotation partners, generated up to 10 million annotation pairs, both PPO and DPO and then did instruct finetuning. So much so that Jeremy Howard suggests to finetune their instruct version rather than the base model they released. We also covered that despite the first reactions to the 8K context window, the community quickly noticed that extending context window for LLama-3 is possible, via existing techniques like Rope scaling, YaRN and a new PoSE method. Wing Lian (Maintainer of Axolotl finetuneing library) is stretching the model to almost 128K context window and doing NIH tests and it seems very promising! Microsoft releases Phi-3 (Announcement, Paper, Model) Microsoft didn't really let Meta take the open models spotlight, and comes with an incredible report and follow up with a model release that's MIT licened, tiny (3.8B parameters) and performs very very well even against Llama-3 70B. Phi is a set of models from Microsoft that train on synthetic high-quality dataset modeled after textbooks-is-all-you-need/TinyStories approach. The chart is quite incredible, the smallest (mini) Phi-3 is beating Llama-3-8B AND Mixtral on MMLU scores, BigBench and Humaneval. Again to simplify, this TINY 3.8B model, half the size of 1 Mixtral expert, beats Mixtral and newly released Llama-3-8B on most benchmark, not to mention GPT-3.5! It's honestly quite a crazy chart to look at, which raises the question, did this model train on these benchmarks? 🤔 I still haven't seen definitive proof that the folks at Microsoft trained on any benchmarks data, I did see engagement from them and a complete denial, however we did see a few attempts at using Phi-3 and the quantized versions and the wrong end token formatting seem to be very prevalent in shaping the early opinion that this model performance is detached from it's very high scoring. Not to mention that model being new, there's confusion about how to use it, see thread from Anton Bacaj about HuggingFace potentially using the wrong end token to finish conversations. Now to an actual performance of this tiny model, I asked it a simple logic based question that trips many models even ones good with logic (Opus and GPT-4 answer it correctly usually) and it performed very well (here a comparison with LLama-3-70B which didn't do as well) Additionally, their tokenizer is very interesting, they have all these terms that receive a full token, things like function_list, calc, ghreview, ghissue, and others, which highlight some interesting potential use-cases they have planned for this set of models or give us a hint at it's training process and how come it's so very good. Snowflake open sources Arctic - a massive 480B MoE Hybrid with Apache 2 license (X, Try it, HF) Snowflake is a name I haven't yet used on ThursdAI and this field is getting crowded, but they just released something interesting (+ a LOT of open source, including training code, checkpoints, research insights etc') The thing I found most interesting is, the massive 128 experts MoE but also the Hybrid architecture. Not quite an MoE and definitely not a dense model. They claim to have found that training Many-but-condensed experts with more expert choices is working well for them based on DeepSpeed research. You can give this model a try here and I have, using the same 2 questions I had for Phi and LLama and found the model not that great at logic to be honest, but it was really fast considering the total size, so inference optimization for this type of architecture is definitely geared towards Enterprise (as well as training cost, they claim it cost just under $2 million dollars to train) Big CO LLMs + APIs Not a lot of super interesting things in this corner, besides Gemini 1.5 pro (the one with 1M context window) finally appearing in the Arena and taking the amazing #2 spot (pushing Llama-3 8B to number 6 on the same day it just appeared in there lol) This is very impressive, and I gotta wonder what happened with Gemini Ultra if pro with larger context beats it outright. It's indeed very good, but not THAT good if you use it om simple logic problems and don't use the whole context length. I suspect that we'll hear much more about their AI stuff during the upcoming Google IO (which I was invited to and am going to cover) Additionally, we've had quite a few AI Unicorns born, with Perplexity becoming a freshly mint Unicorn with an additional round of funding and Devin, the 6-month old agent startup getting to a 2 billion valuation 😮 This weeks Buzz (What I learned with WandB this week) It's been exactly 1 week since our conference in SF and since Joe Spisak by complete chance announced Meta LLama - 3 live on stage a few hours after it was officially announced. In this weeks buzz, I'm very happy to bring you that recording, as promised last week. I will also share that our newly announced new LLM observability tool Weave launched officially during the conference and it'll be my job to get you to use it 🙂 And shoutout to those in the ThursdAI community who already used and provided feedback, it's really helpful! AI Art & Diffusion The fine folks at FAL.ai have launched the LMsys.org for images, and called it.... IMGsys.org 🙂 It's a adversarial arena with different image generators, all hosted on Fal I assume, that lets the user choose which images are "better" which is a vague term. But it's really fun, give it a try! Tools & Hardware Rabbit R1 first impressions We finally got a tease of R1 from Rabbit, as the first customers started receiving this device (where's mine?? I didn't even get a tracking number) Based on the presentation (which I watched so you don't have to) the response time, which was one of the most talked about negative pieces of AI Pin seems very decent. We're going to see a lot of reviews, but I'm very excited about my Rabbit 👏 🐇 Apparently I wasn't as fast as I thought on the pre-order so will have to wait patiently, but meanwhile, check out this review from Riley Brown. That's the deep dive for this week, for the rest of the coverage, please listen to the episode and if you liked it, share with a friend! I'll also be traveling quite a bit in the next two months, I'll be in Seattle for MSFT BUILD, and in San Francisco (more on this soon) a couple of times, hope to meet some of you, please come say hi! 🫡 This is a public episode. If you'd like to discuss this with other subscribers or get access to bonus episodes, visit sub.thursdai.news/subscribe | |||
03 May 2024 | ThursdAI - May 2nd - New GPT2? Copilot Workspace, Evals and Vibes from Reka, LLama3 1M context (+ Nous finetune) & more AI news | 01:49:03 | |
Hey 👋 Look it May or May not be the first AI newsletter you get in May, but it's for sure going to be a very information dense one. As we had an amazing conversation on the live recording today, over 1K folks joined to listen to the first May updates from ThursdAI. As you May know by now, I just love giving the stage to folks who are the creators of the actual news I get to cover from week to week, and this week, we had again, 2 of those conversations. First we chatted with Piotr Padlewski from Reka, the author on the new Vibe-Eval paper & Dataset which they published this week. We've had Yi and Max from Reka on the show before, but it was Piotr's first time and he was super super knowledgeable, and was really fun to chat with. Specifically, as we at Weights & Biases launch a new product called Weave (which you should check out at https://wandb.me/weave) I'm getting more a LOT more interested in Evaluations and LLM scoring, and in fact, we started the whole show today with a full segment on Evals, Vibe checks and covered a new paper from Scale about overfitting. The second deep dive was with my friend Idan Gazit, from GithubNext, about the new iteration of Github Copilot, called Copilot Workspace. It was a great one, and you should definitely give that one a listen as well TL;DR of all topics covered + show notes * Scores and Evals * No notable changes, LLama-3 is still #6 on LMsys * gpt2-chat came and went (in depth chan writeup) * Scale checked for Data Contamination on GSM8K using GSM-1K (Announcement, Paper) * Vibes-Eval from Reka - a set of multimodal evals (Announcement, Paper, HF dataset) * Open Source LLMs * Gradient releases 1M context window LLama-3 finetune (X) * MaziyarPanahi/Llama-3-70B-Instruct-DPO-v0.4 (X, HF) * Nous Research - Hermes Pro 2 - LLama 3 8B (X, HF) * AI Town is running on Macs thanks to Pinokio (X) * LMStudio releases their CLI - LMS (X, Github) * Big CO LLMs + APIs * Github releases Copilot Workspace (Announcement) * AI21 - releases Jamba Instruct w/ 256K context (Announcement) * Google shows Med-Gemini with some great results (Announcement) * Claude releases IOS app and Team accounts (X) * This weeks Buzz * We're heading to SF to sponsor the biggest LLama-3 hackathon ever with Cerebral Valley (X) * Check out my video for Weave our new product, it's just 3 minutes (Youtube) * Vision & Video * Intern LM open sourced a bunch of LLama-3 and Phi based VLMs (HUB) * And they are MLXd by the "The Bloke" of MLX, Prince Canuma (X) * AI Art & Diffusion & 3D * ByteDance releases Hyper-SD - Stable Diffusion in a single inference step (Demo) * Tools & Hardware * Still haven't open the AI Pin, and Rabbit R1 just arrived, will open later today * Co-Hosts and Guests * Piotr Padlewski (@PiotrPadlewski) from Reka AI * Idan Gazit (@idangazit) from Github Next * Wing Lian (@winglian) * Nisten Tahiraj (@nisten) * Yam Peleg (@yampeleg) * LDJ (@ldjconfirmed) * Wolfram Ravenwolf (@WolframRvnwlf) * Ryan Carson (@ryancarson) Scores and Evaluations New corner in today's pod and newsletter given the focus this week on new models and comparing them to existing models. What is GPT2-chat and who put it on LMSys? (and how do we even know it's good?) For a very brief period this week, a new mysterious model appeared on LMSys, and was called gpt2-chat. It only appeared on the Arena, and did not show up on the leaderboard, and yet, tons of sleuths from 4chan to reddit to X started trying to figure out what this model was and wasn't. Folks started analyzing the tokenizer, the output schema, tried to get the system prompt and gauge the context length. Many folks were hoping that this is an early example of GPT4.5 or something else entirely. It did NOT help that uncle SAMA first posted the first tweet and then edited it to remove the - and it was unclear if he's trolling again or foreshadowing a completely new release or an old GPT-2 but retrained on newer data or something. The model was really surprisingly good, solving logic puzzles better than Claude Opus, and having quite amazing step by step thinking, and able to provide remarkably informative, rational, and relevant replies. The average output quality across many different domains places it on, at least, the same level as high-end models such as GPT-4 and Claude Opus. Whatever this model was, the hype around it made LMSYS add a clarification to their terms and temporarily take off the model now. And we're waiting to hear more news about what it is. Reka AI gives us Vibe-Eval a new multimodal evaluation dataset and score (Announcement, Paper, HF dataset) Reka keeps surprising, with only 20 people in the company, their latest Reka Core model is very good in multi modality, and to prove it, they just released a new paper + a new method of evaluating multi modal prompts on VLMS (Vision enabled Language Models) Their new Open Benchmark + Open Dataset is consistent of this format: And I was very happy to hear from one of the authors on the paper @PiotrPadlewski on the pod, where he mentioned that they were trying to create a dataset that was going to be very hard for their own model (Reka Core) and just decided to keep evaluating other models on it. They had 2 main objectives : (i) vibe checking multimodal chat models for day-to-day tasks and (ii) deeply challenging and probing the capabilities of present frontier models. To this end, the hard set contains > 50% questions that all frontier models answer incorrectly Chatting with Piotr about it, he mentioned that not only did they do a dataset, they actually used Reka Core as a Judge to score the replies from all models on that dataset and found that using their model in this way roughly correlates to non-expert human judgement! Very very interesting stuff. The "hard" set is ... well hard! Piotr concluded that if folks want to do research, they will provide free API access to Reka for that, so hit them up over DMs if you want to take this eval for a spin on your new shiny VLM (or indeed verify the metrics they put up) Scale tests for eval dataset contamination with GSM-1K (Announcement, Paper) Scale.ai is one of the most prominent companies in AI you may never have heard of, they are valued at $13B dollars and have pivoted from data processing for autonomous vehicles to being the darling of the government, with agreements from the DoD for data pipeline and evaluation for US Military. They have released a new paper as well, creating (but not releasing) a new dataset that matches the GSM8K (Grade School Math) dataset and evaluation that many frontier companies love to showcase in their release benchmarks with some surprising results! So Scale folks created (but not released) a dataset called GSK 1K, which tracks and is similar to the public GSM-8K dataset, and tested a bunch of existing models on their new one, to see the correlation, and if the different was very stark, assume that some models overfitted (or even had their dataset contaminated) on the publicly available GSM8K. On one end, models like Mistral or Phi do up to 10% worse on GSM1k compared to GSM8k. On the other end, models like Gemini, Claude, or GPT show basically no signs of being overfit. The author goes on to say that overfitting doesn't necessarily mean it's a bad model, and highlights Phi-3 which has a 10% difference on their new GSK-1K score compared to GSM-8K, but still answers 68% of their dataset, while being a tiny 3.8B parameter model. It seems that Scale is now stepping into the Evaluation game and have noticed how much interest there is in actually understanding how models perform, and are stepping into this game, by building (but not releasing so they don't leak) datasets. Jim Fan tweet (and Scale CEO Alex Wang QT) seem to agree that this is the right positioning for Scale (as they don't have models of their own and so can be neutral like Moody's) Open Source LLMs LLama-3 gets 1M context window + Other LLama-3 news In the second week of LLama-3 corner, we are noticing a significant ramp in all things Llama-3, first with the context length. The same folks from last week, Gradient, have spend cycles and upscaled/stretched LLama-3 to a whopping 1 million tokens in the context window (Llama-3 8B Gradient Instruct 1048k), with a very decent Niddle in the Haystack result. The main problem? Transformers have quadratic attention scaling issues for longer context, so this isn't something that you'd be able to run on your mac (nay, on your cluster) any time soon, and it's almost only theoretical at this point. The upside? We had Wing Lian (from Axolotl) on the show, and he talked about a new method called LoRD (which is now part of MergeKit) which is a way to extract Loras from models. Think of it as LLM arithmetic, you take the base model (llama-3 in this case) and the finetune (Llama-3 8B Gradient Instruct 1048k) and simple run a command like so: mergekit-extract-lora llama-3-8B-gradient-instruct-1048K llama-3-8B just-the-context-lora [--no-lazy-unpickle] --rank=desired_rank And boom, in theory, you have a tiny LoRA file that's extracted that is only the difference between these two models, the base and it's finetune. It's really exciting stuff to be able to do brain surgery on these models and extract only one specific essence! First LLama-3 finetunes that beat the instruct version Folks and Nous research give us a new Hermes-Pro on top of Llama-8B (X, HF) that is beating the llama-3 instruct on benchmarks, which is apparently very hard to do, given that Meta created a LOT of human labeled instructions (10M or so) and gave us a really really good instruct model. Nous Hermes 2 pro is also giving Llama-3 additional superpowers like function calling and tool use, specifically mentioning that this is the model to use if you do any type of agentic stuff This new version of Hermes maintains its excellent general task and conversation capabilities - but also excels at Function Calling, JSON Structured Outputs, and has improved on several other metrics as well, scoring a 90% on our function calling evaluation built in partnership with Fireworks.AI, and an 84% on our structured JSON Output evaluation. Kudos Teknium1, Karan and @intrstllrninja on this release, can't wait to try it out 🫡 LMStudio gives us a CLI (Github) And speaking of "trying it out", you guys know that my recommended way of running these local models is LMStudio, and no, Yagil didn't sponsor ThursdAI haha I just love how quickly this piece of software became the go to locally for me running these models. Well during ThursdAI I got a #breakingNews ping from their discord, that LM Studio now has a CLI (command line interface) which allows one to load/unload and run the webserver with the new CLI (kind of similar to Ollama) And since LM Studio exposes an OpenAI compatible completions API once the models are loaded, you are not able to use these models with a simple change to the your script like so: client = OpenAI(base_url="http://localhost:1234/v1", api_key="lm-studio") Which is amazing and I'm very happy about this option, as this opens the door to tons of automations and evaluation possibilities (with something like Weave), in fact while writing this, I downloaded the model from HuggingFace, loaded a web-server and ran my first prompts, and it all took like 5 minutes, and is very easy to do! This weeks Buzz (What happens in Weights & Biases this week) I have so much to share, but I want to make sure I don't overwhelm the newsletter, but here we go. First of all, I'm flying out to SF again! in a few weeks to sponsor and judge the first ever LLama-3 hackathon, together with Meta, and hosted by the fine folks at Cerebral Valley (sign up and come hack!) Cerebral Valley is hosting their events at this beautiful place called Shak-15 which I've mentioned before on the newsletter, and I'm excited to finally take part in one of their events! The second part I can't wait to tell you about, is a week after, I'm going to Microsoft BUILD conference in Seattle, and will be representing Weights & Biases in that huge event (which last year featured Andrej Karpathy giving state of LLMs) Here's a video I recorded for that event, which I worked really hard on, and would love some feedback. Please also let me know if you notice anything that an AI did in this video 👀 There's... something As always, if you're attending any of these events, and see me, please do come say hi and give me a high five. I love meeting ThursdAI community folks in the wild, it really makes up for the fact that I'm working remotely from Denver and really makes this whole thing worth it! Big Companies & APIs Github’s new Copilot Workspace in Technical Preview I was very happy to have friend of the pod Idan Gazit, Senior Director of Research at GitHub Next, the place in Github that comes up with incredible stuff (including where Copilot was born) to talk to us about Copilot's next iteration after the chat experience, workspace! Workspace is indeed that, a workspace for you and copilot to start working together, on github issues specifically, taking into context more than just 1 file, and breaking down the task into planning, iteration and human feedback. It looks really slick, and per Idan, uses a LOT of tokens of gpt-4-turbo, and I've had a chance to get in there and play around. They break down every task into a Specification that Copilot comes up with, and then you iteratively can work on until you get the required result, then into planning model, where you would see a whole plan, and then copilot will get to work and start iterating on your task. Does this remind you of anything? AGENTS you may yell in your head as you read these words, however, I recommend you listen to Idan in our chat on the pod, because his take on agents are, we don't want these tools to replace us, we want them to help us, and what is an agent anyway, this word is very overused. And I have to agree, given the insane valuations we've seen in agent startups like Cognition Labs with Devin. I've taken Workspace for a spin, and asked it for a basic task to translate a repo documentation into Russian, a task I know LLMs are really good at, and it identified all the README files in the repo, and translated them beautifully, but then it didn't place those new translations into a separate folder like I asked, a case Idan admitted they didn't yet build for, and hey, this is why this is a Technical Preview, you just can't build an LLM based product behind the scenes and release it, you need feedback, and evaluations on your product from actual users! You can see my whole session here, in this nice link they give to be able to share (and fork if you have access) a workspace The integration into Github is quite amazing, there's now a text box everyone on Github that you can ask for changes to a repo in natural language + a Raycast extension that allows you to basically kickstart a whole repo using Copilot Workspace from anywhere And here's the result inside a new workspace 👇 I will run this later and see if it actually worked, given that Idan also mentioned, that Copilot does NOT run the code it writes, but it does allow me to easily do so via GIthub Codespaces (a bit confusing of a naming between the two!) and spin up a machine super quick. I strongly recommend to listen to Idan on the pod because he went into a lot of detail about additional features, where they are planning to take this in the future etc' I can go on and on, but I need to play with all the amazing new tools and models we just got today (and also start editing the podcast it's almost 4PM and I have 2 hours to send it!) so with that, thank you for reading , and see you next time 🫡 This is a public episode. If you'd like to discuss this with other subscribers or get access to bonus episodes, visit sub.thursdai.news/subscribe | |||
10 May 2024 | 📅 ThursdAI - May 9 - AlphaFold 3, im-a-good-gpt2-chatbot, Open Devin SOTA on SWE-Bench, DeepSeek V2 super cheap + interview with OpenUI creator & more AI news | 01:47:51 | |
Hey 👋 (show notes and links a bit below) This week has been a great AI week, however, it does feel like a bit "quiet before the storm" with Google I/O on Tuesday next week (which I'll be covering from the ground in Shoreline!) and rumors that OpenAI is not just going to let Google have all the spotlight! Early this week, we got 2 new models on LMsys, im-a-good-gpt2-chatbot and im-also-a-good-gpt2-chatbot, and we've now confirmed that they are from OpenAI, and folks have been testing them with logic puzzles, role play and have been saying great things, so maybe that's what we'll get from OpenAI soon? Also on the show today, we had a BUNCH of guests, and as you know, I love chatting with the folks who make the news, so we've been honored to host Xingyao Wang and Graham Neubig core maintainers of Open Devin (which just broke SOTA on Swe-Bench this week!) and then we had friends of the pod Tanishq Abraham and Parmita Mishra dive deep into AlphaFold 3 from Google (both are medical / bio experts). Also this week, OpenUI from Chris Van Pelt (Co-founder & CIO at Weights & Biases) has been blowing up, taking #1 Github trending spot, and I had the pleasure to invite Chris and chat about it on the show! Let's delve into this (yes, this is I, Alex the human, using Delve as a joke, don't get triggered 😉) TL;DR of all topics covered (trying something new, my Raw notes with all the links and bulletpoints are at the end of the newsletter) * Open Source LLMs * OpenDevin getting SOTA on Swe-Bench with 21% (X, Blog) * DeepSeek V2 - 236B (21B Active) MoE (X, Try It) * Weights & Biases OpenUI blows over 11K stars (X, Github, Try It) * LLama-3 120B Chonker Merge from Maxime Labonne (X, HF) * Alignment Lab open sources Buzz - 31M rows training dataset (X, HF) * xLSTM - new transformer alternative (X, Paper, Critique) * Benchmarks & Eval updates * LLama-3 still in 6th place (LMsys analysis) * Reka Core gets awesome 7th place and Qwen-Max breaks top 10 (X) * No upsets in LLM leaderboard * Big CO LLMs + APIs * Google DeepMind announces AlphaFold-3 (Paper, Announcement) * OpenAI publishes their Model Spec (Spec) * OpenAI tests 2 models on LMsys (im-also-a-good-gpt2-chatbot & im-a-good-gpt2-chatbot) * OpenAI joins Coalition for Content Provenance and Authenticity (Blog) * Voice & Audio * Udio adds in-painting - change parts of songs (X) * 11Labs joins the AI Audio race (X) * AI Art & Diffusion & 3D * ByteDance PuLID - new high quality ID customization (Demo, Github, Paper) * Tools & Hardware * Went to the Museum with Rabbit R1 (My Thread) * Co-Hosts and Guests * Graham Neubig (@gneubig) & Xingyao Wang (@xingyaow_) from Open Devin * Chris Van Pelt (@vanpelt) from Weights & Biases * Nisten Tahiraj (@nisten) - Cohost * Tanishq Abraham (@iScienceLuvr) * Parmita Mishra (@prmshra) * Wolfram Ravenwolf (@WolframRvnwlf) * Ryan Carson (@ryancarson) Open Source LLMs Open Devin getting a whopping 21% on SWE-Bench (X, Blog) Open Devin started as a tweet from our friend Junyang Lin (on the Qwen team at Alibaba) to get an open source alternative to the very popular Devin code agent from Cognition Lab (recently valued at $2B 🤯) and 8 weeks later, with tons of open source contributions, >100 contributors, they have almost 25K stars on Github, and now claim a State of the Art score on the very hard Swe-Bench Lite benchmark beating Devin and Swe-Agent (with 18%) They have done so by using the CodeAct framework developed by Xingyao, and it's honestly incredible to see how an open source can catch up and beat a very well funded AI lab, within 8 weeks! Kudos to the OpenDevin folks for the organization, and amazing results! DeepSeek v2 - huge MoE with 236B (21B active) parameters (X, Try It) The folks at DeepSeek is releasing this huge MoE (the biggest we've seen in terms of experts) with 160 experts, and 6 experts activated per forward pass. A similar trend from the Snowflake team, just extended even longer. They also introduce a lot of technical details and optimizations to the KV cache. With benchmark results getting close to GPT-4, Deepseek wants to take the crown in being the cheapest smartest model you can run, not only in open source btw, they are now offering this model at an incredible .28/1M tokens, that's 28 cents per 1M tokens! The cheapest closest model in price was Haiku at $.25 and GPT3.5 at $0.5. This is quite an incredible deal for a model with 32K (128 in open source) context and these metrics. Also notable is the training cost, they claim that it took them 1/5 the price of what Llama-3 cost Meta, which is also incredible. Unfortunately, running this model locally a nogo for most of us 🙂 I would mention here that metrics are not everything, as this model fails quite humorously on my basic logic tests LLama-3 120B chonker Merge from Maxime LaBonne (X, HF) We're covered Merges before, and we've had the awesome Maxime Labonne talk to us at length about model merging on ThursdAI but I've been waiting for Llama-3 merges, and Maxime did NOT dissapoint! A whopping 120B llama (Maxime added 50 layers to the 70B Llama3) is doing the rounds, and folks are claiming that Maxime achieved AGI 😂 It's really funny, this model, is... something else. Here just one example that Maxime shared, as it goes into an existential crisis about a very simple logic question. A question that Llama-3 answers ok with some help, but this... I've never seen this. Don't forget that merging has no additional training, it's mixing layers from the same model so... we still have no idea what Merging does to a model but... some brain damange definitely is occuring. Oh and also it comes up with words! ThursdAI - Recaps of the most high signal AI weekly spaces is a reader-supported publication. To receive new posts and support my work, consider becoming a free or paid subscriber. Big CO LLMs + APIs Open AI publishes Model Spec (X, Spec, Blog) OpenAI publishes and invites engagement and feedback for their internal set of rules for how their models should behave. Anthropic has something similar with Constitution AI. I specifically liked the new chain of command (Platform > Developer > User > Tool) rebranding they added to the models, making OpenAI the Platform, changing "system" prompts to "developer" and having user be the user. Very welcome renaming and clarifications (h/t Swyx for his analysis) Here are a summarized version of OpenAI's new rules of robotics (thanks to Ethan Mollic) * follow the chain of command: Platform > Developer > User > Tool * Comply with applicable laws * Don't provide info hazards * Protect people's privacy * Don't respond with NSFW contents Very welcome effort from OpenAI, showing this spec in the open and inviting feedback is greately appreciated! This comes on top of a pretty big week for OpenAI, announcing an integration with Stack Overflow, Joining the Coalition for Content Provenance and Authenticity + embedding watermarks in SORA and DALL-e images, telling us they have built a classifier that detects AI images with 96% certainty! im-a-good-gpt2-chatbot and im-also-a-good-gpt2-chatbot Following last week gpt2-chat mystery, Sam Altman trolled us with this tweet And then we got 2 new models on LMSys, im-a-good-gpt2-chatbot and im-also-a-good-gpt2-chatbot, and the timeline exploded with folks trying all their best logic puzzles on these two models trying to understand what they are, are they GPT5? GPT4.5? Maybe a smaller version of GPT2 that's pretrained on tons of new tokens? I think we may see the answer soon, but it's clear that both these models are really good, doing well on logic (better than Llama-70B, and sometimes Claude Opus as well) And the speculation is pretty much over, we know OpenAI is behind them after seeing this oopsie on the Arena 😂 you can try these models as well, they seem to be very favored in the random selection of models, but they show up only in battle mode so you have to try a few times https://chat.lmsys.org/ Google DeepMind announces AlphaFold3 (Paper, Announcement) Developed by DeepMind and IsomorphicLabs, AlphaFold has previously predicted the structure of every molecule known to science, and now AlphaFold 3 was announced which can now predict the structure of other biological complexes as well, paving the way for new drugs and treatments. What's new here, is that they are using diffusion, yes, like Stable Diffusion, starting with noise and then denoising to get a structure, and this method is 50% more accurate than existing methods. If you'd like more info about this very important paper, look no further than the awesome 2 minute paper youtube, who did a thorough analysis here, and listen to the Isomorphic Labs podcast with Weights & Biases CEO Lukas on Gradient Dissent They also released AlphaFold server, a free research tool allowing scientists to access these capabilities and predict structures for non commercial use, however it seems that it's somewhat limited (from a conversation we had with a researcher on stage) This weeks Buzz (What I learned with WandB this week) This week, was amazing for Open Source and Weights & Biases, not every week a side project from a CIO blows up on... well everywhere. #1 trending on Github for Typescript and 6 overall, OpenUI (Github) has passed 12K stars as people are super excited about being able to build UIs with LLms, but in the open source. I had the awesome pleasure to host Chris on the show as he talked about the inspiration and future plans, and he gave everyone his email to send him feedback (a decision which I hope he doesn't regret 😂) so definitely check out the last part of the show for that. Meanwhile here's my quick tutorial and reaction about OpenUI, but just give it a try here and build something cool! Vision I was shared some news but respecting the team I decided not to include it in the newsletter ahead of time, but expect open source to come close to GPT4-V next week 👀 Voice & Audio 11 Labs joins the AI music race (X) Breaking news from 11Labs, that happened during the show (but we didn't notice) is that they are stepping into the AI Music scene and it sounds pretty good!) Udio adds Audio Inpainting (X, Udio) This is really exciting, Udio decided to prove their investment and ship something novel! Inpainting has been around in diffusion models, and now selecting a piece of a song on Udio and having Udio reword it is so seamless it will definitely come to every other AI music, given how powerful this is! Udio also announced their pricing tiers this week, and it seems that this is the first feature that requires subscription AI Art & Diffusion ByteDance PuLID for no train ID Customization (Demo, Github, Paper) It used to take a LONG time to finetune something like Stable Diffusion to generate an image of your face using DreamBooth, then things like LoRA started making this much easier but still needed training. The latest crop of approaches for AI art customization is called ID Customization and ByteDance just released a novel, training free version called PuLID which works very very fast with very decent results! (really, try it on your own face), previous works like InstantID an IPAdapter are also worth calling out, however PuLID seems to be the state of the art here! 🔥 And that's it for the week, well who am I kidding, there's so much more we covered and I just didn't have the space to go deep into everything, but definitely check out the podcast episode for the whole conversation. See you next week, it's going to be 🔥 because of IO and ... other things 👀 This is a public episode. If you'd like to discuss this with other subscribers or get access to bonus episodes, visit sub.thursdai.news/subscribe | |||
17 May 2024 | 📅 ThursdAI - May 16 - OpenAI GPT-4o, Google IO recap, LLama3 hackathon, Yi 1.5, Nous Hermes Merge & more AI news | 01:54:23 | |
Wow, holy s**t, insane, overwhelming, incredible, the future is here!, "still not there", there are many more words to describe this past week. (TL;DR at the end of the blogpost) I had a feeling it's going to be a big week, and the companies did NOT disappoint, so this is going to be a very big newsletter as well. As you may have read last week, I was very lucky to be in San Francisco the weekend before Google IO, to co-host a hackathon with Meta LLama-3 team, and it was a blast, I will add my notes on that in This weeks Buzz section. Then on Monday, we all got to watch the crazy announcements from OpenAI, namely a new flagship model called GPT-4o (we were right, it previously was im-also-a-good-gpt2-chatbot) that's twice faster, 50% cheaper (in English, significantly more so in other languages, more on that later) and is Omni (that's the o) which means it is end to end trained with voice, vision, text on inputs, and can generate text, voice and images on the output. A true MMIO (multimodal on inputs and outputs, that's not the official term) is here and it has some very very surprising capabilities that blew us all away. Namely the ability to ask the model to "talk faster" or "more sarcasm in your voice" or "sing like a pirate", though, we didn't yet get that functionality with the GPT-4o model, it is absolutely and incredibly exciting. Oh and it's available to everyone for free! That's GPT-4 level intelligence, for free for everyone, without having to log in! What's also exciting was how immediate it was, apparently not only the model itself is faster (unclear if it's due to newer GPUs or distillation or some other crazy advancements or all of the above) but that training an end to end omnimodel reduces the latency to incredibly immediate conversation partner, one that you can interrupt, ask to recover from a mistake, and it can hold a conversation very very well. So well, that indeed it seemed like, the Waifu future (digital girlfriends/wives) is very close to some folks who would want it, while we didn't get to try it (we got GPT-4o but not the new voice mode as Sam confirmed) OpenAI released a bunch of videos of their employees chatting with Omni (that's my nickname, use it if you'd like) and many online highlighted how thirsty / flirty it sounded. I downloaded all the videos for an X thread and I named one girlfriend.mp4, and well, just judge for yourself why: Ok, that's not all that OpenAI updated or shipped, they also updated the Tokenizer which is incredible news to folks all around, specifically, the rest of the world. The new tokenizer reduces the previous "foreign language tax" by a LOT, making the model way way cheaper for the rest of the world as well One last announcement from OpenAI was the desktop app experience, and this one, I actually got to use a bit, and it's incredible. MacOS only for now, this app comes with a launcher shortcut (kind of like RayCast) that let's you talk to ChatGPT right then and there, without opening a new tab, without additional interruptions, and it even can understand what you see on the screen, help you understand code, or jokes or look up information. Here's just one example I just had over at X. And sure, you could always do this with another tab, but the ability to do it without context switch is a huge win. OpenAI had to do their demo 1 day before GoogleIO, but even during the excitement about GoogleIO, they had announced that Ilya is not only alive, but is also departing from OpenAI, which was followed by an announcement from Jan Leike (who co-headed the superailgnment team together with Ilya) that he left as well. This to me seemed like a well executed timing to give dampen the Google news a bit. Google is BACK, backer than ever, Alex's Google IO recap On Tuesday morning I showed up to Shoreline theater in Mountain View, together with creators/influencers delegation as we all watch the incredible firehouse of announcements that Google has prepared for us. TL;DR - Google is adding Gemini and AI into all it's products across workspace (Gmail, Chat, Docs), into other cloud services like Photos, where you'll now be able to ask your photo library for specific moments. They introduced over 50 product updates and I don't think it makes sense to cover all of them here, so I'll focus on what we do best. "Google with do the Googling for you" Gemini 1.5 pro is now their flagship model (remember Ultra? where is that? 🤔) and has been extended to 2M tokens in the context window! Additionally, we got a new model called Gemini Flash, which is way faster and very cheap (up to 128K, then it becomes 2x more expensive) Gemini Flash is multimodal as well and has 1M context window, making it an incredible deal if you have any types of videos to process for example. Kind of hidden but important was a caching announcement, which IMO is a big deal, big enough it could post a serious risk to RAG based companies. Google has claimed they have a way to introduce caching of the LLM activation layers for most of your context, so a developer won't have to pay for repeatedly sending the same thing over and over again (which happens in most chat applications) and will significantly speed up work with larger context windows. They also mentioned Gemini Nano, a on device Gemini, that's also multimodal, that can monitor calls in real time for example for older folks, and alert them about being scammed, and one of the cooler announcements was, Nano is going to be baked into the Chrome browser. With Gemma's being upgraded, there's not a product at Google that Gemini is not going to get infused into, and while they counted 131 "AI" mentions during the keynote, I'm pretty sure Gemini was mentioned way more! Project Astra - A universal AI agent helpful in everyday life After a few of the announcements from Sundar, (newly knighted) Sir Demis Hassabis came out and talked about DeepMind research, AlphaFold 3 and then turned to project Astra. This demo was really cool and kind of similar to the GPT-4o conversation, but also different. I'll let you just watch it yourself: TK: project astra demo And this is no fake, they actually had booths with Project Astra test stations and I got to chat with it (I came back 3 times) and had a personal demo from Josh Woodward (VP of Labs) and it works, and works fast! It sometimes disconnects and sometimes there are misunderstandings, like when multiple folks are speaking, but overall it's very very impressive. If you remember the infamous video with the rubber ducky that was edited by Google and caused a major uproar when we found out? It's basically that, on steroids, and real and quite quite fast. Astra has a decent short term memory, so if you ask it where something was, it will remember, and Google cleverly used that trick to also show that they are working on augmented reality glasses with Astra built in, which would make amazing sense. Open Source LLMs Google open sourced PaliGemma VLM Giving us something in the open source department, adding to previous models like RecurrentGemma, Google has uploaded a whopping 116 different checkpoints of a new VLM called PaliGemma to the hub, which is a State of the Art vision model at 3B. It's optimized for finetuning for different workloads such as Visual Q&A, Image and short video captioning and even segmentation! They also mentioned that Gemma 2 is coming next month, will be a 27B parameter model that's optimized to run on a single TPU/GPU. Nous Research Hermes 2 Θ (Theta) - their first Merge! Collaborating with Charles Goddard from Arcee (the creators of MergeKit), Teknium and friends merged the recently trained Hermes 2 Pro with Llama 3 instruct to get a model that's well performant on all the tasks that LLama-3 is good at, while maintaining capabilities of Hermes (function calling, Json mode) Yi releases 1.5 with apache 2 license The folks at 01.ai release Yi 1.5, with 6B, 9B and 34B (base and chat finetunes) Showing decent benchmarks on Math and Chinese, 34B beats LLama on some of these tasks while being 2x smaller, which is very impressive This weeks Buzz - LLama3 hackathon with Meta Before all the craziness that was announced this week, I participated and judged the first ever Llama-3 hackathon. It was quite incredible, with over 350 hackers participating, Groq, Lambda, Meta, Ollama and others sponsoring and giving talks and workshops it was an incredible 24 hours at Shak 15 in SF (where Cerebral Valley hosts their hackathons) Winning hacks were really innovative, ranging from completely open source smart glasses for under 20$, to a LLM debate platform with an LLM judge on any moral issue, and one project that was able to jailbreak llama by doing some advanced LLM arithmetic. Kudos to the teams for winning, and it was amazing to see how many of them adopted Weave as their observability framework as it was really easy to integrate. Oh and I got to co-judge with the 🐐 of HuggingFace This is all the notes for this week, even though there was a LOT lot more, check out the TL;DR and see you here next week, which I'll be recording from Seattle, where I'll be participating in the Microsoft BUILD event, so we'll see Microsoft's answer to Google IO as well. If you're coming to BUILD, come by our booth and give me a high five! TL;DR of all topics covered: * OpenAI Announcements * GPT-4o * Voice mode * Desktop App * Google IO recap: * Google Gemini * Gemini 1.5 Pro: Available globally to developers with a 2-million-token context window, enabling it to handle larger and more complex tasks. * Gemini 1.5 Flash: A faster and less expensive version of Gemini, optimized for tasks requiring low latency. * Gemini Nano with Multimodality: An on-device model that processes various inputs like text, photos, audio, web content, and social videos. * Project Astra: An AI agent capable of understanding and responding to live video and audio in real-time. * Google Search * AI Overviews in Search Results: Provides quick summaries and relevant information for complex search queries. * Video Search with AI: Allows users to search by recording a video, with Google's AI processing it to pull up relevant answers. * Google Workspace * Gemini-powered features in Gmail, Docs, Sheets, and Meet: Including summarizing conversations, providing meeting highlights, and processing data requests. * "Chip": An AI teammate in Google Chat that assists with various tasks by accessing information across Google services. * Google Photos * "Ask Photos": Allows users to search for specific items in photos using natural language queries, powered by Gemini. * Video Generation * Veo Generative Video: Creates 1080p videos from text prompts, offering cinematic effects and editing capabilities. * Other Notable AI Announcements * NotebookLM: An AI tool to organize and interact with various types of information (documents, PDFs, notes, etc.), allowing users to ask questions about the combined information. * Video Overviews (Prototyping): A feature within NotebookLM that generates audio summaries from uploaded documents. * Code VR: A generative video AI model capable of creating high-quality videos from various prompts. * AI Agents: A demonstration showcasing how AI agents could automate tasks across different software and systems. * Generative Music: Advancements in AI music generation were implied but not detailed. * Open Source LLMs * Google PaliGemma 3B - sota open base VLM (Blog) * Gemma 2 - 27B coming next month * Hermes 2 Θ (Theta) - Merge of Hermes Pro & Llama-instruct (X, HF) * Yi 1.5 - Apache 2 licensed 6B, 9B and 34B (X) * Tiger Lab - MMLU-pro - a harder MMLU with 12K questions (X, HuggingFace) * This weeks Buzz (What I learned with WandB this week) * Llama3 hackathon with Meta, Cerebral Valley, HuggingFace and Weights & Biases * Vision & Video * Google announces VEO - High quality cinematic generative video generation (X) * AI Art & Diffusion & 3D * Google announces Imagen3 - their latest Gen AI art model (Blog) * Tools * Cursor trained a model that does 1000tokens/s and editing 😮 (X) This is a public episode. If you'd like to discuss this with other subscribers or get access to bonus episodes, visit sub.thursdai.news/subscribe | |||
23 May 2024 | 📅 ThursdAI - May 23 - OpenAI troubles, Microsoft Build, Phi-3 small/large, new Mistral & more AI news | 01:43:00 | |
Hello hello everyone, this is Alex, typing these words from beautiful Seattle (really, it only rained once while I was here!) where I'm attending Microsoft biggest developer conference BUILD. This week we saw OpenAI get in the news from multiple angles, none of them positive and Microsoft clapped back at Google from last week with tons of new AI product announcements (CoPilot vs Gemini) and a few new PCs with NPU (Neural Processing Chips) that run alongside CPU/GPU combo we're familiar with. Those NPUs allow for local AI to run on these devices, making them AI native devices! While I'm here I also had the pleasure to participate in the original AI tinkerers thanks to my friend Joe Heitzberg who operates and runs the aitinkerers.org (of which we are a local branch in Denver) and it was amazing to see tons of folks who listen to ThursdAI + read the newsletter and talk about Weave and evaluations with all of them! (Btw, one the left is Vik from Moondream, which we covered multiple times). I Ok let's get to the news: TL;DR of all topics covered: * Open Source LLMs * HuggingFace commits 10M in ZeroGPU (X) * Microsoft open sources Phi-3 mini, Phi-3 small (7B) Medium (14B) and vision models w/ 128K context (Blog, Demo) * Mistral 7B 0.3 - Base + Instruct (HF) * LMSys created a "hard prompts" category (X) * Cohere for AI releases Aya 23 - 3 models, 101 languages, (X) * Big CO LLMs + APIs * Microsoft Build recap - New AI native PCs, Recall functionality, Copilot everywhere * Will post a dedicated episode to this on Sunday * OpenAI pauses GPT-4o Sky voice because Scarlet Johansson complained * Microsoft AI PCs - Copilot+ PCs (Blog) * Anthropic - Scaling Monosemanticity paper - about mapping the features of an LLM (X, Paper) * Vision & Video * OpenBNB - MiniCPM-Llama3-V 2.5 (X, HuggingFace) * Voice & Audio * OpenAI pauses Sky voice due to ScarJo hiring legal counsel * Tools & Hardware * Humane is looking to sell (blog) Open Source LLMs Microsoft open sources Phi-3 mini, Phi-3 small (7B) Medium (14B) and vision models w/ 128K context (Blog, Demo) Just in time for Build, Microsoft has open sourced the rest of the Phi family of models, specifically the small (7B) and the Medium (14B) models on top of the mini one we just knew as Phi-3. All the models have a small context version (4K and 8K) and a large that goes up to 128K (tho they recommend using the small if you don't need that whole context) and all can run on device super quick. Those models have MIT license, so use them as you will, and are giving an incredible performance comparatively to their size on benchmarks. Phi-3 mini, received an interesting split in the vibes, it was really good for reasoning tasks, but not very creative in it's writing, so some folks dismissed it, but it's hard to dismiss these new releases, especially when the benchmarks are that great! LMsys just updated their arena to include a hard prompts category (X) which select for complex, specific and knowledge based prompts and scores the models on those. Phi-3 mini actually gets a big boost in ELO ranking when filtered on hard prompts and beats GPT-3.5 😮 Can't wait to see how the small and medium versions perform on the arena. Mistral gives us function calling in Mistral 0.3 update (HF) Just in time for the Mistral hackathon in Paris, Mistral has released an update to the 7B model (and likely will update the MoE 8x7B and 8x22B Mixtrals) with function calling and a new vocab. This is awesome all around because function calling is important for agenting capabilities, and it's about time all companies have it, and apparently the way Mistral has it built in matches the Cohere Command R way and is already supported in Ollama, using raw mode. Big CO LLMs + APIs Open AI is not having a good week - Sky voice has paused, Employees complain OpenAI is in hot waters this week, starting with pausing the Sky voice (arguably the best most natural sounding voice out of the ones that launched) due to complains for Scarlett Johansson about this voice being similar to hers. Scarlett appearance in the movie Her, and Sam Altman tweeting "her" to celebrate the release of the incredible GPT-4o voice mode were all talked about when ScarJo has released a statement saying she was shocked when her friends and family told her that OpenAI's new voice mode sounds just like her. Spoiler, it doesn't really, and they hired an actress and have had this voice out since September last year, as they outlined in their blog following ScarJo complaint. Now, whether or not there's legal precedent here, given that Sam Altman reached out to Scarlet twice, including once a few days before the event, I won't speculate, but for me, personally, not only Sky doesn't sound like ScarJo, it was my favorite voice even before they demoed it, and I'm really sad that it's paused, and I think it's unfair to the actress who was hired for her voice. See her own statement: Microsoft Build - CoPilot all the things I have recorded a Built recap with Ryan Carson from Intel AI and will be posting that as it's own episode on Sunday, so look forward to that, but for now, here are the highlights from BUILD: * Copilot everywhere, Microsoft builds the CoPilot as a platform * AI native laptops with NPU chips for local AI * Recall an on device AI that let's you search through everything you saw or typed with natural language * Github Copilot Workspace + Extensions * Microsoft stepping into education with sponsoring Khan Academy free for all teaches in the US * Copilot Team member and Agent - Copilot will do things proactively as your team member * GPT-4o voice mode is coming to windows and to websites! Hey, if you like reading this, can you share with 1 friend? It’ll be an awesome way to support this pod/newsletter! Anthropic releases the Scaling Monosemanticity paper This is quite a big thing that happened this week for Mechanistic Interpretability and Alignment, with Anthropic releasing a new paper and examples of their understanding of what LLM "thinks". They have done incredible work in this area, and now they have scaled it up all the way to production models like Claude Haiku, which shows that this work can actually understand which "features" are causing which tokens to output. In the work they highlighted features such as "deception", "bad code" and even a funny one called "Golden Gate bridge" and showed that clamping these features can affect the model outcomes. One these features have been identified, they can be turned on or off with various levels of power, for example they turned up the Golden Gate Bridge feature up to the maximum, and the model thought it was the Golden Gate bridge. While a funny example, they also found features for racism, bad / wrong code, inner conflict, gender bias, sycophancy and more, you can play around with some examples here and definitely read the full blog if this interests you, but overall it shows incredible promise in alignment and steer-ability of models going forward on large scale This weeks Buzz (What I learned with WandB this week) I was demoing Weave all week long in Seattle, first at the AI Tinkerers event, and then at MSFT BUILD. They had me record a pre-recorded video of my talk, and then have a 5 minute demo on stage, which (was not stressful at all!) so here's the pre-recorded video that turned out really good! Also, we're sponsoring the Mistral Hackathon this weekend in Paris, so if you're in EU and want to hack with us, please go, it's hosted by Cerebral Valley and HuggingFace and us → Vision Phi-3 mini Vision In addition to Phi-3 small and Phi-3 Medium, Microsoft released Phi-3 mini with vision, which does an incredible job understanding text and images! (You can demo it right here) Interestingly, the Phi-3 mini with vision has 128K context window which is amazing and even beats Mistral 7B as a language model! Give it a try OpenBNB - MiniCPM-Llama3-V 2.5 (X, HuggingFace, Demo) Two state of the art vision models in one week? well that's incredible. A company I haven't heard of OpenBNB have released MiniCPM 7B trained on top of LLama3 and they claim that they outperform the Phi-3 vision They claim that it has GPT-4 vision level performance and achieving an 700+ score on OCRBench, surpassing proprietary models such as GPT-4o, GPT-4V-0409, Qwen-VL-Max and Gemini Pro In my tests, Phi-3 performed a bit better, I showed both the same picture, and Phi was more factual on the hard prompts: Phi-3 Vision: And that's it for this week's newsletter, look out for the Sunday special full MSFT Build recap and definitely give the whole talk a listen, it's full of my co-hosts and their great analysis of this weeks events! This is a public episode. If you'd like to discuss this with other subscribers or get access to bonus episodes, visit sub.thursdai.news/subscribe | |||
31 May 2024 | 📅 ThursdAI - May 30 - 1000 T/s inference w/ SambaNova, <135ms TTS with Cartesia, SEAL leaderboard from Scale & more AI news | 01:52:52 | |
Hey everyone, Alex here! Can you believe it's already end of May? And that 2 huge AI companies conferences are behind us (Google IO, MSFT Build) and Apple's WWDC is just ahead in 10 days! Exciting! I was really looking forward to today's show, had quite a few guests today, I'll add all their socials below the TL;DR so please give them a follow and if you're only in reading mode of the newsletter, why don't you give the podcast a try 🙂 It's impossible for me to add the density of knowledge that's being shared on stage for 2 hours here in the newsletter! Also, before we dive in, I’m hosting a free workshop soon, about building evaluations from scratch, if you’re building anything with LLMs in production, more than welcome to join us on June 12th (it’ll be virtual) TL;DR of all topics covered: * Open Source LLMs * Mistral open weights Codestral - 22B dense coding model (X, Blog) * Nvidia open sources NV-Embed-v1 - Mistral based SOTA embeddings (X, HF) * HuggingFace Chat with tool support (X, demo) * Aider beats SOTA on Swe-Bench with 26% (X, Blog, Github) * OpenChat - Sota finetune of Llama3 (X, HF, Try It) * LLM 360 - K2 65B - fully transparent and reproducible (X, Paper, HF, WandB) * Big CO LLMs + APIs * Scale announces SEAL Leaderboards - with private Evals (X, leaderboard) * SambaNova achieves >1000T/s on Llama-3 full precision * Groq hits back with breaking 1200T/s on Llama-3 * Anthropic tool support in GA (X, Blogpost) * OpenAI adds GPT4o, Web Search, Vision, Code Interpreter & more to free users (X) * Google Gemini & Gemini Flash are topping the evals leaderboards, in GA(X) * Gemini Flash finetuning coming soon * This weeks Buzz (What I learned at WandB this week) * Sponsored a Mistral hackathon in Paris * We have an upcoming workshop in 2 parts - come learn with me * Vision & Video * LLama3-V - Sota OSS VLM (X, Github) * Voice & Audio * Cartesia AI - super fast SSM based TTS with very good sounding voices (X, Demo) * Tools & Hardware * Jina Reader (https://jina.ai/reader/) * Co-Hosts and Guests * Rodrigo Liang (@RodrigoLiang) & Anton McGonnell (@aton2006) from SambaNova * Itamar Friedman (@itamar_mar) Codium * Arjun Desai (@jundesai) - Cartesia * Nisten Tahiraj (@nisten) - Cohost * Wolfram Ravenwolf (@WolframRvnwlf) * Eric Hartford (@erhartford) * Maziyar Panahi (@MaziyarPanahi) Scale SEAL leaderboards (Leaderboard) Scale AI has announced their new initiative, called SEAL leaderboards, which aims to provide yet another point of reference in how we understand frontier models and their performance against each other. We've of course been sharing LMSys arena rankings here, and openLLM leaderboard from HuggingFace, however, there are issues with both these approaches, and Scale is approaching the measuring in a different way, focusing on very private benchmarks and dataset curated by their experts (Like Riley Goodside) The focus of SEAL is private and novel assessments across Coding, Instruction Following, Math, Spanish and more, and the main reason they keep this private, is so that models won't be able to train on these benchmarks if they leak to the web, and thus show better performance due to data contamination. They are also using ELO scores (Bradley-Terry) and I love this footnote from the actual website: "To ensure leaderboard integrity, we require that models can only be featured the FIRST TIME when an organization encounters the prompts" This means they are taking the contamination thing very seriously and it's great to see such dedication to being a trusted source in this space. Specifically interesting also that on their benchmarks, GPT-4o is not better than Turbo at coding, and definitely not by 100 points like it was announced by LMSys and OpenAI when they released it! Gemini 1.5 Flash (and Pro) in GA and showing impressive performance As you may remember from my Google IO recap, I was really impressed with Gemini Flash, and I felt that it went under the radar for many folks. Given it's throughput speed, 1M context window, and multimodality and price tier, I strongly believed that Google was onto something here. Well this week, not only was I proven right, I didn't actually realize how right I was 🙂 as we heard breaking news from Logan Kilpatrick during the show, that the models are now in GA, and that Gemini Flash gets upgraded to 1000 RPM (requests per minute) and announced that finetuning is coming and will be free of charge! Not only with finetuning won't cost you anything, inference on your tuned model is going to cost the same, which is very impressive. There was a sneaky price adjustment from the announced pricing to the GA pricing that upped the pricing by 2x on output tokens, but even despite that, Gemini Flash with $0.35/1MTok for input and $1.05/1MTok on output is probably the best deal there is right now for LLMs of this level. This week it was also confirmed both on LMsys, and on Scale SEAL leaderboards that Gemini Flash is a very good coding LLM, beating Claude Sonnet and LLama-3 70B! SambaNova + Groq competing at 1000T/s speeds What a week for inference speeds! SambaNova (an AI startup with $1.1B in investment from Google Ventures, Intel Capital, Samsung, Softbank founded in 2017) has announced that they broke the 1000T/s inference barrier on Llama-3-8B in full precision mode (suing their custom hardware called RDU (reconfigurable dataflow unit) As you can see, this is incredible fast, really, try it yourself here. Seeing this, the folks at Groq, who had the previous record on super fast inference (as I reported just in February) decided to not let this slide, and released an incredible 20% improvement on their own inference of LLama-3-8B, getting to 1200Ts, showing that they are very competitive. This bump in throughput is really significant, many inference providers that use GPUs, and not even hitting 200T/s, and Groq improved their inference by that amount within 1 day of being challenged. I had the awesome pleasure to have Rodrigo the CEO on the show this week to chat about SambaNova and this incredible achievement, their ability to run this in full precision, and future plans, so definitely give it a listen. This weeks Buzz (What I learned with WandB this week) This week was buzzing at Weights & Biases! After co-hosting a Hackathon with Meta a few weeks ago, we cohosted another Hackathon, this time with Mistral, in Paris. (where we also announced our new integration with their Finetuning!) The organizers Cerebral Valley have invited us to participate and it was amazing to see the many projects that use WandB and Weave in their finetuning presentations, including a friend of the pod Maziyar Panahi who's team nabbed 2nd place (you can read about their project here) 👏 Also, I'm going to do a virtual workshop together with my colleague Anish, about prompting and building evals, something we know a thing or two about, it's free and I would very much love to invite you to register and learn with us! Cartesia AI (try it) Hot off the press, we're getting a new Audio TTS model, based on the State Space model architecture (remember Mamba?) from a new startup called Cartesia AI, who aim to bring real time intelligence to on device compute! The most astonishing thing they released was actually the speed with which they model starts to generate voices, under 150ms, which is effectively instant, and it's a joy to play with their playground, just look at how fast it started generating this intro I recorded using their awesome 1920's radio host voice Co-founded by Albert Gu, Karan Goel and Arjun Desai (who joined the pod this week) they have shown incredible performance but also showed that transformer alternative architectures like SSMs can really be beneficial for audio specifically, just look at this quote! On speech, a parameter-matched and optimized Sonic model trained on the same data as a widely used Transformer improves audio quality significantly (20% lower perplexity, 2x lower word error, 1 point higher NISQA quality). With lower latency (1.5x lower time-to-first-audio), faster inference speed (2x lower real-time factor) and higher throughput (4x) In Open Source news: Mistral released Codestral 22B - their flagship code model with a new non commercial license Codestral is now available under the new Mistral license for non-commercial R&D use. With a larger context window of 32K, Codestral outperforms all other models in RepoBench, a long-range evaluation for code generation. Its fill-in-the-middle capability is favorably compared to DeepSeek Coder 33B. Codestral is supported in VSCode via a plugin and is accessible through their API, Le Platforme, and Le Chat. HuggingFace Chat with tool support (X, demo) This one is really cool, HF added Cohere's Command R+ with tool support and the tools are using other HF spaces (with ZeroGPU) to add capabilities like image gen, image editing, web search and more! LLM 360 - K2 65B - fully transparent and reproducible (X, Paper, HF, WandB) The awesome team at LLM 360 released K2 65B, which is an open source model that comes very close to LLama70B on benchmarks, but the the most important thing, is that they open source everything, from code, to datasets, to technical write-ups, they even open sourced their WandB plots 👏 This is so important to the open source community, that we must highlight and acknowledge the awesome effort from LLM360 ai of doing as much open source! Tools - Jina reader In the tools category, while we haven't discussed this on the pod, I really wanted to highlight Jina reader. We've had Bo from Jina AI talk to us about Embeddings in the past episodes, and since then Jina folks released this awesome tool that's able to take any URL and parse it in a nice markdown format that's very digestable to LLMs. You can pass any url, and it even does vision understanding! And today they released PDF understanding as well so you can pass the reader PDF files and have it return a nicely formatted text! The best part, it's free! (for now at least!) And that’s a wrap for today, see you guys next week, and if you found any of this interesting, please share with a friend 🙏 This is a public episode. If you'd like to discuss this with other subscribers or get access to bonus episodes, visit sub.thursdai.news/subscribe | |||
07 Jun 2024 | 📅 ThursdAI - Jun 6th - 👑 Qwen2 Beats Llama-3! Jina vs. Nomic for Multimodal Supremacy, new Chinese SORA, Suno & Udio user uploads & more AI news | 01:43:45 | |
Hey hey! This is Alex! 👋 Some podcasts have 1 or maaaybe 2 guests an episode, we had 6! guests today, each has had an announcement, an open source release, or a breaking news story that we've covered! (PS, this edition is very multimodal so click into the Substack as videos don't play in your inbox) As you know my favorite thing is to host the folks who make the news to let them do their own announcements, but also, hitting that BREAKING NEWS button when something is actually breaking (as in, happened just before or during the show) and I've actually used it 3 times this show! It's not every week that we get to announce a NEW SOTA open model with the team that worked on it. Junyang (Justin) Lin from Qwen is a friend of the pod, a frequent co-host, and today gave us the breaking news of this month, as Qwen2 72B, is beating LLama-3 70B on most benchmarks! That's right, a new state of the art open LLM was announced on the show, and Justin went deep into details 👏 (so don't miss this conversation, listen to wherever you get your podcasts) We also chatted about SOTA multimodal embeddings with Jina folks (Bo Wand and Han Xiao) and Zach from Nomic, dove into an open source compute grant with FALs Batuhan Taskaya and much more! TL;DR of all topics covered: * Open Source LLMs * Alibaba announces Qwen 2 - 5 model suite (X, HF) * Jina announces Jina-Clip V1 - multimodal embeddings beating CLIP from OAI (X, Blog, Web Demo) * Nomic announces Nomic-Embed-Vision (X, BLOG) * MixEval - arena style rankings with Chatbot Arena model rankings with 2000× less time (5 minutes) and 5000× less cost ($0.6) (X, Blog) * Vision & Video * Kling - open access video model SORA competitor from China (X) * This Weeks Buzz * WandB supports Mistral new finetuning service (X) * Register to my June 12 workshop on building Evals with Weave HERE * Voice & Audio * StableAudio Open - X, BLOG, TRY IT * Suno launches "upload your audio" feature to select few - X * Udio - upload your own audio feature - X * AI Art & Diffusion & 3D * Stable Diffusion 3 weights are coming on June 12th (Blog) * JasperAI releases Flash Diffusion (X, TRY IT, Blog) * Big CO LLMs + APIs * Group of ex-OpenAI sign a new letter - righttowarn.ai * A hacker releases TotalRecall - a tool to extract all the info from MS Recall Feature (Github) Open Source LLMs QWEN 2 - new SOTA open model from Alibaba (X, HF) This is definitely the biggest news for this week, as the folks at Alibaba released a very surprising and super high quality suite of models, spanning from a tiny 0.5B model to a new leader in open models, Qwen 2 72B To add to the distance from Llama-3, these new models support a wide range of context length, all large, with 7B and 72B support up to 128K context. Justin mentioned on stage that actually finding sequences of longer context lengths is challenging, and this is why they are only at 128K. In terms of advancements, the highlight is advanced Code and Math capabilities, which are likely to contribute to overall model advancements across other benchmarks as well. It's also important to note that all models (besides the 72B) are now released with Apache 2 license to help folks actually use globally, and speaking of globality, these models have been natively trained with 27 additional languages, making them considerably better at multilingual prompts! One additional amazing thing was, that a finetune was released by Eric Hartford and Cognitive Computations team, and AFAIK this is the first time a new model drops with an external finetune. Justing literally said "It is quite amazing. I don't know how they did that. Well, our teammates don't know how they did that, but, uh, it is really amazing when they use the Dolphin dataset to train it." Here's the Dolphin finetune metrics and you can try it out here ThursdAI - Recaps of the most high signal AI weekly spaces is a reader-supported publication. To receive new posts and support my work, consider becoming a free or paid subscriber. Jina-Clip V1 and Nomic-Embed-Vision SOTA multimodal embeddings It's quite remarkable that we got 2 separate SOTA of a similar thing during the same week, and even more cool that both companies came to talk about it on ThursdAI! First we welcomed back Bo Wang from Jina (who joined by Han Xiao the CEO) and Bo talked about multimodal embeddings that beat OpenAI CLIP (which both conceded was a very low plank) Jina Clip V1 is apache 2 open sourced, while Nomic Embed is beating it on benchmarks but is CC-BY-NC non commercially licensed, but in most cases, if you're embedding, you'd likely use an API, and both companies offer these embeddings via their respective APIs One thing to note about Nomic, is that they have mentioned that these new embeddings are backwards compatible with the awesome Nomic embed endpoints and embeddings, so if you've used that, now you've gone multimodal! Because these models are fairly small, there are now web versions, thanks to transformer.js, of Jina and Nomic Embed (caution, this will download large-ish files) built by non other than our friend Xenova. If you're building any type of multimodal semantic search, these two embeddings systems are now open up all your RAG needs for multi modal data! This weeks Buzz (What I learned with WandB this week) Mistral announced built in finetuning server support, and has a simple WandB integration! (X) Also, my workshop about building evals 101 is coming up next week, June 12, excited to share with you a workshop that we wrote for in person crowd, please register here and hope to see you next week! Vision & Video New SORA like video generation model called KLING in open access (DEMO) This one has to be seen to be believed, out of nowhere, an obscure (to us) chinese company kuaishou.com dropped a landing page with tons of videos that are clearly AI generated, and they all look very close to SORA quality, way surpassing everything else we've seen in this category (Runaway, Pika, SVD) And they claim that they offer support for it via their App (but you need apparently a Chinese phone number, so not for me) It's really hard to believe that this quality exists already outside of a frontier lab full of GPUs like OpenAI and it's now in waitlist mode, where as SORA is "coming soon" Voice & Audio Stability open sources Stable Audio Open (X, BLOG, TRY IT) A new open model from Stability is always fun, and while we wait for SD3 to drop weights (June 12! we finally have a date) we get this awesome model from Dadabots at team at Stability. It's able to generate 47s seconds of music, and is awesome at generating loops, drums and other non vocal stuff, so not quite where Suno/Udio are, but the samples are very clean and sound very good. Prompt: New York Subway They focus the model on being able to get Finetuned on a specific drummers style for example, and have it be open and specialize in samples, and sound effects and not focused on melodies or finalized full songs but it has some decent skills in simple prompts, like "progressive house music" This model has a non commercial license and can be played with here Suno & Udio let users upload their own audio! This one is big, so big in fact, that I am very surprised that both companies announced this exact feature the same week. Suno has reached out to me and a bunch of other creators, and told us that we are now able to upload our own clips, be it someone playing solo guitar, or even whistling and have Suno remix it into a real proper song. In this example, this is a very viral video, this guy sings at a market selling fish (to ladies?) and Suno was able to create this remix for me, with the drop, the changes in his voice, the melody, everything, it’s quite remarkable! AI Art & Diffusion Flash Diffusion from JasperAI / Clipdrop team (X, TRY IT, Blog, Paper) Last but definitely not least, we now have a banger of a diffusion update, from the Clipdrop team (who was amazing things before Stability bought them and then sold them to JasperAI) Diffusion models likle Stable Diffusion often take 30-40 inference steps to get you the image, searching for your prompt through latent space you know? Well recently there have been tons of these new distill methonds, models that are like students, who learn from the teacher model (Stable Diffusion XL for example) and distill the same down to a few steps (sometimes as low as 2!) Often the results are, distilled models that can run in real time, like SDXL Turbo, Lightning SDXL etc Now Flash Diffusion achieves State-of-the-Art (SOTA) performance metrics, specifically in terms of Fréchet Inception Distance (FID) and CLIP Score. These metrics are the default for evaluating the quality and relevance of generated images. And Jasper has open sourced the whole training code to allow for reproducibility which is very welcome! Flash diffusion also comes in not only image generation, but also inpaining and upscaling, allowing it to be applied to other methods to speed them up as well. — This is all for this week, I mean, there are TONS more stuff we could have covered, and we did mention them on the pod, but I aim to serve as a filter to the most interesting things as well so, until next week 🫡 This is a public episode. If you'd like to discuss this with other subscribers or get access to bonus episodes, visit sub.thursdai.news/subscribe | |||
13 Jun 2024 | ThursdAI - June 13th, 2024 - Apple Intelligence recap, Elons reaction, Luma's Dream Machine, AI Engineer invite, SD3 & more AI news from this past week | 01:46:25 | |
Happy Apple AI week everyone (well, those of us who celebrate, some don't) as this week we finally got told what Apple is planning to do with this whole generative AI wave and presented Apple Intelligence (which is AI, get it? they are trying to rebrand AI!) This weeks pod and newsletter main focus will be Apple Intelligence of course, as it was for most people compared to how the market reacted ($APPL grew over $360B in a few days after this announcement) and how many people watched each live stream (10M at the time of this writing watched the WWDC keynote on youtube, compared to 4.5 for the OpenAI GPT-4o, 1.8 M for Google IO) On the pod we also geeked out on new eval frameworks and benchmarks including a chat with the authors of MixEvals which I wrote about last week and a new benchmark called Live Bench from Abacus and Yan Lecun Plus a new video model from Luma and finally SD3, let's go! 👇 TL;DR of all topics covered: * Apple WWDC recap and Apple Intelligence (X) * This Weeks Buzz * AI Engineer expo in SF (June 25-27) come see my talk, it's going to be Epic (X, Schedule) * Open Source LLMs * Microsoft Samba - 3.8B MAMBA + Sliding Window Attention beating Phi 3 (X, Paper) * Sakana AI releases LLM squared - LLMs coming up with preference algorithms to train better LLMS (X, Blog) * Abacus + Yan Lecun release LiveBench.AI - impossible to game benchmark (X, Bench * Interview with MixEval folks about achieving 96% arena accuracy with 5000x less price * Big CO LLMs + APIs * Mistral announced a 600M series B round * Revenue at OpenAI DOUBLED in the last 6 month and is now at $3.4B annualized (source) * Elon drops lawsuit vs OpenAI * Vision & Video * Luma drops DreamMachine - SORA like short video generation in free access (X, TRY IT) * AI Art & Diffusion & 3D * Stable Diffusion Medium weights are here (X, HF, FAL) * Tools * Google releases GenType - create an alphabet with diffusion Models (X, Try It) Apple Intelligence Technical LLM details Let's dive right into what wasn't show on the keynote, in a 6 minute deep dive video from the state of the union for developers and in a follow up post on machine learning blog, Apple shared some very exciting technical details about their on device models and orchestration that will become Apple Intelligence. Namely, on device they have trained a bespoke 3B parameter LLM, which was trained on licensed data, and uses a bunch of very cutting edge modern techniques to achieve quite an incredible on device performance. Stuff like GQA, Speculative Decoding, a very unique type of quantization (which they claim is almost lossless) To maintain model , we developed a new framework using LoRA adapters that incorporates a mixed 2-bit and 4-bit configuration strategy — averaging 3.5 bits-per-weight — to achieve the same accuracy as the uncompressed models [...] on iPhone 15 Pro we are able to reach time-to-first-token latency of about 0.6 millisecond per prompt token, and a generation rate of 30 tokens per second These small models (they also have a bespoke image diffusion model as well) are going to be finetuned with a lot of LORA adapters for specific tasks like Summarization, Query handling, Mail replies, Urgency and more, which gives their foundational models the ability to specialize itself on the fly to the task at hand, and be cached in memory as well for optimal performance. Personal and Private (including in the cloud) While these models are small, they will also benefit from 2 more things on device, a vector store of your stuff (contacts, recent chats, calendar, photos) they call semantic index and a new thing apple is calling App Intents, which developers can expose (and the OS apps already do) that will allows the LLM to use tools like moving files, extracting data across apps, and do actions, this already makes the AI much more personal and helpful as it has in its context things about me and what my apps can do on my phone. Handoff to the Private Cloud (and then to OpenAI) What the local 3B LLM + context can't do, it'll hand off to the cloud, in what Apple claims is a very secure way, called Private Cloud, in which they will create a new inference techniques in the cloud, on Apple Silicon, with Secure Enclave and Secure Boot, ensuring that the LLM sessions that run inference on your data are never stored, and even Apple can't access those sessions, not to mention train their LLMs on your data. Here are some benchmarks Apple posted for their On-Device 3B model and unknown size server model comparing it to GPT-4-Turbo (not 4o!) on unnamed benchmarks they came up with. In cases where Apple Intelligence cannot help you with a request (I'm still unclear when this actually would happen) IOS will now show you this dialog, suggesting you use chatGPT from OpenAI, marking a deal with OpenAI (in which apparently nobody pays nobody, so neither Apple is getting paid by OpenAI to be placed there, nor does Apple pay OpenAI for the additional compute, tokens, and inference) Implementations across the OS So what will people be able to actually do with this intelligence? I'm sure that Apple will add much more in the next versions of iOS, but at least for now, Siri is getting an LLM brain transplant and is going to be much more smarter and capable, from understanding natural speech better (and just, having better ears, the on device speech to text is improved and is really good now in IOS 18 beta) to being able to use app intents to do actions for you across several apps. Other features across the OS will use Apple Intelligence to prioritize your notifications, and also summarize group chats that are going off, and have built in tools for rewriting, summarizing, and turning any text anywhere into anything else. Basically think of many of the tasks you'd use chatGPT for, are now built into the OS level itself for free. Apple is also adding AI Art diffusion features like GenMoji (the ability to generate any emoji you can think of, like chefs kiss, or a seal with a clown nose) and while this sounds absurd, I've never been in a slack or a discord that didn't have their own unique custom emojis uploaded by their members. And one last feature I'll highlight is this Image Playground, Apple's take on generating images, which is not only just text, but a contextual understanding of your conversation, and let's you create with autosuggested concepts instead of just text prompts and is going to be available to all developers to bake into their apps. Elon is SALTY - and it's not because of privacy I wasn't sure if to include this segment, but in what became my most viral tweet since the beginning of this year, I posted about Elon muddying the water about what Apple actually announced, and called it a Psyop that worked. Many MSMs and definitely the narrative on X, turned into what Elon thinks about those announcements, rather than the announcements themselves and just look at this insane reach. We've covered Elon vs OpenAI before (a lawsuit that he actually withdrew this week, because emails came out showing he knew and was ok with OpenAI not being Open) and so it's no surprise that when Apple decided to partner with OpenAI and not say... XAI, Elon would promote absolutely incorrect and ignorant takes to take over the radio waves like he will ban apple devices from all his companies, or that OpenAI will get access to train on your iPhone data. This weeks BUZZ (Weights & Biases Update) Hey, if you're reading this, it's very likely that you've already registered or at least heard of ai.engineer and if you haven't, well I'm delighted to tell you, that we're sponsoring this awesome event in San Francisco June 25-27. Not only are we official sponsors, both Lukas (the Co-Founder and CEO) and I will be there giving talks (mine will likely be crazier than his) and we'll have a booth there, so if your'e coming, make sure to come by my talk (or Lukas's if you're a VP and are signed up for that exclusive track) Everyone in our corder of the world is going to be there, Swyx told me that many of the foundational models labs are coming, OpenAI, Anthropic, Google, and there's going to be tons of tracks (My talk is of course in the Evals track, come, really, I might embarrass myself on stage to eternity you don't want to miss this) Swyx kindly provided listeners and readers of ThursdAI with a special coupon feeltheagi so even more of a reason to try and convince your boss and come see me on stage in a costume (I've said too much!) Vision & Video Luma drops DreamMachine - SORA like short video generation in free access (X, TRY IT) In an absolute surprise, Luma AI, a company that (used to) specialize in crafting 3D models, has released a free access video model similar to SORA, and Kling (which we covered last week) that generates 5 second videos (and doesn't require a chinese phone # haha) It's free to try, and supports text to video, image to video, cinematic prompt instructions, great and cohesive narrative following, character consistency and a lot more. Here's a comparison of the famous SORA videos and LDM (Luma Dream Machine) videos that I was provided on X by a AmebaGPT, however, worth noting that these are cherry picked SORA videos while LDM is likely a much smaller and quicker model and that folks are creating some incredible things already! AI Art & Diffusion & 3D Stable Diffusion Medium weights are here (X, HF, FAL) It's finally here (well, I'm using finally carefully here, and really hoping that this isn't the last thing Stability AI releases) ,the weights for Stable Diffusion 3 are available on HuggingFace! SD3 offers improved photorealism and awesome prompt adherence, like asking for multiple subjects doing multiple things. It's also pretty good at typography and fairly resource efficient compared to previuos versions, though I'm still waiting for the super turbo distilled versions that will likely come soon! ThursdAI - Recaps of the most high signal AI weekly spaces is a reader-supported publication. To receive new posts and support my work, consider becoming a free or paid subscriber. And that's it for this week folks, it's been a hell of a week, I really do appreciate each and one of you who makes it to the end reading, engaging and would love to ask for feedback, so if anything didn't resonate, too long / too short, or on the podcast itself, too much info, to little info, please do share, I will take it into account 🙏 🫡 Also, we're coming up to the 52nd week I've been sending these, which will mark ThursdAI BirthdAI for real (the previous one was for the live shows) and I'm very humbled that so many of you are now reading, sharing and enjoying learning about AI together with me 🙏 See you next week, Alex This is a public episode. If you'd like to discuss this with other subscribers or get access to bonus episodes, visit sub.thursdai.news/subscribe | |||
20 Jun 2024 | 📅 ThursdAI - June 20th - 👑 Claude Sonnet 3.5 new LLM king, DeepSeek new OSS code king, Runway Gen-3 SORA competitor, Ilya's back & more AI news from this crazy week | 01:09:24 | |
Hey, this is Alex. Don't you just love when assumptions about LLMs hitting a wall just get shattered left and right and we get new incredible tools released that leapfrog previous state of the art models, that we barely got used to, from just a few months ago? I SURE DO! Today is one such day, this week was already busy enough, I had a whole 2 hour show packed with releases, and then Anthropic decided to give me a reason to use the #breakingNews button (the one that does the news show like sound on the live show, you should join next time!) and announced Claude Sonnet 3.5 which is their best model, beating Opus while being 2x faster and 5x cheaper! (also beating GPT-4o and Turbo, so... new king! For how long? ¯\_(ツ)_/¯) Critics are already raving, it's been half a day and they are raving! Ok, let's get to the TL;DR and then dive into Claude 3.5 and a few other incredible things that happened this week in AI! 👇 TL;DR of all topics covered: * Open Source LLMs * NVIDIA - Nemotron 340B - Base, Instruct and Reward model (X) * DeepSeek coder V2 (230B MoE, 16B) (X, HF) * Meta FAIR - Chameleon MMIO models (X) * HF + BigCodeProject are deprecating HumanEval with BigCodeBench (X, Bench) * NousResearch - Hermes 2 LLama3 Theta 70B - GPT-4 level OSS on MT-Bench (X, HF) * Big CO LLMs + APIs * Gemini Context Caching is available * Anthropic releases Sonnet 3.5 - beating GPT-4o (X, Claude.ai) * Ilya Sutskever starting SSI.inc - safe super intelligence (X) * Nvidia is the biggest company in the world by market cap * This weeks Buzz * Alex in SF next week for AIQCon, AI Engineer. ThursdAI will be sporadic but will happen! * W&B Weave now has support for tokens and cost + Anthropic SDK out of the box (Weave Docs) * Vision & Video * Microsoft open sources Florence 230M & 800M Vision Models (X, HF) * Runway Gen-3 - (t2v, i2v, v2v) Video Model (X) * Voice & Audio * Google Deepmind teases V2A video-to-audio model (Blog) * AI Art & Diffusion & 3D * Flash Diffusion for SD3 is out - Stable Diffusion 3 in 4 steps! (X) ThursdAI - Recaps of the most high signal AI weekly spaces is a reader-supported publication. To receive new posts and support my work, consider becoming a free or paid subscriber. 🦀 New king of LLMs in town - Claude 3.5 Sonnet 👑 Ok so first things first, Claude Sonnet, the previously forgotten middle child of the Claude 3 family, has now received a brain upgrade! Achieving incredible performance on many benchmarks, this new model is 5 times cheaper than Opus at $3/1Mtok on input and $15/1Mtok on output. It's also competitive against GPT-4o and turbo on the standard benchmarks, achieving incredible scores on MMLU, HumanEval etc', but we know that those are already behind us. Sonnet 3.5, aka Claw'd (which is a great marketing push by the Anthropic folks, I love to see it), is beating all other models on Aider.chat code editing leaderboard, winning on the new livebench.ai leaderboard and is getting top scores on MixEval Hard, which has 96% correlation with LMsys arena. While benchmarks are great and all, real folks are reporting real findings of their own, here's what Friend of the Pod Pietro Skirano had to say after playing with it: there's like a lot of things that I saw that I had never seen before in terms of like creativity and like how much of the model, you know, actually put some of his own understanding into your request -@Skirano What's notable a capability boost is this quote from the Anthropic release blog: In an internal agentic coding evaluation, Claude 3.5 Sonnet solved 64% of problems, outperforming Claude 3 Opus which solved 38%. One detail that Alex Albert from Anthropic pointed out from this released was, that on GPQA (Graduate-Level Google-Proof Q&A) Benchmark, they achieved a 67% with various prompting techniques, beating PHD experts in respective fields in this benchmarks that average 65% on this. This... this is crazy Beyond just the benchmarks This to me is a ridiculous jump because Opus was just so so good already, and Sonnet 3.5 is jumping over it with agentic solving capabilities, and also vision capabilities. Anthropic also announced that vision wise, Claw'd is significantly better than Opus at vision tasks (which, again, Opus was already great at!) and lastly, Claw'd now has a great recent cutoff time, it knows about events that happened in February 2024! Additionally, claude.ai got a new capability which significantly improves the use of Claude, which they call artifacts. It needs to be turned on in settings, and then Claude will have access to files, and will show you in an aside, rendered HTML, SVG files, Markdown docs, and a bunch more stuff, and it'll be able to reference different files it creates, to create assets and then a game with these assets for example! 1 Ilya x 2 Daniels to build Safe SuperIntelligence Ilya Sutskever, Co-founder and failed board Coup participant (leader?) at OpenAI, has resurfaced after a long time of people wondering "where's Ilya" with one hell of an announcement. The company is called SSI of Safe Super Intelligence, and he's cofounding it with Daniel Levy (prev OpenAI, PHD Stanford) and Daniel Gross (AI @ Apple, AIgrant, AI Investor). The only mandate of this company is apparently to have a straight shot at safe super-intelligence, skipping AGI, which is no longer the buzzword (Ilya is famous for the "feel the AGI" chant within OpenAI) Notable also that the company will be split between Palo Alto and Tel Aviv, where they have the ability to hire top talent into a "cracked team of researchers" Our singular focus means no distraction by management overhead or product cycles Good luck to these folks! Open Source LLMs DeepSeek coder V2 (230B MoE, 16B) (X, HF) The folks at DeepSeek are not shy about their results, and until the Sonnet release above, have released a 230B MoE model that beats GPT4-Turbo at Coding and Math! With a great new 128K context window and an incredible open license (you can use this in production!) this model is the best open source coder in town, getting to number 3 on aider code editing and number 2 on BigCodeBench (which is a new Benchmark we covered on the pod with the maintainer, definitely worth a listen. HumanEval is old and getting irrelevant) Notable also that DeepSeek has launched an API service that seems to be so competitively priced that it doesn't make sense to use anything else, with $0.14/$0.28 I/O per Million Tokens, it's a whopping 42 times cheaper than Claw'd 3.5! Support of 338 programming languages, it should also run super quick given it's MoE architecture, the bigger model is only 21B active parameters which scales amazing on CPUs. They also released a tiny 16B MoE model called Lite-instruct and it's 2.4B active params. This weeks Buzz (What I learned with WandB this week) Folks, in a week, I'm going to go up on stage in front of tons of AI Engineers wearing a costume, and... it's going to be epic! I finished writing my talk, now I'm practicing and I'm very excited. If you're there, please join the Evals track 🙂 Also in W&B this week, coinciding with Claw'd release, we've added a native integration with the Anthropic Python SDK which now means that all you need to do to track your LLM calls with Claw'd is pip install weave and import weave and weave.init('your project name' THAT'S IT! and you get this amazing dashboard with usage tracking for all your Claw'd calls for free, it's really crazy easy, give it a try! Vision & Video Runway Gen-3 - SORA like video model announced (X, blog) Runway, you know the company who everyone was "sorry for" when SORA was announced by OpenAI, is not sitting around waiting to "be killed" and is announcing Gen-3, an incredible video model capable of realistic video generations, physics understanding, and a lot lot more. The videos took over my timeline, and this looks to my eyes better than KLING and better than Luma Dream Machine from last week, by quite a lot! Not to mention that Runway has been in video production for way longer than most, so they have other tools that work with this model, like motion brush, lip syncing, temporal controls and many more, that allow you to be the director of the exactly the right scene. Google Deepmind video-to-audio (X) You're going to need to turn your sound on for this one! Google has released a tease of a new model of theirs that can be paired amazingly well with the above type generative video models (of which Google also has one, that they've teased and it's coming bla bla bla) This one, watches your video and provides acoustic sound fitting the scene, with on-sceen action sound! They showed a few examples and honestly they look so good, a drummer playing drums and that model generated the drums sounds etc' 👏 Will we ever see this as a product from google though? Nobody knows! Microsoft releases tiny (0.23B, 0.77B) Vision Models Florence (X, HF, Try It) This one is a very exciting release because it's MIT licensed, and TINY! Less than 1 Billion parameters, meaning it can completely run on device, it's a vision model, that beats MUCH bigger vision models by a significant amount on tasks like OCR, segmentation, object detection, image captioning and more! They have leveraged (and supposedly going to release) a FLD-5B dataset, and they have specifically made this model to be fine-tunable across these tasks, which is exciting because open source vision models are going to significantly benefit from this release almost immediately. Just look at this hand written OCR capability! Stellar! NousResearch - Hermes 2 Theta 70B - inching over GPT-4 on MT-Bench Teknium and the Nous Reseach crew have released a new model just to mess with me, you see, the live show was already recorded and edited, the file exported, the TL'DR written, and the newsletter draft almost ready to submit, and then I check the Green Room (DM group for all friends of the pod for ThursdAI, it's really an awesome Group Chat) and Teknium drops that they've beat GPT-4 (unsure which version) on MT-Bench with a finetune and a merge of LLama-3 They beat Llama-3 instruct which on its own is very hard, by merging in Llama-3 instruct into their model with Charles Goddards help (merge-kit author) As always, these models from Nous Research are very popular, but apparently a bug at HuggingFace shows that this one is extra super duper popular, clocking in at almost 25K downloads in the past hour since release, which doesn't quite make sense 😅 anyway, I'm sure this is a great one, congrats on the release friends! ThursdAI - Recaps of the most high signal AI weekly spaces is a reader-supported publication. To receive new posts and support my work, consider becoming a free or paid subscriber. Phew, somehow we covered all (most? all of the top interesting) AI news and breakthroughs of this week? Including interviews and breaking news! I think that this is our almost 1 year anniversary since we started putting ThursdAI on a podcast, episode #52 is coming shortly! Next week is going to be a big one as well, see you then, and if you enjoy these, give us a 5 start review on whatever podcast platform you're using? It really helps 🫡 This is a public episode. If you'd like to discuss this with other subscribers or get access to bonus episodes, visit sub.thursdai.news/subscribe | |||
27 Jun 2024 | 📅 ThursdAI - Gemma 2, AI Engineer 24', AI Wearables, New LLM leaderboard | 01:21:04 | |
Hey everyone, sending a quick one today, no deep dive, as I'm still in the middle of AI Engineer World's Fair 2024 in San Francisco (in fact, I'm writing this from the incredible floor 32 presidential suite, that the team here got for interviews, media and podcasting, and hey to all new folks who I’ve just met during the last two days!) It's been an incredible few days meeting so many ThursdAI community members, listeners and folks who came on the pod! The list honestly is too long but I've got to meet friends of the pod Maxime Labonne, Wing Lian, Joao Morra (crew AI), Vik from Moondream, Stefania Druga not to mention the countless folks who came up and gave high fives, introduced themselves, it was honestly a LOT of fun. (and it's still not over, if you're here, please come and say hi, and let's take a LLM judge selfie together!) On today's show, we recorded extra early because I had to run and play dress up, and boy am I relieved now that both the show and the talk are behind me, and I can go an enjoy the rest of the conference 🔥 (which I will bring you here in full once I get the recording!) On today's show, we had the awesome pleasure to have Surya Bhupatiraju who's a research engineer at Google DeepMind, talk to us about their newly released amazing Gemma 2 models! It was very technical, and a super great conversation to check out! Gemma 2 came out with 2 sizes, a 9B and a 27B parameter models, with 8K context (we addressed this on the show) and this 27B model incredible performance is beating LLama-3 70B on several benchmarks and is even beating Nemotron 340B from NVIDIA! This model is also now available on the Google AI studio to play with, but also on the hub! We also covered the renewal of the HuggingFace open LLM leaderboard with their new benchmarks in the mix and normalization of scores, and how Qwen 2 is again the best model that's tested! It's was a very insightful conversation, that's worth listening to if you're interested in benchmarks, definitely give it a listen. Last but not least, we had a conversation with Ethan Sutin, the co-founder of Bee Computer. At the AI Engineer speakers dinner, all the speakers received a wearable AI device as a gift, and I onboarded (cause Swyx asked me) and kinda forgot about it. On the way back to my hotel I walked with a friend and chatted about my life. When I got back to my hotel, the app prompted me with "hey, I now know 7 new facts about you" and it was incredible to see how much of the conversation it was able to pick up, and extract facts and eve TODO's! So I had to have Ethan on the show to try and dig a little bit into the privacy and the use-cases of these hardware AI devices, and it was a great chat! Sorry for the quick one today, if this is the first newsletter after you just met me and register, usually there’s a deeper dive here, expect a more in depth write-ups in the next sessions, as now I have to run down and enjoy the rest of the conference! Here's the TL;DR and my RAW show notes for the full show, in case it's helpful! * AI Engineer is happening right now in SF * Tracks include Multimodality, Open Models, RAG & LLM Frameworks, Agents, Al Leadership, Evals & LLM Ops, CodeGen & Dev Tools, Al in the Fortune 500, GPUs & Inference * Open Source LLMs * HuggingFace - LLM Leaderboard v2 - (Blog) * Old Benchmarks sucked and it's time to renew * New Benchmarks * MMLU-Pro (Massive Multitask Language Understanding - Pro version, paper) * GPQA (Google-Proof Q&A Benchmark, paper). GPQA is an extremely hard knowledge dataset * MuSR (Multistep Soft Reasoning, paper). * MATH (Mathematics Aptitude Test of Heuristics, Level 5 subset, paper) * IFEval (Instruction Following Evaluation, paper) * 🤝 BBH (Big Bench Hard, paper). BBH is a subset of 23 challenging tasks from the BigBench dataset * The community will be able to vote for models, and we will prioritize running models with the most votes first * Mozilla announces Builders Accelerator @ AI Engineer (X) * Theme: Local AI * 100K non dilutive funding * Google releases Gemma 2 (X, Blog) * Big CO LLMs + APIs * UMG, Sony, Warner sue Udio and Suno for copyright (X) * were able to recreate some songs * sue both companies * have 10 unnamed individuals who are also on the suit * Google Chrome Canary has Gemini nano (X) * * Super easy to use window.ai.createTextSession() * Nano 1 and 2, at a 4bit quantized 1.8B and 3.25B parameters has decent performance relative to Gemini Pro * Behind a feature flag * Most text gen under 500ms * Unclear re: hardware requirements * Someone already built extensions * someone already posted this on HuggingFace * Anthropic Claude share-able projects (X) * Snapshots of Claude conversations shared with your team * Can share custom instructions * Anthropic has released new "Projects" feature for Claude AI to enable collaboration and enhanced workflows * Projects allow users to ground Claude's outputs in their own internal knowledge and documents * Projects can be customized with instructions to tailor Claude's responses for specific tasks or perspectives * "Artifacts" feature allows users to see and interact with content generated by Claude alongside the conversation * Claude Team users can share their best conversations with Claude to inspire and uplevel the whole team * North Highland consultancy has seen 5x faster content creation and analysis using Claude * Anthropic is committed to user privacy and will not use shared data to train models without consent * Future plans include more integrations to bring in external knowledge sources for Claude * OpenAI voice mode update - not until Fall * AI Art & Diffusion & 3D * Fal open sourced AuraSR - a 600M upscaler based on GigaGAN (X, Fal) * Interview with Ethan Sutin from Bee Computer * We all got Bees as a gifts * AI Wearable that extracts TODOs, knows facts, etc' * This is a public episode. If you'd like to discuss this with other subscribers or get access to bonus episodes, visit sub.thursdai.news/subscribe | |||
04 Jul 2024 | 📆 🎂 - ThursdAI #52 - Moshi Voice, Qwen2 finetunes, GraphRag deep dive and more AI news on this celebratory 1yr ThursdAI | 01:50:25 | |
Hey everyone! Happy 4th of July to everyone who celebrates! I celebrated today by having an intimate conversation with 600 of my closest X friends 😂 Joking aside, today is a celebratory episode, 52nd consecutive weekly ThursdAI show! I've been doing this as a podcast for a year now! Which means, there are some of you, who've been subscribed for a year 😮 Thank you! Couldn't have done this without you. In the middle of my talk at AI Engineer (I still don't have the video!) I had to plug ThursdAI, and I asked the 300+ audience who is a listener of ThursdAI, and I saw a LOT of hands go up, which is honestly, still quite humbling. So again, thank you for tuning in, listening, subscribing, learning together with me and sharing with your friends! This week, we covered a new (soon to be) open source voice model from KyutAI, a LOT of open source LLM, from InternLM, Cognitive Computations (Eric Hartford joined us), Arcee AI (Lukas Atkins joined as well) and we have a deep dive into GraphRAG with Emil Eifrem CEO of Neo4j (who shares why it was called Neo4j in the first place, and that he's a ThursdAI listener, whaaat? 🤯), this is definitely a conversation you don't want to miss, so tune in, and read a breakdown below: TL;DR of all topics covered: * Voice & Audio * KyutAI releases Moshi - first ever 7B end to end voice capable model (Try it) * Open Source LLMs * Microsoft Updated Phi-3-mini - almost a new model * InternLM 2.5 - best open source model under 12B on Hugging Face (HF, Github) * Microsoft open sources GraphRAG (Announcement, Github, Paper) * OpenAutoCoder-Agentless - SOTA on SWE Bench - 27.33% (Code, Paper) * Arcee AI - Arcee Agent 7B - from Qwen2 - Function / Tool use finetune (HF) * LMsys announces RouteLLM - a new Open Source LLM Router (Github) * DeepSeek Chat got an significant upgrade (Announcement) * Nomic GPT4all 3.0 - Local LLM (Download, Github) * This weeks Buzz * New free Prompts course from WandB in 4 days (pre sign up) * Big CO LLMs + APIs * Perplexity announces their new pro research mode (Announcement) * X is rolling out "Grok Analysis" button and it's BAD in "fun mode" and then paused roll out * Figma pauses the rollout of their AI text to design tool "Make Design" (X) * Vision & Video * Cognitive Computations drops DolphinVision-72b - VLM (HF) * Chat with Emil Eifrem - CEO Neo4J about GraphRAG, AI Engineer Voice & Audio KyutAI Moshi - a 7B end to end voice model (Try It, See Announcement) Seemingly out of nowhere, another french AI juggernaut decided to drop a major announcement, a company called KyutAI, backed by Eric Schmidt, call themselves "the first European private-initiative laboratory dedicated to open research in artificial intelligence" in a press release back in November of 2023, have quite a few rockstar co founders ex Deep Mind, Meta AI, and have Yann LeCun on their science committee. This week they showed their first, and honestly quite mind-blowing release, called Moshi (Japanese for Hello, Moshi Moshi), which is an end to end voice and text model, similar to GPT-4o demos we've seen, except this one is 7B parameters, and can run on your mac! While the utility of the model right now is not the greatest, not remotely close to anything resembling the amazing GPT-4o (which was demoed live to me and all of AI Engineer by Romain Huet) but Moshi shows very very impressive stats! Built by a small team during only 6 months or so of work, they have trained an LLM (Helium 7B) an Audio Codec (Mimi) a Rust inference stack and a lot more, to give insane performance. Model latency is 160ms and mic-to-speakers latency is 200ms, which is so fast it seems like it's too fast. The demo often responds faster than I'm able to finish my sentence, and it results in an uncanny, "reading my thoughts" type feeling. The most important part is this though, a quote of KyutAI post after the announcement : Developing Moshi required significant contributions to audio codecs, multimodal LLMs, multimodal instruction-tuning and much more. We believe the main impact of the project will be sharing all Moshi’s secrets with the upcoming paper and open-source of the model. I'm really looking forward to how this tech can be applied to the incredible open source models we already have out there! Speaking to out LLMs is now officially here in the Open Source, way before we got GPT-4o and it's exciting! Open Source LLMs Microsoft stealth update Phi-3 Mini to make it almost a new model So stealth in fact, that I didn't even have this update in my notes for the show, but thanks to incredible community (Bartowsky, Akshay Gautam) who made sure we don't miss this, because it's so huge. The model used additional post-training data leading to substantial gains on instruction following and structure output. We also improve multi-turn conversation quality, explicitly support <|system|> tag, and significantly improve reasoning capability Phi-3 June update is quite significant across the board, just look at some of these scores, 354.78% improvement in JSON structure output, 30% at GPQA But also specifically for coding, a 33→93 jump in Java coding, 33→73 in Typescript, 27→ 85 in Python! These are just incredible numbers, and I definitely agree with Bartowski here, there's enough here to call this a whole new model rather than an "seasonal update" Qwen-2 is the start of the show right now Week in and week out, ThursdAI seems to be the watercooler for the best finetuners in the community to come, hang, share notes, and announce their models. A month after Qwen-2 was announced on ThursdAI stage live by friend of the pod and Qwen dev lead Junyang Lin, and a week after it re-took number 1 on the revamped open LLM leaderboard on HuggingFace, we now have great finetunes on top of Qwen-2. Qwen-2 is the star of the show right now. Because there's no better model. This is like GPT 4 level. It's Open Weights GPT 4. We can do what we want with it, and it's so powerful, and it's multilingual, and it's everything, it's like the dream model. I love it Eric Hartford - Cognitive Computations We've had 2 models finetunes based on Qwen 2 and their authors on the show this week, first was Lukas Atkins from Arcee AI (company behind MergeKit), they released Arcee Agent, a 7B Qwen-2 finetune/merge specifically focusing on tool use and function calling. We also had a chat with Eric Hartford from Cognitive Computations (which Lukas previously participated in) with the biggest open source VLM on top of Qwen-2, a 72B parameter Dolphin Vision (Trained by StableQuan, available on the HUB) ,and it's likely the biggest open source VLM that we've seen so far. The most exciting part about it, is Fernando Neta's "SexDrugsRockandroll" dataset, which supposedly contains, well.. a lot of uncensored stuff, and it's perfectly able to discuss and analyze images with mature and controversial content. InternLM 2.5 - SOTA open source under 12B with 1M context (HF, Github) The folks at Shanghai AI release InternLM 2.5 7B, and a chat version along with a whopping 1M context window extension. These metrics are ridiculous, beating LLama-3 8B on literally every metric on the new HF leaderboard, and even beating Llama-3 70B on MATH and coming close on GPQA! The folks at Intern not only released a beast of a model, but also have released a significantly imporved tool use capabilities with it, including their own agentic framework called Lagent, which comes with Code Interpreter (python execution), Search Capabilities, and of course the abilities to plug in your own tools. How will you serve 1M context on production you ask? Well, these folks ALSO open sourced LMDeploy, "an efficient, user-friendly toolkit designed for compressing, deploying, and serving LLM models" which has been around for a while, but is now supporting this new model of course, handles dynamic NTK and some offloading of context etc' So an incredible model + tools release, can't wait to play around with this! ThursdAI - Recaps of the most high signal AI weekly spaces is a reader-supported publication. To receive new posts and support my work, consider becoming a free or paid subscriber. This weeks Buzz (What I learned with WandB this week) Hey, did you know we at Weights & Biases have free courses? While some folks ask you for a LOT of money for basic courses, at Weights & Biases, they are... you guessed it, completely free! And a lot of effort goes into recording and building the agenda, so I'm happy to announce that our "Developer's Guide to LLM Prompting" course is going to launch in 4 days! Delivered by my colleague Anish (who's just an amazing educator) and Teodora from AutogenAI, you will learn everything prompt building related, and even if you are a seasoned prompting pro, there will be something for you there! Pre-register for the course HERE Big CO LLMs + APIs How I helped roll back an XAI feature and Figma rolled back theirs We've covered Grok (with a K this time) from XAI multiple times, and while I don't use it's chat interface that much, or the open source model, I do think they have a huge benefit in having direct access to real time data from the X platform. Given that I basically live on X (to be able to deliver all these news to you) I started noticing a long promised, Grok Analysis button show up under some posts, first on mobile, then on web versions of X. Of course I had to test it, and whoa, I was honestly shocked at just how unhinged and profanity laced the analysis was. Now I'm not easily shocked, I've seen jailbroken LLMs before, I tried to get chatGPT to say curse words multiple times, but it's one thing when you expect it and a complete another thing when a billion dollar company releases a product that answers... well like this: Luckily Igor Babushkin (Co founder of XAI) noticed and the roll out was paused, so looks like I helped red team grok! 🫡 Figma pauses AI "make design" feature Another AI feature was paused by a big company after going viral on X (what is it about X specifically?) and this time it was Figma! In a super viral post, Andy Allen posted a video where he asks the new AI feature from Figma called "Make Design" a simple "weather app" and what he receives looks almost 100% identical to the iOS weather app! This was acknowledged by the CEO of Figma and almost immediately paused as well. GraphRAG... GraphRAG everywhere Microsoft released a pre-print paper called GraphRag (2404.16130) which talks about utilizing LLMs to first build and the use Graph databases to achieve better accuracy and performance for retrieval tasks such as "global questions directed at an entire text corpus" This week, Microsoft open sourced GraphRag on Github 👏 and I wanted to dive a little deeper into what this actually means, as this is a concept I haven't head of before last week, and suddenly it's everywhere. Last week during AI Engineer, the person who first explained this concept to me (and tons of other folks in the crowd at his talk) was Emil Eifrem, CEO of Neo4J and I figured he'd be the right person to explain the whole concept in a live conversation to the audience as well, and he was! Emil and I (and other folks in the audience) had a great, almost 40 minute conversation about the benefits of using Graph databases for RAG, how LLMs unlocked the ability to convert unstructured data into Graph linked databases, accuracy enhancements and unlocks like reasoning over the whole corpus of data, developer experience improvements, and difficulties / challenges with this approach. Emil is a great communicator, with a deep understanding of this field, so I really recommend to listen to this deep dive. Thank you for reading ThursdAI - Recaps of the most high signal AI weekly spaces. This post is public so feel free to share it. This is it for this weeks newsletter, and a wrap on year 1 of ThursdAI as a podcast (this being out 52nd weekly release!) I'm going on vacation next week, but I will likely still send the TL;DR, so look out for that, and have a great independence day, and rest of your holiday weekend if you celebrate, and if you're not, I'm sure there will be cool AI things announced by the next time we meet 🫡 As always, appreciate your attention, Alex This is a public episode. If you'd like to discuss this with other subscribers or get access to bonus episodes, visit sub.thursdai.news/subscribe | |||
11 Jul 2024 | 📅 ThursdAI - July 11 - Mixture of Agents & Open Router interviews (no news this week) | 01:09:02 | |
Hey all, Alex here… well, not actually here, I’m scheduling this post in advance, which I haven’t yet done, because I'm going on vacation! That’s right, next week is my birthday 🎉 and a much needed break, somewhere with a beach is awaiting, but I didn’t want to leave you hanging for too long, so posting this episode with some amazing un-released before material. Mixture of Agents x2 Back in the far away days of June 20th (not that long ago but feels like ages!), Together AI announced a new paper, released code and posted a long post about a new method to collaboration between smaller models to beat larger models. They called it Mixture of Agents, and James Zou joined us to chat about that effort. Shortly after that - in fact, during the live ThursdAI show, Kyle Corbitt announced that OpenPipe also researched an approached similar to the above, using different models and a bit of a different reasoning, and also went after the coveted AlpacaEval benchmark, and achieved SOTA score of 68.8 using this method. And I was delighted to invite both James and Kyle to chat about their respective approach the same week that both broke AlpacaEval SOTA and hear how utilizing collaboration between LLMs can significantly improve their outputs! This weeks buzz - what I learned at W&B this week So much buzz this week from the Weave team, it’s hard to know what to put in here. I can start with the incredible integrations my team landed, Mistral AI, LLamaIndex, DSPy, OpenRouter and even Local Models served by Ollama, LmStudio, LLamaFile can be now auto tracked with Weave, which means you literally have to only instantiate Weave and it’ll auto track everything for you! But I think the biggest, hugest news from this week is this great eval comparison system that the Weave Tim just pushed, it’s honestly so feature rich that I’ll have to do a deeper dive on it later, but I wanted to make sure I include at least a few screencaps because I think it looks fantastic! Open Router - A unified interface for LLMs I’ve been a long time fan of OpenRouter.ai and I was very happy to have Alex Atallah on the show to talk about Open Router (even if this did happen back in April!) and I’m finally satisfied with the sound quality to released this conversation. Open Router is serving both foundational models like GPT, Claude, Gemini and also Open Source ones, and supports the OpenAI SDK format, making it super simple to play around and evaluate all of them on the same code. They even provide a few models for free! Right now you can use Phi for example completely free via their API. Alex goes deep into the areas of Open Router that I honestly didn’t really know about, like being a marketplace, knowing what trendy LLMs are being used by people in near real time (check out WebSim!) and more very interesting things! Give that conversation a listen, I’m sure you’ll enjoy it! That’s it folks, no news this week, I would instead like to recommend a new newsletter by friends of the pod Tanishq Abraham and Aran Komatsuzaki both of whom are doing a weekly paper X space and recently start posting it on Substack as well! It’s called AI papers of the week, and if you’re into papers which we don’t usually cover, there’s no better duo! In fact, Tanishq often used to come to ThursdAI to explain papers so you may recognize his voice :) See you all in two weeks after I get some seriously needed R&R 👋 😎🏖️ This is a public episode. If you'd like to discuss this with other subscribers or get access to bonus episodes, visit sub.thursdai.news/subscribe | |||
25 Jul 2024 | 🧨 ThursdAI - July 25 - OpenSource GPT4 intelligence has arrived - Meta LLaMa 3.1 405B beats GPT4o! Mistral Large 2 also, Deepseek Code v2 ALSO - THIS WEEK | 01:38:10 | |
Holy s**t, folks! I was off for two weeks, last week OpenAI released GPT-4o-mini and everyone was in my mentions saying, Alex, how are you missing this?? and I'm so glad I missed that last week and not this one, because while GPT-4o-mini is incredible (GPT-4o level distill with incredible speed and almost 99% cost reduction from 2 years ago?) it's not open source. So welcome back to ThursdAI, and buckle up because we're diving into what might just be the craziest week in open-source AI since... well, ever! This week, we saw Meta drop LLAMA 3.1 405B like it's hot (including updated 70B and 8B), Mistral joining the party with their Large V2, and DeepSeek quietly updating their coder V2 to blow our minds. Oh, and did I mention Google DeepMind casually solving math Olympiad problems at silver level medal 🥈? Yeah, it's been that kind of week. TL;DR of all topics covered: * Open Source * Meta LLama 3.1 updated models (405B, 70B, 8B) - Happy LLama Day! (X, Announcement, Zuck, Try It, Try it Faster, Evals, Provider evals) * Mistral Large V2 123B (X, HF, Blog, Try It) * DeepSeek-Coder-V2-0724 update (API only) * Big CO LLMs + APIs * 🥈 Google Deepmind wins silver medal at Math Olympiad - AlphaGeometry 2 (X) * OpenAI teases SearchGPT - their reimagined search experience (Blog) * OpenAI opens GPT-4o-mini finetunes + 2 month free (X) * This weeks Buzz * I compare 5 LLama API providers for speed and quantization using Weave (X) * Voice & Audio * Daily announces a new open standard for real time Voice and Video RTVI-AI (X, Try it, Github) Meta LLAMA 3.1: The 405B Open Weights Frontier Model Beating GPT-4 👑 Let's start with the star of the show: Meta's LLAMA 3.1. This isn't just a 0.1 update; it's a whole new beast. We're talking about a 405 billion parameter model that's not just knocking on GPT-4's door – it's kicking it down. Here's the kicker: you can actually download this internet scale intelligence (if you have 820GB free). That's right, a state-of-the-art model beating GPT-4 on multiple benchmarks, and you can click a download button. As I said during the show, "This is not only refreshing, it's quite incredible." Some highlights: * 128K context window (finally!) * MMLU score of 88.6 * Beats GPT-4 on several benchmarks like IFEval (88.6%), GSM8K (96.8%), and ARC Challenge (96.9%) * Has Tool Use capabilities (also beating GPT-4) and is Multilingual (ALSO BEATING GPT-4) But that's just scratching the surface. Let's dive deeper into what makes LLAMA 3.1 so special. The Power of Open Weights Mark Zuckerberg himself dropped an exclusive interview with our friend Rowan Cheng from Rundown AI. And let me tell you, Zuck's commitment to open-source AI is no joke. He talked about distillation, technical details, and even released a manifesto on why open AI (the concept, not the company) is "the way forward". As I mentioned during the show, "The fact that this dude, like my age, I think he's younger than me... knows what they released to this level of technical detail, while running a multi billion dollar company is just incredible to me." Evaluation Extravaganza The evaluation results for LLAMA 3.1 are mind-blowing. We're not just talking about standard benchmarks here. The model is crushing it on multiple fronts: * MMLU (Massive Multitask Language Understanding): 88.6% * IFEval (Instruction Following): 88.6% * GSM8K (Grade School Math): 96.8% * ARC Challenge: 96.9% But it doesn't stop there. The fine folks at meta also for the first time added new categories like Tool Use (BFCL 88.5) and Multilinguality (Multilingual MGSM 91.6) (not to be confused with MultiModality which is not yet here, but soon) Now, these are official evaluations from Meta themselves, that we know, often don't really represent the quality of the model, so let's take a look at other, more vibey results shall we? On SEAL leaderboards from Scale (held back so can't be trained on) LLama 405B is beating ALL other models on Instruction Following, getting 4th at Coding and 2nd at Math tasks. On MixEval (the eval that approximates LMsys with 96% accuracy), my colleagues Ayush and Morgan got a whopping 66%, placing 405B just after Clause Sonnet 3.5 and above GPT-4o And there are more evals that all tell the same story, we have a winner here folks (see the rest of the evals in my thread roundup) The License Game-Changer Meta didn't just release a powerful model; they also updated their license to allow for synthetic data creation and distillation. This is huge for the open-source community. LDJ highlighted its importance: "I think this is actually pretty important because even though, like you said, a lot of people still train on OpenAI outputs anyways, there's a lot of legal departments and a lot of small, medium, and large companies that they restrict the people building and fine-tuning AI models within that company from actually being able to build the best models that they can because of these restrictions." This update could lead to a boom in custom models and applications across various industries as companies can start distilling, finetuning and creating synthetic datasets using these incredibly smart models. 405B: A Double-Edged Sword While the 405B model is incredibly powerful, it's not exactly practical for most production use cases as you need 2 nodes of 8 H100s to run it in full precision. Despite the fact that pricing wars already started, and we see inference providers at as low as 2.7$/1M tokens, this hardly makes sense when GPT-4o mini is 15 cents. However, this model shines in other areas: * Synthetic Data Generation & Distillation: Its power and the new license make it perfect for creating high-quality training data and use it to train smaller models * LLM as a Judge: The model's reasoning capabilities make it an excellent candidate for evaluating other AI outputs. * Research and Experimentation: For pushing the boundaries of what's possible in AI. The Smaller Siblings: 70B and 8B While the 405B model is grabbing headlines, don't sleep on its smaller siblings. The 70B and 8B models got significant upgrades too. The 70B model saw impressive gains: * MMLU: 80.9 to 86 * IFEval: 82 to 87 * GPQA: 39 to 46 The 8B model, in particular, could be a hidden gem. As Kyle Corbitt from OpenPipe discovered, a fine-tuned 8B model could potentially beat a prompted GPT-4 Mini in specific tasks. No multi-modality While Meta definitely addressed everything we had to ask for from the Llama 3 release, context window, incredible performance, multi-linguality, tool-use, we still haven't seen multi-modality with Llama. We still can't show it pictures or talk to it! However, apparently they have trained it to be mutli-modal as well but haven't yet released those weights, but they went into this in great detail in the paper and even showed a roadmap, stating that they will release it soon-ish (not in EU though) This Week's Buzz: Weave-ing Through LLama Providers In the spirit of thorough evaluation, I couldn't resist putting LLAMA 3.1 through its paces across different providers. Using Weights & Biases Weave (https://wandb.me/weave), our evaluation and tracing framework for LLMs, I ran a comparison between various LLAMA providers. Here's what I found: * Different providers are running the model with varying optimizations (VLLM, FlashAttention3, etc.) * Some are serving quantized versions, which can affect output style and quality * Latency and throughput vary significantly between providers The full results are available in a Weave comparison dashboard, which you can check out for a deep dive into the nuances of model deployment and code is up on Github if you want to verify this yourself or see how easy this is to do with Weave Mistral Crashes the Party with Large V2 123B model (X, HF, Blog, Try It) Just when we thought Meta had stolen the show, Mistral AI decided to drop their own bombshell: Mistral Large V2. This 123 billion parameter dense model is no joke, folks. With an MMLU score of 84.0, 128K context window and impressive performance across multiple benchmarks, it's giving LLAMA 3.1 a run for its money, especially in some coding tasks while being optimized to run on a single node! Especially interesting is the function calling on which they claim SOTA, without telling us which metric they used (or comparing to Llama 3.1) but are saying that they now support parallel and sequential function calling! DeepSeek updates DeepSeek Coder V2 to 0724 While everyone was busy gawking at Meta and Mistral, DeepSeek quietly updated their coder model, and holy smokes, did they deliver! DeepSeek Coder v2 is now performing at GPT-4 and Claude 3.5 Sonnet levels on coding tasks. As Junyang Lin noted during our discussion, "DeepSeek Coder and DeepSeek Coder v2 should be the state of the art of the code-specific model." Here's the result from BigCodeBench and from Aider Chat (code editing dashboard) But it's not just about raw performance. DeepSeek is bringing some serious innovation to the table. They've added JSON mode, function calling, and even a fill-in-the-middle completion feature in beta. Plus, they've bumped up their max token generation to 8K. And let's talk about that API pricing – it's ridiculously cheap, at 14c / 1M tokens!. We're talking about costs that are competitive with GPT-4 Mini, but with potentially better performance on coding tasks. It's a game-changer for developers and companies looking to integrate powerful coding AI without breaking the bank. Google DeepMind's Math Wizardry: From Silver Medals to AI Prodigies Just when we thought this week couldn't get any crazier, Google DeepMind decides to casually drop a bombshell that would make even the most decorated mathletes sweat. They've created an AI system that can solve International Mathematical Olympiad (IMO) problems at a silver medalist level. I mean, come on! As if the AI world wasn't moving fast enough, now we've got silicon-based Math Olympians? This isn't just any run-of-the-mill calculator on steroids. We're talking about a combination of AlphaProof, a new breakthrough model for formal reasoning, and AlphaGeometry 2, an upgraded version of their previous system. These AI math whizzes tackled this year's six IMO problems, covering everything from algebra to number theory, and managed to solve four of them. That's 28 points, folks - enough to bag a silver medal if it were human! But here's where it gets really interesting. For non-geometry problems, AlphaProof uses the Lean theorem prover, coupling a pre-trained language model with the same AlphaZero reinforcement learning algorithm that taught itself to crush humans at chess and Go. And for geometry? They've got AlphaGeometry 2, a neuro-symbolic hybrid system powered by a Gemini-based language model. It's like they've created a math genius that can not only solve problems but also explain its reasoning in a formal, verifiable way. The implications here are huge, folks. We're not just talking about an AI that can do your homework; we're looking at a system that could potentially advance mathematical research and proof verification in ways we've never seen before. OpenAI takes on Google, Perplexity (and Meta's ownership of this week) with SearchGPT waitlist (Blog) As I write these words, Sam posts a tweet, saying that they are launching SearchGPT, their new take on search, and as I click, I see a waitlist 😅 But still, this looks so sick, just look: RTVI - new open standard for real time Voice and Video RTVI-AI (X, Github, Try it) Ok this is also great and can't be skipped, even tho this week was already insane. These models are great to text with but we want to talk to them, and while we all wait for GPT-4 Omni with voice to actually ship, we get a new contender that gives us an open standard and a killer demo! Daily + Groq + Cartesia + a lot of other great companies have releases this incredible demo (which you can try yourself here) and an open source standard to deliver something like a GPT-4o experience with incredible end to end latency, which feels like almost immediate responses. While we've chatted with Moshi previously which has these capabilities in the same model, the above uses LLama 3.1 70B even, which is an actual production grade LLM, which is a significant different from what Moshi offers. 🔥 Ok holy s**t, did I actually finish the writeup for this insane week? This was indeed one of the craziest weeks in Open Source AI, I honestly did NOT expect this to happen but I'm so excited to keep playing with all these tools, but also to see how the amazing open source community of finetuners will meet all these LLamas. Which I'm sure I'll be reporting on from now on until the next huge big AI breakthrough! Till then, see you next week, if you're listening to the podcast, please give us 5 stars on Apple podcast / Spotify? It really does help, and I'll finish with this: IT'S SO GOOD TO BE BACK! 😂🫡 This is a public episode. If you'd like to discuss this with other subscribers or get access to bonus episodes, visit sub.thursdai.news/subscribe | |||
01 Aug 2024 | 📆 ThursdAI - August 1st - Meta SAM 2 for video, Gemini 1.5 is king now?, GPT-4o Voice is here (for some), new Stability, Apple Intelligence also here & more AI news | 01:52:36 | |
Starting Monday, Apple released iOS 18.1 with Apple Intelligence, then Meta dropped SAM-2 (Segment Anything Model) and then Google first open sourced Gemma 2B and now (just literally 2 hours ago, during the live show) released Gemini 1.5 0801 experimental that takes #1 on LMsys arena across multiple categories, to top it all off we also got a new SOTA image diffusion model called FLUX.1 from ex-stability folks and their new Black Forest Lab. This week on the show, we had Joseph & Piotr Skalski from Roboflow, talk in depth about Segment Anything, and as the absolute experts on this topic (Skalski is our returning vision expert), it was an incredible deep dive into the importance dedicated vision models (not VLMs). We also had Lukas Atkins & Fernando Neto from Arcee AI talk to use about their new DistillKit and explain model Distillation in detail & finally we had Cristiano Giardina who is one of the lucky few that got access to OpenAI advanced voice mode + his new friend GPT-4o came on the show as well! Honestly, how can one keep up with all this? by reading ThursdAI of course, that's how but ⚠️ buckle up, this is going to be a BIG one (I think over 4.5K words, will mark this as the longest newsletter I penned, I'm sorry, maybe read this one on 2x? 😂) [ Chapters ] 00:00 Introduction to the Hosts and Their Work 01:22 Special Guests Introduction: Piotr Skalski and Joseph Nelson 04:12 Segment Anything 2: Overview and Capabilities 15:33 Deep Dive: Applications and Technical Details of SAM2 19:47 Combining SAM2 with Other Models 36:16 Open Source AI: Importance and Future Directions 39:59 Introduction to Distillation and DistillKit 41:19 Introduction to DistilKit and Synthetic Data 41:41 Distillation Techniques and Benefits 44:10 Introducing Fernando and Distillation Basics 44:49 Deep Dive into Distillation Process 50:37 Open Source Contributions and Community Involvement 52:04 ThursdAI Show Introduction and This Week's Buzz 53:12 Weights & Biases New Course and San Francisco Meetup 55:17 OpenAI's Advanced Voice Mode and Cristiano's Experience 01:08:04 SearchGPT Release and Comparison with Perplexity 01:11:37 Apple Intelligence Release and On-Device AI Capabilities 01:22:30 Apple Intelligence and Local AI 01:22:44 Breaking News: Black Forest Labs Emerges 01:24:00 Exploring the New Flux Models 01:25:54 Open Source Diffusion Models 01:30:50 LLM Course and Free Resources 01:32:26 FastHTML and Python Development 01:33:26 Friend.com: Always-On Listening Device 01:41:16 Google Gemini 1.5 Pro Takes the Lead 01:48:45 GitHub Models: A New Era 01:50:01 Concluding Thoughts and Farewell Show Notes & Links * Open Source LLMs * Meta gives SAM-2 - segment anything with one shot + video capability! (X, Blog, DEMO) * Google open sources Gemma 2 2.6B (Blog, HF) * MTEB Arena launching on HF - Embeddings head to head (HF) * Arcee AI announces DistillKit - (X, Blog, Github) * AI Art & Diffusion & 3D * Black Forest Labs - FLUX new SOTA diffusion models (X, Blog, Try It) * Midjourney 6.1 update - greater realism + potential Grok integration (X) * Big CO LLMs + APIs * Google updates Gemini 1.5 Pro with 0801 release and is #1 on LMsys arena (X) * OpenAI started alpha GPT-4o voice mode (examples) * OpenAI releases SearchGPT (Blog, Comparison w/ PPXL) * Apple releases beta of iOS 18.1 with Apple Intelligence (X, hands on, Intents ) * Apple released a technical paper of apple intelligence * This weeks Buzz * AI Salons in SF + New Weave course for WandB featuring yours truly! * Vision & Video * Runway ML adds Gen -3 image to video and makes it 7x faster (X) * Tools & Hardware * Avi announces friend.com * Jeremy Howard releases FastHTML (Site, Video) * Applied LLM course from Hamel dropped all videos Open Source It feels like everyone and their grandma is open sourcing incredible AI this week! Seriously, get ready for segment-anything-you-want + real-time-video capability PLUS small AND powerful language models. Meta Gives Us SAM-2: Segment ANYTHING Model in Images & Videos... With One Click! Hold on to your hats, folks! Remember Segment Anything, Meta's already-awesome image segmentation model? They've just ONE-UPPED themselves. Say hello to SAM-2 - it's real-time, promptable (you can TELL it what to segment), and handles VIDEOS like a champ. As I said on the show: "I was completely blown away by segment anything 2". But wait, what IS segmentation? Basically, pixel-perfect detection - outlining objects with incredible accuracy. My guests, the awesome Piotr Skalski and Joseph Nelson (computer vision pros from Roboflow), broke it down historically, from SAM 1 to SAM 2, and highlighted just how mind-blowing this upgrade is. "So now, Segment Anything 2 comes out. Of course, it has all the previous capabilities of Segment Anything ... But the segment anything tool is awesome because it also can segment objects on the video". - Piotr Skalski Think about Terminator vision from the "give me your clothes" bar scene: you see a scene, instantly "understand" every object separately, AND track it as it moves. SAM-2 gives us that, allowing you to click on a single frame, and BAM - perfect outlines that flow through the entire video! I played with their playground, and you NEED to try it - you can blur backgrounds, highlight specific objects... the possibilities are insane. Playground Link In this video, Piotr annotated only the first few frames of the top video, and SAM understood the bottom two shot from 2 different angles! Okay, cool tech, BUT why is it actually USEFUL? Well, Joseph gave us incredible examples - from easier sports analysis and visual effects (goodbye manual rotoscoping) to advances in microscopic research and even galactic exploration! Basically, any task requiring precise object identification gets boosted to a whole new level. "SAM does an incredible job at creating pixel perfect outlines of everything inside visual scenes. And with SAM2, it does it across videos super well, too ... That capability is still being developed for a lot of AI Models and capabilities. So having very rich ability to understand what a thing is, where that thing is, how big that thing is, allows models to understand spaces and reason about them" - Joseph Nelson AND if you combine this power with other models (like Piotr is already doing!), you get zero-shot segmentation - literally type what you want to find, and the model will pinpoint it in your image/video. It's early days, but get ready for robotics applications, real-time video analysis, and who knows what else these clever hackers are dreaming up! 🤯 Check out Piotr's Zero Shot Florence + Sam2 Implementation Best of all? Apache 2 license, baby! As Joseph said, "Open source is foundational to making the accessibility, the use cases, and the advancement of the field overall", and this is a prime example. Huge kudos to Meta for empowering us with this tech. The whole conversation w/ Piotr & Joseph is very much worth listening to on the pod 🎙️ Google Throws Down The Gauntlet: Open Sourcing GemMA 2 2.6B It was Meta vs. Google on Monday because NOT to be outdone, Google also went on an open-sourcing spree. This time, they gifted us GemMA 2 (a 2.6 billion parameter powerhouse), alongside a safety-focused suite called ShieldGemMA AND a transparency tool called GemmaScope. So what makes Gemma 2 special? First off, it's optimized for on-device use, meaning super-efficient local running. BUT there's a catch, folks... They claim it beats Mixtral AND Llama 2 70B on the LMsys Arena leaderboard, with an ELO score of 1126. Hold on, a 2 billion parameter model outperforming the big boys? 🤨 As LDJ (one of my regular co-hosts) said on the show: "Yeah, I think my best theory here is... there's at least two or three variables at play ... In LMSys, people are much more likely to do single turn, and within LMSys, people will usually be biased more towards rating models with a more recent knowledge cutoff as higher". Translation? It might be gaming the system a bit, but either way, Gemma 2 is an exciting release - super fast, small enough for on-device applications, and coming with safety tools right out the gate! I think Zenova (our Hugging Face wizard) is already running this on WebGPU! You NEED to try it out. And GemmaScope? That's some cool, cool stuff too. Think about peeking inside the "brain" of the model - you can actually SEE how Gemma 2 processes information. Remember Anthropic Mechinterp? It's like that, giving us unprecedented transparency into how these systems actually "think". You gotta see it on Neuronpedia. Neuronpedia link It's Meta versus Google - round one, FIGHT! 🥊 Distilling Knowlege: Arcee AI Drops DistilKit! Just when I thought the week was done throwing surprises, Arcee AI casually dropped DistilKit - an open source tool to build distilled language models. Now, this is some NEXT level stuff, folks. We talked with Lukas Atkins and Fernando (the brilliant minds behind DistillKit), and I finally learned what the heck "distillation" really means. "TLDR - we teach a smaller model to think like a bigger model" In a nutshell: teach a smaller model how to think like a larger one. Think GPT-4o and GPT-4 Mini, where the smaller model supposedly got the "essence" of the bigger version. Or imagine a tiny Llama that inherited the smarts of 405B - ridiculous! 🤯 As Fernando eloquently put it: So in the finetuning that we have been doing, just in terms of generating text instructions and so on, we were observing only the token that was generated from the teacher model. And now with the distillation, we are observing the whole distribution of the tokens that could be sampled Now I admit, even after Fernando's expert breakdown, my brain still kind of melted. 🫠 BUT, here's why this matters: distilled models are super efficient, saving on cost and resources. Imagine powerful AI that runs seamlessly on your phone! 🤯 Arcee is making this possible for everyone. Was it pure coincidence they released this on the same week as the Llama 3.1 LICENSE CHANGE (Zuckerberg is clearly watching ThursdAI...), which makes distillation perfectly legal? It's wild, exciting, AND I predict a massive surge in smaller, specialized AI tools that inherit the intelligence of the big boys. This weeks buzz Did I already tell you that someone came up to me and said, hey, you're from Weights & Biases, you are the guys who make the courses right? 😂 I said, well yeah, we have a bunch of free courses on wandb.courses but we also have a world leading ML experiment tracking software and an LLM observability toolkit among other things. It was really funny he thought we're just courses company! Well this last week, my incredible colleague Agata who's in charge of our courses, took an initiative and stitched together a course about Weave from a bunch of videos that I already had recorded! It's awesome, please check it out if you're interested to learn about Weave 👏 P.S - we are also starting a series of AI events in our SF office called AI Salons, the first one is going to feature Shreya Shankar, and focus on evaluations, it's on August 15th, so if you're in SF, you're invited for free as a ThursdAI subscriber! Get free tickets Big Co AI - LLMs & APIs Not only was open source popping off, but those walled-garden mega corps wanted in on the action too! SearchGPT, anyone? From Whispers to Reality: OpenAI Alpha Tests GPT-4 Voice (and IT'S WILD) This was THE moment I waited for, folks - GPT-4 with ADVANCED VOICE is finally trickling out to alpha users. Did I get access? NO. 😩 But my new friend, Cristiano Giardina, DID and you've probably seen his viral videos of this tech - they're blowing up MY feed, even Sam Altman retweeted the above one! I said on the show, this new voice "feels like a big next unlock for AI" What sets this apart from the "regular" GPT-4 voice we have now? As Cristiano told us: "the biggest difference is that the emotion , and the speech is very real and it follows instructions regarding emotion very well, like you can ask it to speak in a more animated way, you can ask it to be angry, sad, and it really does a good job of doing that." We did a LIVE DEMO (it worked, thank God), and y'all... I got CHILLS. We heard counting with a breath, depressed Soviet narrators, even a "GET TO THE CHOPPA" Schwarzenegger moment that still makes me laugh 😂 It feels like a completely different level of interaction, something genuinely conversational and even emotional. Check out Cristiano's profile for more insane demos - you won't be disappointed.Follow Cristiano Here For Amazing Voice Mode Videos Can't wait for access, if anyone from OpenAI is reading this, hook me up 🙏 I'll trade my SearchGPT access! SearchGPT: OpenAI Throws Their Hat Into The Ring (again?) Did OpenAI want to remind everyone they're STILL here amidst the LLama/Mistral frenzy? Maybe that's why they released SearchGPT - their newest "search engine that can hold a conversation" tool. Again, waitlisted, but unlike with voice mode... I got access. 😅 The good: Fast. Really fast. And impressively competent, considering it's still a demo. Handles complex queries well, and its "follow-up" ability blows even Perplexity out of the water (which is impressive). The less-good: Still feels early, especially for multi-language and super local stuff. Honestly, feels more like a sneak peek of an upcoming ChatGPT integration than a standalone competitor to Google. But either way, it's an interesting development - as you may have already learned from my full breakdown of SearchGPT vs. Perplexity Apple Intelligence is here! (sort of) And speaking of big companies, how could I not mention the Apple Intelligence release this week? Apple finally dropped iOS 18.1 with the long-awaited ON-DEVICE intelligence, powered by the Apple Foundational Model (AFM). Privacy nerds rejoice! 🎉 But how good is it? Mixed bag, I'd say. It's there, and definitely usable for summarization, rewriting tools, text suggestions... but Siri STILL isn't hooked up to it yet, tho speech to text is way faster and she does look more beautiful. 🤔 Apple did release a ridiculously detailed paper explaining how they trained this model on Apple silicon... and as Nisten (ever the voice of honesty) said on the show, "It looks like they've stacked a lot of the tricks that had been working ... overall, they're not actually really doing anything new ... the important thing here is how they apply it all as a system that has access to all your personal data." Yeah, ouch, BUT still exciting, especially as we get closer to truly personal, on-device AI experiences. Right now, it's less about revolutionary advancements, and more about how Apple can weave this into our lives seamlessly - they're focusing heavily on app intents, meaning AI that can actually DO things for you (think scheduling appointments, drafting emails, finding that photo buried in your library). I'll keep testing this, the more I play around the more I find out, like it suddenly started suggesting replies in messages for me for example, or I haven't yet seen the filtered notifications view where it smartly only lets important messages go through your focus mode. So stay tuned but it's likely not worth the beta iOS upgrade if you're not a dev or a very strong enthusiast. Wait, MORE Breaking News?? The AI World Doesn't Sleep! If this episode wasn't already enough... the very day of the live show, as we're chatting, I get bombarded with breaking news alerts from my ever-vigilant listeners. 1. Gemini 1.5 Pro 0801 - Now #1 on LMsys Arena! 🤯 Google apparently loves to ship big right AFTER I finish recording ThursdAI (this happened last week too!). Gemini's new version, released WHILE we were talking about older Gemini versions, claimed the top spot with an insane 1300 ELO score - crushing GPT-4 and taking home 1st place in Math, Instruction Following, and Hard Prompts! It's experimental, it's up on Google AI Studio... Go play with it! (and then tag me with your crazy findings!) And you know what? Some of this blog was drafted by this new model, in fact, I had the same prompt sent to Claude Sonnet 3.5, Mistral Large v2 and I tried LLama 3.1 405B but couldn't find any services that host a full context window, and this Gemini just absolutely demolished all of them on tone, on imitating my style, it even took some of the links from my TL;DR and incorporated them into the draft on its own! I've never seen any other model do that! I haven't used any LLMs so far for this blog besides proof-reading because, well they all kinda sucked, but damn, I dare you to try and find out where in this blog it was me and where it was Gemini. 2. GitHub Does a Hugging Face: Introducing GitHub Models! This dropped just as we wrapped - basically a built-in marketplace where you can try, test, and deploy various models right within GitHub! They've already got LLaMa, Mistral, and some Azure-hosted GPT-4o stuff - very intriguing... Time will tell what Microsoft is cooking here, but you can bet I'll be investigating!🕵️ AI Art & Diffusion New Stability: Black Forest Labs and FLUX.1 Rise! Talk about a comeback story: 14 EX Stability AI pros led by Robin Rombach, Andreas Blatmann & Patrick Esser the OG creaters of Stable Diffusion with $31 million in funding from a16z, and are back to make diffusion dreams come true. Enter Black Forest Labs. Their first gift? FLUX.1 - a suite of text-to-image models so good, they're breaking records. I saw those demos and wow. It's good, like REALLY good. 🤯 And the real bomb? They're working on open-source TEXT-TO-VIDEO! That's right, imagine generating those mind-blowing moving visuals... with code anyone can access. It's in their "Up Next" section, so watch that space - it's about to get REAL interesting. Also... Midjourney 6.1 also came out, and it looks GOOD And you can see a comparison between the two new leading models in this thread by Noah Hein Tools & Hardware: When AI Gets Real (And Maybe Too Real...) You knew I had to close this madness out with some Hardware, because hardware means that we actually are interacting with these incredible models in a meaningful way. Friend.com: When Your AI Is... Always Listening? 🤨 And then this happened... Avi Schiffman (finally) announces friend.com. with an amazingly dystopian promo video from Sandwich. Videos. ~ 22 million views and counting, not by accident! Link to Video. It's basically an always-on, listening pendant. "A little like wearing a wire" as Nisten so eloquently put it. 🎧 Not for memory extension or productivity... for friendship. Target audience? Lonely people who want a device that captures and understands their entire lives, but in an almost comforting way (or maybe unsettling, depending on your viewpoint!). The debate about privacy is already RAGING... But as Nisten pointed out: "Overall, it is a positive. ...The entire privacy talk and data ownership, I think that's a very important conversation to have". I kinda get the vision. Combine THIS tech with GPT-4 Voice speed... you could actually have engaging conversations, 24/7! 🤯 I don't think it's as simple as "this is dystopian, end of story". Character AI is EXPLODING right now, remember those usage stats, over 20 million users and counting? The potential to help with loneliness is real... The Developer Corner: Tools for Those Hacking This Future Gotta love these shoutouts: * FastHTML from Jeremy Howard: Not strictly AI, but if you hate JS and love Python, this one's for you - insanely FAST web dev using a mind-bending new syntax. FastHTML website link * Hamel Hussain's Applied LLM Course - All Videos NOW Free!: Want to learn from some of the best minds in the field (including Jeremy Howard, Shreya Shankar evaluation QUEEN, Charles Frye and tons of other great speakers)? This course covers it all - from finetuning to Rag Building to optimizing your prompts.Applied LLMs course - free videos link AND ALSO ... Nisten blew everyone's minds again in the end! Remember last week, we thought it'd take time before anyone could run Llama 3.1 405B on just CPU? Well, this crazy genius already cracked the code - seven tokens per second on a normal CPU! 🤯 If you're a researcher who hates using cloud GPUs (or wants to use ALL THOSE CORES in your Lambda machine, wink wink)... get ready. Look, I'm not going to sit here and pretend that weeks are not getting crazier, it takes me longer and longer to prep for the show, and really is harder and harder to contain the show to 2 hours, and we had 3 breaking news stories just today! So we're accelerating, and I'll likely be using a bit of support from AI, but only if it's good, and only if it's proof read by me, so please let me know if you smell slop! I really wanna know! ThursdAI - Recaps of the most high signal AI weekly spaces is a reader-supported publication. To receive new posts and support my work, consider becoming a free or paid subscriber. This is a public episode. If you'd like to discuss this with other subscribers or get access to bonus episodes, visit sub.thursdai.news/subscribe | |||
08 Aug 2024 | 📅 ThursdAI - Aug8 - Qwen2-MATH King, tiny OSS VLM beats GPT-4V, everyone slashes prices + 🍓 flavored OAI conspiracy | 01:44:14 | |
Hold on tight, folks, because THIS week on ThursdAI felt like riding a roller coaster through the wild world of open-source AI - extreme highs, mind-bending twists, and a sprinkle of "wtf is happening?" conspiracy theories for good measure. 😂 Theme of this week is, Open Source keeps beating GPT-4, while we're inching towards intelligence too cheap to meter on the API fronts. We even had a live demo so epic, folks at the Large Hadron Collider are taking notice! Plus, strawberry shenanigans abound (did Sam REALLY tease GPT-5?), and your favorite AI evangelist nearly got canceled on X! Buckle up; this is gonna be another long one! 🚀 Qwen2-Math Drops a KNOWLEDGE BOMB: Open Source Wins AGAIN! When I say "open source AI is unstoppable", I MEAN IT. This week, the brilliant minds from Alibaba's Qwen team decided to show everyone how it's DONE. Say hello to Qwen2-Math-72B-Instruct - a specialized language model SO GOOD at math, it's achieving a ridiculous 84 points on the MATH benchmark. 🤯 For context, folks... that's beating GPT-4, Claude Sonnet 3.5, and Gemini 1.5 Pro. We're not talking incremental improvements here - this is a full-blown DOMINANCE of the field, and you can download and use it right now. 🔥 Get Qwen-2 Math from HuggingFace here What made this announcement EXTRA special was that Junyang Lin , the Chief Evangelist Officer at Alibaba Qwen team, joined ThursdAI moments after they released it, giving us a behind-the-scenes peek at the effort involved. Talk about being in the RIGHT place at the RIGHT time! 😂 They painstakingly crafted a massive, math-specific training dataset, incorporating techniques like Chain-of-Thought reasoning (where the model thinks step-by-step) to unlock this insane level of mathematical intelligence. "We have constructed a lot of data with the form of ... Chain of Thought ... And we find that it's actually very effective. And for the post-training, we have done a lot with rejection sampling to create a lot of data sets, so the model can learn how to generate the correct answers" - Junyang Lin Now I gotta give mad props to Qwen for going beyond just raw performance - they're open-sourcing this beast under an Apache 2.0 license, meaning you're FREE to use it, fine-tune it, adapt it to your wildest mathematical needs! 🎉 But hold on... the awesomeness doesn't stop there! Remember those smaller, resource-friendly LLMs everyone's obsessed with these days? Well, Qwen released 7B and even 1.5B versions of Qwen-2 Math, achieving jaw-dropping scores for their size (70 for the 1.5B?? That's unheard of!).🤯 Nisten nearly lost his mind when he heard that - and trust me, he's seen things. 😂 "This is insane! This is... what, Sonnet 3.5 gets what, 71? 72? This gets 70? And it's a 1.5B? Like I could run that on someone's watch. Real." - Nisten With this level of efficiency, we're talking about AI-powered calculators, tutoring apps, research tools that run smoothly on everyday devices. The potential applications are endless! MiniCPM-V 2.6: A Pocket-Sized GPT-4 Vision... Seriously! 🤯 If Qwen's Math marvel wasn't enough open-source goodness for ya, OpenBMB had to get in on the fun too! This time, they're bringing the 🔥 to vision with MiniCPM-V 2.6 - a ridiculous 8 billion parameter VLM (visual language model) that packs a serious punch, even outperforming GPT-4 Vision on OCR benchmarks! OpenBMB drops a bomb on X here I'll say this straight up: talking about vision models in a TEXT-based post is hard. You gotta SEE it to believe it. But folks... TRUST ME on this one. This model is mind-blowing, capable of analyzing single images, multi-image sequences, and EVEN VIDEOS with an accuracy that rivaled my wildest hopes for open-source.🤯 Check out their playground and prepare to be stunned It even captured every single nuance in this viral toddler speed-running video I threw at it, with an accuracy I haven't seen in models THIS small: "The video captures a young child's journey through an outdoor park setting. Initially, the child ... is seen sitting on a curved stone pathway besides a fountain, dressed in ... a green t-shirt and dark pants. As the video progresses, the child stands up and begins to walk ..." Junyang said that they actually collabbed with the OpenBMB team and knows firsthand how much effort went into training this model: "We actually have some collaborations with OpenBMB... it's very impressive that they are using, yeah, multi-images and video. And very impressive results. You can check the demo... the performance... We care a lot about MMMU [the benchmark], but... it is actually relying much on large language models." - Junyang Lin Nisten and I have been talking for months about the relationship between these visual "brains" and the larger language model base powering their "thinking." While it seems smaller models are catching up fast, combining a top-notch visual processor like MiniCPM-V with a monster LLM like Quen72B or Llama 405B could unlock truly unreal capabilities. This is why I'm excited - open source lets us mix and match like this! We can Frankenstein the best parts together and see what emerges... and it's usually something mind-blowing. 🤯 Thank you for reading ThursdAI - Recaps of the most high signal AI weekly spaces. This post is public so feel free to share it. From the Large Hadron Collider to YOUR Phone: This Model Runs ANYWHERE 🚀 While Qwen2-Math is breaking records on one hand, Nisten's latest creation, Biggie-SmoLlm, is showcasing the opposite side of the spectrum. Trying to get the smallest/fastest coherent LLM possible, Nisten blew up on HuggingFace. Biggie-SmoLlm (Hugging Face) is TINY, efficient, and with some incredible optimization work from the folks right here on the show, it's reaching an insane 330 tokens/second on regular M3 chips. 🤯 That's WAY faster than real-time conversation, folks! And thanks to Eric Hartford's (from Cognitive Computation) awesome new optimizer, (Grok AdamW) it's surprisingly coherent for such a lil' fella. The cherry on top? Someone messaged Nisten saying they're using Biggie-SmoLlm at the Large. Hadron. Collider. 😳 I'll let that sink in for a second. It was incredible having ALL the key players behind Biggie-SmoLlm right there on stage: LDJ (whose Capybara dataset made it teaching-friendly), Junyang (whose Qwen work served as the base), and Eric (the optimizer mastermind himself). THIS, my friends, is what the ThursdAI community is ALL about! 🚀 Speaking of which this week we got a new friend of the pod, Mark Saroufim, a long time PyTorch core maintainer, to join the community. This Week's Buzz (and Yes, It Involves Making AI Even Smarter) 🤓 NeurIPS Hacker Cup 2024 - Can You Solve Problems Humans Struggle With? 🤔 I've gotta hand it to my PyTorch friend, Mark Saroufim. He knows how to make AI interesting! He and his incredible crew (Weiwei from MSFT, some WandB brainiacs, and more) are bringing you NeurIPS Hacker Cup 2024 - a competition to push those coding agents to their ABSOLUTE limits. 🚀 This isn't your typical "LeetCode easy" challenge, folks... These are problems SO hard, years of competitive programming experience are required to even attempt them! Mark himself said, “At this point, like, if a model does make a significant dent in this competition, uh, I think people would need to acknowledge that, like, LLMs can do a form of planning. ” And don't worry, total beginners: Mark and Weights & Biases are hosting a series of FREE sessions to level you up. Get those brain cells prepped and ready for the challenge and then Join the NeurIPS Hacker Cup Discord P.S. We're ALSO starting a killer AI Salon series in our SF office August 15th! You'll get a chance to chat with researches like Shreya Shankar - she's a leading voice on evaluation. More details and free tickets right here! AI Salons Link Big Co & APIs - Towards intelligence too cheap to meter Open-source was crushing it this week... but that didn't stop Big AI from throwing a few curveballs. OpenAI is doubling down on structured data (AND cheaper models!), Google slashed Gemini prices again (as we trend towards intelligence too cheap to meter), and a certain strawberry mystery took over Twitter. DeepSeek context caching lowers price by 90% automatiically DeepSeek, those masters of ridiculously-good coding AI, casually dropped a bombshell - context caching for their API! 🤯 If you're like "wait, what does THAT mean?", listen up because this is game-changing for production-grade AI: * Problem: LLMs get fed the ENTIRE conversation history EVERY. SINGLE. TIME. This wastes compute (and $$$) when info is repeated. * Solution: DeepSeek now remembers what you've said, automatically pulling from a cache when the conversation goes down familiar paths. * The Win: Up to 90% cheaper API calls. Yes, NINETY.😳 It costs 1.4 CENTS per million tokens for cached content. Let THAT sink in. 🤯 As Nisten (always bringing the technical breakdowns) explained: "Everyone should be using LLMs this way!...The simplest way is to have a long conversation ... then you save it on disk... you don't have to wait again ... [it's] kind of free. DeepSeek... did this in a more dynamic way". - Nisten Even Matt Shumer, who usually advocates for clever prompting over massive context, got legitimately hyped about the possibilities: "For me, and how we use LLMs... instead of gathering a million examples... curate a hundred gold examples... you have something better than if you fine-tuned it, and cheaper, and faster..." - Matt Shumer Think about this... instead of painstakingly fine-tuning, we can "guide" models with expertly crafted examples, letting them learn "on the fly" with minimal cost. Context as the NEW fine-tuning! 🤯 P.S - Google actually also has caching on its Gemini API, but you have to opt-in, while this happens automatically with DeepSeek API! Google Goes "Price War Nuclear": Gemini Flash is Officially TOO CHEAP Speaking of sneaky advancements from Google... they also dropped an update SO casually impactful, it almost got lost in the shuffle. Gemini Flash (their smallest, but still crazy-good model) is now... 7.5 cents per million tokens for input and 30 cents per million tokens for output... (for up to 128k of context) I REPEAT: 7.5 cents... with LONG context!? 🤯 Google, please chill, MY SANITY cannot handle this price free-fall any longer! 😂 Full Breakdown of Gemini’s Crazy New Prices on Google’s Blog While this USUALLY means a model's performance gets quietly nerfed in exchange for lower costs... in Gemini's case? Let's just say... even I, a staunch defender of open-source, am kinda SHOOK by how GOOD this thing is NOW! After Google threw down this gauntlet, I actually used Gemini to draft my last ThursdAI newsletter (for the first time!). It nailed my tone and style better than any other model I've tried - and I've TRIED them ALL. 🤯 Even Nisten, who's super picky about his coding LLMs, gave it a rare nod of approval. Gemini's image understanding capabilities have improved significantly too! 🤯 Google also added improvements in how Gemini understands PDFs that are worth mentioning 👀 From JSON Headaches to REASONING Gains: What's Really New with GPT-4? While Matt Shumer, my go-to expert on all things practical AI, might not be immediately impressed by OpenAI's new structured output features, they're still a huge win for many developers. Tired of LLM JSON going haywire? Well, GPT-4 can now adhere to your exact schemas, delivering 100% reliable structured data, no need for Instructor! 🙌 This solves a real problem, even if the prompting gurus (like Matt) have figured out their own workarounds. The key is: * Determinism: This ain't your typical LLM chaos - they're guaranteeing consistency, essential for building reliable applications. * Ease of use: No need for external libraries - it's built right into the API! Plus... a sneaky price drop, folks! GPT-4 is now 50% cheaper for input tokens and 33% cheaper for output. As I said on the show: "Again, quite insane... we're getting 50% cheaper just without fanfare. We're going towards 'intelligence too cheap to meter'... it's crazy". And HERE'S the plot twist... multiple folks on stage (including the eager newcomer N8) noticed significant reasoning improvements in this new GPT-4 model. They tested it on tasks like lateral thinking puzzles and even anecdotally challenging tasks - and guess what? It consistently outperformed older versions. 🤯 "I have my own benchmark... of lateral thinking puzzles... the new GPT-4 [scored] roughly five to 10% higher... these are like really hard lateral thinking puzzles that require innovative reasoning ability". - N8 OpenAI isn't bragging about this upgrade explicitly, which makes me even MORE curious... 🤔 Mistral Joins the AGENT Hype Train (But Their Version is Different) Everybody wants a piece of that AI "Agent" pie, and now Mistral (the scrappy, efficient French company) is stepping up. They announced a double whammy this week: fine-tuning is here AND "les agents" have arrived... but their agents are NOT quite what we're seeing elsewhere (think AutoGPT, CrewAI, all those looped assistants). 🤔 Mistral's Blog Post - Fine-tuning & Agents... Ooh La La! Their fine-tuning service is pretty straightforward: upload your data and they'll host a bespoke Mistral Large V2 running through their API at no extra cost (very cool!). Their agents aren't based on agentic loop-running like what we see from those recursive assistants. As I pointed out on ThursdAI: "[Mistral] agents are not agentic... They're more similar to... GPTs for OpenAI or 'Projects' in Anthropic, where... you as a user add examples and preload context". It's more about defining agents with examples and system prompts, essentially letting Mistral "pre-tune" their models for specific tasks. This lets you deploy those agents via the API or to their LeChat platform - pretty darn neat! Build your OWN agent - Mistral's "Agent Builder" is slick! While not as flashy as those recursive agents that build websites and write symphonies on their own, Mistral's take on the agent paradigm is strategic. It plays to their strengths: * Developer-focused: It's about creating bespoke, task-specific tools - think API integrations, code reviewers, or content generators. * Ease of deployment: No need for complex loop management, Mistral handles the hard parts for you! Mistral even teased that they'll eventually be incorporating tool use... so these "pre-tuned" agents could quickly evolve into something very interesting. 😏 NVIDIA leak about downloading videos went viral (And the Internet... Didn't Like That!) This week, I found myself unexpectedly at the center of an X drama explosion (fun times! 😅 ) when some leaked NVIDIA Slack messages showed them discussing which YouTube channels to scrape. My crime? I dared to ask how this is different from how Google creating Street View, filming every street possible without asking for permission. My Honest Question that Sparked AI Outrage The Internet, as it often does, had thoughts . The tweet blew up (like a million views blew up). I was labeled an apologist, a shill, all kinds of charming things... 😂 It got so intense, I had to MUTE the whole thing for my sanity's sake. BUT it brings up serious issues: * AI & Copyright: Where the Heck are the Lines? When does inspiration become infringement when a model's trained on massive datasets? There's no legal precedent, folks, which is scary . * Ethics vs. Innovation: AI progress moves FAST... sometimes FASTER than our ability to grasp the implications. That's unsettling. * Twitter Pile-Ons & Nuance (aka What NOT to do): Look, I GET being passionate. BUT when criticism turns into name-calling and mob mentality, it shuts down any chance of meaningful conversation. That's not helping ANYONE. Strawberry Shenanigans: Theories, Memes, and a Little AI LARPing?🍓 And now, for the MAIN EVENT: STRAWBERRY! You might have heard whispers... seen those cryptic tweets... maybe witnessed that wild Twitter account firsthand! It all started with Sam Altman casually posting a pic of a strawberry garden with the caption "nice summer day". Then came the deluge - more pics of strawberries from OpenAI folks, even those cryptic, semi-official domain names LDJ uncovered... I even spotted a strawberry IN OUR audience for crying out loud! This thing spread like wildfire. 🔥 We spent a solid chunk of the episode piecing together the lore: Q*, the mystery model shrouded in secrecy for years, then that Bloomberg leak claiming it was code-named "Strawberry", and now this. It was peak AI conspiracy-theory land! We still don't have hard confirmation on Q*... but that strawberry account, spitting out fruit puns and pinging ChatGPT like a maniac? Some on ThursdAI (Yam, mostly) believe that this may not have been a human at all, but an early, uncontrolled attempt to have an AI manage its own PR. 😳 I almost bought it - especially the way it reacted to some of my live comments - but now... the LARP explanation seems more likely Many folks at OpenAI posted things with strawberries as well, was this a sign of something to come or were they just trying to bury the news that 3 executives departed the company this week under a mountain of 🍓? Cursor & Composer: When Coding Becomes AI-Powered Magic ✨ I love a good tool... and this week, my dev heart was a-flutter over Cursor . Tried it yet? Seriously, you need to! It's VS Code, but SUPERCHARGED with AI that'll make you question why Copilot ever existed. 😂 You can edit code by CHAT, summarize entire files with one click, zap bugs instantly ... but they just dropped their ultimate weapon: Composer. It's essentially a coding AGENT that does multi-file edits. 🤯 Matt Shumer (my SaaS wizard friend who adopted Cursor early) had some jaw-dropping examples: " [Composer] ... takes all the parts of Cursor you like and strings them together as an agent... it takes away a lot of the grunt work... you can say 'go add this feature'... it searches your files, figures out what to edit, then puts it together. ...I literally built a SaaS in 20 minutes!" - Matt Shumer Matt also said that using Cursor is required at their company! Even my stoic PyTorch friend, Mark, couldn't help but express some curiosity: "It's cool they're doing things like multi-file editing... pretty curious to see more projects along those lines" - Mark Serafim Yeah, it's still in the rough-around-the-edges stage (UX could use some polish). But THIS, folks, is the future of coding - less about hammering out syntax, more about describing INTENT and letting the AI handle the magic! 🤯 I can't wait to see what they do next. Download at cursor.sh and let me know what you think Conclusion: The Future Is FAST, Open, And Maybe a Bit TOO Spicy? 🌶️😂 Honestly, every single week leaves me awestruck by how fast this AI world is moving. 🤯 We went from "transformers? Huh?" to 70-point math models running on SMARTWATCHES and AI building ENTIRE web apps in less than two years. And I still haven't got GPT-4's new voice model yet!! Open source keeps proving its power, even THOSE BIG companies are getting in on the action (look at those Google prices! 😍), and then you've got those captivating mysteries keeping us on our toes... like those damned strawberries! 🍓 What DOES OpenAI have up their sleeve?? As always, huge THANK YOU to the amazing guests who make this show what it is - this week, extra kudos to Junyang, Nisten, LDJ, Mark, Yam, and Eric, you guys ROCK. 🔥 And HUGE gratitude to each and every ONE of you readers/listeners (and NEW folks who stuck around after those Strawberry bait tweets! 😂) You make this ThursdAI community truly unstoppable. 💪 Keep on building, stay insanely curious, and I'll see you next Thursday - ready or not, that AI future is coming in hot! 🔥🚀 ThursdAI - Recaps of the most high signal AI weekly spaces is a reader-supported publication. To receive new posts and support my work, consider becoming a free or paid subscriber. This is a public episode. If you'd like to discuss this with other subscribers or get access to bonus episodes, visit sub.thursdai.news/subscribe | |||
15 Aug 2024 | 📅 ThursdAI - ChatGPT-4o back on top, Nous Hermes 3 LLama finetune, XAI uncensored Grok2, Anthropic LLM caching & more AI news from another banger week | 02:02:29 | |
Look these crazy weeks don't seem to stop, and though this week started out a bit slower (while folks were waiting to see how the speculation about certain red berry flavored conspiracies are shaking out) the big labs are shipping! We've got space uncle Elon dropping an "almost-gpt4" level Grok-2, that's uncensored, has access to real time data on X and can draw all kinds of images with Flux, OpenAI announced a new ChatGPT 4o version (not the one from last week that supported structured outputs, a different one!) and Anthropic dropping something that makes AI Engineers salivate! Oh, and for the second week in a row, ThursdAI live spaces were listened to by over 4K people, which is very humbling, and awesome because for example today, Nous Research announced Hermes 3 live on ThursdAI before the public heard about it (and I had a long chat w/ Emozilla about it, very well worth listening to) TL;DR of all topics covered: * Big CO LLMs + APIs * Xai releases GROK-2 - frontier level Grok, uncensored + image gen with Flux (𝕏, Blog, Try It) * OpenAI releases another ChatGPT-4o (and tops LMsys again) (X, Blog) * Google showcases Gemini Live, Pixel Bugs w/ Gemini, Google Assistant upgrades ( Blog) * Anthropic adds Prompt Caching in Beta - cutting costs by u to 90% (X, Blog) * AI Art & Diffusion & 3D * Flux now has support for LORAs, ControlNet, img2img (Fal, Replicate) * Google Imagen-3 is out of secret preview and it looks very good (𝕏, Paper, Try It) * This weeks Buzz * Using Weights & Biases Weave to evaluate Claude Prompt Caching (X, Github, Weave Dash) * Open Source LLMs * NousResearch drops Hermes 3 - 405B, 70B, 8B LLama 3.1 finetunes (X, Blog, Paper) * NVIDIA Llama-3.1-Minitron 4B (Blog, HF) * AnswerAI - colbert-small-v1 (Blog, HF) * Vision & Video * Runway Gen-3 Turbo is now available (Try It) Big Companies & LLM APIs Grok 2: Real Time Information, Uncensored as Hell, and… Flux?! The team at xAI definitely knows how to make a statement, dropping a knowledge bomb on us with the release of Grok 2. This isn't your uncle's dad joke model anymore - Grok 2 is a legitimate frontier model, folks. As Matt Shumer excitedly put it “If this model is this good with less than a year of work, the trajectory they’re on, it seems like they will be far above this...very very soon” 🚀 Not only does Grok 2 have impressive scores on MMLU (beating the previous GPT-4o on their benchmarks… from MAY 2024), it even outperforms Llama 3 405B, proving that xAI isn't messing around. But here's where things get really interesting. Not only does this model access real time data through Twitter, which is a MOAT so wide you could probably park a rocket in it, it's also VERY uncensored. Think generating political content that'd make your grandma clutch her pearls or imagining Disney characters breaking bad in a way that’s both hilarious and kinda disturbing all thanks to Grok 2’s integration with Black Forest Labs Flux image generation model. With an affordable price point ($8/month for x Premium including access to Grok 2 and their killer MidJourney competitor?!), it’ll be interesting to see how Grok’s "truth seeking" (as xAI calls it) model plays out. Buckle up, folks, this is going to be wild, especially since all the normies now have the power to create political memes, that look VERY realistic, within seconds. Oh yeah… and there’s the upcoming Enterprise API as well… and Grok 2’s made its debut in the wild on the LMSys Arena, lurking incognito as "sus-column-r" and is now placed on TOP of Sonnet 3.5 and comes in as number 5 overall! OpenAI last ChatGPT is back at #1, but it's all very confusing 😵💫 As the news about Grok-2 was settling in, OpenAI decided to, well… drop yet another GPT-4.o update on us. While Google was hosting their event no less. Seriously OpenAI? I guess they like to one-up Google's new releases (they also kicked Gemini from the #1 position after only 1 week there) So what was anonymous-chatbot in Lmsys for the past week, was also released in ChatGPT interface, is now the best LLM in the world according to LMSYS and other folks, it's #1 at Math, #1 at complex prompts, coding and #1 overall. It is also available for us developers via API, but... they don't recommend using it? 🤔 The most interesting thing about this release is, they don't really know to tell us why it's better, they just know that it is, qualitatively and that it's not a new frontier-class model (ie, not 🍓 or GPT5) Their release notes on this are something else 👇 Meanwhile it's been 3 months, and the promised Advanced Voice Mode is only in the hands of a few lucky testers so far. Anthropic Releases Prompt Caching to Slash API Prices By up to 90% Anthropic joined DeepSeek's game of "Let's Give Devs Affordable Intelligence," this week rolling out prompt caching with up to 90% cost reduction on cached tokens (yes NINETY…🤯 ) for those of you new to all this technical sorcery Prompt Caching allows the inference provider to save users money by reusing repeated chunks of a long prompt form cache, reducing pricing and increasing time to first token, and is especially beneficial for longer contexts (>100K) use-cases like conversations with books, agents with a lot of memory, 1000 examples in prompt etc' We covered caching before with Gemini (in Google IO) and last week with DeepSeek, but IMO this is a better implementation from a frontier lab that's easy to get started, manages the timeout for you (unlike Google) and is a no brainer implementation. And, you'll definitely want to see the code to implement it all yourself, (plus Weave is free!🤩): "In this week's buzz category… I used Weave, our LLM observability tooling to super quickly evaluate how much cheaper Cloud Caching from Anthropic really is, I did a video of it and I posted the code … If you're into this and want to see how to actually do this … how to evaluate, the code is there for you" - Alex With the ridiculous 90% price drop for those cached calls (Haiku basically becomes FREE and cached Claude is costs like Haiku, .30 cents per 1Mtok). For context, I took 5 transcripts of 2 hour podcast conversations, and it amounted to ~110,000 tokens overall, and was able to ask questions across all this text, and it cost me less than $1 (see in the above video) Code Here + Weave evaluation Dashboard here AI Art, Diffusion, and Personalized AI On the Fly Speaking of mind blowing, Flux took over this week, thanks in no small part to Elon strategically leveraging their tech in Grok (and everyone reminding everyone else, that it's not Grok creating images, it's Flux!) Now, remember, the REAL magic happens when code meets open source, “Flux now has support for LORAs, ControlNet, img2img…" meaning developers have turned those foundational tools into artistic wizardry. With as little as $5 bucks and a few pictures, “You can train the best image model on your own face. ”🤯 (Seriously folks, head up to Fal.ai, give it a whirl… it’s awesome) Now if you combine the LORA tech with ControlNet tech, you can get VERY creepy very fast (I'm using my own face here but you get the idea), here's "me" as the distracted boyfriend meme, and the girlfriend, and the distraction 😂 (I'm sorry you had to see this, AI has gone too far! Shut it all down!) If seeing those creepy faces on screen isn't for you (I totally get that) there’s also Google IMAGEN 3, freshly escaped from secret preview and just waiting for you to unleash those artistic prompts on it! Google, despite being… Google, somehow figured out that a little competition does a lab good and rolled out a model that’s seriously impressive. Runway Video Gets a "Turbocharged" Upgrade🚀🚀🚀 Ever tried those jaw-dropping text-to-video generators but groaned as you watched those seconds of video render painfully slowly?😭 Well Runway, creators of Gen 3, answered our prayers with the distilled turbocharged version that churns out those visuals in a blink 🤯🤯🤯 . What's truly cool is they unlocked it for FREE tier users (sign up and unleash those cinematic prompts right now!), letting everyday folks dip their toes in those previously-unfathomable waters. Even the skeptics at OpenBMB (Junyang knows what I'm talking about…) had to acknowledge that their efforts with MiniCPM V are impressive, especially the smooth way it captures video sequences better than models even twice its size 🤯. Open Source: Hermes 3 and The Next Generation of Open AI 🚀 NousResearch Dropped Hermes 3: Your New Favorite AI (Yes Really) In the ultimate “We Dropped This On ThursdAI Before Even HuggingFace”, the legendary team at NousResearch dropped the hottest news since Qwen decided to play math God: Hermes 3 is officially here! 🤯 “You’re about to get to use the FIRST big Finetune of LLama 3.1 405B… We don’t think there have been finetunes,” announced Emozilla who’s both co founder and resident master wizard of all things neural net, “And it's available to try for free thanks to Lambda, you can try it out right here ” (you’re all racing to their site as I type this, I KNOW it!). Not ONLY does this beauty run ridiculously smooth on Lambda, but here’s the real TL;DR: * Hermes 3 isn’t just 405B; there are 70B and 8B versions dropping simultaneously on Hugging Face, ready to crush benchmarks and melt your VRAM (in a GOOD way… okay maybe not so great for your power bill 😅). * On Benchmark, they beat LLama 3.1 instruct on a few evals and lose on some, which is quite decent, given that Meta team did an amazing job with their instruct finetuning (and probably spent millions of $ on it too) * Hermes 3 is all about user alignment, which our open source champion Wolfram Ravenwolf summarized beautifully: “When you have a model, and you run it on your system, IT MUST BE LOYAL TO YOU.” 😈 Hermes 3 does just that with incredibly precise control via its godlike system prompt: “In Hermes 3 the system prompt is KING,” confirmed Emoz. It’s so powerful that the 405B version was practically suffering existential angst in their first conversation… I read that part outloud during the space, but here you go, this is their first conversation, and he goes into why this they thing this happened, in our chat that's very worth listening to This model was trained on a bunch of datasources that they will release in the future, and includes tool use, and a slew of tokens that you can add in the system prompt, that will trigger abilities in this model to do chain of thought, to do scratchpad (think, and then rethink), to cite from sources for RAG purposes and a BUNCH more. The technical report is HERE and is worth diving into as is our full conversation with Emozilla on the pod. Wrapping Things Up… But We’re Just Getting Started! 😈 I know, I KNOW, your brain is already overflowing but we barely SCRATCHED the surface… We also dove into NVIDIA's research into new pruning and distilling techniques, TII Falcon’s attempt at making those State Space models finally challenge the seemingly almighty Transformer architecture (it's getting closer... but has a way to go!), plus AnswerAI's deceptively tiny Colbert-Small-V1, achieving remarkable search accuracy despite its featherweight size and a bunch more... See you all next week for what’s bound to be yet another wild AI news bonanza… Get those download speeds prepped, we’re in for a wild ride. 🔥 This is a public episode. If you'd like to discuss this with other subscribers or get access to bonus episodes, visit sub.thursdai.news/subscribe | |||
22 Aug 2024 | 📅 AI21 Jamba 1.5, DIY Meme Faces, 8yo codes with AI and a Doomsday LLM Device?! | 01:41:39 | |
Hey there, Alex here with an end of summer edition of our show, which did not disappoint. Today is the official anniversary of stable diffusion 1.4 can you believe it? It's the second week in the row that we have an exclusive LLM launch on the show (after Emozilla announced Hermes 3 on last week's show), and spoiler alert, we may have something cooking for next week as well! This edition of ThursdAI is brought to you by W&B Weave, our LLM observability toolkit, letting you evaluate LLMs for your own use-case easily Also this week, we've covered both ends of AI progress, doomerist CEO saying "Fck Gen AI" vs an 8yo coder and I continued to geek out on putting myself into memes (I promised I'll stop... at some point) so buckle up, let's take a look at another crazy week: TL;DR * Open Source LLMs * AI21 releases Jamba1.5 Large / Mini hybrid Mamba MoE (X, Blog, HF) * Microsoft Phi 3.5 - 3 new models including MoE (X, HF) * BFCL 2 - Berkley Function Calling Leaderboard V2 (X, Blog, Leaderboard) * NVIDIA - Mistral Nemo Minitron 8B - Distilled / Pruned from 12B (HF) * Cohere paper proves - code improves intelligence (X, Paper) * MOHAWK - transformer → Mamba distillation method (X, Paper, Blog) * AI Art & Diffusion & 3D * Ideogram launches v2 - new img diffusion king 👑 + API (X, Blog, Try it) * Midjourney is now on web + free tier (try it finally) * Flux keeps getting better, cheaper, faster + adoption from OSS (X, X, X) * Procreate hates generative AI (X) * Big CO LLMs + APIs * Grok 2 full is finally available on X - performs well on real time queries (X) * OpenAI adds GPT-4o Finetuning (blog) * Google API updates - 1000 pages PDFs + LOTS of free tokens (X) * This weeks Buzz * Weights & Biases Judgement Day SF Hackathon in September 21-22 (Sign up to hack) * Video * Hotshot - new video model - trained by 4 guys (try it, technical deep dive) * Luma Dream Machine 1.5 (X, Try it) * Tools & Others * LMStudio 0.0.3 update - local RAG, structured outputs with any model & more (X) * Vercel - Vo now has chat (X) * Ark - a completely offline device - offline LLM + worlds maps (X) * Ricky's Daughter coding with cursor video is a must watch (video) The Best of the Best: Open Source Wins with Jamba, Phi 3.5, and Surprise Function Calling Heroes We kick things off this week by focusing on what we love the most on ThursdAI, open-source models! We had a ton of incredible releases this week, starting off with something we were super lucky to have live, the official announcement of AI21's latest LLM: Jamba. AI21 Officially Announces Jamba 1.5 Large/Mini – The Powerhouse Architecture Combines Transformer and Mamba While we've covered Jamba release on the show back in April, Jamba 1.5 is an updated powerhouse. It's 2 models, Large and Mini, both MoE and both are still hybrid architecture of Transformers + Mamba that try to get both worlds. Itay Dalmedigos, technical lead at AI21, joined us on the ThursdAI stage for an exclusive first look, giving us the full rundown on this developer-ready model with an awesome 256K context window, but it's not just the size – it’s about using that size effectively. AI21 measured the effective context use of their model on the new RULER benchmark released by NVIDIA, an iteration of the needle in the haystack and showed that their models have full utilization of context, as opposed to many other models. “As you mentioned, we’re able to pack many, many tokens on a single GPU. Uh, this is mostly due to the fact that we are able to quantize most of our parameters", Itay explained, diving into their secret sauce, ExpertsInt8, a novel quantization technique specifically designed for MoE models. Oh, and did we mention Jamba is multilingual (eight languages and counting), natively supports structured JSON, function calling, document digestion… basically everything developers dream of. They even chucked in citation generation, as it's long context can contain full documents, your RAG app may not even need to chunk anything, and the citation can cite full documents! Berkeley Function Calling Leaderboard V2: Updated + Live (link) Ever wondered how to measure the real-world magic of those models boasting "I can call functions! I can do tool use! Look how cool I am!" 😎? Enter the Berkeley Function Calling Leaderboard (BFCL) 2, a battleground where models clash to prove their function calling prowess. Version 2 just dropped, and this ain't your average benchmark, folks. It's armed with a "Live Dataset" - a dynamic, user-contributed treasure trove of real-world queries, rare function documentations, and specialized use-cases spanning multiple languages. Translation: NO more biased, contaminated datasets. BFCL 2 is as close to the real world as it gets. So, who’s sitting on the Function Calling throne this week? Our old friend Claude 3.5 Sonnet, with an impressive score of 73.61. But breathing down its neck is GPT 4-0613 (the OG Function Calling master) with 73.5. That's right, the one released a year ago, the first one with function calling, in fact the first LLM with function calling as a concept IIRC! Now, prepare for the REAL plot twist. The top-performing open-source model isn’t some big name, resource-heavy behemoth. It’s a tiny little underdog called Functionary Medium 3.1, a finetuned version of Llama 3.1 that blew everyone away. It even outscored both versions of Claude 3 Opus AND GPT 4 - leaving folks scrambling to figure out WHO created this masterpiece. “I’ve never heard of this model. It's MIT licensed from an organization called MeetKai. Have you guys heard about Functionary Medium?” I asked, echoing the collective bafflement in the space. Yep, turns out there’s gold hidden in the vast landscape of open source models, just waiting to be unearthed ⛏️. Microsoft updates Phi 3.5 - 3 new models including an MoE + MIT license 3 new Phi's dropped this week, including an MoE one, and a new revamped vision one. They look very decent on benchmark yet again, with the mini version (3.8B) seemingly beating LLama 3.1 8B on a few benchmarks. However, as previously the excitement is met with caution because Phi models seem great on benchmarks but then actually talking with them, folks are not as impressed usually. Terry from BigCodeBench also saw a significant decrease in coding ability for Phi 3.5 vs 3.1 Of course, we're not complaining, the models released with 128K context and MIT license. The thing I'm most excited about is the vision model updates, it has been updated with "multi-frame image understanding and reasoning" which is a big deal! This means understanding videos more natively across scenes. This weeks Buzz Hey, if you're reading this, while sitting in the bay area, and you don't have plans for exactly a month from now, why don't you come and hack with me? (Register Free) Announcing, the first W&B hackathon, Judgement Day that's going to be focused on LLM as a judge! Come hack on innovative LLM as a judge ideas, UIs, evals and more, meet other like minded hackers and AI engineers and win great prizes! 🎨 AI Art: Ideogram Crowns Itself King, Midjourney Joins the Internet & FLUX everywhere While there was little news from big LLM labs this week, there is a LOT of AI art news, which is fitting to celebrate 2 year Stable Diffusion 1.4 anniversary! 👑 Ideogram v2: Text Wizardry and API Access (But No Loras… Yet?) With significantly improved realism, and likely the best text generation across all models out there, Ideogram v2 just took over the AI image generation game! Just look at that text sharpness! They now offer a selection of styles (Realistic, Design, 3D, Anime) and any aspect ratios you'd like and also, brands can now provide color palettes to control the outputs! Adding to this is a new API offering (.8c per image for the main model, .5c for the new turbo model of v2!) and a new IOS app, they also added the option (for premium users only) to search through a billion generations and their prompts, which is a great offering as well, as sometimes you don't even know what to prompt. They claim a significant improvement over Flux[pro] and Dalle-3 in text, alignment and overall, interesting that MJ was not compared! Meanwhile, Midjourney finally launched a website and a free tier, so no longer do you have to learn to use Discord to even try Midjourney. Meanwhile Flux enjoys the fruits of Open Source While the Ideogram and MJ fight it out for the closed source, Black Forest Labs enjoys the fruits of released their weights in the open. Fal just released an update that LORAs run 2.5x faster and 2.5x cheaper, CivitAI has LORAs for pretty much every character and celebrity ported to FLUX already, different techniques like ControlNets Unions, IPAdapters and more are being trained as we speak and tutorials upon tutorials are released of how to customize these models, for free (shoutout to my friend Matt Wolfe for this one) you can now train your own face on fal.ai , replicate.com and astria.ai , and thanks to astria, I was able to find some old generations of my LORAs from the 1.5 days (not quite 1.4, but still, enough to show the difference between then and now) and whoa. 🤔 Is This AI Tool Necessary, Bro? Let’s end with a topic that stirred up a hornets nest of opinions this week: Procreate, a beloved iPad design app, publicly declared their "fing hate” for Generative AI. Yeah, you read that right. Hate. The CEO, in a public statement went FULL scorched earth - proclaiming that AI-powered features would never sully the pristine code of their precious app. “Instead of trying to bridge the gap, he’s creating more walls", Wolfram commented, echoing the general “dude… what?” vibe in the space. “It feels marketeerial”, I added, pointing out the obvious PR play (while simultaneously acknowledging the very REAL, very LOUD segment of the Procreate community that cheered this decision). Here’s the thing: you can hate the tech. You can lament the potential demise of the human creative spark. You can rail against the looming AI overlords. But one thing’s undeniable: this tech isn't going anywhere. Meanwhile, 8yo coders lean in fully into AI As a contrast to this doomerism take, just watch this video of Ricky Robinette's eight-year-old daughter building a Harry Potter website in 45 minutes, using nothing but a chat interface in Cursor. No coding knowledge. No prior experience. Just prompts and the power of AI ✨. THAT’s where we’re headed, folks. It might be terrifying. It might be inspiring. But it’s DEFINITELY happening. Better to understand it, engage with it, and maybe try to nudge it in a positive direction, than burying your head in the sand and muttering “I bleeping hate this progress” like a cranky, Luddite hermit. Just sayin' 🤷♀️. AI Device to reboot civilization (if needed) I was scrolling through my feed (as I do VERY often, to bring you this every week) and I saw this and super quickly decided to invite the author to the show to talk about it. Adam Cohen Hillel has prototyped an AI hardware device, but this one isn't trying to record you or be your friend, no, this one comes with offline LLMs finetuned with health and bio information, survival tactics, and all of the worlds maps and works completely offline! This to me was a very exciting use for an LLM, a distilled version of all human knowledge, buried in a faraday cage, with replaceable batteries that runs on solar and can help you survive in the case of something bad happening, like really bad happening (think a solar flare that takes out the electrical grid or an EMP device). While improbable, I thought this was a great idea and had a nice chat with the creator, you should definitely give this one a listen, and if you want to buy one, he is going to sell them soon here This is it for this week, there have been a few updates from the big labs, OpenAI has opened Finetuneing for GPT-4o, and you can use your WandB API key in there to track those, which is cool, Gemini API now accepts incredibly large PDF files (up to 1000 pages) and Grok 2 is finally on X (not mini from last week) See you next week (we will have another deep dive!) This is a public episode. If you'd like to discuss this with other subscribers or get access to bonus episodes, visit sub.thursdai.news/subscribe | |||
30 Aug 2024 | 📅 ThursdAI - Aug 29 - AI Plays DOOM, Cerebras breaks inference records, Google gives new Geminis, OSS vision SOTA & 100M context windows!? | 01:35:04 | |
Hey, for the least time during summer of 2024, welcome to yet another edition of ThursdAI, also happy skynet self-awareness day for those who keep track :) This week, Cerebras broke the world record for fastest LLama 3.1 70B/8B inference (and came on the show to talk about it) Google updated 3 new Geminis, Anthropic artifacts for all, 100M context windows are possible, and Qwen beats SOTA on vision models + much more! As always, this weeks newsletter is brought to you by Weights & Biases, did I mention we're doing a hackathon in SF in September 21/22 and that we have an upcoming free RAG course w/ Cohere & Weaviate? TL;DR * Open Source LLMs * Nous DisTrO - Distributed Training (X , Report) * NousResearch/ hermes-function-calling-v1 open sourced - (X, HF) * LinkedIN Liger-Kernel - OneLine to make Training 20% faster & 60% more memory Efficient (Github) * Cartesia - Rene 1.3B LLM SSM + Edge Apache 2 acceleration (X, Blog) * Big CO LLMs + APIs * Cerebras launches the fastest AI inference - 447t/s LLama 3.1 70B (X, Blog, Try It) * Google - Gemini 1.5 Flash 8B & new Gemini 1.5 Pro/Flash (X, Try it) * Google adds Gems & Imagen to Gemini paid tier * Anthropic artifacts available to all users + on mobile (Blog, Try it) * Anthropic publishes their system prompts with model releases (release notes) * OpenAI has project Strawberry coming this fall (via The information) * This weeks Buzz * WandB Hackathon hackathon hackathon (Register, Join) * Also, we have a new RAG course w/ Cohere and Weaviate (RAG Course) * Vision & Video * Zhipu AI CogVideoX - 5B Video Model w/ Less 10GB of VRAM (X, HF, Try it) * Qwen-2 VL 72B,7B,2B - new SOTA vision models from QWEN (X, Blog, HF) * AI Art & Diffusion & 3D * GameNgen - completely generated (not rendered) DOOM with SD1.4 (project) * FAL new LORA trainer for FLUX - trains under 5 minutes (Trainer, Coupon for ThursdAI) * Tools & Others * SimpleBench from AI Explained - closely matches human experience (simple-bench.com) ThursdAI - Recaps of the most high signal AI weekly spaces is a reader-supported publication. To receive new posts and support my work, consider becoming a free or paid subscriber. Open Source Let's be honest - ThursdAI is a love letter to the open-source AI community, and this week was packed with reasons to celebrate. Nous Research DiStRO + Function Calling V1 Nous Research was on fire this week (aren't they always?) and they kicked off the week with the release of DiStRO, which is a breakthrough in distributed training. You see, while LLM training requires a lot of hardware, it also requires a lot of network bandwidth between the different GPUs, even within the same data center. Proprietary networking solutions like Nvidia NVLink, and more open standards like Ethernet work well within the same datacenter, but training across different GPU clouds has been unimaginable until now. Enter DiStRo, a new decentralized training by the mad geniuses at Nous Research, in which they reduced the required bandwidth to train a 1.2B param model from 74.4GB to just 86MB (857x)! This can have massive implications for training across compute clusters, doing shared training runs, optimizing costs and efficiency and democratizing LLM training access! So don't sell your old GPUs just yet, someone may just come up with a folding@home but for training the largest open source LLM, and it may just be Nous! Nous Research also released their function-calling-v1 dataset (HF) that was used to train Hermes-2, and we had InterstellarNinja who authored that dataset, join the show and chat about it. This is an incredible unlock for the open source community, as function calling become a de-facto standard now. Shout out to the Glaive team as well for their pioneering work that paved the way! LinkedIn's Liger Kernel: Unleashing the Need for Speed (with One Line of Code) What if I told you, that whatever software you develop, you can add 1 line of code, and it'll run 20% faster, and require 60% less memory? This is basically what Linkedin researches released this week with Liger Kernel, yes you read that right, Linkedin, as in the website you career related posts on! "If you're doing any form of finetuning, using this is an instant win"Wing Lian - Axolotl This absolutely bonkers improvement in training LLMs, now works smoothly with Flash Attention, PyTorch FSDP and DeepSpeed. If you want to read more about the implementation of the triton kernels, you can see a deep dive here, I just wanted to bring this to your attention, even if you're not technical, because efficiency jumps like these are happening all the time. We are used to seeing them in capabilities / intelligence, but they are also happening on the algorithmic/training/hardware side, and it's incredible to see! Huge shoutout to Byron and team at Linkedin for this unlock, check out their Github if you want to get involved! Qwen-2 VL - SOTA image and video understanding + open weights mini VLM You may already know that we love the folks at Qwen here on ThursdAI, not only because Junyang Lin is a frequeny co-host and we get to hear about their releases as soon as they come out (they seem to be releasing them on thursdays around the time of the live show, I wonder why!) But also because, they are committed to open source, and have released 2 models 7B and 2B with complete Apache 2 license! First of all, their Qwen-2 VL 72B model, is now SOTA at many benchmarks, beating GPT-4, Claude 3.5 and other much bigger models. This is insane. I literally had to pause Junyang and repeat what he said, this is a 72B param model, that beats GPT-4o on document understanding, on math, on general visual Q&A. Additional Capabilities & Smaller models They have added new capabilities in these models, like being able to handle arbitrary resolutions, but the one I'm most excited about is the video understanding. These models can now understand up to 20 minutes of video sequences, and it's not just "split the video to 10 frames and do image caption", no, these models understand video progression and if I understand correctly how they do it, it's quite genius. They the video embed time progression into the model using a new technique called M-RoPE, which turns the time progression into rotary positional embeddings. Now, the 72B model is currently available via API, but we do get 2 new small models with Apache 2 license and they are NOT too shabby either! 7B parameters (HF) and 2B Qwen-2 VL (HF) are small enough to run completely on your machine, and the 2B parameter, scores better than GPT-4o mini on OCR-bench for example! I can't wait to finish writing and go play with these models! Big Companies & LLM APIs The biggest news this week came from Cerebras System, a relatively unknown company, that shattered the world record for LLM inferencing out of the blue (and came on the show to talk about how they are doing it) Cerebras - fastest LLM inference on wafer scale chips Cerebras has introduced the concept of wafer scale chips to the world, which is, if you imagine a microchip, they are the size of a post stamp maybe? GPUs are bigger, well, Cerebras are making chips the sizes of an iPad (72 square inches), largest commercial chips in the world. And now, they created an inference stack on top of those chips, and showed that they have the fastest inference in the world, how fast? Well, they can server LLama 3.1 8B at a whopping 1822t/s. No really, this is INSANE speeds, as I was writing this, I copied all the words I had so far, went to inference.cerebras.ai , asked to summarize, pasted and hit send, and I immediately got a summary! "The really simple explanation is we basically store the entire model, whether it's 8B or 70B or 405B, entirely on the chip. There's no external memory, no HBM. We have 44 gigabytes of memory on chip."James Wang They not only store the whole model (405B coming soon), but they store it in full fp16 precision as well, so they don't quantize the models. Right now, they are serving it with 8K tokens in context window, and we had a conversation about their next steps being giving more context to developers. The whole conversation is well worth listening to, James and Ian were awesome to chat with, and while they do have a waitlist, as they gradually roll out their release, James said to DM him on X and mention ThursdAI, and he'll put you through, so you'll be able to get an OpenAI compatible API key and be able to test this insane speed. P.S - we also did an independent verification of these speeds, using Weave, and found Cerebras to be quite incredible for agentic purposes, you can read our report here and the weave dashboard here Anthropic - unlocking just-in-time applications with artifacts for all Well, if you aren't paying claude, maybe this will convince you. This week, anthropic announced that artifacts are available to all users, not only their paid customers. Artifacts are a feature in Claude that is basically a side pane (and from this week, a drawer in their mobile apps) that allows you to see what Claude is building, by rendering the web application almost on the fly. They have also trained Claude in working with that interface, so it knows about the different files etc Effectively, this turns Claude into a web developer that will build mini web applications (without backend) for you, on the fly, for any task you can think of. Drop a design, and it'll build a mock of it, drop some data in a CSV and it'll build an interactive onetime dashboard visualizing that data, or just ask it to build an app helping you split the bill between friends by uploading a picture of a bill. Artifacts are share-able and remixable, so you can build something and share with friends, so here you go, an artifact I made, by dropping my notes into claude, and asking for a magic 8 Ball, that will spit out a random fact from today's editing of ThursdAI. I also provided Claude with an 8Ball image, but it didn't work due to restrictions, so instead I just uploaded that image to claude and asked it to recreate it with SVG! And viola, a completely un-nessesary app that works! Google’s Gemini Keeps Climbing the Charts (But Will It Be Enough?) Sensing a disturbance in the AI force (probably from that Cerebras bombshell), Google rolled out a series of Gemini updates, including a new experimental Gemini 1.5 Pro (0827) with sharper coding skills and logical reasoning. According to LMSys, it’s already nipping at the heels of ChatGPT 4o and is number 2! Their Gemini 1.5 Flash model got a serious upgrade, vaulting to the #6 position on the arena. And to add to the model madness, they even released an Gemini Flash 8B parameter version for folks who need that sweet spot between speed and size. Oh, and those long-awaited Gems are finally starting to roll out. But get ready to open your wallet – this feature (preloading Gemini with custom context and capabilities) is a paid-tier exclusive. But hey, at least Imagen-3 is cautiously returning to the image generation game! AI Art & Diffusion Doom Meets Stable Diffusion: AI Dreams in 20FPS Glory (GameNGen) The future of video games is, uh, definitely going to be interesting. Just as everyone thought AI would be conquering Go or Chess, it seems we've stumbled into a different battlefield: first-person shooters. 🤯 This week, researchers in DeepMind blew everyone's minds with their GameNgen research. What did they do? They trained Stable Diffusion 1.4 on Doom, and I'm not just talking about static images - I'm talking about generating actual Doom gameplay in near real time. Think 20FPS Doom running on nothing but the magic of AI. The craziest part to me is this quote "Human raters are only slightly better than random chance at distinguishing short clips of the game from clips of the simulation" FAL Drops the LORA Training Time Bomb (and I Get a New Meme!) As you see, I haven't yet relaxed from making custom AI generations with Flux and customizing them with training LORAs. Two weeks ago, this used to take 45 minutes, a week ago, 20 minutes, and now, the wizards at FAL, created a new trainer that shrinks the training times down to less than 5 minutes! So given that the first upcoming SpaceX commercial spacewalk Polaris Dawn, I trained a SpaceX astronaut LORA and then combined my face with it, and viola, here I am, as a space X astronaut! BTW because they are awesome, Jonathan and Simo (who is the magician behind this new trainer) came to the show, announced the new trainer, but also gave all listeners of ThursdAI a coupon to train a LORA effectively for free, just use this link and start training! (btw I get nothing out of this, just trying to look out for my listeners!) That's it for this week, well almost that's it, magic.dev announced a new funding round of 320 million, and that they have a 100M context window capable models and coding product to go with it, but didn't yet release it, just as we were wrapping up. Sam Altman tweeted that OpenAI now has over 200 Million active users on ChatGPT and that OpenAI will collaborate with AI Safety institute. Ok now officially that's it! See you next week, when it's going to be 🍁 already brrr ThursdAI - Recaps of the most high signal AI weekly spaces is a reader-supported publication. To receive new posts and support my work, consider becoming a free or paid subscriber. This is a public episode. If you'd like to discuss this with other subscribers or get access to bonus episodes, visit sub.thursdai.news/subscribe | |||
06 Sep 2024 | 📅 ThursdAI - Sep 5 - 👑 Reflection 70B beats Claude 3.5, Anthropic Enterprise 500K context, 100% OSS MoE from AllenAI, 1000 agents world sim, Replit agent is the new Cursor? and more AI news | 01:44:56 | |
Welcome back everyone, can you believe it's another ThursdAI already? And can you believe me when I tell you that friends of the pod Matt Shumer & Sahil form Glaive.ai just dropped a LLama 3.1 70B finetune that you can download that will outperform Claude Sonnet 3.5 while running locally on your machine? Today was a VERY heavy Open Source focused show, we had a great chat w/ Niklas, the leading author of OLMoE, a new and 100% open source MoE from Allen AI, a chat with Eugene (pico_creator) about RWKV being deployed to over 1.5 billion devices with Windows updates and a lot more. In the realm of the big companies, Elon shook the world of AI by turning on the biggest training cluster called Colossus (100K H100 GPUs) which was scaled in 122 days 😮 and Anthropic announced that they have 500K context window Claude that's only reserved if you're an enterprise customer, while OpenAI is floating an idea of a $2000/mo subscription for Orion, their next version of a 100x better chatGPT?! TL;DR * Open Source LLMs * Matt Shumer / Glaive - Reflection-LLama 70B beats Claude 3.5 (X, HF) * Allen AI - OLMoE - first "good" MoE 100% OpenSource (X, Blog, Paper, WandB) * RWKV.cpp is deployed with Windows to 1.5 Billion devices * MMMU pro - more robust multi disipline multimodal understanding bench (proj) * 01AI - Yi-Coder 1.5B and 9B (X, Blog, HF) * Big CO LLMs + APIs * Replit launches Agent in beta - from coding to production (X, Try It) * Ilya SSI announces 1B round from everyone (Post) * Cohere updates Command-R and Command R+ on API (Blog) * Claude Enterprise with 500K context window (Blog) * Claude invisibly adds instructions (even via the API?) (X) * Google got structured output finally (Docs) * Amazon to include Claude in Alexa starting this October (Blog) * X ai scaled Colossus to 100K H100 GPU goes online (X) * DeepMind - AlphaProteo new paper (Blog, Paper, Video) * This weeks Buzz * Hackathon did we mention? We're going to have Eugene and Greg as Judges! * AI Art & Diffusion & 3D * ByteDance - LoopyAvatar - Audio Driven portait avatars (Page) Open Source LLMs Reflection Llama-3.1 70B - new 👑 open source LLM from Matt Shumer / GlaiveAI This model is BANANAs folks, this is a LLama 70b finetune, that was trained with a new way that Matt came up with, that bakes CoT and Reflection into the model via Finetune, which results in model outputting its thinking as though you'd prompt it in a certain way. This causes the model to say something, and then check itself, and then reflect on the check and then finally give you a much better answer. Now you may be thinking, we could do this before, RefleXion (arxiv.org/2303.11366) came out a year ago, so what's new? What's new is, this is now happening inside the models head, you don't have to reprompt, you don't even have to know about these techniques! So what you see above, is just colored differently, but all of it, is output by the model without extra prompting by the user or extra tricks in system prompt. the model thinks, plans, does chain of thought, then reviews and reflects, and then gives an answer! And the results are quite incredible for a 70B model 👇 Looking at these evals, this is a 70B model that beats GPT-4o, Claude 3.5 on Instruction Following (IFEval), MATH, GSM8K with 99.2% 😮 and gets very close to Claude on GPQA and HumanEval! (Note that these comparisons are a bit of a apples to ... different types of apples. If you apply CoT and reflection to the Claude 3.5 model, they may in fact perform better on the above, as this won't be counted 0-shot anymore. But given that this new model is effectively spitting out those reflection tokens, I'm ok with this comparison) This is just the 70B, next week the folks are planning to drop the 405B finetune with the technical report, so stay tuned for that! Kudos on this work, go give Matt Shumer and Glaive AI a follow! Allen AI OLMoE - tiny "good" MoE that's 100% open source, weights, code, logs We've previously covered OLMO from Allen Institute, and back then it was obvious how much commitment they have to open source, and this week they continued on this path with the release of OLMoE, an Mixture of Experts 7B parameter model (1B active parameters), trained from scratch on 5T tokens, which was completely open sourced. This model punches above its weights on the best performance/cost ratio chart for MoEs and definitely highest on the charts of releasing everything. By everything here, we mean... everything, not only the final weights file; they released 255 checkpoints (every 5000 steps), the training code (Github) and even (and maybe the best part) the Weights & Biases logs! It was a pleasure to host the leading author of the OLMoE paper, Niklas Muennighoff on the show today, so definitely give this segment a listen, he's a great guest and I learned a lot! Big Companies LLMs + API Anthropic has 500K context window Claude but only for Enterprise? Well, this sucks (unless you work for Midjourney, Airtable or Deloitte). Apparently Anthropic has been sitting on Claude that can extend to half a million tokens in the context window, and decided to keep it to themselves and a few trial enterprises, and package it as an Enterprise offering. This offering now includes, beyond just the context window, also a native Github integration, and a few key enterprise features like access logs, provisioning and SCIM and all kinds of "procurement and CISO required" stuff enterprises look for. To be clear, this is a great move for Anthropic, and this isn't an API tier, this is for their front end offering, including the indredible artifacts tool, so that companies can buy their employees access to Claude.ai and have them be way more productive coding (hence the Github integration) or summarizing (very very) long documents, building mockups and one off apps etc' Anthropic is also in the news this week, because Amazon announced that it'll use Claude as the backbone for the smart (or "remarkable" as they call it) Alexa brains coming up in October, which, again, incredible for Anthropic distribution, as there are maybe 100M Alexa users in the world or so. Prompt injecting must stop! And lastly, there have been mounting evidence, including our own Wolfram Ravenwolf that confirmed it, that Anthropic is prompt injecting additional context into your own prompts, in the UI but also via the API! This is awful practice and if anyone from there reads this newsletter, please stop or at least acknowledge. Claude apparently just... thinks that it's something my users said, when in fact, it's some middle layer of anthropic security decided to just inject some additional words in there! XAI turns on the largest training GPU SuperCluster Colossus - 100K H100 GPUS This is a huge deal for AI, specifically due to the time this took and the massive massive scale of this SuperCluster. SuperCluster means all these GPUs sit in one datacenter, drawing from the same power-grid and can effectively run single training jobs. This took just 122 days for Elon and the XAI team to go from an empty warehouse in Memphis to booting up an incredible 100K H100, and they claim that they will double this capacity by adding 50K H200 in the next few months. As Elon mentioned when they released Grok2, it was trained on 15K, and it matched GPT4! Per SemiAnalisys, this new Supercluster can train a GPT-4 level model in just 4 days 🤯 XAI was founded a year ago, and by end of this year, they plan for Grok to be the beast LLM in the world, and not just get to GPT-4ish levels, and with this + 6B investment they have taken in early this year, it seems like they are well on track, which makes some folks at OpenAI reportedly worried This weeks buzz - we're in SF in less than two weeks, join our hackathon! This time I'm very pleased to announce incredible judges for our hackathon, the spaces are limited, but there's still some spaces so please feel free to sign up and join us I'm so honored to announce that we'll have Eugene Yan (@eugeneyan), Greg Kamradt (@GregKamradt) and Charles Frye (@charles_irl) on the Judges panel. 🤩 It'll be incredible to have these folks see what hackers come up with, and I'm excited as this comes closer! Replit launches Agents beta - a fully integrated code → deployment agent Replit is a great integrated editing environment, with database and production in 1 click and they've had their LLMs trained on a LOT of code helping folks code for a while. Now they are launching agents, which seems very smart from them, given that development is much more than just coding. All the recent excitement we see about Cursor, is omitting the fact that those demos are only working for folks who already know how to set up the environment, and then there's the need to deploy to production, maintain. Replit has that basically built in, and now their Agent can build a plan and help you build those apps, and "ship" them, while showing you what they are doing. This is massive, and I can't wait to play around with this! The additional benefit of Replit is that they nailed the mobile app experience as well, so this now works from mobile, on the go! In fact, as I was writing this, I got so excited that I paused for 30 minutes, payed the yearly subscription and decided to give building an app a try! The fact that this can deploy and run the server and the frontend, detect errors, fix them, and then also provision a DB for me, provision Stripe, login buttons and everything else is quite insane. Can't wait to see what I can spin up with this 🔥 (and show all of you!) Loopy - Animated Avatars from ByteDance A new animated avatar project from folks at ByteDance just dropped, and it’s WAY clearer than anything we’ve seen before, like EMO or anything else. I will just add this video here for you to enjoy and look at the earring movements, vocal cords, eyes, everything! I of course wanted to know if I’ll ever be able to use this, and .. likely no, here’s the response I got from Jianwen one of the Authors today. That's it for this week, we've talked about so much more in the pod, please please check it out. As for me, while so many exciting things are happening, I'm going on a small 🏝️ vacation until next ThursdAI, which will happen on schedule, so planning to decompress and disconnect, but will still be checking in, so if you see things that are interesting, please tag me on X 🙏 P.S - I want to shout out a dear community member that's been doing just that, @PresidentLin has been tagging me in many AI related releases, often way before I would even notice them, so please give them a follow! 🫡 ThursdAI - Recaps of the most high signal AI weekly spaces is a reader-supported publication. To receive new posts and support my work, consider becoming a free or paid subscriber. ThursdAI - Recaps of the most high signal AI weekly spaces is a reader-supported publication. To receive new posts and support my work, consider becoming a free or paid subscriber. This is a public episode. If you'd like to discuss this with other subscribers or get access to bonus episodes, visit sub.thursdai.news/subscribe | |||
13 Sep 2024 | 🔥 📅 ThursdAI - Sep 12 - OpenAI's 🍓 is called 01 and is HERE, reflecting on Reflection 70B, Google's new auto podcasts & more AI news from last week | 01:58:14 | |
March 14th, 2023 was the day ThursdAI was born, it was also the day OpenAI released GPT-4, and I jumped into a Twitter space and started chaotically reacting together with other folks about what a new release of a paradigm shifting model from OpenAI means, what are the details, the new capabilities. Today, it happened again! Hey, it's Alex, I'm back from my mini vacation (pic after the signature) and boy am I glad I decided to not miss September 12th! The long rumored 🍓 thinking model from OpenAI, dropped as breaking news in the middle of ThursdAI live show, giving us plenty of time to react live! But before this, we already had an amazing show with some great guests! Devendra Chaplot from Mistral came on and talked about their newly torrented (yeah they did that again) Pixtral VLM, their first multi modal! , and then I had the honor to host Steven Johnson and Raiza Martin from NotebookLM team at Google Labs which shipped something so uncannily good, that I legit said "holy fu*k" on X in a reaction! So let's get into it (TL;DR and links will be at the end of this newsletter) OpenAI o1, o1 preview and o1-mini, a series of new "reasoning" models This is it folks, the strawberries have bloomed, and we finally get to taste them. OpenAI has released (without a waitlist, 100% rollout!) o1-preview and o1-mini models to chatGPT and API (tho only for tier-5 customers) 👏 and are working on releasing 01 as well. These are models that think before they speak, and have been trained to imitate "system 2" thinking, and integrate chain-of-thought reasoning internally, using Reinforcement Learning and special thinking tokens, which allows them to actually review what they are about to say before they are saying it, achieving remarkable results on logic based questions. Specifically you can see the jumps in the very very hard things like competition math and competition code, because those usually require a lot of reasoning, which is what these models were trained to do well. New scaling paradigm Noam Brown from OpenAI calls this a "new scaling paradigm" and Dr Jim Fan explains why, with this new way of "reasoning", the longer the model thinks - the better it does on reasoning tasks, they call this "test-time compute" or "inference-time compute" as opposed to compute that was used to train the model. This shifting of computation down to inference time is the essence of the paradigm shift, as in, pre-training can be very limiting computationally as the models scale in size of parameters, they can only go so big until you have to start building out a huge new supercluster of GPUs to host the next training run (Remember Elon's Colossus from last week?). The interesting thing to consider here is, while current "thinking" times are ranging between a few seconds to a minute, imagine giving this model hours, days, weeks to think about new drug problems, physics problems 🤯. Prompting o1 Interestingly, a new prompting paradigm has also been introduced. These models now have CoT (think "step by step") built-in, so you no longer have to include it in your prompts. By simply switching to o1-mini, most users will see better results right off the bat. OpenAI has worked with the Devin team to test drive these models, and these folks found that asking the new models to just give the final answer often works better and avoids redundancy in instructions. The community of course will learn what works and doesn't in the next few hours, days, weeks, which is why we got 01-preview and not the actual (much better) o1. Safety implications and future plans According to Greg Brokman, this inference time compute also greatly helps with aligning the model to policies, giving it time to think about policies at length, and improving security and jailbreak preventions, not only logic. The folks at OpenAI are so proud of all of the above that they have decided to restart the count and call this series o1, but they did mention that they are going to release GPT series models as well, adding to the confusing marketing around their models. Open Source LLMs Reflecting on Reflection 70B Last week, Reflection 70B was supposed to launch live on the ThursdAI show, and while it didn't happen live, I did add it in post editing, and sent the newsletter, and packed my bag, and flew for my vacation. I got many DMs since then, and at some point couldn't resist checking and what I saw was complete chaos, and despite this, I tried to disconnect still until last night. So here's what I could gather since last night. The claims of a llama 3.1 70B finetune that Matt Shumer and Sahil Chaudhary from Glaive beating Sonnet 3.5 are proven false, nobody was able to reproduce those evals they posted and boasted about, which is a damn shame. Not only that, multiple trusted folks from our community, like Kyle Corbitt, Alex Atallah have reached out to Matt in to try to and get to the bottom of how such a thing would happen, and how claims like these could have been made in good faith. (or was there foul play) The core idea of something like Reflection is actually very interesting, but alas, the inability to replicate, but also to stop engaging with he community openly (I've reached out to Matt and given him the opportunity to come to the show and address the topic, he did not reply), keep the model on hugging face where it's still trending, claiming to be the world's number 1 open source model, all these smell really bad, despite multiple efforts on out part to give the benefit of the doubt here. As for my part in building the hype on this (last week's issues till claims that this model is top open source model), I addressed it in the beginning of the show, but then twitter spaces crashed, but unfortunately as much as I'd like to be able to personally check every thing I cover, I often have to rely on the reputation of my sources, which is easier with established big companies, and this time this approached failed me. This weeks Buzzzzzz - One last week till our hackathon! Look at this point, if you read this newsletter and don't know about our hackathon, then I really didn't do my job prompting it, but it's coming up, September 21-22 ! Join us, it's going to be a LOT of fun! 🖼️ Pixtral 12B from Mistral Mistral AI burst onto the scene with Pixtral, their first multimodal model! Devendra Chaplot, research scientist at Mistral, joined ThursdAI to explain their unique approach, ditching fixed image resolutions and training a vision encoder from scratch. "We designed this from the ground up to...get the most value per flop," Devendra explained. Pixtral handles multiple images interleaved with text within a 128k context window - a far cry from the single-image capabilities of most open-source multimodal models. And to make the community erupt in thunderous applause (cue the clap emojis!) they released the 12 billion parameter model under the ultra-permissive Apache 2.0 license. You can give Pixtral a whirl on Hyperbolic, HuggingFace, or directly through Mistral. DeepSeek 2.5: When Intelligence Per Watt is King Deepseek 2.5 launched amid the reflection news and did NOT get the deserved attention it.... deserves. It folded (no deprecated) Deepseek Coder into 2.5 and shows incredible metrics and a truly next-gen architecture. "It's like a higher order MOE", Nisten revealed, "which has this whole like pile of brain and it just like picks every time, from that." 🤯. DeepSeek 2.5 achieves maximum "intelligence per active parameter" Google's turning text into AI podcast for auditory learners with Audio Overviews Today I had the awesome pleasure of chatting with Steven Johnson and Raiza Martin from the NotebookLM team at Google Labs. NotebookLM is a research tool, that if you haven't used, you should definitely give it a spin, and this week they launched something I saw in preview and was looking forward to checking out and honestly was jaw-droppingly impressed today. NotebookLM allows you to upload up to 50 "sources" which can be PDFs, web links that they will scrape for you, documents etc' (no multimodality so far) and will allow you to chat with them, create study guides, dive deeper and add notes as you study. This week's update allows someone who doesn't like reading, to turn all those sources into a legit 5-10 minute podcast, and that sounds so realistic, that I was honestly blown away. I uploaded a documentation of fastHTML in there.. and well hear for yourself The conversation with Steven and Raiza was really fun, podcast definitely give it a listen! Not to mention that Google released (under waitlist) another podcast creating tool called illuminate, that will convert ArXiv papers into similar sounding very realistic 6-10 minute podcasts! ThursdAI - Recaps of the most high signal AI weekly spaces is a reader-supported publication. To receive new posts and support my work, consider becoming a free or paid subscriber. There are many more updates from this week, there was a whole Apple keynote I missed, which had a new point and describe feature with AI on the new iPhones and Apple Intelligence, Google also released new DataGemma 27B, and more things in TL'DR which are posted here in raw format See you next week 🫡 Thank you for being a subscriber, weeks like this are the reason we keep doing this! 🔥 Hope you enjoy these models, leave in comments what you think about them TL;DR in raw format * Open Source LLMs * Reflect on Reflection 70B & Matt Shumer (X, Sahil) * Mixtral releases Pixtral 12B - multimodal model (X, try it) * Pixtral is really good at OCR says swyx * Interview with Devendra Chaplot on ThursdAI * Initial reports of Pixtral beating GPT-4 on WildVision arena from AllenAI * JinaIA reader-lm-0.5b and reader-lm-1.5b (X) * ZeroEval updates * Deepseek 2.5 - * Deepseek coder is now folded into DeepSeek v2.5 * 89 HumanEval (up from 84 from deepseek v2) * 9 on MT-bench * Google - DataGemma 27B (RIG/RAG) for improving results * Retrieval-Interleaved Generation * 🤖 DataGemma: AI models that connect LLMs to Google's Data Commons * 📊 Data Commons: A vast repository of trustworthy public data * 🔍 Tackling AI hallucination by grounding LLMs in real-world data * 🔍 Two approaches: RIG (Retrieval-Interleaved Generation) and RAG (Retrieval-Augmented Generation) * 🔍 Preliminary results show enhanced accuracy and reduced hallucinations * 🔓 Making DataGemma open models to enable broader adoption * 🌍 Empowering informed decisions and deeper understanding of the world * 🔍 Ongoing research to refine the methodologies and scale the work * 🔍 Integrating DataGemma into Gemma and Gemini AI models * 🤝 Collaborating with researchers and developers through quickstart notebooks * Big CO LLMs + APIs * Apple event * Apple Intelligence - launching soon * Visual Intelligence with a dedicated button * Google Illuminate - generate arXiv paper into multiple speaker podcasts (Website) * 5-10 min podcasts * multiple speakers * any paper * waitlist * has samples * sounds super cool * Google NotebookLM is finally available - multi modal research tool + podcast (NotebookLM) * Has RAG like abilities, can add sources from drive or direct web links * Currently not multimodal * Generation of multi speaker conversation about this topic to present it, sounds really really realistic * Chat with Steven and Raiza * OpenAI reveals new o1 models, and launches o1 preview and o1-mini in chat and API (X, Blog) * Trained with RL to think before it speaks with special thinking tokens (that you pay for) * new scaling paradigm * This weeks Buzz * Vision & Video * Adobe announces Firefly video model (X) * Voice & Audio * Hume launches EVI 2 (X) * Fish Speech 1.4 (X) * Instant Voice Cloning * Ultra low latenc * ~1GB model weights * LLaMA-Omni, a new model for speech interaction (X) * Tools * New Jina reader (X) This is a public episode. If you'd like to discuss this with other subscribers or get access to bonus episodes, visit sub.thursdai.news/subscribe | |||
19 Sep 2024 | ThursdAI - Sep 19 - 👑 Qwen 2.5 new OSS king LLM, MSFT new MoE, Nous Research's Forge announcement, and Talking AIs in the open source! | 01:56:06 | |
Hey folks, Alex here, back with another ThursdAI recap – and let me tell you, this week's episode was a whirlwind of open-source goodness, mind-bending inference techniques, and a whole lotta talk about talking AIs! We dove deep into the world of LLMs, from Alibaba's massive Qwen 2.5 drop to the quirky, real-time reactions of Moshi. We even got a sneak peek at Nous Research's ambitious new project, Forge, which promises to unlock some serious LLM potential. So grab your pumpkin spice latte (it's that time again isn't it? 🍁) settle in, and let's recap the AI awesomeness that went down on ThursdAI, September 19th! ThursdAI is brought to you (as always) by Weights & Biases, we still have a few spots left in our Hackathon this weekend and our new advanced RAG course is now released and is FREE to sign up! TL;DR of all topics + show notes and links * Open Source LLMs * Alibaba Qwen 2.5 models drop + Qwen 2.5 Math and Qwen 2.5 Code (X, HF, Blog, Try It) * Qwen 2.5 Coder 1.5B is running on a 4 year old phone (Nisten) * KyutAI open sources Moshi & Mimi (Moshiko & Moshika) - end to end voice chat model (X, HF, Paper) * Microsoft releases GRIN-MoE - tiny (6.6B active) MoE with 79.4 MMLU (X, HF, GIthub) * Nvidia - announces NVLM 1.0 - frontier class multimodal LLMS (no weights yet, X) * Big CO LLMs + APIs * OpenAI O1 results from LMsys do NOT disappoint - vibe checks also confirm, new KING llm in town (Thread) * NousResearch announces Forge in waitlist - their MCTS enabled inference product (X) * This weeks Buzz - everything Weights & Biases related this week * Judgement Day (hackathon) is in 2 days! Still places to come hack with us Sign up * Our new RAG Course is live - learn all about advanced RAG from WandB, Cohere and Weaviate (sign up for free) * Vision & Video * Youtube announces DreamScreen - generative AI image and video in youtube shorts ( Blog) * CogVideoX-5B-I2V - leading open source img2video model (X, HF) * Runway, DreamMachine & Kling all announce text-2-video over API (Runway, DreamMachine) * Runway announces video 2 video model (X) * Tools * Snap announces their XR glasses - have hand tracking and AI features (X) Open Source Explosion! 👑 Qwen 2.5: new king of OSS llm models with 12 model releases, including instruct, math and coder versions This week's open-source highlight was undoubtedly the release of Alibaba's Qwen 2.5 models. We had Justin Lin from the Qwen team join us live to break down this monster drop, which includes a whopping seven different sizes, ranging from a nimble 0.5B parameter model all the way up to a colossal 72B beast! And as if that wasn't enough, they also dropped Qwen 2.5 Coder and Qwen 2.5 Math models, further specializing their LLM arsenal. As Justin mentioned, they heard the community's calls for 14B and 32B models loud and clear – and they delivered! "We do not have enough GPUs to train the models," Justin admitted, "but there are a lot of voices in the community...so we endeavor for it and bring them to you." Talk about listening to your users! Trained on an astronomical 18 trillion tokens (that’s even more than Llama 3.1 at 15T!), Qwen 2.5 shows significant improvements across the board, especially in coding and math. They even open-sourced the previously closed-weight Qwen 2 VL 72B, giving us access to the best open-source vision language models out there. With a 128K context window, these models are ready to tackle some serious tasks. As Nisten exclaimed after putting the 32B model through its paces, "It's really practical…I was dumping in my docs and my code base and then like actually asking questions." It's safe to say that Qwen 2.5 coder is now the best coding LLM that you can use, and just in time for our chat, a new update from ZeroEval confirms, Qwen 2.5 models are the absolute kings of OSS LLMS, beating Mistral large, 4o-mini, Gemini Flash and other huge models with just 72B parameters 👏 Moshi: The Chatty Cathy of AI We've covered Moshi Voice back in July, and they have promised to open source the whole stack, and now finally they did! Including the LLM and the Mimi Audio Encoder! This quirky little 7.6B parameter model is a speech-to-speech marvel, capable of understanding your voice and responding in kind. It's an end-to-end model, meaning it handles the entire speech-to-speech process internally, without relying on separate speech-to-text and text-to-speech models. While it might not be a logic genius, Moshi's real-time reactions are undeniably uncanny. Wolfram Ravenwolf described the experience: "It's uncanny when you don't even realize you finished speaking and it already starts to answer." The speed comes from the integrated architecture and efficient codecs, boasting a theoretical response time of just 160 milliseconds! Moshi uses (also open sourced) Mimi neural audio codec, and achieves 12.5 Hz representation with just 1.1 kbps bandwidth. You can download it and run on your own machine or give it a try here just don't expect a masterful conversationalist hehe Gradient-Informed MoE (GRIN-MoE): A Tiny Titan Just before our live show, Microsoft dropped a paper on GrinMoE, a gradient-informed Mixture of Experts model. We were lucky enough to have the lead author, Liyuan Liu (aka Lucas), join us impromptu to discuss this exciting development. Despite having only 6.6B active parameters (16 x 3.8B experts), GrinMoE manages to achieve remarkable performance, even outperforming larger models like Phi-3 on certain benchmarks. It's a testament to the power of clever architecture and training techniques. Plus, it's open-sourced under the MIT license, making it a valuable resource for the community. NVIDIA NVLM: A Teaser for Now NVIDIA announced NVLM 1.0, their own set of multimodal LLMs, but alas, no weights were released. We’ll have to wait and see how they stack up against the competition once they finally let us get our hands on them. Interestingly, while claiming SOTA on some vision tasks, they haven't actually compared themselves to Qwen 2 VL, which we know is really really good at vision tasks 🤔 Nous Research Unveils Forge: Inference Time Compute Powerhouse (beating o1 at AIME Eval!) Fresh off their NousCon event, Karan and Shannon from Nous Research joined us to discuss their latest project, Forge. Described by Shannon as "Jarvis on the front end," Forge is an inference engine designed to push the limits of what’s possible with existing LLMs. Their secret weapon? Inference-time compute. By implementing sophisticated techniques like Monte Carlo Tree Search (MCTS), Forge can outperform larger models on complex reasoning tasks beating OpenAI's o1-preview at the AIME Eval, competition math benchmark, even with smaller, locally runnable models like Hermes 70B. As Karan emphasized, “We’re actually just scoring with Hermes 3.1, which is available to everyone already...we can scale it up to outperform everything on math, just using a system like this.” Forge isn't just about raw performance, though. It's built with usability and transparency in mind. Unlike OpenAI's 01, which obfuscates its chain of thought reasoning, Forge provides users with a clear visual representation of the model's thought process. "You will still have access in the sidebar to the full chain of thought," Shannon explained, adding, “There’s a little visualizer and it will show you the trajectory through the tree… you’ll be able to see exactly what the model was doing and why the node was selected.” Forge also boasts built-in memory, a graph database, and even code interpreter capabilities, initially supporting Python, making it a powerful platform for building complex LLM applications. Forge is currently in a closed beta, but a waitlist is open for eager users. Karan and Shannon are taking a cautious approach to the rollout, as this is Nous Research’s first foray into hosting a product. For those lucky enough to gain access, Forge offers a tantalizing glimpse into the future of LLM interaction, promising greater transparency, improved reasoning, and more control over the model's behavior. For ThursdAI readers early, here's a waitlist form to test it out! Big Companies and APIs: The Reasoning Revolution OpenAI’s 01: A New Era of LLM Reasoning The big story in the Big Tech world is OpenAI's 01. Since we covered it live last week as it dropped, many of us have been playing with these new reasoning models, and collecting "vibes" from the community. These models represent a major leap in reasoning capabilities, and the results speak for themselves. 01 Preview claimed the top spot across the board on the LMSys Arena leaderboard, demonstrating significant improvements in complex tasks like competition math and coding. Even the smaller 01 Mini showed impressive performance, outshining larger models in certain technical areas. (and the jump in ELO score above the rest in MATH is just incredible to see!) and some folks made this video viral, of a PHD candidate reacting to 01 writing in 1 shot, code that took him a year to write, check it out, it’s priceless. One key aspect of 01 is the concept of “inference-time compute”. As Noam Brown from OpenAI calls it, this represents a "new scaling paradigm", allowing the model to spend more time “thinking” during inference, leading to significantly improved performance on reasoning tasks. The implications of this are vast, opening up the possibility of LLMs tackling long-horizon problems in areas like drug discovery and physics. However, the opacity surrounding 01’s chain of thought reasoning being hidden/obfuscated and the ban on users asking about it was a major point of contention at least within the ThursdAI chat. As Wolfram Ravenwolf put it, "The AI gives you an answer and you can't even ask how it got there. That is the wrong direction." as he was referring to the fact that not only is asking about the reasoning impossible, some folks were actually getting threatening emails and getting banned from using the product all together 😮 This Week's Buzz: Hackathons and RAG Courses! We're almost ready to host our Weights & Biases Judgment Day Hackathon (LLMs as a judge, anyone?) with a few spots left, so if you're reading this and in SF, come hang out with us! And the main thing I gave an update about is our Advanced RAG course, packed with insights from experts at Weights & Biases, Cohere, and Weaviate. Definitely check those out if you want to level up your LLM skills (and it's FREE in our courses academy!) Vision & Video: The Rise of Generative Video Generative video is having its moment, with a flurry of exciting announcements this week. First up, the open-source CogVideoX-5B-I2V, which brings accessible image-to-video capabilities to the masses. It's not perfect, but being able to generate video on your own hardware is a game-changer. On the closed-source front, YouTube announced the integration of generative AI into YouTube Shorts with their DreamScreen feature, bringing AI-powered video generation to a massive audience. We also saw API releases from three leading video model providers: Runway, DreamMachine, and Kling, making it easier than ever to integrate generative video into applications. Runway even unveiled a video-to-video model, offering even more control over the creative process, and it's wild, check out what folks are doing with video-2-video! One last thing here, Kling is adding a motion brush feature to help users guide their video generations, and it just looks so awesome I wanted to show you Whew! That was one hell of a week, tho from the big companies perspective, it was a very slow week, getting a new OSS king, an end to end voice model and a new hint of inference platform from Nous, and having all those folks come to the show was awesome! If you're reading all the way down to here, it seems that you like this content, why not share it with 1 or two friends? 👇 And as always, thank you for reading and subscribing! 🫶 P.S - I’m traveling for the next two weeks, and this week the live show was live recorded from San Francisco, thanks to my dear friends swyx & Alessio for hosting my again in their awesome Latent Space pod studio at Solaris SF! This is a public episode. If you'd like to discuss this with other subscribers or get access to bonus episodes, visit sub.thursdai.news/subscribe | |||
26 Sep 2024 | 📅 ThursdAI - Sep 26 - 🔥 Llama 3.2 multimodal & meta connect recap, new Gemini 002, Advanced Voice mode & more AI news | 01:47:15 | |
Hey everyone, it's Alex (still traveling!), and oh boy, what a week again! Advanced Voice Mode is finally here from OpenAI, Google updated their Gemini models in a huge way and then Meta announced MultiModal LlaMas and on device mini Llamas (and we also got a "better"? multimodal from Allen AI called MOLMO!) From Weights & Biases perspective, our hackathon was a success this weekend, and then I went down to Menlo Park for my first Meta Connect conference, full of news and updates and will do a full recap here as well. ThursdAI - Recaps of the most high signal AI weekly spaces is a reader-supported publication. To receive new posts and support my work, consider becoming a free or paid subscriber. Overall another crazy week in AI, and it seems that everyone is trying to rush something out the door before OpenAI Dev Day next week (which I'll cover as well!) Get ready, folks, because Dev Day is going to be epic! TL;DR of all topics covered: * Open Source LLMs * Meta llama 3.2 Multimodal models (11B & 90B) (X, HF, try free) * Meta Llama 3.2 tiny models 1B & 3B parameters (X, Blog, download) * Allen AI releases MOLMO - open SOTA multimodal AI models (X, Blog, HF, Try It) * Big CO LLMs + APIs * OpenAI releases Advanced Voice Mode to all & Mira Murati leaves OpenAI * Google updates Gemini 1.5-Pro-002 and 1.5-Flash-002 (Blog) * This weeks Buzz * Our free course is LIVE - more than 3000 already started learning how to build advanced RAG++ * Sponsoring tonights AI Tinkerers in Seattle, if you're in Seattle, come through for my demo * Voice & Audio * Meta also launches voice mode (demo) * Tools & Others * Project ORION - holographic glasses are here! (link) Meta gives us new LLaMas and AI hardware LLama 3.2 Multimodal 11B and 90B This was by far the biggest OpenSource release of this week (tho see below, may not be the "best"), as a rumored released finally came out, and Meta has given our Llama eyes! Coming with 2 versions (well 4 if you count the base models which they also released), these new MultiModal LLaMas were trained with an adapter architecture, keeping the underlying text models the same, and placing a vision encoder that was trained and finetuned separately on top. LLama 90B is among the best open-source mutlimodal models available — Meta team at launch These new vision adapters were trained on a massive 6 Billion images, including synthetic data generation by 405B for questions/captions, and finetuned with a subset of 600M high quality image pairs. Unlike the rest of their models, the Meta team did NOT claim SOTA on these models, and the benchmarks are very good but not the best we've seen (Qwen 2 VL from a couple of weeks ago, and MOLMO from today beat it on several benchmarks) With text-only inputs, the Llama 3.2 Vision models are functionally the same as the Llama 3.1 Text models; this allows the Llama 3.2 Vision models to be a drop-in replacement for Llama 3.1 8B/70B with added image understanding capabilities. Seems like these models don't support multi image or video as well (unlike Pixtral for example) nor tool use with images. Meta will also release these models on meta.ai and every other platform, and they cited a crazy 500 million monthly active users of their AI services across all their apps 🤯 which marks them as the leading AI services provider in the world now. Llama 3.2 Lightweight Models (1B/3B) The additional and maybe more exciting thing that we got form Meta was the introduction of the small/lightweight models of 1B and 3B parameters. Trained on up to 9T tokens, and distilled / pruned from larger models, these are aimed for on-device inference (and by device here we mean from laptops to mobiles to soon... glasses? more on this later) In fact, meta released an IOS demo, that runs these models, takes a group chat, summarizes and calls the calendar tool to schedule based on the conversation, and all this happens on device without the info leaving to a larger model. They have also been able to prune down the LLama-guard safety model they released to under 500Mb and have had demos of it running on client side and hiding user input on the fly as the user types something bad! Interestingly, here too, the models were not SOTA, even in small category, with tiny models like Qwen 2.5 3B beating these models on many benchmarks, but they are outlining a new distillation / pruning era for Meta as they aim for these models to run on device, eventually even glasses (and some said Smart Thermostats) In fact they are so tiny, that the communtiy quantized them, released and I was able to download these models, all while the keynote was still going! Here I am running the Llama 3B during the developer keynote! Speaking AI - not only from OpenAI Zuck also showcased a voice based Llama that's coming to Meta AI (unlike OpenAI it's likely a pipeline of TTS/STT) but it worked really fast and Zuck was able to interrupt it. And they also showed a crazy animated AI avatar of a creator, that was fully backed by Llama, while the human creator was on stage, Zuck chatted with his avatar and reaction times were really really impressive. AI Hardware was glasses all along? Look we've all seen the blunders of this year, the Humane AI Ping, the Rabbit R1 (which sits on my desk and I haven't recharged in two months) but maybe Meta is the answer here? Zuck took a bold claim that glasses are actually the perfect form factor for AI, it sits on your face, sees what you see and hears what you hear, and can whisper in your ear without disrupting the connection between you and your conversation partner. They haven't announced new Meta Raybans, but did update the lineup with a new set of transition lenses (to be able to wear those glasses inside and out) and a special edition clear case pair that looks very sleek + new AI features like memories to be able to ask the glasses "hey Meta where did I park" or be able to continue the conversation. I had to get me a pair of this limited edition ones! Project ORION - first holographic glasses And of course, the biggest announcement of the Meta Connect was the super secret decade old project of fully holographic AR glasses, which they called ORION. Zuck introduced these as the most innovative and technologically dense set of glasses in the world. They always said the form factor will become just "glasses" and they actually did it ( a week after Snap spectacles ) tho those are not going to get released to any one any time soon, hell they only made a few thousand of these and they are extremely expensive. With 70 deg FOV, cameras, speakers and a compute puck, these glasses pack a full day battery with under 100grams of weight, and have a custom silicon, custom displays with MicroLED projector and just... tons of more innovation in there. They also come in 3 pieces, the glasses themselves, the compute wireless pack that will hold the LLaMas in your pocket and the EMG wristband that allows you to control these devices using muscle signals. These won't ship as a product tho so don't expect to get them soon, but they are real, and will allow Meta to build the product that we will get on top of these by 2030 AI usecases So what will these glasses be able to do? well, they showed off a live translation feature on stage that mostly worked, where you just talk and listen to another language in near real time, which was great. There are a bunch of mixed reality games, you'd be able to call people and see them in your glasses on a virtual screen and soon you'll show up as an avatar there as well. The AI use-case they showed beyond just translation was MultiModality stuff, where they had a bunch of ingredients for a shake, and you could ask your AI assistant, which shake you can make with what it sees. Do you really need I'm so excited about these to finally come to people I screamed in the audience 👀👓 OpenAI gives everyone* advanced voice mode It's finally here, and if you're paying for chatGPT you know this, the long announced Advanced Voice Mode for chatGPT is now rolled out to all plus members. The new updated since the beta are, 5 new voices (Maple, Spruce, Vale, Arbor and Sol), finally access to custom instructions and memory, so you can ask it to remember things and also to know who you are and your preferences (try saving your jailbreaks there) Unfortunately, as predicted, by the time it rolled out to everyone, this feels way less exciting than it did 6 month ago, the model is way less emotional, refuses to sing (tho folks are making it anyway) and generally feels way less "wow" than what we saw. Less "HER" than we wanted for sure Seriously, they nerfed the singing! Why OpenAI, why? Pro tip of mine that went viral : you can set your action button on the newer iphones to immediately start the voice conversation with 1 click. *This new mode is not available in EU This weeks Buzz - our new advanced RAG++ course is live I had an awesome time with my colleagues Ayush and Bharat today, after they finally released a FREE advanced RAG course they've been working so hard on for the past few months! Definitely check out our conversation, but better yet, why don't you roll into the course? it's FREE and you'll get to learn about data ingestion, evaluation, query enhancement and more! New Gemini 002 is 50% cheaper, 2x faster and better at MMLU-pro It seems that every major lab (besides Anthropic) released a big thing this week to try and get under Meta's skin? Google announced an update to their Gemini Pro/Flash models, called 002, which is a very significant update! Not only are these models 50% cheaper now (Pro price went down by 50% on <128K context lengths), they are 2x faster on outputs with 3x lower latency on first tokens. It's really quite something to see The new models have also improved scores, with the Flash models (the super cheap ones, remember) from September, now coming close to or beating the Pro scores from May 2024! Definitely a worthy update from the team at Google! Hot off the press, the folks at Google Labs also added a feature to the awesome NotebookLM that allows it to summarize over 50h of youtube videos in the crazy high quality Audio Overview feature! ThursdAI - Recaps of the most high signal AI weekly spaces is a reader-supported publication. To receive new posts and support my work, consider becoming a free or paid subscriber. That's it for the week, we of course chatted about way way more during the show, so make sure to listen to the podcast this week, but otherwise, signing off for this week, as I travel back home for a weekend, before returning to SF for the OpenAI dev day next week! Expect full Dev Day coverage live next tuesday and a recap on the newsletter. Meanwhile, if you've already subscribed, please share this newsletter with 1 or two people who are interested in AI 🙇♂️ and see you next week. This is a public episode. If you'd like to discuss this with other subscribers or get access to bonus episodes, visit sub.thursdai.news/subscribe | |||
01 Oct 2024 | OpenAI Dev Day 2024 keynote | 00:05:55 | |
Hey, Alex here. Super quick, as I’m still attending Dev Day, but I didn’t want to leave you hanging (if you're a paid subscriber!), I have decided to outsource my job and give the amazing podcasters of NoteBookLM the whole transcript of the opening keynote of OpenAI Dev Day. You can see a blog of everything they just posted here Here’s a summary of all what was announced: * Developer-Centric Approach: OpenAI consistently emphasized the importance of developers in their mission to build beneficial AGI. The speaker stated, "OpenAI's mission is to build AGI that benefits all of humanity, and developers are critical to that mission... we cannot do this without you." * Reasoning as a New Frontier: The introduction of the GPT-4 series, specifically the "O1" models, marks a significant step towards AI with advanced reasoning capabilities, going beyond the limitations of previous models like GPT-3. * Multimodal Capabilities: OpenAI is expanding the potential of AI applications by introducing multimodal capabilities, particularly focusing on real-time speech-to-speech interaction through the new Realtime API. * Customization and Fine-Tuning: Empowering developers to customize models is a key theme. OpenAI introduced Vision for fine-tuning with images and announced easier access to fine-tuning with model distillation tools. * Accessibility and Scalability: OpenAI demonstrated a commitment to making AI more accessible and cost-effective for developers through initiatives like price reductions, prompt caching, and model distillation tools. Important Ideas and Facts: 1. The O1 Models: * Represent a shift towards AI models with enhanced reasoning capabilities, surpassing previous generations in problem-solving and logical thought processes. * O1 Preview is positioned as the most powerful reasoning model, designed for complex problems requiring extended thought processes. * O1 Mini offers a faster, cheaper, and smaller alternative, particularly suited for tasks like code debugging and agent-based applications. * Both models demonstrate advanced capabilities in coding, math, and scientific reasoning. * OpenAI highlighted the ability of O1 models to work with developers as "thought partners," understanding complex instructions and contributing to the development process. Quote: "The shift to reasoning introduces a new shape of AI capability. The ability for our model to scale and correct the process is pretty mind-blowing. So we are resetting the clock, and we are introducing a new series of models under the name O1." 2. Realtime API: * Enables developers to build real-time AI experiences directly into their applications using WebSockets. * Launches with support for speech-to-speech interaction, leveraging the technology behind ChatGPT's advanced voice models. * Offers natural and seamless integration of voice capabilities, allowing for dynamic and interactive user experiences. * Showcased the potential to revolutionize human-computer interaction across various domains like driving, education, and accessibility. Quote: "You know, a lot of you have been asking about building amazing speech-to-speech experiences right into your apps. Well now, you can." 3. Vision, Fine-Tuning, and Model Distillation: * Vision introduces the ability to use images for fine-tuning, enabling developers to enhance model performance in image understanding tasks. * Fine-tuning with Vision opens up opportunities in diverse fields such as product recommendations, medical imaging, and autonomous driving. * OpenAI emphasized the accessibility of these features, stating that "fine-tuning with Vision is available to every single developer." * Model distillation tools facilitate the creation of smaller, more efficient models by transferring knowledge from larger models like O1 and GPT-4. * This approach addresses cost concerns and makes advanced AI capabilities more accessible for a wider range of applications and developers. Quote: "With distillation, you take the outputs of a large model to supervise, to teach a smaller model. And so today, we are announcing our own model distillation tools." 4. Cost Reduction and Accessibility: * OpenAI highlighted its commitment to lowering the cost of AI models, making them more accessible for diverse use cases. * Announced a 90% decrease in cost per token since the release of GPT-3, emphasizing continuous efforts to improve affordability. * Introduced prompt caching, automatically providing a 50% discount for input tokens the model has recently processed. * These initiatives aim to remove financial barriers and encourage wider adoption of AI technologies across various industries. Quote: "Every time we reduce the price, we see new types of applications, new types of use cases emerge. We're super far from the price equilibrium. In a way, models are still too expensive to be bought at massive scale." Conclusion: OpenAI DevDay conveyed a strong message of developer empowerment and a commitment to pushing the boundaries of AI capabilities. With new models like O1, the introduction of the Realtime API, and a dedicated focus on accessibility and customization, OpenAI is paving the way for a new wave of innovative and impactful AI applications developed by a global community. This is a public episode. If you'd like to discuss this with other subscribers or get access to bonus episodes, visit sub.thursdai.news/subscribe | |||
04 Oct 2024 | 📆 ThursdAI - Oct 3 - OpenAI RealTime API, ChatGPT Canvas & other DevDay news (how I met Sam Altman), Gemini 1.5 8B is basically free, BFL makes FLUX 1.1 6x faster, Rev breaks whisper records... | 01:45:14 | |
Hey, it's Alex. Ok, so mind is officially blown. I was sure this week was going to be wild, but I didn't expect everyone else besides OpenAI to pile on, exactly on ThursdAI. Coming back from Dev Day (number 2) and am still processing, and wanted to actually do a recap by humans, not just the NotebookLM one I posted during the keynote itself (which was awesome and scary in a "will AI replace me as a podcaster" kind of way), and was incredible to have Simon Willison who was sitting just behind me most of Dev Day, join me for the recap! But then the news kept coming, OpenAI released Canvas, which is a whole new way of interacting with chatGPT, BFL released a new Flux version that's 8x faster, Rev released a Whisper killer ASR that does diarizaiton and Google released Gemini 1.5 Flash 8B, and said that with prompt caching (which OpenAI now also has, yay) this will cost a whopping 0.01 / Mtok. That's 1 cent per million tokens, for a multimodal model with 1 million context window. 🤯 This whole week was crazy, as last ThursdAI after finishing the newsletter I went to meet tons of folks at the AI Tinkerers in Seattle, and did a little EvalForge demo (which you can see here) and wanted to share EvalForge with you as well, it's early but very promising so feedback and PRs are welcome! WHAT A WEEK, TL;DR for those who want the links and let's dive in 👇 * OpenAI - Dev Day Recap (Alex, Simon Willison) * Recap of Dev Day * RealTime API launched * Prompt Caching launched * Model Distillation is the new finetune * Finetuning 4o with images (Skalski guide) * Fireside chat Q&A with Sam * Open Source LLMs * NVIDIA finally releases NVML (HF) * This weeks Buzz * Alex discussed his demo of EvalForge at the AI Tinkers event in Seattle in "This Week's Buzz". (Demo, EvalForge, AI TInkerers) * Big Companies & APIs * Google has released Gemini Flash 8B - 0.01 per million tokens cached (X, Blog) * Voice & Audio * Rev breaks SOTA on ASR with Rev ASR and Rev Diarize (Blog, Github, HF) * AI Art & Diffusion & 3D * BFL relases Flux1.1[pro] - 3x-6x faster than 1.0 and higher quality (was 🫐) - (Blog, Try it) The day I met Sam Altman / Dev Day recap Last Dev Day (my coverage here) was a "singular" day in AI for me, given it also had the "keep AI open source" with Nous Research and Grimes, and this Dev Day I was delighted to find out that the vibe was completely different, and focused less on bombastic announcements or models, but on practical dev focused things. This meant that OpenAI cherry picked folks who actively develop with their tools, and they didn't invite traditional media, only folks like yours truly, @swyx from Latent space, Rowan from Rundown, Simon Willison and Dan Shipper, you know, newsletter and podcast folks who actually build! This also allowed for many many OpenAI employees who work on the products and APIs we get to use, were there to receive feedback, help folks with prompting, and just generally interact with the devs, and build that community. I want to shoutout my friends Ilan (who was in the keynote as the strawberry salesman interacting with RealTime API agent), Will DePue from the SORA team, with whom we had an incredible conversation about ethics and legality of projects, Christine McLeavey who runs the Audio team, with whom I shared a video of my daughter crying when chatGPT didn't understand her, Katia, Kevin and Romain on the incredible DevEx/DevRel team and finally, my new buddy Jason who does infra, and was fighting bugs all day and only joined the pub after shipping RealTime to all of us. I've collected all these folks in a convenient and super high signal X list here so definitely give that list a follow if you'd like to tap into their streams For the actual announcements, I've already covered this in my Dev Day post here (which was payed subscribers only, but is now open to all) and Simon did an incredible summary on his Substack as well The highlights were definitely the new RealTime API that let's developers build with Advanced Voice Mode, Prompt Caching that will happen automatically and reduce all your long context API calls by a whopping 50% and finetuning of models that they are rebranding into Distillation and adding new tools to make it easier (including Vision Finetuning for the first time!) Meeting Sam Altman While I didn't get a "media" pass or anything like this, and didn't really get to sit down with OpenAI execs (see Swyx on Latent Space for those conversations), I did have a chance to ask Sam multiple things. First at the closing fireside chat between Sam and Kevin Weil (CPO at OpenAI), Kevin first asked Sam a bunch of questions, and then they gave out the microphones to folks, and I asked the only question that got Sam to smile Sam and Kevin went on for a while, and that Q&A was actually very interesting, so much so, that I had to recruit my favorite Notebook LM podcast hosts, to go through it and give you an overview, so here's that Notebook LM, with the transcript of the whole Q&A (maybe i'll publish it as a standalone episode? LMK in the comments) After the official day was over, there was a reception, at the same gorgeous Fort Mason location, with drinks and light food, and as you might imagine, this was great for networking. But the real post dev day event was hosted by OpenAI devs at a bar, Palm House, which both Sam and Greg Brokman just came to and hung out with folks. I missed Sam last time and was very eager to go and ask him follow up questions this time, when I saw he was just chilling at that bar, talking to devs, as though he didn't "just" complete the largest funding round in VC history ($6.6B at $175B valuation) and went through a lot of drama/turmoil with the departure of a lot of senior leadership! Sam was awesome to briefly chat with, tho as you might imagine, it was loud and tons of folks wanted selfies, but we did discuss how AI affects the real world, job replacement stuff were brought up, and how developers are using the OpenAI products. What we learned, thanks to Sigil, is that o1 was named partly as a "reset" like the main blogpost claimed and partly as "alien of extraordinary ability" , which is the the official designation of the o1 visa, and that Sam came up with this joke himself. Is anyone here smarter than o1? Do you think you still will by o2? One of the highest impact questions was by Sam himself to the audience. Who feels like they've spent a lot of time with O1, and they would say, like, I feel definitively smarter than that thing? — Sam Altman When Sam asked this at first, a few hands hesitatingly went up. He then followed up with Do you think you still will by O2? No one. No one taking the bet.One of the challenges that we face is like, we know how to go do this thing that we think will be like, at least probably smarter than all of us in like a broad array of tasks This was a very palpable moment that folks looked around and realized, what OpenAI folks have probably internalized a long time ago, we're living in INSANE times, and even those of us at the frontier or research, AI use and development, don't necessarily understand or internalize how WILD the upcoming few months, years will be. And then we all promptly forgot to have an existential crisis about it, and took our self driving Waymo's to meet Sam Altman at a bar 😂 This weeks Buzz from Weights & Biases Hey so... after finishing ThursdAI last week I went to Seattle Tinkerers event and gave a demo (and sponsored the event with a raffle of Meta Raybans). I demoed our project called EvalForge, which I built the frontend of and my collegue Anish on backend, as we tried to replicate the Who validates the validators paper by Shreya Shankar, here’s that demo, and EvalForge Github for many of you who asked to see it. Please let me know what you think, I love doing demos and would love feedback and ideas for the next one (coming up in October!) OpenAI chatGPT Canvas - a complete new way to interact with chatGPT Just 2 days after Dev Day, and as breaking news during the show, OpenAI also shipped a new way to interact with chatGPT, called Canvas! Get ready to say goodbye to simple chats and hello to a whole new era of AI collaboration! Canvas, a groundbreaking interface that transforms ChatGPT into a true creative partner for writing and coding projects. Imagine having a tireless copy editor, a brilliant code reviewer, and an endless source of inspiration all rolled into one – that's Canvas! Canvas moves beyond the limitations of a simple chat window, offering a dedicated space where you and ChatGPT can work side-by-side. Canvas opens in a separate window, allowing for a more visual and interactive workflow. You can directly edit text or code within Canvas, highlight sections for specific feedback, and even use a handy menu of shortcuts to request tasks like adjusting the length of your writing, debugging code, or adding final polish. And just like with your favorite design tools, you can easily restore previous versions using the back button. Per Karina, OpenAI has trained a special GPT-4o model specifically for Canvas, enabling it to understand the context of your project and provide more insightful assistance. They used synthetic data, generated by O1 which led them to outperform the basic version of GPT-4o by 30% in accuracy. A general pattern emerges, where new frontiers in intelligence are advancing also older models (and humans as well). Gemini Flash 8B makes intelligence essentially free Google folks were not about to take this week litely and decided to hit back with one of the most insane upgrades to pricing I've seen. The newly announced Gemini Flash 1.5 8B is goint to cost just... $0.01 per million tokens 🤯 (when using caching, 3 cents when not cached) This basically turns intelligence free. And while it is free, it's still their multimodal model (supports images) and has a HUGE context window of 1M tokens. The evals look ridiculous as well, this 8B param model, now almost matches Flash from May of this year, less than 6 month ago, while giving developers 2x the rate limits and lower latency as well. What will you do with free intelligence? What will you do with free intelligence of o1 quality in a year? what about o2 quality in 3 years? Bye Bye whisper? Rev open sources Reverb and Reverb Diarize + turbo models (Blog, HF, Github) With a "WTF just happened" breaking news, a company called Rev.com releases what they consider a SOTA ASR model, that obliterates Whisper (English only for now) on metrics like WER, and includes a specific diarization focused model. Trained on 200,000 hours of English speech, expertly transcribed by humans, which according to their claims, is the largest dataset that any ASR model has been trained on, they achieve some incredible results that blow whisper out of the water (lower WER is better) They also released a seemingly incredible diarization model, which helps understand who speaks when (and is usually added on top of Whisper) For diarization, Rev used the high-performance pyannote.audio library to fine-tune existing models on 26,000 hours of expertly labeled data, significantly improving their performance While this is for English only, getting a SOTA transcription model in the open, is remarkable. Rev opened up this model on HuggingFace with a non commercial license, so folks can play around (and distill?) it, while also making it available in their API for very cheap and also a self hosted solution in a docker container Black Forest Labs feeding up blueberries - new Flux 1.1[pro] is here (Blog, Try It) What is a ThursdAI without multiple SOTA advancements in all fields of AI? In an effort to prove this to be very true, the folks behind FLUX, revealed that the mysterious 🫐 model that was trending on some image comparison leaderboards is in fact a new version of Flux pro, specifically 1.1[pro] FLUX1.1 [pro] provides six times faster generation than its predecessor FLUX.1 [pro] while also improving image quality, prompt adherence, and diversity Just a bit over 2 month since the inital release, and proving that they are THE frontier lab for image diffusion models, folks at BLF are dropping a model that outperforms their previous one on users voting and quality, while being a much faster! They have partnered with Fal, Together, Replicate to disseminate this model (it's not on X quite yet) but are now also offering developers direct access to their own API and at a competitive pricing of just 4 cents per image generation (while being faster AND cheaper AND higher quality than the previous Flux 😮) and you can try it out on Fal here Phew! What a whirlwind! Even I need a moment to catch my breath after that AI news tsunami. But don’t worry, the conversation doesn't end here. I barely scratched the surface of these groundbreaking announcements, so dive into the podcast episode for the full scoop – Simon Willison’s insights on OpenAI’s moves are pure gold, and Maxim LaBonne spills the tea on Liquid AI's audacious plan to dethrone transformers (yes, you read that right). And for those of you who prefer skimming, check out my Dev Day summary (open to all now). As always, hit me up in the comments with your thoughts. What are you most excited about? Are you building anything cool with these new tools? Let's keep the conversation going!Alex This is a public episode. If you'd like to discuss this with other subscribers or get access to bonus episodes, visit sub.thursdai.news/subscribe | |||
10 Oct 2024 | 📆 ThursdAI - Oct 10 - Two Nobel Prizes in AI!? Meta Movie Gen (and sounds ) amazing, Pyramid Flow a 2B video model, 2 new VLMs & more AI news! | 01:30:01 | |
Hey Folks, we are finally due for a "relaxing" week in AI, no more HUGE company announcements (if you don't consider Meta Movie Gen huge), no conferences or dev days, and some time for Open Source projects to shine. (while we all wait for Opus 3.5 to shake things up) This week was very multimodal on the show, we covered 2 new video models, one that's tiny and is open source, and one massive from Meta that is aiming for SORA's crown, and 2 new VLMs, one from our friends at REKA that understands videos and audio, while the other from Rhymes is apache 2 licensed and we had a chat with Kwindla Kramer about OpenAI RealTime API and it's shortcomings and voice AI's in general. ThursdAI - Recaps of the most high signal AI weekly spaces is a reader-supported publication. To receive new posts and support my work, consider becoming a free or paid subscriber. All right, let's TL;DR and show notes, and we'll start with the 2 Nobel prizes in AI 👇 * 2 AI nobel prizes * John Hopfield and Geoffrey Hinton have been awarded a Physics Nobel prize * Demis Hassabis, John Jumper & David Baker, have been awarded this year's #NobelPrize in Chemistry. * Open Source LLMs & VLMs * TxT360: a globally deduplicated dataset for LLM pre-training ( Blog, Dataset) * Rhymes Aria - 25.3B multimodal MoE model that can take image/video inputs Apache 2 (Blog, HF, Try It) * Maitrix and LLM360 launch a new decentralized arena (Leaderboard, Blog) * New Gradio 5 with server side rendering (X) * LLamaFile now comes with a chat interface and syntax highlighting (X) * Big CO LLMs + APIs * OpenAI releases MLEBench - new kaggle focused benchmarks for AI Agents (Paper, Github) * Inflection is still alive - going for enterprise lol (Blog) * new Reka Flash 21B - (X, Blog, Try It) * This weeks Buzz * We chatted about Cursor, it went viral, there are many tips * WandB releases HEMM - benchmarks of text-to-image generation models (X, Github, Leaderboard) * Vision & Video * Meta presents Movie Gen 30B - img and text to video models (blog, paper) * Pyramid Flow - open source img2video model MIT license (X, Blog, HF, Paper, Github) * Voice & Audio * Working with OpenAI RealTime Audio - Alex conversation with Kwindla from trydaily.com * Cartesia Sonic goes multilingual (X) * Voice hackathon in SF with 20K prizes (and a remote track) - sign up * Tools * LM Studio ships with MLX natively (X, Download) * UITHUB.com - turn any github repo into 1 long file for LLMs A Historic Week: TWO AI Nobel Prizes! This week wasn't just big; it was HISTORIC. As Yam put it, "two Nobel prizes for AI in a single week. It's historic." And he's absolutely spot on! Geoffrey Hinton, often called the "grandfather of modern AI," alongside John Hopfield, were awarded the Nobel Prize in Physics for their foundational work on neural networks - work that paved the way for everything we're seeing today. Think back propagation, Boltzmann machines – these are concepts that underpin much of modern deep learning. It’s about time they got the recognition they deserve! Yoshua Bengio posted about this in a very nice quote: @HopfieldJohn and @geoffreyhinton, along with collaborators, have created a beautiful and insightful bridge between physics and AI. They invented neural networks that were not only inspired by the brain, but also by central notions in physics such as energy, temperature, system dynamics, energy barriers, the role of randomness and noise, connecting the local properties, e.g., of atoms or neurons, to global ones like entropy and attractors. And they went beyond the physics to show how these ideas could give rise to memory, learning and generative models; concepts which are still at the forefront of modern AI research And Hinton's post-Nobel quote? Pure gold: “I’m particularly proud of the fact that one of my students fired Sam Altman." He went on to explain his concerns about OpenAI's apparent shift in focus from safety to profits. Spicy take! It sparked quite a conversation about the ethical implications of AI development and who’s responsible for ensuring its safe deployment. It’s a discussion we need to be having more and more as the technology evolves. Can you guess which one of his students it was? Then, not to be outdone, the AlphaFold team (Demis Hassabis, John Jumper, and David Baker) snagged the Nobel Prize in Chemistry for AlphaFold 2. This AI revolutionized protein folding, accelerating drug discovery and biomedical research in a way no one thought possible. These awards highlight the tangible, real-world applications of AI. It's not just theoretical anymore; it's transforming industries. Congratulations to all winners, and we gotta wonder, is this a start of a trend of AI that takes over every Nobel prize going forward? 🤔 Open Source LLMs & VLMs: The Community is COOKING! The open-source AI community consistently punches above its weight, and this week was no exception. We saw some truly impressive releases that deserve a standing ovation. First off, the TxT360 dataset (blog, dataset). Nisten, resident technical expert, broke down the immense effort: "The amount of DevOps and…operations to do this work is pretty rough." This globally deduplicated 15+ trillion-token corpus combines the best of Common Crawl with a curated selection of high-quality sources, setting a new standard for open-source LLM training. We talked about the importance of deduplication for model training - avoiding the "memorization" of repeated information that can skew a model's understanding of language. TxT360 takes a 360-degree approach to data quality and documentation – a huge win for accessibility. Apache 2 Multimodal MoE from Rhymes AI called Aria (blog, HF, Try It ) Next, the Rhymes Aria model (25.3B total and only 3.9B active parameters!) This multimodal marvel operates as a Mixture of Experts (MoE), meaning it activates only the necessary parts of its vast network for a given task, making it surprisingly efficient. Aria excels in understanding image and video inputs, features a generous 64K token context window, and is available under the Apache 2 license – music to open-source developers’ ears! We even discussed its coding capabilities: imagine pasting images of code and getting intelligent responses. I particularly love the focus on long multimodal input understanding (think longer videos) and super high resolution image support. I uploaded this simple pin-out diagram of RaspberriPy and it got all the right answers correct! Including ones I missed myself (and won against Gemini 002 and the new Reka Flash!) Big Companies and APIs OpenAI new Agentic benchmark, can it compete with MLEs on Kaggle? OpenAI snuck in a new benchmark, MLEBench (Paper, Github), specifically designed to evaluate AI agents performance on Machine Learning Engineering tasks. Designed around a curated collection of Kaggle competitions, creating a diverse set of challenging tasks that test real-world ML engineering skills such as training models, preparing datasets, and running experiments. They found that the best-performing setup--OpenAI's o1-preview with AIDE scaffolding--achieves at least the level of a Kaggle bronze medal in 16.9% of competitions (though there are some that throw shade on this score) Meta comes for our reality with Movie Gen But let's be honest, Meta stole the show this week with Movie Gen (blog). This isn’t your average video generation model; it’s like something straight out of science fiction. Imagine creating long, high-definition videos, with different aspect ratios, personalized elements, and accompanying audio – all from text and image prompts. It's like the Holodeck is finally within reach! Unfortunately, despite hinting at its size (30B) Meta is not releasing this model (just yet) nor is it available widely so far! But we'll keep our fingers crossed that it drops before SORA. One super notable thing is, this model generates audio as well to accompany the video and it's quite remarkable. We listened to a few examples from Meta’s demo, and the sound effects were truly remarkable – everything from fireworks to rustling leaves. This model isn't just creating video, it's crafting experiences. (Sound on for the next example!) They also have personalization built in, which is showcased here by one of the leads of LLama ,Roshan, as a scientist doing experiments and the realism is quite awesome to see (but I get why they are afraid of releasing this in open weights) This Week’s Buzz: What I learned at Weights & Biases this week My "buzz" this week was less about groundbreaking models and more about mastering the AI tools we have. We had a team meeting to share our best tips and tricks for using Cursor, and when I shared those insights on X (thread), they went surprisingly viral! The big takeaway from the thread? Composer, Cursor’s latest feature, is a true game-changer. It allows for more complex refactoring and code generation across multiple files – the kind of stuff that would take hours manually. If you haven't tried Composer, you're seriously missing out. We also covered strategies for leveraging different models for specific tasks, like using O1 mini for outlining and then switching to the more robust Cloud 3.5 for generating code. Another gem we uncovered: selecting any text in the console and hitting opt+D will immediately send it to the chat to debug, super useful! Over at Weights & Biases, my talented teammate, Soumik, released HEMM (X, Github), a comprehensive benchmark specifically designed for text-to-image generation models. Want to know how different models fare on image quality and prompt comprehension? Head over to the leaderboard on Weave (Leaderboard) and find out! And yes, it's true, Weave, our LLM observability tool, is multimodal (well within the theme of today's update) Voice and Audio: Real-Time Conversations and the Quest for Affordable AI OpenAI's DevDay was just a few weeks back, but the ripple effects of their announcements are still being felt. The big one for voice AI enthusiasts like myself? The RealTime API, offering developers a direct line to Advanced Voice Mode. My initial reaction was pure elation – finally, a chance to build some seriously interactive voice experiences that sound incredible and in near real time! That feeling was quickly followed by a sharp intake of breath when I saw the price tag. As I discovered building my Halloween project, real-time streaming of this caliber isn’t exactly budget-friendly (yet!). Kwindla from trydaily.com, a voice AI expert, joined the show to shed some light on this issue. We talked about the challenges of scaling these models and the complexities of context management in real-time audio processing. The conversation shifted to how OpenAI's RealTime API isn’t just about the model itself but also the innovative way they're managing the user experience and state within a conversation. He pointed out, however, that what we see and hear from the API isn’t exactly what’s going on under the hood, “What the model hears and what the transcription events give you back are not the same”. Turns out, OpenAI relies on Whisper for generating text transcriptions – it’s not directly from the voice model. The pricing really threw me though, only testing a little bit, not even doing anything on production, and OpenAI charged almost 10$, the same conversations are happening across Reddit and OpenAI forums as well. Hallo-Weave project update: So as I let folks know on the show, I'm building a halloween AI decoration as a project, and integrating it into Weights & Biases Weave (that's why it's called HalloWeave) After performing brain surgery, futzing with wires and LEDs, I finally have it set up so it wakes up on a trigger word (it's "Trick or Treat!"), takes a picture with the webcam (actual webcam, raspberryPi camera was god awful) and sends it to Gemini Flash to detect which costume this is and write a nice customized greeting. Then I send that text to Cartesia to generate the speech using a British voice, and then I play it via a bluetooth speaker. Here's a video of the last stage (which still had some bluetooth issues, it's a bit better now) Next up: I should decide if I care to integrate OpenAI Real time (and pay a LOT of $$$ for it) or fallback to existing LLM - TTS services and let kids actually have a conversation with the toy! Stay tuned for more updates as we get closer to halloween, the project is open source HERE and the Weave dashboard will be open once it's live. ThursdAI - Recaps of the most high signal AI weekly spaces is a reader-supported publication. To receive new posts and support my work, consider becoming a free or paid subscriber. One More Thing… UIThub! Before signing off, one super useful tool for you! It's so useful I recorded (and created an edit) video on it. I've also posted it on my brand new TikTok, Instagram, Youtube and Linkedin accounts, where it promptly did not receive any views, but hey, gotta start somewhere right? 😂 Phew! That’s a wrap for this week’s ThursdAI. From Nobel Prizes to new open-source tools, and even meta's incredibly promising (but still locked down) video gen models, the world of AI continues to surprise and delight (and maybe cause a mild existential crisis or two!). I'd love to hear your thoughts – what caught your eye? Are you building anything cool? Let me know in the comments, and I'll see you back here next week for more AI adventures! Oh, and don't forget to subscribe to the podcast (five-star ratings always appreciated 😉). This is a public episode. If you'd like to discuss this with other subscribers or get access to bonus episodes, visit sub.thursdai.news/subscribe | |||
18 Oct 2024 | 📆 ThursdAI - Oct 17 - Robots, Rockets, and Multi Modal Mania with open source voice cloning, OpenAI new voice API and more AI news | 01:35:10 | |
Hey folks, Alex here from Weights & Biases, and this week has been absolutely bonkers. From robots walking among us to rockets landing on chopsticks (well, almost), the future is feeling palpably closer. And if real-world robots and reusable spaceship boosters weren't enough, the open-source AI community has been cooking, dropping new models and techniques faster than a Starship launch. So buckle up, grab your space helmet and noise-canceling headphones (we’ll get to why those are important!), and let's blast off into this week’s AI adventures! TL;DR and show-notes + links at the end of the post 👇 Robots and Rockets: A Glimpse into the Future I gotta start with the real-world stuff because, let's be honest, it's mind-blowing. We had Robert Scoble (yes, the Robert Scoble) join us after attending the Tesla We, Robot AI event, reporting on Optimus robots strolling through crowds, serving drinks, and generally being ridiculously futuristic. Autonomous robo-taxis were also cruising around, giving us a taste of a driverless future. Robert’s enthusiasm was infectious: "It was a vision of the future, and from that standpoint, it succeeded wonderfully." I couldn't agree more. While the market might have had a mini-meltdown (apparently investors aren't ready for robot butlers yet), the sheer audacity of Tesla’s vision is exhilarating. These robots aren't just cool gadgets; they represent a fundamental shift in how we interact with technology and the world around us. And they’re learning fast. Just days after the event, Tesla released a video of Optimus operating autonomously, showcasing the rapid progress they’re making. And speaking of audacious visions, SpaceX decided to one-up everyone (including themselves) by launching Starship and catching the booster with Mechazilla – their giant robotic chopsticks (okay, technically a launch tower, but you get the picture). Waking up early with my daughter to watch this live was pure magic. As Ryan Carson put it, "It was magical watching this… my kid who's 16… all of his friends are getting their imaginations lit by this experience." That’s exactly what we need - more imagination and less doomerism! The future is coming whether we like it or not, and I, for one, am excited. Open Source LLMs and Tools: The Community Delivers (Again!) Okay, back to the virtual world (for now). This week's open-source scene was electric, with new model releases and tools that have everyone buzzing (and benchmarking like crazy!). * Nemotron 70B: Hype vs. Reality: NVIDIA dropped their Nemotron 70B instruct model, claiming impressive scores on certain benchmarks (Arena Hard, AlpacaEval), even suggesting it outperforms GPT-4 and Claude 3.5. As always, we take these claims with a grain of salt (remember Reflection?), and our resident expert, Nisten, was quick to run his own tests. The verdict? Nemotron is good, "a pretty good model to use," but maybe not the giant-killer some hyped it up to be. Still, kudos to NVIDIA for pushing the open-source boundaries. (Hugging Face, Harrison Kingsley evals) * Zamba 2 : Hybrid Vigor: Zyphra, in collaboration with NVIDIA, released Zamba 2, a hybrid Sparse Mixture of Experts (SME) model. We had Paolo Glorioso, a researcher from Ziphra, join us to break down this unique architecture, which combines the strengths of transformers and state space models (SSMs). He highlighted the memory and latency advantages of SSMs, especially for on-device applications. Definitely worth checking out if you’re interested in transformer alternatives and efficient inference. * Zyda 2: Data is King (and Queen): Alongside Zamba 2, Zyphra also dropped Zyda 2, a massive 5 trillion token dataset, filtered, deduplicated, and ready for LLM training. This kind of open-source data release is a huge boon to the community, fueling the next generation of models. (X) * Ministral: Pocket-Sized Power: On the one-year anniversary of the iconic Mistral 7B release, Mistral announced two new smaller models – Ministral 3B and 8B. Designed for on-device inference, these models are impressive, but as always, Qwen looms large. While Mistral didn’t include Qwen in their comparisons, early tests suggest Qwen’s smaller models still hold their own. One point of contention: these Ministrals aren't as open-source as the original 7B, which is a bit of a bummer, with the 3B not being even released anywhere besides their platform. (Mistral Blog) * Entropix (aka Shrek Sampler): Thinking Outside the (Sample) Box: This one is intriguing! Entropix introduces a novel sampling technique aimed at boosting the reasoning capabilities of smaller LLMs. Nisten’s yogurt analogy explains it best: it’s about “marinating” the information and picking the best “flavor” (token) at the end. Early examples look promising, suggesting Entropix could help smaller models tackle problems that even trip up their larger counterparts. But, as with all shiny new AI toys, we're eagerly awaiting robust evals. Tim Kellog has an detailed breakdown of this method here * Gemma-APS: Fact-Finding Mission: Google released Gemma-APS, a set of models specifically designed for extracting claims and facts from text. While LLMs can already do this to some extent, a dedicated model for this task is definitely interesting, especially for applications requiring precise information retrieval. (HF) 🔥 OpenAI adds voice to their completion API (X, Docs) In the last second of the pod, OpenAI decided to grace us with Breaking News! Not only did they launch their Windows native app, but also added voice input and output to their completion APIs. This seems to be the same model as the advanced voice mode (and priced super expensively as well) and the one they used in RealTime API released a few weeks ago at DevDay. This is of course a bit slower than RealTime but is much simpler to use, and gives way more developers access to this incredible resource (I'm definitely planning to use this for ... things 😈) This isn't their "TTS" or "STT (whisper) models, no, this is an actual omni model that understands audio natively and also outputs audio natively, allowing for things like "count to 10 super slow" I've played with it just now (and now it's after 6pm and I'm still writing this newsletter) and it's so so awesome, I expect it to be huge because the RealTime API is very curbersome and many people don't really need this complexity. This weeks Buzz - Weights & Biases updates Ok I wanted to send a completely different update, but what I will show you is, Weave, our observability framework is now also Multi Modal! This couples very well with the new update from OpenAI! So here's an example usage with today's announcement, I'm going to go through the OpenAI example and show you how to use it with streaming so you can get the audio faster, and show you the Weave multimodality as well 👇 You can find the code for this in this Gist and please give us feedback as this is brand new Non standard use-cases of AI corner This week I started noticing and collecting some incredible use-cases of Gemini and it's long context and multimodality and wanted to share with you guys, so we had some incredible conversations about non-standard use cases that are pushing the boundaries of what's possible with LLMs. Hrishi blew me away with his experiments using Gemini for transcription and diarization. Turns out, Gemini is not only great at transcription (it beats whisper!), it’s also ridiculously cheaper than dedicated ASR models like Whisper, around 60x cheaper! He emphasized the unexplored potential of prompting multimodal models, adding, “the prompting on these things… is still poorly understood." So much room for innovation here! Simon Willison then stole the show with his mind-bending screen-scraping technique. He recorded a video of himself clicking through emails, fed it to Gemini Flash, and got perfect structured data in return. This trick isn’t just clever; it’s practically free, thanks to the ridiculously low cost of Gemini Flash. I even tried it myself, recording my X bookmarks and getting a near-perfect TLDR of the week’s AI news. The future of data extraction is here, and it involves screen recordings and very cheap (or free) LLMs. Here's Simon's example of how much this would cost him had he actually be charged for it. 🤯 Speaking of Simon Willison , he broke the news that NotebookLM has got an upgrade, with the ability to steer the speakers with custom commands, which Simon promptly used to ask the overview hosts to talk like Pelicans Voice Cloning, Adobe Magic, and the Quest for Real-Time Avatars Voice cloning also took center stage this week, with the release of F5-TTS. This open-source model performs zero-shot voice cloning with just a few seconds of audio, raising all sorts of ethical questions (and exciting possibilities!). I played a sample on the show, and it was surprisingly convincing (though not without it's problems) for a local model! This, combined with Hallo 2's (also released this week!) ability to animate talking avatars, has Wolfram Ravenwolf dreaming of real-time AI assistants with personalized faces and voices. The pieces are falling into place, folks. And for all you Adobe fans, Firefly Video has landed! This “commercially safe” text-to-video and image-to-video model is seamlessly integrated into Premiere, offering incredible features like extending video clips with AI-generated frames. Photoshop also got some Firefly love, with mind-bending relighting capabilities that could make AI-generated images indistinguishable from real photographs. Wrapping Up: Phew, that was a marathon, not a sprint! From robots to rockets, open source to proprietary, and voice cloning to video editing, this week has been a wild ride through the ever-evolving landscape of AI. Thanks for joining me on this adventure, and as always, keep exploring, keep building, and keep pushing those AI boundaries. The future is coming, and it’s going to be amazing. P.S. Don’t forget to subscribe to the podcast and newsletter for more AI goodness, and if you’re in Seattle next week, come say hi at the AI Tinkerers meetup. I’ll be demoing my Halloween AI toy – it’s gonna be spooky! ThursdAI - Recaps of the most high signal AI weekly spaces is a reader-supported publication. To receive new posts and support my work, consider becoming a free or paid subscriber. TL;DR - Show Notes and Links * Open Source LLMs * Nvidia releases Llama 3.1-Nemotron-70B instruct: Outperforms GPT-40 and Anthropic Claude 3.5 on several benchmarks. Available on Hugging Face and Nvidia. (X, Harrison Eval) * Zamba2-7B: A hybrid Sparse Mixture of Experts model from Zyphra and Nvidia. Claims to outperform Mistral, Llama2, and Gemmas in the 58B weight class. (X, HF) * Zyda-2: 57B token dataset distilled from high-quality sources for training LLMs. Released by Zyphra and Nvidia. (X) * Ministral 3B & 8B - Mistral releases 2 new models for on device, claims SOTA (Blog) • Entropix aims to mimic advanced reasoning in small LLMs (Github, Breakdown) * Google releases Gemma-APS: A collection of Gemma models for text-to-propositions segmentation, distilled from Gemini Pro and fine-tuned on synthetic data. (HF) * Big CO LLMs + APIs * OpenAI ships advanced voice model in chat completions API endpoints with multimodality (X, Docs, My Example) * Amazon, Microsoft, Google all announce nuclear power for AI future * Yi-01.AI launches Yi-Lightning: A proprietary model accessible via API. * New Gemini API parameters: Google has shipped new Gemini API parameters, including logprobs, candidateCount, presencePenalty, seed, frequencyPenalty, and model_personality_in_response. * Google NotebookLM is no longer "experimental" and now allows for "steering" the hosts (Announcement) * XAI - GROK 2 and Grok2-mini are now available via API in OpenRouter - (X, OR) * This weeks Buzz (What I learned with WandB this week) * Weave is now MultiModal (supports audio and text!) (X, Github Example) * Vision & Video * Adobe Firefly Video: Adobe's first commercially safe text-to-video and image-to-video generation model. Supports prompt coherence. (X) * Voice & Audio * Ichigo-Llama3.1 Local Real-Time Voice AI: Improvements allow it to talk back, recognize when it can't comprehend input, and run on a single Nvidia 3090 GPU. (X) * F5-TTS: Performs zero-shot voice cloning with less than 15 seconds of audio, using audio clips to generate additional audio. (HF, Paper) * AI Art & Diffusion & 3D * RF-Inversion: Zero-shot inversion and editing framework for Flux, introduced by Litu Rout. Allows for image editing and personalization without training, optimization, or prompt-tuning. (X) * Tools * Fastdata: A library for synthesizing 1B tokens. (X) This is a public episode. If you'd like to discuss this with other subscribers or get access to bonus episodes, visit sub.thursdai.news/subscribe | |||
25 Oct 2024 | 📅 ThursdAI - Oct 24 - Claude 3.5 controls your PC?! Talking AIs with 🦾, Multimodal Weave, Video Models mania + more AI news from this 🔥 week. | 01:56:20 | |
Hey all, Alex here, coming to you from the (surprisingly) sunny Seattle, with just a mind-boggling week of releases. Really, just on Tuesday there was so much news already! I had to post a recap thread, something I do usually after I finish ThursdAI! From Anthropic reclaiming close-second sometimes-first AI lab position + giving Claude the wheel in the form of computer use powers, to more than 3 AI video generation updates with open source ones, to Apple updating Apple Intelligence beta, it's honestly been very hard to keep up, and again, this is literally part of my job! But once again I'm glad that we were able to cover this in ~2hrs, including multiple interviews with returning co-hosts ( Simon Willison came back, Killian came back) so definitely if you're only a reader at this point, listen to the show! Ok as always (recently) the TL;DR and show notes at the bottom (I'm trying to get you to scroll through ha, is it working?) so grab a bucket of popcorn, let's dive in 👇 ThursdAI - Recaps of the most high signal AI weekly spaces is a reader-supported publication. To receive new posts and support my work, consider becoming a free or paid subscriber. Claude's Big Week: Computer Control, Code Wizardry, and the Mysterious Case of the Missing Opus Anthropic dominated the headlines this week with a flurry of updates and announcements. Let's start with the new Claude Sonnet 3.5 (really, they didn't update the version number, it's still 3.5 tho a different API model) Claude Sonnet 3.5: Coding Prodigy or Benchmark Buster? The new Sonnet model shows impressive results on coding benchmarks, surpassing even OpenAI's O1 preview on some. "It absolutely crushes coding benchmarks like Aider and Swe-bench verified," I exclaimed on the show. But a closer look reveals a more nuanced picture. Mixed results on other benchmarks indicate that Sonnet 3.5 might not be the universal champion some anticipated. My friend who has held back internal benchmarks was disappointed highlighting weaknesses in scientific reasoning and certain writing tasks. Some folks are seeing it being lazy-er for some full code completion, while the context window is now doubled from 4K to 8K! This goes to show again, that benchmarks don't tell the full story, so we wait for LMArena (formerly LMSys Arena) and the vibe checks from across the community. However it absolutely dominates in code tasks, that much is clear already. This is a screenshot of the new model on Aider code editing benchmark, a fairly reliable way to judge models code output, they also have a code refactoring benchmark Haiku 3.5 and the Vanishing Opus: Anthropic's Cryptic Clues Further adding to the intrigue, Anthropic announced Claude 3.5 Haiku! They usually provide immediate access, but Haiku remains elusive, saying that it's available by end of the month, which is very very soon. Making things even more curious, their highly anticipated Opus model has seemingly vanished from their website. "They've gone completely silent on 3.5 Opus," Simon Willison (𝕏) noted, mentioning conspiracy theories that this new Sonnet might simply be a rebranded Opus? 🕯️ 🕯️ We'll make a summoning circle for new Opus and update you once it lands (maybe next year) Claude Takes Control (Sort Of): Computer Use API and the Dawn of AI Agents (𝕏) The biggest bombshell this week? Anthropic's Computer Use. This isn't just about executing code; it’s about Claude interacting with computers, clicking buttons, browsing the web, and yes, even ordering pizza! Killian Lukas (𝕏), creator of Open Interpreter, returned to ThursdAI to discuss this groundbreaking development. "This stuff of computer use…it’s the same argument for having humanoid robots, the web is human shaped, and we need AIs to interact with computers and the web the way humans do" Killian explained, illuminating the potential for bridging the digital and physical worlds. Simon, though enthusiastic, provided a dose of realism: "It's incredibly impressive…but also very much a V1, beta.” Having tackled the setup myself, I agree; the current reliance on a local Docker container and virtual machine introduces some complexity and security considerations. However, seeing Claude fix its own Docker installation error was an unforgettably mindblowing experience. The future of AI agents is upon us, even if it’s still a bit rough around the edges. Here's an easy guide to set it up yourself, takes 5 minutes, requires no coding skills and it's safely tucked away in a container. Big Tech's AI Moves: Apple Embraces ChatGPT, X.ai API (+Vision!?), and Cohere Multimodal Embeddings The rest of the AI world wasn’t standing still. Apple made a surprising integration, while X.ai and Cohere pushed their platforms forward. Apple iOS 18.2 Beta: Siri Phones a Friend (ChatGPT) Apple, always cautious, surprisingly integrated ChatGPT directly into iOS. While Siri remains…well, Siri, users can now effortlessly offload more demanding tasks to ChatGPT. "Siri is still stupid," I joked, "but can now ask it to write some stuff and it'll tell you, hey, do you want me to ask my much smarter friend ChatGPT about this task?" This approach acknowledges Siri's limitations while harnessing ChatGPT’s power. The iOS 18.2 beta also includes GenMoji (custom emojis!) and Visual Intelligence (multimodal camera search) which are both welcome, tho I didn't really get the need of the Visual Intelligence (maybe I'm jaded with my Meta Raybans that already have this and are on my face most of the time) and I didn't get into the GenMoji waitlist still waiting to show you some custom emojis! X.ai API: Grok's Enterprise Ambitions and a Secret Vision Model Elon Musk's X.ai unveiled their API platform, focusing on enterprise applications with Grok 2 beta. They also teased an undisclosed vision model, and they had vision APIs for some folks who joined their hackathon. While these models are still not worth using necessarily, the next Grok-3 is promising to be a frontier model, and for some folks, it's relaxed approach to content moderation (what Elon is calling maximally seeking the truth) is going to be a convincing point for some! I just wish they added fun mode and access to real time data from X! Right now it's just the Grok-2 model, priced at a very non competative $15/mTok 😒 Cohere Embed 3: Elevating Multimodal Embeddings (Blog) Cohere launched Embed 3, enabling embeddings for both text and visuals such as graphs and designs. "While not the first multimodal embeddings, when it comes from Cohere, you know it's done right," I commented. Open Source Power: JavaScript Transformers and SOTA Multilingual Models The open-source AI community continues to impress, making powerful models accessible to all. Massive kudos to Xenova (𝕏) for the release of Transformers.js v3! The addition of WebGPU support results in a staggering "up to 100 times faster" performance boost for browser-based AI, dramatically simplifying local, private, and efficient model running. We also saw DeepSeek’s Janus 1.3B, a multimodal image and text model, and Cohere For AI's Aya Expanse, supporting 23 languages. This Week’s Buzz: Hackathon Triumphs and Multimodal Weave On ThursdAI, we also like to share some of the exciting things happening behind the scenes. AI Chef Showdown: Second Place and Lessons Learned Happy to report that team Yes Chef clinched second place in a hackathon with an unconventional creation: a Gordon Ramsay-inspired robotic chef hand puppet, complete with a cloned voice and visual LLM integration. We bought and 3D printed and assembled an Open Source robotic arm, made it become a ventriloquist operator by letting it animate a hand puppet, and cloned Ramsey's voice. It was so so much fun to build, and the code is here Weave Goes Multimodal: Seeing and Hearing Your AI Even more exciting was the opportunity to leverage Weave's newly launched multimodal functionality. "Weave supports you to see and play back everything that's audio generated," I shared, emphasizing its usefulness in debugging our vocal AI chef. For a practical example, here's ALL the (NSFW) roasts that AI Chef has cooked me with, it's honestly horrifying haha. For full effect, turn on the background music first and then play the chef audio 😂 📽️ Video Generation Takes Center Stage: Mochi's Motion Magic and Runway's Acting Breakthrough Video models made a quantum leap this week, pushing the boundaries of generative AI. Genmo Mochi-1: Diffusion Transformers and Generative Motion Genmo's Ajay Jain (Genmo) joined ThursdAI to discuss Mochi-1, their powerful new diffusion transformer. "We really focused on…prompt adherence and motion," he explained. Mochi-1's capacity to generate complex and realistic motion is truly remarkable, and with an HD version on its way, the future looks bright (and animated!). They also get bonus points for dropping a torrent link in the announcement tweet. So far this apache 2, 10B Diffusion Transformer is open source, but not for the GPU-poors, as it requires 4 GPUs to run, but apparently there was already an attempt to run in on one single 4090 which, Ajay highlighted was one of the reasons they open sourced it! Runway Act-One: AI-Powered Puppetry and the Future of Acting (blog) Ok this one absolutely seems bonkers! Runway unveiled Act-One! Forget just generating video from text; Act-One takes a driving video and character image to produce expressive and nuanced character performances. "It faithfully represents elements like eye-lines, micro expressions, pacing, and delivery," I noted, excited by the transformative potential for animation and filmmaking. So no need for rigging, for motion capture suites on faces of actors, Runway now, does this, so you can generate characters with Flux, and animate them with Act-One 📽️ Just take a look at this insanity 👇 11labs Creative Voices: Prompting Your Way to the Perfect Voice 11labs debuted an incredible feature: creating custom voices using only text prompts. Want a high-pitched squeak or a sophisticated British accent? Just ask. This feature makes bespoke voice creation significantly easier. I was really really impressed by this, as this is perfect for my Skeleton Halloween project! So far I struggled to get the voice "just right" between the awesome Cartesia voice that is not emotional enough, and the very awesome custom OpenAI voice that needs a prompt to act, and sometimes stops acting in the middle of a sentence. With this new Elevenlabs feature, I can describe the exact voice I want with a prompt, and then keep iterating until I find the perfect one, and then boom, it's available for me! Great for character creation, and even greater for the above Act-One model, as you can now generate a character with Flux, Drive the video with Act-one and revoice yourself with a custom prompted voice from 11labs! Which is exactly what I'm going to build for the next hackathon! If you'd like to support me in this journey, here's an 11labs affiliate link haha but I already got a yearly account so don't sweat it. AI Art & Diffusion Updates: Stable Diffusion 3.5, Ideogram Canvas, and OpenAI's Sampler Surprise The realm of AI art and diffusion models saw its share of action as well. Stable Diffusion 3.5 (Blog) and Ideogram Canvas: Iterative Improvements and Creative Control Stability AI launched Stable Diffusion 3.5, bringing incremental enhancements to image quality and prompt accuracy. Ideogram, meanwhile, introduced Canvas, a groundbreaking interface enabling mixing, matching, extending, and fine-tuning AI-generated artwork. This opens doors to unprecedented levels of control and creative expression. Midjourney also announced a web editor, and folks are freaking out, and I'm only left thinking, is MJ a bit a cult? There are so much offerings out there, but it seems like everything MJ releases gets tons more excitement from that part of X than other way more incredible stuff 🤔 Seattle Pic Ok wow that was a LOT of stuff to cover, honestly, the TL;DR for this week became so massive that I had to zoom out to take 1 screenshot of it all ,and I wasn't sure we'd be able to cover all of it! Massive massive week, super exciting releases, and the worst thing about this is, I barely have time to play with many of these! But I'm hoping to have some time during the Tinkerer AI hackathon we're hosting on Nov 2-3 in our SF office, limited spots left, so come and hang with me and some of the Tinkerers team, and maybe even win a Meta Rayban special Weave prize! RAW TL;DR + Show notes and links * Open Source LLMs * Xenova releases Transformers JS version 3 (X) * ⚡ WebGPU support (up to 100x faster than WASM)🔢 New quantization formats (dtypes)🏛 120 supported architectures in total📂 25 new example projects and templates🤖 Over 1200 pre-converted models🌐 Node.js (ESM + CJS), Deno, and Bun compatibility🏡 A new home on GitHub and NPM * DeepSeek drops Janus 1.3B (X, HF, Paper) * DeepSeek releases Janus 1.3B 🔥 * 🎨 Understands and generates both images and text * 👀Combines DeepSeek LLM 1.3B with SigLIP-L for vision * ✂️ Decouples the vision encoding * Cohere for AI releases Aya expanse 8B, 32B (X, HF, Try it) * Aya Expanse is an open-weight research release of a model with highly advanced multilingual capabilities. It focuses on pairing a highly performant pre-trained Command family of models with the result of a year’s dedicated research from Cohere For AI, including data arbitrage, multilingual preference training, safety tuning, and model merging. The result is a powerful multilingual large language model serving 23 languages. * 23 languages * Big CO LLMs + APIs * New Claude Sonnet 3.5, Claude Haiku 3.5 * New Claude absolutely crushes coding benchmarks like Aider and Swe-bench verified. * But I'm getting mixed signals from folks with internal benchmarks, as well as some other benches like Aidan Bench and Arc challenge in which it performs worse. * 8K output token limit vs 4K * Other folks swear by it, Skirano, Corbitt say it's an absolute killer coder * Haiku is 2x the price of 4o-mini and Flash * Anthropic Computer use API + docker (X) * Computer use is not new, see open interpreter etc * Adept has been promising this for a while, so was LAM from rabbit. * Now Anthropic has dropped a bomb on all these with a specific trained model to browse click and surf the web with a container * Examples of computer use are super cool, Corbitt built agent.exe which uses it to control your computer * Killian will join to talk about what this computer use means * Folks are trying to order food (like Anthropic shows in their demo of ordering pizzas for the team) * Claude launches code interpreter mode for claude.ai (X) * Cohere released Embed 3 for multimodal embeddings (Blog) * 🔍 Multimodal Embed 3: Powerful AI search model * 🌍 Unlocks value from image data for enterprises * 🔍 Enables fast retrieval of relevant info & assets * 🛒 Transforms e-commerce search with image search * 🎨 Streamlines design process with visual search * 📊 Improves data-driven decision making with visual insights * 🔝 Industry-leading accuracy and performance * 🌐 Multilingual support across 100+ languages * 🤝 Partnerships with Azure AI and Amazon SageMaker * 🚀 Available now for businesses and developers * X ai has a new API platform + secret vision feature (docs) * grok-2-beta $5.0 / $15.00 mtok * Apple releases IOS 18.2 beta with GenMoji, Visual Intelligence, ChatGPT integration & more * Siri is still stupid, but can now ask chatGPT to write s**t * This weeks Buzz * Got second place for the hackathon with our AI Chef that roasts you in the kitchen (X, Weave dash) * Weave is now multimodal and supports audio! (Weave) * Tinkerers Hackathon in less than a week! * Vision & Video * Genmo releases Mochi-1 txt2video model w/ Apache 2.0 license * Gen mo - generative motion * 10B DiT - diffusion transformer * 5.5 seconds video * Apache 2.0 * Comparison thread between Genmo Mochi-1 and Hailuo * Genmo, the company behind Mochi 1, has raised $28.4M in Series A funding from various investors. Mochi 1 is an open-source video generation model that the company claims has "superior motion quality, prompt adherence and exceptional rendering of humans that begins to cross the uncanny valley." The company is open-sourcing their base 480p model, with an HD version coming soon. Summary Bullet Points: * Genmo announces $28.4M Series A funding * Mochi 1 is an open-source video generation model * Mochi 1 has "superior motion quality, prompt adherence and exceptional rendering of humans" * X is open-sourcing their base 480p Mochi 1 model * HD version of Mochi 1 is coming soon * Mochi 1 is available via Genmo's playground or as downloadable weights, or on Fal * Mochi 1 is licensed under Apache 2.0 * Rhymes AI - Allegro video model (X) * Meta a bunch of releases - Sam 2.1, Spirit LM * Runway introduces puppetry video 2 video with emotion transfer (X) * The webpage introduces Act-One, a new technology from Runway that allows for the generation of expressive character performances using a single driving video and character image, without the need for motion capture or rigging. Act-One faithfully represents elements like eye-lines, micro expressions, pacing, and delivery in the final generated output. It can translate an actor's performance across different character designs and styles, opening up new avenues for creative expression. Summary in 10 Bullet Points: * Act-One is a new technology from Runway * It generates expressive character performances * Uses a single driving video and character image * No motion capture or rigging required * Faithfully represents eye-lines, micro expressions, pacing, and delivery * Translates performance across different character designs and styles * Allows for new creative expression possibilities * Works with simple cell phone video input * Replaces complex, multi-step animation workflows * Enables capturing the essence of an actor's performance * Haiper releases a new video model * Meta releases Sam 2.1 * Key updates to SAM 2: * New data augmentation for similar and small objects * Improved occlusion handling * Longer frame sequences in training * Tweaks to positional encoding SAM 2 Developer Suite released: * Open source code package * Training code for fine-tuning * Web demo front-end and back-end code * Voice & Audio * OpenAI released custom voice support for chat completion API (X, Docs) * Pricing is still insane ($200/1mtok) * This is not just TTS, this is advanced voice mode! * The things you can ddo with them are very interesting, like asking for acting, or singing. * 11labs create voices with a prompt is super cool (X) * Meta Spirit LM: An open source language model for seamless speech and text integration (Blog, weights) * Meta Spirit LM is a multimodal language model that: * Combines text and speech processing * Uses word-level interleaving for cross-modality generation * Has two versions: * Base: uses phonetic tokens * Expressive: uses pitch and style tokens for tone * Enables more natural speech generation * Can learn tasks like ASR, TTS, and speech classification * MoonShine for audio * AI Art & Diffusion & 3D * Stable Diffusion 3.5 was released (X, Blog, HF) * including Stable Diffusion 3.5 Large and Stable Diffusion 3.5 Large Turbo. * table Diffusion 3.5 Medium will be released on October 29th. * the permissive Stability AI Community License. * 🚀 Introducing Stable Diffusion 3.5 - powerful, customizable, and free models * 🔍 Improved prompt adherence and image quality compared to previous versions * ⚡️ Stable Diffusion 3.5 Large Turbo offers fast inference times * 🔧 Multiple variants for different hardware and use cases * 🎨 Empowering creators to distribute and monetize their work * 🌐 Available for commercial and non-commercial use under permissive license * 🔍 Listening to community feedback to advance their mission * 🔄 Stable Diffusion 3.5 Medium to be released on October 29th * 🤖 Commitment to transforming visual media with accessible AI tools * 🔜 Excited to see what the community creates with Stable Diffusion 3.5 * Ideogram released Canvas (X) * Canvas is a mix of Krea and Everart * Ideogram is a free AI tool for generating realistic images, posters, logos * Extend tool allows expanding images beyond original borders * Magic Fill tool enables editing specific image regions and details * Ideogram Canvas is a new interface for organizing, generating, editing images * Ideogram uses AI to enhance the creative process with speed and precision * Developers can integrate Ideogram's Magic Fill and Extend via the API * Privacy policy and other legal information available on the website * Ideogram is free-to-use, with paid plans offering additional features * Ideogram is available globally, with support for various browsers * OpenAI released a new sampler paper trying to beat diffusers (Blog) * Researchers at OpenAI have developed a new approach called sCM that simplifies the theoretical formulation of continuous-time consistency models, allowing them to stabilize and scale the training of these models for large datasets. The sCM approach achieves sample quality comparable to leading diffusion models, while using only two sampling steps - a 50x speedup over traditional diffusion models. Benchmarking shows sCM produces high-quality samples using less than 10% of the effective sampling compute required by other state-of-the-art generative models.The key innovation is that sCM models scale commensurately with the teacher diffusion models they are distilled from. As the diffusion models grow larger, the relative difference in sample quality between sCM and the teacher model diminishes. This allows sCM to leverage the advances in diffusion models to achieve impressive sample quality and generation speed, unlocking new possibilities for real-time, high-quality generative AI across domains like images, audio, and video. * 🔍 Simplifying continuous-time consistency models * 🔨 Stabilizing training for large datasets * 🔍 Scaling to 1.5 billion parameters on ImageNet * ⚡ 2-step sampling for 50x speedup vs. diffusion * 🎨 Comparable sample quality to diffusion models * 📊 Benchmarking against state-of-the-art models * 🗺️ Visualization of diffusion vs. consistency models * 🖼️ Selected 2-step samples from 1.5B model * 📈 Scaling sCM with teacher diffusion models * 🔭 Limitations and future work * Midjourney announces an editor (X) * announces the release of two new features for Midjourney users - an image editor for uploaded images and * image re-texturing for exploring materials, surfacing, and lighting. * These features will initially be available only to yearly members, members who have been subscribers for the past 12 months, and members with at least 10,000 images. * The post emphasizes the need to give the community, human moderators, and AI moderation systems time to adjust to the new features * Tools PS : Subscribe to the newsletter and podcast, and I'll be back next week with more AI escapades! 🫶 This is a public episode. If you'd like to discuss this with other subscribers or get access to bonus episodes, visit sub.thursdai.news/subscribe | |||
01 Nov 2024 | 📆 ThursdAI - Spooky Halloween edition with Video! | 01:49:05 | |
Hey everyone, Happy Halloween! Alex here, coming to you live from my mad scientist lair! For the first ever, live video stream of ThursdAI, I dressed up as a mad scientist and had my co-host, Fester the AI powered Skeleton join me (as well as my usual cohosts haha) in a very energetic and hopefully entertaining video stream! Since it's Halloween today, Fester (and I) have a very busy schedule, so no super length ThursdAI news-letter today, as we're still not in the realm of Gemini being able to write a decent draft that takes everything we talked about and cover all the breaking news, I'm afraid I will have to wish you a Happy Halloween and ask that you watch/listen to the episode. The TL;DR and show links from today, don't cover all the breaking news but the major things we saw today (and caught live on the show as Breaking News) were, ChatGPT now has search, Gemini has grounded search as well (seems like the release something before Google announces it streak from OpenAI continues). Here's a quick trailer of the major things that happened: This weeks buzz - Halloween AI toy with Weave In this weeks buzz, my long awaited Halloween project is finally live and operational! I've posted a public Weave dashboard here and the code (that you can run on your mac!) here Really looking forward to see all the amazing costumers the kiddos come up with and how Gemini will be able to respond to them, follow along! ThursdAI - Recaps of the most high signal AI weekly spaces is a reader-supported publication. To receive new posts and support my work, consider becoming a free or paid subscriber. Ok and finally my raw TL;DR notes and links for this week. Happy halloween everyone, I'm running off to spook the kiddos (and of course record and post about it!) ThursdAI - Oct 31 - TL;DR TL;DR of all topics covered: * Open Source LLMs: * Microsoft's OmniParser: SOTA UI parsing (MIT Licensed) 𝕏 * Groundbreaking model for web automation (MIT license). * State-of-the-art UI parsing and understanding. * Outperforms GPT-4V in parsing web UI. * Designed for web automation tasks. * Can be integrated into various development workflows. * ZhipuAI's GLM-4-Voice: End-to-end Chinese/English speech 𝕏 * End-to-end voice model for Chinese and English speech. * Open-sourced and readily available. * Focuses on direct speech understanding and generation. * Potential applications in various speech-related tasks. * Meta releases LongVU: Video LM for long videos 𝕏 * Handles long videos with impressive performance. * Uses DINOv2 for downsampling, eliminating redundant scenes. * Fuses features using DINOv2 and SigLIP. * Select tokens are passed to Qwen2/Llama-3.2-3B. * Demo and model are available on HuggingFace. * Potential for significant advancements in video understanding. * OpenAI new factuality benchmark (Blog, Github) * Introducing SimpleQA: new factuality benchmark * Goal: high correctness, diversity, challenging for frontier models * Question Curation: AI trainers, verified by second trainer * Quality Assurance: 3% inherent error rate * Topic Diversity: wide range of topics * Grading Methodology: "correct", "incorrect", "not attempted" * Model Comparison: smaller models answer fewer correctly * Calibration Measurement: larger models more calibrated * Limitations: only for short, fact-seeking queries * Conclusion: drive research on trustworthy AI * Big CO LLMs + APIs: * ChatGPT now has Search! (X) * Grounded search results in browsing the web * Still hallucinates * Reincarnation of Search GPT inside ChatGPT * Apple Intelligence Launch: Image features for iOS 18.2 [𝕏]( Link not provided in source material) * Officially launched for developers in iOS 18.2. * Includes Image Playground and Gen Moji. * Aims to enhance image creation and manipulation on iPhones. * GitHub Universe AI News: Co-pilot expands, new Spark tool 𝕏 * GitHub Co-pilot now supports Claude, Gemini, and OpenAI models. * GitHub Spark: Create micro-apps using natural language. * Expanding the capabilities of AI-powered coding tools. * Copilot now supports multi-file edits in VS Code, similar to Cursor, and faster code reviews. * GitHub Copilot extensions are planned for release in 2025. * Grok Vision: Image understanding now in Grok 𝕏 * Finally has vision capabilities (currently via 𝕏, API coming soon). * Can now understand and explain images, even jokes. * Early version, with rapid improvements expected. * OpenAI advanced voice mode updates (X) * 70% cheaper in input tokens because of automatic caching (X) * Advanced voice mode is now on desktop app * Claude this morning - new mac / pc App * This week's Buzz: * My AI Halloween toy skeleton is greeting kids right now (and is reporting to Weave dashboard) * Vision & Video: * Meta's LongVU: Video LM for long videos 𝕏 (see Open Source LLMs for details) * Grok Vision on 𝕏: 𝕏 (see Big CO LLMs + APIs for details) * Voice & Audio: * MaskGCT: New SoTA Text-to-Speech 𝕏 * New open-source state-of-the-art text-to-speech model. * Zero-shot voice cloning, emotional TTS, long-form synthesis, variable speed synthesis, bilingual (Chinese & English). * Available on Hugging Face. * ZhipuAI's GLM-4-Voice: End-to-end Chinese/English speech 𝕏 (see Open Source LLMs for details) * Advanced Voice Mode on Desktops: 𝕏 (See Big CO LLMs + APIs for details). * AI Art & Diffusion: (See Red Panda in "This week's Buzz" above) * Redcraft Red Panda: new SOTA image diffusion 𝕏 * High-performing image diffusion model, beating Black Forest Labs Flux. * 72% win rate, higher ELO than competitors. * Creates SVG files, editable as vector files. * From Redcraft V3. * Tools: * Bolt.new by StackBlitz: In-browser full-stack dev environment 𝕏 * Platform for prompting, editing, running, and deploying full-stack apps directly in your browser. * Uses WebContainers. * Supports npm, Vite, Next.js, and integrations with Netlify, Cloudflare, and SuperBase. * Free to use. * Jina AI's Meta-Prompt: Improved LLM Codegen 𝕏 This is a public episode. If you'd like to discuss this with other subscribers or get access to bonus episodes, visit sub.thursdai.news/subscribe | |||
08 Nov 2024 | 📆 ThursdAI - Nov 7 - Video version, full o1 was given and taken away, Anthropic price hike-u, halloween 💀 recap & more AI news | 01:38:22 | |
👋 Hey all, this is Alex, coming to you from the very Sunny California, as I'm in SF again, while there is a complete snow storm back home in Denver (brrr). I flew here for the Hackathon I kept telling you about, and it was glorious, we had over 400 registered, over 200 approved hackers, 21 teams submitted incredible projects 👏 You can follow some of these here I then decided to stick around and record the show from SF, and finally pulled the plug and asked for some budget, and I present, the first ThursdAI, recorded from the newly minted W&B Podcast studio at our office in SF 🎉 This isn't the only first, today also, for the first time, all of the regular co-hosts of ThursdAI, met on video for the first time, after over a year of hanging out weekly, we've finally made the switch to video, and you know what? Given how good AI podcasts are getting, we may have to stick around with this video thing! We played one such clip from a new model called hertz-dev, which is a <10B model for full duplex audio. Given that today's episode is a video podcast, I would love for you to see it, so here's the timestamps for the chapters, which will be followed by the TL;DR and show notes in raw format. I would love to hear from folks who read the longer form style newsletters, do you miss them? Should I bring them back? Please leave me a comment 🙏 (I may send you a survey) This was a generally slow week (for AI!! not for... ehrm other stuff) and it was a fun podcast! Leave me a comment about what you think about this new format. Chapter Timestamps 00:00 Introduction and Agenda Overview 00:15 Open Source LLMs: Small Models 01:25 Open Source LLMs: Large Models 02:22 Big Companies and LLM Announcements 04:47 Hackathon Recap and Community Highlights 18:46 Technical Deep Dive: HertzDev and FishSpeech 33:11 Human in the Loop: AI Agents 36:24 Augmented Reality Lab Assistant 36:53 Hackathon Highlights and Community Vibes 37:17 Chef Puppet and Meta Ray Bans Raffle 37:46 Introducing Fester the Skeleton 38:37 Fester's Performance and Community Reactions 39:35 Technical Insights and Project Details 42:42 Big Companies API Updates 43:17 Haiku 3.5: Performance and Pricing 43:44 Comparing Haiku and Sonnet Models 51:32 XAI Grok: New Features and Pricing 57:23 OpenAI's O1 Model: Leaks and Expectations 01:08:42 Transformer ASIC: The Future of AI Hardware 01:13:18 The Future of Training and Inference Chips 01:13:52 Oasis Demo and Etched AI Controversy 01:14:37 Nisten's Skepticism on Etched AI 01:19:15 Human Layer Introduction with Dex 01:19:24 Building and Managing AI Agents 01:20:54 Challenges and Innovations in AI Agent Development 01:21:28 Human Layer's Vision and Future 01:36:34 Recap and Closing Remarks ThursdAI - Recaps of the most high signal AI weekly spaces is a reader-supported publication. To receive new posts and support my work, consider becoming a free or paid subscriber. Show Notes and Links: * Interview * Dexter Horthy (X) from HumanLayer * Open Source LLMs * SmolLM2: the new, best, and open 1B-parameter language mode (X) * Meta released MobileLLM (125M, 350M, 600M, 1B) (HF) * Tencent Hunyuan Large - 389B X 52B (Active) MoE (X, HF, Paper) * Big CO LLMs + APIs * OpenAI buys and opens chat.com * Anthropic releases Claude Haiku 3.5 via API (X, Blog) * OpenAI drops o1 full - and pulls it back (but not before it got Jailbroken) * X.ai now offers $25/mo free of Grok API credits (X, Platform) * Etched announces Sonu - first Transformer ASIC - 500K tok/s (etched) * PPXL is not valued at 9B lol * This weeks Buzz * Recap of SF Hackathon w/ AI Tinkerers (X) * Fester the Halloween Toy aka Project Halloweave videos from trick or treating (X, Writeup) * Voice & Audio * Hertz-dev - 8.5B conversation audio gen (X, Blog ) * Fish Agent v0.1 3B - Speech to Speech model (HF, Demo) * AI Art & Diffusion & 3D * FLUX 1.1 [pro] is how HD - 4x resolution (X, blog) Full Transcription for convenience below: This is a public episode. If you'd like to discuss this with other subscribers or get access to bonus episodes, visit sub.thursdai.news/subscribe | |||
15 Nov 2024 | 📆 ThursdAI - Nov 14 - Qwen 2.5 Coder, No Walls, Gemini 1114 👑 LLM, ChatGPT OS integrations & more AI news | 01:48:42 | |
This week is a very exciting one in the world of AI news, as we get 3 SOTA models, one in overall LLM rankings, on in OSS coding and one in OSS voice + a bunch of new breaking news during the show (which we reacted to live on the pod, and as we're now doing video, you can see us freak out in real time at 59:32) 00:00 Welcome to ThursdAI 00:25 Meet the Hosts 02:38 Show Format and Community 03:18 TLDR Overview 04:01 Open Source Highlights 13:31 Qwen Coder 2.5 Release 14:00 Speculative Decoding and Model Performance 22:18 Interactive Demos and Artifacts 28:20 Training Insights and Future Prospects 33:54 Breaking News: Nexus Flow 36:23 Exploring Athene v2 Agent Capabilities 36:48 Understanding ArenaHard and Benchmarking 40:55 Scaling and Limitations in AI Models 43:04 Nexus Flow and Scaling Debate 49:00 Open Source LLMs and New Releases 52:29 FrontierMath Benchmark and Quantization Challenges 58:50 Gemini Experimental 1114 Release and Performance 01:11:28 LLM Observability with Weave 01:14:55 Introduction to Tracing and Evaluations 01:15:50 Weave API Toolkit Overview 01:16:08 Buzz Corner: Weights & Biases 01:16:18 Nous Forge Reasoning API 01:26:39 Breaking News: OpenAI's New MacOS Features 01:27:41 Live Demo: ChatGPT Integration with VS Code 01:34:28 Ultravox: Real-Time AI Conversations 01:42:03 Tilde Research and Stargazer Tool 01:46:12 Conclusion and Final Thoughts This week also, there was a debate online, whether deep learning (and scale is all you need) has hit a wall, with folks like Ilya Sutskever being cited by publications claiming it has, folks like Yann LeCoon calling "I told you so". TL;DR? multiple huge breakthroughs later, and both Oriol from DeepMind and Sam Altman are saying "what wall?" and Heiner from X.ai saying "skill issue", there is no walls in sight, despite some tech journalism love to pretend there is. Also, what happened to Yann? 😵💫 Ok, back to our scheduled programming, here's the TL;DR, afterwhich, a breakdown of the most important things about today's update, and as always, I encourage you to watch / listen to the show, as we cover way more than I summarize here 🙂 TL;DR and Show Notes: * Open Source LLMs * Qwen Coder 2.5 32B (+5 others) - Sonnet @ home (HF, Blog, Tech Report) * The End of Quantization? (X, Original Thread) * Epoch : FrontierMath new benchmark for advanced MATH reasoning in AI (Blog) * Common Corpus: Largest multilingual 2T token dataset (blog) * NexusFlow - Athena v2 - open model suite (X, Blog, HF) * Big CO LLMs + APIs * Gemini 1114 is new king LLM #1 LMArena (X) * Nous Forge Reasoning API - beta (Blog, X) * Reuters reports "AI is hitting a wall" and it's becoming a meme (Article) * Cursor acq. SuperMaven (X) * This Weeks Buzz * Weave JS/TS support is here 🙌 * Voice & Audio * Fixie releases UltraVox SOTA (Demo, HF, API) * Suno v4 is coming and it's bonkers amazing (Alex Song, SOTA Jingle) * Tools demoed * Qwen artifacts - HF Demo * Tilde Galaxy - Interp Tool This is a public episode. If you'd like to discuss this with other subscribers or get access to bonus episodes, visit sub.thursdai.news/subscribe | |||
22 Nov 2024 | 📆 ThursdAI - Nov 21 - The fight for the LLM throne, OSS SOTA from AllenAI, Flux new tools, Deepseek R1 reasoning & more AI news | 01:45:25 | |
Hey folks, Alex here, and oof what a 🔥🔥🔥 show we had today! I got to use my new breaking news button 3 times this show! And not only that, some of you may know that one of the absolutely biggest pleasures as a host, is to feature the folks who actually make the news on the show! And now that we're in video format, you actually get to see who they are! So this week I was honored to welcome back our friend and co-host Junyang Lin, a Dev Lead from the Alibaba Qwen team, who came back after launching the incredible Qwen Coder 2.5, and Qwen 2.5 Turbo with 1M context. We also had breaking news on the show that AI2 (Allen Institute for AI) has fully released SOTA LLama post-trained models, and I was very lucky to get the core contributor on the paper, Nathan Lambert to join us live and tell us all about this amazing open source effort! You don't want to miss this conversation! Lastly, we chatted with the CEO of StackBlitz, Eric Simons, about the absolutely incredible lightning in the bottle success of their latest bolt.new product, how it opens a new category of code generator related tools. 00:00 Introduction and Welcome 00:58 Meet the Hosts and Guests 02:28 TLDR Overview 03:21 Tl;DR 04:10 Big Companies and APIs 07:47 Agent News and Announcements 08:05 Voice and Audio Updates 08:48 AR, Art, and Diffusion 11:02 Deep Dive into Mistral and Pixtral 29:28 Interview with Nathan Lambert from AI2 30:23 Live Reaction to Tulu 3 Release 30:50 Deep Dive into Tulu 3 Features 32:45 Open Source Commitment and Community Impact 33:13 Exploring the Released Artifacts 33:55 Detailed Breakdown of Datasets and Models 37:03 Motivation Behind Open Source 38:02 Q&A Session with the Community 38:52 Summarizing Key Insights and Future Directions 40:15 Discussion on Long Context Understanding 41:52 Closing Remarks and Acknowledgements 44:38 Transition to Big Companies and APIs 45:03 Weights & Biases: This Week's Buzz 01:02:50 Mistral's New Features and Upgrades 01:07:00 Introduction to DeepSeek and the Whale Giant 01:07:44 DeepSeek's Technological Achievements 01:08:02 Open Source Models and API Announcement 01:09:32 DeepSeek's Reasoning Capabilities 01:12:07 Scaling Laws and Future Predictions 01:14:13 Interview with Eric from Bolt 01:14:41 Breaking News: Gemini Experimental 01:17:26 Interview with Eric Simons - CEO @ Stackblitz 01:19:39 Live Demo of Bolt's Capabilities 01:36:17 Black Forest Labs AI Art Tools 01:40:45 Conclusion and Final Thoughts As always, the show notes and TL;DR with all the links I mentioned on the show and the full news roundup below the main new recap 👇 Google & OpenAI fighting for the LMArena crown 👑 I wanted to open with this, as last week I reported that Gemini Exp 1114 has taken over #1 in the LMArena, in less than a week, we saw a new ChatGPT release, called GPT-4o-2024-11-20 reclaim the arena #1 spot! Focusing specifically on creating writing, this new model, that's now deployed on chat.com and in the API, is definitely more creative according to many folks who've tried it, with OpenAI employees saying "expect qualitative improvements with more natural and engaging writing, thoroughness and readability" and indeed that's what my feed was reporting as well. I also wanted to mention here, that we've seen this happen once before, last time Gemini peaked at the LMArena, it took less than a week for OpenAI to release and test a model that beat it. But not this time, this time Google came prepared with an answer! Just as we were wrapping up the show (again, Logan apparently loves dropping things at the end of ThursdAI), we got breaking news that there is YET another experimental model from Google, called Gemini Exp 1121, and apparently, it reclaims the stolen #1 position, that chatGPT reclaimed from Gemini... yesterday! Or at least joins it at #1 LMArena Fatigue? Many folks in my DMs are getting a bit frustrated with these marketing tactics, not only the fact that we're getting experimental models faster than we can test them, but also with the fact that if you think about it, this was probably a calculated move by Google. Release a very powerful checkpoint, knowing that this will trigger a response from OpenAI, but don't release your most powerful one. OpenAI predictably releases their own "ready to go" checkpoint to show they are ahead, then folks at Google wait and release what they wanted to release in the first place. The other frustration point is, the over-indexing of the major labs on the LMArena human metrics, as the closest approximation for "best". For example, here's some analysis from Artificial Analysis showing that the while the latest ChatGPT is indeed better at creative writing (and #1 in the Arena, where humans vote answers against each other), it's gotten actively worse at MATH and coding from the August version (which could be a result of being a distilled much smaller version) . In summary, maybe the LMArena is no longer 1 arena is all you need, but the competition at the TOP scores of the Arena has never been hotter. DeepSeek R-1 preview - reasoning from the Chinese Whale While the American labs fight for the LM titles, the real interesting news may be coming from the Chinese whale, DeepSeek, a company known for their incredibly cracked team, resurfaced once again and showed us that they are indeed, well super cracked. They have trained and released R-1 preview, with Reinforcement Learning, a reasoning model that beasts O1 at AIME and other benchmarks! We don't know many details yet, besides them confirming that this model comes to the open source! but we do know that this model , unlike O1, is showing the actual reasoning it uses to achieve it's answers (reminder: O1 hides its actual reasoning and what we see is actually another model summarizing the reasoning) The other notable thing is, DeepSeek all but confirmed the claim that we have a new scaling law with Test Time / Inference time compute law, where, like with O1, the more time (and tokens) you give a model to think, the better it gets at answering hard questions. Which is a very important confirmation, and is a VERY exciting one if this is coming to the open source! Right now you can play around with R1 in their demo chat interface. In other Big Co and API news In other news, Mistral becomes a Research/Product company, with a host of new additions to Le Chat, including Browse, PDF upload, Canvas and Flux 1.1 Pro integration (for Free! I think this is the only place where you can get Flux Pro for free!). Qwen released a new 1M context window model in their API called Qwen 2.5 Turbo, making it not only the 2nd ever 1M+ model (after Gemini) to be available, but also reducing TTFT (time to first token) significantly and slashing costs. This is available via their APIs and Demo here. Open Source is catching up AI2 open sources Tulu 3 - SOTA 8B, 70B LLama post trained FULLY open sourced (Blog ,Demo, HF, Data, Github, Paper) Allen AI folks have joined the show before, and this time we got Nathan Lambert, the core contributor on the Tulu paper, join and talk to us about Post Training and how they made the best performing SOTA LLama 3.1 Funetunes with careful data curation (which they also open sourced), preference optimization, and a new methodology they call RLVR (Reinforcement Learning with Verifiable Rewards). Simply put, RLVR modifies the RLHF approach by using a verification function instead of a reward model. This method is effective for tasks with verifiable answers, like math problems or specific instructions. It improves performance on certain benchmarks (e.g., GSM8K) while maintaining capabilities in other areas. The most notable thing is, just how MUCH is open source, as again, like the last time we had AI2 folks on the show, the amount they release is staggering In the show, Nathan had me pull up the paper and we went through the deluge of models, code and datasets they released, not to mention the 73 page paper full of methodology and techniques. Just absolute ❤️ to the AI2 team for this release! 🐝 This weeks buzz - Weights & Biases corner This week, I want to invite you to a live stream announcement that I am working on behind the scenes to produce, on December 2nd. You can register HERE (it's on LinkedIn, I know, I'll have the YT link next week, promise!) We have some very exciting news to announce, and I would really appreciate the ThursdAI crew showing up for that! It's like 5 minutes and I helped produce 🙂 Pixtral Large is making VLMs cool again Mistral had quite the week this week, not only adding features to Le Chat, but also releasing Pixtral Large, their updated multimodal model, which they claim state of the art on multiple benchmarks. It's really quite good, not to mention that it's also included, for free, as part of the le chat platform, so now when you upload documents or images to le chat you get Pixtral Large. The backbone for this model is Mistral Large (not the new one they also released) and this makes this 124B model a really really good image model, albeit a VERY chonky one that's hard to run locally. The thing I loved about the Pixtral release the most is, they used the new understanding to ask about Weights & Biases charts 😅 and Pixtral did a pretty good job! Some members of the community though, reacted to the SOTA claims by Mistral in a very specific meme-y way: This meme has become a very standard one, when labs tend to not include Qwen VL 72B or other Qwen models in the evaluation results, all while claiming SOTA. I decided to put these models to a head to head test myself, only to find out, that ironically, both models say the other one is better, while both hallucinate some numbers. BFL is putting the ART in Artificial Intelligence with FLUX.1 Tools (blog) With the absolute breaking news bombastic release, the folks at BFL (Black Forest Labs) have released Flux.1 Tools, which will allow AI artist to use these models in all kind of creative inspiring ways. These tools are: FLUX.1 Fill (for In/Out painting), FLUX.1 Depth/Canny (Structural Guidance using depth map or canny edges) and FLUX.1 Redux for image variation and restyling. These tools are not new to the AI Art community conceptually, but they have been patched over onto Flux from other models like SDXL, and now the actual lab releasing them gave us the crème de la crème, and the evals speak for themselves, achieving SOTA on image variation benchmark! The last thing I haven't covered here, is my interview with Eric Simons, the CEO of StackBlitz, who came in to talk about the insane rise of bolt.new, and I would refer you to the actual recording for that, because it's really worth listening to it (and seeing me trying out bolt in real time!) That's most of the recap, we talked about a BUNCH of other stuff of course, and we finished on THIS rap song that ChatGPT wrote, and Suno v4 produced with credits to Kyle Shannon. TL;DR and Show Notes: * Open Source LLMs * Mistral releases Pixtral Large (Blog, HF, LeChat) * Mistral - Mistral Large 2411 (a HF) * Sage Attention the next Flash Attention? (X) * AI2 open sources Tulu 3 - SOTA 8B, 70B LLama Finetunes FULLY open sourced (Blog ,Demo, HF, Data, Github, Paper) * Big CO LLMs + APIs * Alibaba - Qwen 2.5 Turbo with 1M tokens (X, HF Demo) * Mistral upgrades to a product company with le chat 2.0 (Blog, Le Chat) * DeepSeek R1-preview - the first reasoning model from the Chinese whale (X, chat) * OpenAI updates ChatGPT in app and API - reclaims #1 on LMArena (X) * Gemini Exp 1121 - rejoins #1 spot on LMArena after 1 day of being beaten (X) * Agents News * Perplexity is going to do the shopping for you (X, Shop) * Stripe Agent SDK - allowing agents to transact (Blog) * This weeks Buzz * We have an important announcement coming on December 2nd! (link) * Voice & Audio * Suno V4 released - but for real this time (X) * ChatGPT new creative writing does Eminem type rap with new Suno v4 (link) * AI Art & Diffusion & 3D * BFL announcing Flux Tools today (blog, fal) * Free BFL Flux Pro on Mistral Le Chat! * Thank you, see you next week 🫡 This is a public episode. If you'd like to discuss this with other subscribers or get access to bonus episodes, visit sub.thursdai.news/subscribe | |||
28 Nov 2024 | 🦃 ThursdAI - Thanksgiving special 24' - Qwen Open Sources Reasoning, BlueSky hates AI, H controls the web & more AI news | 01:46:16 | |
Hey ya'll, Happy Thanskgiving to everyone who celebrates and thank you for being a subscriber, I truly appreciate each and every one of you! We had a blast on today's celebratory stream, especially given that today's "main course" was the amazing open sourcing of a reasoning model from Qwen, and we had Junyang Lin with us again to talk about it! First open source reasoning model that you can run on your machine, that beats a 405B model, comes close to o1 on some metrics 🤯 We also chatted about a new hybrid approach from Nvidia called Hymba 1.5B (Paper, HF) that beats Qwen 1.5B with 6-12x less training, and Allen AI releasing Olmo 2, which became the best fully open source LLM 👏 (Blog, HF, Demo), though they didn't release WandB logs this time, they did release data! I encourage you to watch todays show (or listen to the show, I don't judge), there's not going to be a long writeup like I usually do, as I want to go and enjoy the holiday too, but of course, the TL;DR and show notes are right here so you won't miss a beat if you want to use the break to explore and play around with a few things! ThursdAI - Recaps of the most high signal AI weekly spaces is a reader-supported publication. To receive new posts and support my work, consider becoming a free or paid subscriber. TL;DR and show notes * Qwen QwQ 32B preview - the first open weights reasoning model (X, Blog, HF, Try it) * Allen AI - Olmo 2 the best fully open language model (Blog, HF, Demo) * NVIDIA Hymba 1.5B - Hybrid smol model beating Qwen, SmolLM w/ 6-12x less training (X, Paper, HF) * Big CO LLMs + APIs * Anthropic MCP - model context protocol (X,Blog, Spec, Explainer) * Cursor, Jetbrains now integrate with ChatGPT MacOS app (X) * Xai is going to be a Gaming company?! (X) * H company shows Runner H - WebVoyager Agent (X, Waitlist) * This weeks Buzz * Interview w/ Thomas Cepelle about Weave scorers and guardrails (Guide) * Vision & Video * OpenAI SORA API was "leaked" on HuggingFace (here) * Runway launches video Expand feature (X) * Rhymes Allegro-TI2V - updated image to video model (HF) * Voice & Audio * OuteTTS v0.2 - 500M smol TTS with voice cloning (Blog, HF) * AI Art & Diffusion & 3D * Runway launches an image model called Frames (X, Blog) * ComfyUI Desktop app was released 🎉 * Chat * 24 hours of AI hate on 🦋 (thread) * Tools * Cursor agent (X thread) * Google Generative Chess toy (Link) See you next week and happy Thanks Giving 🦃 Thanks for reading ThursdAI - Recaps of the most high signal AI weekly spaces! This post is public so feel free to share it. Full Subtitles for convenience [00:00:00] Alex Volkov: let's get it going. [00:00:10] Alex Volkov: Welcome, welcome everyone to ThursdAI November 28th Thanksgiving special. My name is Alex Volkov. I'm an AI evangelist with Weights Biases. You're on ThursdAI. We are live [00:00:30] on ThursdAI. Everywhere pretty much. [00:00:32] Alex Volkov: [00:00:32] Hosts and Guests Introduction [00:00:32] Alex Volkov: I'm joined here with two of my co hosts. [00:00:35] Alex Volkov: Wolfram, welcome. [00:00:36] Wolfram Ravenwolf: Hello everyone! Happy Thanksgiving! [00:00:38] Alex Volkov: Happy Thanksgiving, man. [00:00:39] Alex Volkov: And we have Junyang here. Junyang, welcome, man. [00:00:42] Junyang Lin: Yeah, hi everyone. Happy Thanksgiving. Great to be here. [00:00:46] Alex Volkov: You had a busy week. We're going to chat about what you had. I see Nisten joining us as well at some point. [00:00:51] Alex Volkov: Yam pe joining us as well. Hey, how, Hey Yam. Welcome. Welcome, as well. Happy Thanksgiving. It looks like we're assembled folks. We're across streams, across [00:01:00] countries, but we are. [00:01:01] Overview of Topics for the Episode [00:01:01] Alex Volkov: For November 28th, we have a bunch of stuff to talk about. Like really a big list of stuff to talk about. So why don't we just we'll just dive in. We'll just dive in. So obviously I think the best and the most important. [00:01:13] DeepSeek and Qwen Open Source AI News [00:01:13] Alex Volkov: Open source kind of AI news to talk about this week is going to be, and I think I remember last week, Junyang, I asked you about this and you were like, you couldn't say anything, but I asked because last week, folks, if you remember, we talked about R1 from DeepSeek, a reasoning model from [00:01:30] DeepSeek, which really said, Oh, maybe it comes as a, as open source and maybe it doesn't. [00:01:33] Alex Volkov: And I hinted about, and I asked, Junyang, what about some reasoning from you guys? And you couldn't say anything. so this week. I'm going to do a TLDR. So we're going to actually talk about the stuff that, you know, in depth a little bit later, but this week, obviously one of the biggest kind of open source or sorry, open weights, and news is coming from our friends at Qwen as well, as we always celebrate. [00:01:56] Alex Volkov: So one of the biggest things that we get as. [00:02:00] is, Qwen releases, I will actually have you tell me what's the pronunciation here, Junaid, what is, I say Q W Q or maybe quick, what is the pronunciation of this? [00:02:12] Junyang Lin: I mentioned it in the blog, it is just like the word quill. Yeah. yeah, because for the qw you can like work and for the q and you just like the U, so I just combine it together and create a new pronunciation called Quill. [00:02:28] Junyang Lin: Yeah. [00:02:28] Alex Volkov: So we're saying it's Quin [00:02:30] Quill 32 B. Is that the right pronunciation to say this? [00:02:33] Junyang Lin: Yeah, it's okay. I would just call it qui quill. It is, some something funny because,the ca the characters look very funny. Oh, we have a subculture,for these things. Yeah. Just to express some, yeah. [00:02:46] Junyang Lin: our. feelings. [00:02:49] Alex Volkov: Amazing. Qwen, Quill, 32B, and it's typed,the name is typed QWQ, 32Breview. This is the first OpenWeights reasoning model. This [00:03:00] model is not only predicting tokens, it's actually doing reasoning behind this. What this means is we're going to tell you what this means after we get to this. [00:03:07] Alex Volkov: So we're still in the, we're still in the TLDR area. We also had. Another drop from Alien Institute for AI, if you guys remember last week we chatted with Nathan, our dear friend Nathan, from Alien Institute about 2. 0. 3, about their efforts for post training, and he gave us all the details about post training, so they released 2. [00:03:28] Alex Volkov: 0. 3, this week they released Olmo 2. [00:03:30] 0. We also talked about Olmo with the friends from Alien Institute a couple of months ago, and now they released Olmo 2. 0. Which they claim is the best fully open sourced, fully open sourced language models, from Allen Institute for AI.and, so we're going to chat about, Olmo a little bit as well. [00:03:46] Alex Volkov: And last minute addition we have is NVIDIA Haimba, which is a hybrid small model from NVIDIA, very tiny one, 1. 5 billion parameters. small model building Qwen and building small LLM as well. this is in the area [00:04:00] of open source. I [00:04:01] Alex Volkov: Okay, in the big companies, LLMs and APIs, I want to run through a few things. [00:04:06] Anthropic's MCP and ChatGPT macOS Integrations [00:04:06] Alex Volkov: So first of all, Anthropic really something called MCP. It's a, something they called Model Context Protocol. We're going to briefly run through this. It's a, it's a kind of a release from them that's aimed for developers is a protocol that enables secure connections between a host application, like a cloud desktop, for example, [00:04:24] Alex Volkov: there's also a bunch of new integrations for the ChatGPT macOS app. If you guys remember a couple of [00:04:30] weeks ago, We actually caught this live. [00:04:31] Alex Volkov: I refreshed my MacOS app and there's ta da, there's a new thing. And we discovered this live. It was very fun. The MacOS app for ChatGPT integrates with VS Code, et cetera. and so we tried to run this with Cursor. It didn't work. So now it works with Cursor, [00:04:43] Wolfram Ravenwolf: [00:04:43] Alex Volkov: So the next thing we're going to look at, I don't know if it's worth mentioning, but you guys know the XAI, the company that Elon Musk is raising another 6 billion for that tries to compete with OpenAI [00:04:54] Alex Volkov: Do you guys hear that it's going to be a gaming company as well? I don't know if it's worth talking about, but we'll at least [00:05:00] mention this. And the one thing that I wanted to chat about is H, the French company, H that showed a runner that looks. Three times as fast and as good as the Claude computer use runner, and we're definitely going to show examples of this, video live because that looks just incredible. [00:05:18] Alex Volkov: this out of nowhere company, the biggest fundraise or the biggest seed round that Europe has ever seen, at least French has ever seen, just show they, An agent that controls your [00:05:30] computer that's tiny, ridiculously tiny, I think it's like the three billion parameter, two billion parameter or something. [00:05:36] Alex Volkov: And it runs way better than computer, cloud computer use. Something definitely worth talking about. after with, after which in this week's Bars, we're going to talk with Thomas Capelli, from, from my team at Weights Biases. about LLM guardrails, that's gonna be fun. and in vision video category, we're gonna cover that OpenAI Sora quote unquote leaked, this week. [00:05:56] Alex Volkov: And this leak wasn't really a leak, but, definitely [00:06:00] we saw some stuff. and then there's also a new expand feature that we saw in, Runway. And we saw another video model from, Rhymes called Allegro TIV2. which is pretty cool in voice and audio. If we get there in voice and audio, we saw out TTS vision 0. [00:06:19] Alex Volkov: 2, which is a new TTS, a 500 million parameter, small TTS you can run in your browser and sounds pretty dope.art in the fusion, super quick runway launches an image [00:06:30] model. Yep, Runway, the guys who do video, they launched an image model that looks pretty sick, and we're definitely going to look at some examples of this, and Confi UI Desktop, for those of you who are celebrating something like this, Confi UI now is runnable with desktop, and there's a bunch of tool stuff, but honestly, I can talk about two things. [00:06:47] Alex Volkov: Tools and there's a cool thing with Google generative chess toy. I can show you this so you can show your folks in Thanksgiving and going to impress them with a generative chess toy. But honestly, instead of this, I would love to chat about the thing that [00:07:00] some of us saw on the other side of the social media networks. [00:07:04] Alex Volkov: And definitely we'll chat about this, for the past 24 hours. So chat, for the past. 24 hours, on BlueSky, we saw a little bit of a mob going against the Hug Face folks and then, other friends of ours on,from the AI community and the anti AI mob on BlueSky. So we're going to chat about that. [00:07:26] Alex Volkov: And hopefully give you our feelings about what's going on, about this [00:07:30] world. And this is a pro AI show. And when we see injustice happens against ai, we have to speak out about against this. And I think that this is mostly what we're gonna cover this show, unless this is. [00:07:42] Wolfram Ravenwolf: Where I could insert the two things I have. [00:07:44] Wolfram Ravenwolf: One is a tool, which is the AI video composer, which, allows you to talk to, ff mpac, which is a complicated comment line tool, but very powerful. And so you have a UI where you just use natural language to control the tool. So that is one tool. Maybe we get to [00:08:00] it, if not just Google or ask for Plexity or anything. [00:08:03] Alex Volkov: No, we'll drop it in. Yeah, we'll drop it in show notes, absolutely. [00:08:04] Wolfram Ravenwolf: Yeah, that's the best part. Okay. And echo mimic. Version 2 is also an HN Synthesia alternative for local use, which is also, yeah, a great open source local runnable tool. [00:08:17] Alex Volkov: What do we call this? EcoMimic? [00:08:19] Wolfram Ravenwolf: EcoMimic. EcoMimic [00:08:21] Alex Volkov: v2. [00:08:21] Wolfram Ravenwolf: EcoMimic [00:08:23] Alex Volkov: 2. [00:08:24] Alex Volkov: Alright, we have a special guest here that we're gonna add Alpin. Hey Alpen, [00:08:30] welcome, feel free to stay anonymous and don't jump, we're gonna start with open source AI and then we're gonna chat with you briefly about the experience you had. [00:08:38] Alpin Dale: hello everyone. [00:08:39] Alex Volkov: Hey man. Yeah, you've been on the show before, right Alton? You've been on the show. [00:08:43] Alpin Dale: a few times, yeah. it's nice to be back here again. [00:08:46] Alex Volkov: Yeah. Alton, we're gonna get, we're gonna chat with you soon, right? We're gonna start with open source. We need to go to Junyang and talk about reasoning models. [00:08:52] Alex Volkov: so feel free to stay with us. And then I definitely want to hear about some of the stuff we're going to cover after open source. We're going to cover the [00:09:00] anti AI mob over there. [00:09:05] Alex Volkov: Alrighty folks, it's time to start with the,with the corner we love the most, yeah? let's dive into this. Let's dive in straight to Open Source AI. [00:09:29] Alex Volkov: Open Source AI, [00:09:30] let's get it started. Let's start it. [00:09:35] Alex Volkov: Okay, folks, so open source this week, we're going to get, let me cover the other two things super quick before we dive in. [00:09:43] NVIDIA Haimba Hybrid Model Discussion [00:09:43] Alex Volkov: Alright, so I want to like briefly cover the Haimba paper super quick, because we're going to get the least interesting stuff out of the way so we can focus on the main topic. Course, NVIDIA released Heimbar 1. 5 parameters. Heimbar is a hybrid small model, from NVIDIA. We talked about hybrid models [00:10:00] multiple times before. [00:10:00] Alex Volkov: we have our friend of the pod, LDJ here. He loves talking about hybrid models. He actually brought this to our attention in the, in, in the group chat. We talked about, you guys know the Transformer, we love talking about the Transformer. Haimba specifically is a hybrid model between Transformer and I think they're using a hybrid attention with Mamba layers in parallel. [00:10:22] Alex Volkov: they claim they're beating Lama and Qwen and SmallLM with 6 to 12 times less training as well. Let's look [00:10:30] at the, let's look at their, let's look at their X.so this is what they're, this is what they're showing, this is the table they're showing some impressive numbers, the interesting thing is, this is a table of comparison that they're showing, and in this table of comparison, the comparison is not only Evaluations. [00:10:47] Alex Volkov: The comparison they're showing is also cache size and throughput, which I like. it's do you guys know what this reminds me of? This reminds me of when you have a electric vehicle [00:11:00] and you have a gas based vehicle or standard combustion engine vehicle, and then they compare the electric vehicle and acceleration. [00:11:07] Alex Volkov: It's Oh, our car is faster. But you get this by default, you get the acceleration by default with all the electric vehicles. This is how the model works. This is how those model works. So for me, when you compare like hybrid models, or, non transformer based models, a Mamba based models, the throughput speed up is generally faster because of it. [00:11:29] Alex Volkov: [00:11:30] But definitely the throughput is significantly higher. Tokens per second. is significantly higher. So for comparison for folks who are listening to us, just so you, you'll hear the comparison, the throughput for this 1. 5 billion model is 664 tokens per second versus a small LM 238 tokens per second, or something like Qwen 1. [00:11:54] Alex Volkov: 5 at 400. So 600 versus 400. the training cost in [00:12:00] tokens, they say this was, 1. 5 trillion tokens versus Qwen at 18. I don't know if Junyang you want to confirm or deny the 18 mentioned here that they added. Sometimes they, they say different things, but yeah, definitely the highlight of this Heimwehr thing. [00:12:14] Alex Volkov: And this is from NVIDIA, by the way, I think it's very worth like shouting out that this specific thing comes from this model comes from NVIDIA. Um,they specifically mentioned that the cost, And outperformance of this model comes at 6 to 12 times less [00:12:30] training, which is very impressive. [00:12:31] Alex Volkov: what else about this model? Performance wise, MMLU at 52, which is lower than Qwen at 59, at, at 1. 5 billion parameters. GSM 8K, we know the GSM 8K is not that interesting anymore, I think, at this point. We're not like over, we're not over, we're not looking at this like too much. What else should we say about this model? [00:12:52] Alex Volkov: GPK is pretty interesting at 31. GPK is usually knowledge versus something. [00:13:00] Anything else to say about this model? Yeah, you have anything to say Nisten? Anything to say about the small models? About the hybrid model specifically? I know that like our friend LDJ said that like this seems like the first actual model that competes apples to apples. [00:13:13] Alex Volkov: Because usually when we compare Hybrid models specifically, those usually people say that those are not like necessarily one to one comparisons between hybrid models and just formal models. [00:13:24] Nisten Tahiraj: I was just going to say that fromfrom NVIDIA, we've heard these [00:13:30] claims before and they didn't quite turn out that way, so I'm going to start off a little bit more skeptical on that end. also from, from the Mistral Mamba, Mambastral, that one was not very performant. [00:13:44] Nisten Tahiraj: it seemed like it was going to be good for long context stuff. The runtime wasn't that good as well. yeah, I'm going to give this one a test because. Again, the promise of, of like hybrid, SSM models is that it can do better [00:14:00] in longer contexts and it can run faster. So it is worth testing given what, what they're claiming. [00:14:06] Nisten Tahiraj: But, again, on MMLU, it didn't do that well, but, yeah, overall the numbers do look great actually for what it is, but I think we do need to do further testing on this, whether it is practically. That's good. Because I'm not sure how well it's going to hold up after you just throw like 32k of context of it. [00:14:25] Nisten Tahiraj: I guess it's going to remember all that, but, yeah, this on paper, this does [00:14:30] look like it's one of the first ones that is Applesauce. [00:14:33] Alex Volkov: Yeah. All right. anything else to say here? Yeah, the architecture. Jan, go ahead. [00:14:39] Yam Peleg: Yeah, about the architecture. I tweeted about it.It is, I think it has extreme potential and, it might, I just by looking at the attention maps, from the paper, like just a glimpse is enough for you to see that. [00:14:55] Yam Peleg: They really do solve something really profound [00:15:00] with many of the models that we have today. basically, I'm really simplifying here, but basically, when you look at the Attention versus Mamba, they act very differently in terms of how they process the tokens, sliding window ones, you could say. [00:15:20] Yam Peleg: And of course self attention is like global, to everything, but Mamba is not exactly global, it's sequential, and sliding window is also not exactly [00:15:30] global, but it's not the same sequential, it's like everything to everything, but with a window. So what they did is combine the two, and you can really see the difference in attention map of the trained model. [00:15:44] Yam Peleg: it's not exactly the same as just, hybrid Mamba attention models that we all saw before.there is a lot to this model and I really want to see one of those. I just [00:16:00] trained for like at scale, like a large one on, on, on a huge data set, because I think it might be an improvement to either,just by looking at the way the model learned, but you cannot know until you actually try. [00:16:15] Yam Peleg: I tweeted about it just like briefly. So if you want to go and look at, I'm just, I'm just pointing out that go and check the paper out because the architecture is unique. There is, there is a reason the model is, for its size, very performant. [00:16:30] [00:16:30] Alex Volkov: Yeah, I'm gonna add your tweet. [00:16:31] Alex Volkov: All right, folks, time for us to move to the second thing. [00:16:36] Allen Institute's Olmo 2.0 Release [00:16:36] Alex Volkov: The folks at Allen AI, surprises with another release this week, and they have, as always they do, they say, hey folks, we divide the categories of open source to not open source at all, then somewhat open weights maybe, and then fully open source, the folks who release the checkpoints, the data, the, the training code. [00:16:57] Alex Volkov: I will say this, they used to release Weights [00:17:00] Biases logs as well, and they stopped. So if somebody listens to the show from LMAI, as I know they do, folks, what's up with the Weights Biases logs? We know, and we love them, so please release the Weights Biases logs again. but, they released Olmo 2. [00:17:14] Alex Volkov: Congrats, folks, for releasing Olmo 2. Let me actually do the clap as well. Yay!Olmo 2 is, they claim, is, they claim,the best open, fully open language model to date, and they show this nice graph as well, where, they released two models, Olmo [00:17:30] 2. 7b and Olmo 2. 13b, and they cite multiple things, to, to attribute for the best performance here. [00:17:37] Alex Volkov: specifically the training stability, they ran this for a significant longer before. they cite some of the recipes of. What we talked about last week from TULU3 methodology, the kind of the state of the art post training methodology from TULU3 that we've talked with Nathan last week, specifically the verifiable framework, thing that we've talked about, multiple other technical things like rate [00:18:00] annealing and the data curriculum. [00:18:01] Alex Volkov: And obviously they're focusing on their data. they have their, Ohm's selection of tasks on which they compared these models and,the breakdown that I told you about that they do is the open weights models, partially open models, and then fully open models. So this is the breakdown that they have in the area of open weights models. [00:18:18] Alex Volkov: They have Lama 2. 13b and Mistral 7b, for example, they put Qwen in there as well. So Qwen 2. 57 and 14. And the partially open models, they put Zamba and Stable [00:18:30] LLM. And the fully open models, they put themselves and Olmo and, Ember7B and Olmo2 beats all of that category with some nice, average of stats. [00:18:40] Alex Volkov: they talk about pre training and a bunch of other stuff. and the instruct category specifically with the Tulu kind of,recipes. What else can we say about Olmo? That's very interesting for folks before we jump into Qwen. What else can we say about Olmo? The, oh, the fact that the thing about the fully open source, we always mention this, is the data set. [00:18:59] Alex Volkov: We [00:19:00] always talk about the data, they release all of the data sets, so Olmo mix was released, Dolmino mix was released, the SFT training data, post training data set was released as well. yeah, folks, comments. You can also try this model at playground. lnai. org. I've tried it. It's interesting. it's not look, uh,the best about this is the best among open source. [00:19:21] Alex Volkov: Obviously it's not the best at, generally with closed source data, you can get more significantly better than this. But comments from folks about OMO? [00:19:30] [00:19:30] Wolfram Ravenwolf: Yeah, it's not multilingual, they said that there is only English, but they are working on putting that in, I think, in another version, but, yeah, it's a truly open source model, not just OpenWeights, so a big applause for them, releasing everything, that is a big thing and I always appreciate it. [00:19:46] Wolfram Ravenwolf: Thank you. [00:19:48] Alex Volkov: A hundred percent. All right, folks, it looks like we got Eugene back. Eugene, talk to us about Heimbar. [00:19:54] Eugen Cheugh: Yeah, no, sorry, I was just saying that as someone who works on transformer [00:20:00] alternative,it's actually really awesome to get the data point because we all haven't decided what's the best arrangement, what's the percentage of transformer versus non transformer? [00:20:08] Eugen Cheugh: Is the non transformer layers in the front or the back? It's like you say, the car and the car scenario, it's like electric car, do we even know if we want the electric engine in front or the back? and these are data points that we love to test to just, find out more and it's. And I appreciate what NVIDIA is doing as well and looking forward to more research in this space. [00:20:26] Alex Volkov: Awesome. thanks for joining us and feel free to stay. The more the merrier. This is like a [00:20:30] Thanksgiving kind of pre party for all of us. The more the merrier, folks. If you're listening to this only and you're not like on the live stream, I encourage you to go and check us out because like we're also like showing stuff. [00:20:40] Alex Volkov: We're like showing the papers. We're like, we're waving. We're like showing Turkey, whatever. we're having fun. all right, folks. I think it's time to talk about the main course. We just ate the mashed potatoes. Let's eat the turkey for open source. [00:20:53] Qwen Quill 32B Reasoning Model [00:20:53] Alex Volkov: In this week's Open Source Turkey dinner, the Reasoning Model, the first ever Reasoning Open [00:21:00] Source, we got Qwen Quill, Qwen Quill? [00:21:04] Alex Volkov: Yes, Qwen Quill 32 bit preview, the first open source. Let's go! Let's go! The first open source Reasoning Model from our friends at Qwen. We have Jun Yang here, Jun Yang and Justin Lin, to talk to us about this release. Folks at OpenAI released this, they worked for, the rest of about O1, we released a couple of months ago. [00:21:25] Alex Volkov: Then the folks at DeepSeek released R1, that they just released it, they [00:21:30] promised to give us, maybe at some point. The folks at O1 did not release the reasoning. So, what you see in O1 is the reasoning being obfuscated from us, so we can't actually see how the model reasons. R1 gave us the reasoning itself. [00:21:44] Alex Volkov: But didn't release the model. And so now we have a reasoning model that you can actually download and use. And unlike reflection, this model actually does the thing that it promises to do. Junyang, how did you do it? What did you do? Please give us all the details as much as possible. Please do the announcement yourself. [00:21:58] Alex Volkov: Thank you for joining us. [00:22:00] Junyang from Qwen. [00:22:00] Junyang Lin: Yeah, thanks everyone for the attention and for the appreciation, and I'm Junyang from the Qwen team, and we just released the new model for reasoning, but we just added a tag that it is a preview. Yeah, it is something very experimental, but we would really like to receive some feedback to see how people use it and to see what people think. [00:22:24] Junyang Lin: The internal problems,they really are. Yeah, it is called QUIL. it is [00:22:30] something, very interesting naming,because we like to see that, we first called it like Q1,things like that, but we think it's something too normal and we'd like to see there was something connected with IQ, EQ, then we call it QQ, and then we found out, QWEN with a W there. [00:22:47] Junyang Lin: And we found a very interesting expression because it looks really cute. There is a subculture in China with the text expression to express the feelings. So it is something very interesting. So we [00:23:00] just decided to use the name and for. For the pronunciation, it's just like the word Q, because I combined QW, the pronunciation of QW, with U together, and it's still just cute. [00:23:13] Junyang Lin: Yeah, there's something beside the model, and it is actually a model, which can, And this is the reason before it reaches the final response. If you just try with our demo and you will find that it just keeps talking to itself. And it's something really [00:23:30] surprising for us. If it asks you a question, it just keeps talking to itself to discover more possibilities as possible. [00:23:42] Junyang Lin: And sometimes will lead to some new things. Endless generation. So we have some limitations there. So we mentioned the limitations in the almost the second paragraph, which includes endless generation. But it is very interesting. I [00:24:00] don't say it is a really strong model, something like competitive to O1 or outcompeting R1. [00:24:06] Junyang Lin: It is not Simply like that, we show the benchmark scores, but it is something for your reference to see that, maybe it is at this level, and then if you really check the model performance, when it processes like mathematics and coding problems, it really thinks step by step, and it really discovers more possibilities.[00:24:30] [00:24:30] Junyang Lin: Maybe it is a bit like brute forcing, just like discovering all possibilities. If there are 1 plus 2 is equal to 1, and it discovers a lot of possibilities, but it sometimes finishes,can finish some very difficult tasks. I think, you guys can wait for our more official release, maybe one month or two months later. [00:24:53] Junyang Lin: We'll make sure, And the next one will be much better than this preview one, but you can play with it. It is something really interesting, [00:25:00] very different from the previous models. [00:25:02] Alex Volkov: So first of all, a huge congrats on releasing something that, everybody, it looks like it piqued interest for, tons of folks, absolutely. [00:25:09] Alex Volkov: Second of all, it definitely thinks, it looks like it's,Actually, this seems like this. you can see the thinking, like we're actually showing this right now for folks who are just listening and I'll just read you the actual kind of ice cube question that we have that,somebody places four ice cubes and then at the start of the first minute, and then five ice cubes at the start of the second minute, how many ice cubes there are at the [00:25:30] start of the third minute,we should probably have prepared like a turkey based question,for this one, but basically the answer is zero. [00:25:36] Alex Volkov: Oh, the ice cubes melt within a minute, and the answer is zero, and people know the answer is zero because, ice cubes melt faster than a minute. But, the,LLM starts going into math and s**t, and, just to be clear, O1 answers this question, it understands the answer is zero. Quill does not. [00:25:53] Alex Volkov: But the reasoning process is still pretty cool and compared to like other models like you see you can see it thinking It's let me set up an equation. Oh, [00:26:00] actually, it's not correct Ah, now the equation asking for this and this and this and it goes like This is confusing Let me read the problem again. [00:26:06] Alex Volkov: And so it tries to read the problem again. This feels Not like just spitting tokens. So Junyang, what, could you tell us like what's the difference between this and training at a regular Qwen 2. 5? So as far as I saw, this is based on Qwen 5, correct? [00:26:27] Junyang Lin: Yeah, it is based on Qwen 2. 5 [00:26:30] 32 billion de instruct Model. Yeah, we have tried a lot of options, maybe we will release more technical details later, but I can tell you something that, we mostly simply do some, do some work on the, post training data. Because it is actually based on our previous model, so we did not change the pre training, because we are actually very confident in our pre training, because we have trained it with [00:27:00] a lot of tokens, so there should be some knowledge about reasoning there, and in Qwen 2. [00:27:05] Junyang Lin: 5, we also have some text reasoning, relative data, in the pre training process, so we just try to see that if we can align with the behavior of such, reasoning. So we have some very simple,superfines, fine tuning, and we find that while it can generate things like that, we have done a bit like RL stuff, and we also have done something like, RFT, Rejection, [00:27:30] Finetuning, so we can add more data from it. [00:27:33] Junyang Lin: And there are a lot of techniques, just like self aligned. We use the base language model to use in context learning to build samples for us, to just We've built something like that make the model that can reason and we found that it's really surprising. We did not do very complex stuff, but we find that it has this behavior, but we still find that there is still much room in the reinforcement learning [00:28:00] from human feedback because we found that if you add some RL, you can improve the performance very significantly, so we have some belief that Maybe we, if we have done some more in a process where we're modeling LLM critiques and also things like building more nuanced data for the multi step reasoning, the model will be much better. [00:28:26] Junyang Lin: Yeah. But this one is interesting. You can keep [00:28:30] talking to it. It keeps talking to itself, just talking about some strange thinking and sometimes maybe I'm wrong. I will check the question again and maybe I'm wrong again and then do it again and again. And sometimes it's generally too long because we have some limitations in long text generation. [00:28:49] Junyang Lin: I think All models have this problem, so when it reaches maybe some bound and it will turn into some crazy behaviors, it just never [00:29:00] stops generating. We just mentioned this limitation. Just [00:29:05] Alex Volkov: to make sure folks understand, this is a preview, this is not like an official release. You guys are like, hey, this is a preview, this is a test of you guys. [00:29:12] Alex Volkov: You guys are like trying this out, like folks should give feedback, folks should try it out. Maybe Finetune also on top of it. Yeah. There's definitely we're trying this out. This is [00:29:21] Yam Peleg: it's like chatGPT is a research preview. It's not exactly a preview. It beats the benchmarks on so many problems. [00:29:29] Yam Peleg: We would [00:29:29] Junyang Lin: like [00:29:30] to make it a fun, funny stuff to make people happy. It's now Thanksgiving and people are always expecting models from us. And they're just talking that all out. where's our reasoning model or things like that. Yeah. so we showed this one to you. And. [00:29:48] Alex Volkov: Yeah, Jan Wolfram, folks, comments about the reasoning model from Qwen. [00:29:53] Yam Peleg: Oh, I have a lot of comments. That's a lot. I don't know if you can hear me. Yeah, Jan, [00:30:00] go ahead. [00:30:00] Alex Volkov: There's just a delay, but we're good. [00:30:02] Yam Peleg: Yeah, I just want to say, it's like, uh, CGPT is, uh, is a research preview. It's it's a really good thing. [00:30:10] Yam Peleg: It's a really good model. Seriously. So, I mean, it can be a preview, but it's extremely powerful. How did you guys train this? I mean, what, what, what's the data? How did you generate it? Can you Can I just create data that looks like O1 and Finetune and it's going to work? or, like, give us some details. [00:30:28] Yam Peleg: it's a really hard thing to [00:30:30] do. it's really, really, really successful. Sohow did you make it? [00:30:35] Alex Volkov: Give us some details if you can, I'm saying. if you can. Don't let Yam, don't let Yam go into give some details that you cannot give details. but hey, it looks like we may have lost Junyang for a bit with some connection issues, but while he reconnects, we got Maybe he can't, maybe he can't hear details, so [00:30:52] Wolfram Ravenwolf: They put the plug. [00:30:53] Alex Volkov: and Wolfram, what's your, I saw your take. Let's, meanwhile, let's take a look. You did some testing for this model as well, right? [00:30:59] Wolfram Ravenwolf: [00:31:00] Yeah. And I just ran the, the IceCube prompt and on my run, it got the zero correct. [00:31:04] Wolfram Ravenwolf: So that is a bit of a red flag. Oh, you [00:31:06] Alex Volkov: did get it correct. [00:31:07] Wolfram Ravenwolf: Yeah. it was fun because it wrote, Over 10, 000 characters, but in the end it said, okay, so confusing, they all melted zero. So that worked. But of course you have to run benchmarks multiple times. I did run the MMLU Pro computer science benchmark twice. [00:31:23] Wolfram Ravenwolf: And what is very interesting is, Also here, it generated much more tokens than any other model. The second, highest [00:31:30] number of tokens was GPT 40, the latest one, which was 160, 000 tokens for the whole benchmark. And here we have over 200, 000, 232, 000 tokens it generated. So it took me two and a half hours to run it. [00:31:45] Wolfram Ravenwolf: And, yeah, it's an 8B model, no, a 32B model at 8 bit in my system where I was running it, because I have 48GB VRAM, so you can run it locally and look at it, it's, it's placed above the 405B [00:32:00] Lama 3. 1, it's above the big Mistral, it's above the GBT, JGBT latest, and the GBT 4. 0 from, yeah, the most recent one. [00:32:08] Wolfram Ravenwolf: So just to recap [00:32:09] Alex Volkov: what you're saying. On the MMLU Pro Benchmark, this is a model that you run on your Mac, or whatever PC, and it beats Llama 3. 5, 4 or 5 billion parameter on this benchmark, because it's reasoning and it's smart, it runs for longer, and it uses those test time compute, inference time [00:32:30] compute, Compute, Scaling, Loss that we talked about multiple times. [00:32:33] Alex Volkov: It runs for longer and achieves a better score. This is like the excitement. This is the stuff. so Junyang, now that you're back with us, could you answer, or at least some of Yam's question, if you couldn't hear this before, I will repeat this for you. How? What does the data look like? can you just come up with some O1 stuff? [00:32:51] Alex Volkov: By the way, welcome, welcome Nisten. [00:32:53] Nisten Tahiraj: But I tried it. [00:32:54] Introduction to the New Google Model [00:32:54] Nisten Tahiraj: It got the Martian.Rail Train Launcher, it got it perfectly [00:33:00] on first try, and I saw that it did take it three tries, so I use this as a standard question on most models, is if you're going to launch a train from the highest mountain in the solar system, which is on Mars, and you want to accelerate it at two G's, so Still comfortable. [00:33:21] Nisten Tahiraj: how long would that track need to be in order for you to get to orbital velocity and in order for you to get to, to leave [00:33:30] Mars gravity well? And it's a very good question because there's so many steps to solve it and you can just change it to, you can say 2. 5G and that completely changes the order of the steps for, that the model has to solve. [00:33:42] Alex Volkov: So it's unlikely to be in the training data and it got it perfectly. It's again, it's this one, it's the new Google preview, even Sonnet takes two tries, two or three tries often to get the right answer. So,yeah, the model worked, and I had the same thing as [00:34:00] Wolfram, he did put out a lot of tokens, but again, it's pretty fast to run locally, Folks, it's a good model. It's, it, for a test preview, for something that was released, as a first, open weights reasoning model, we are very impressed. [00:34:14] Model Performance and Availability [00:34:14] Alex Volkov: we're gonna give Junaid, one more, one more attempt here, Junaid, I see you on the spaces. and you're as a speaker, maybe you can unmute there and speak to us through the spaces,while we try this out, I will just tell to folks that like you are, you can download this model. [00:34:27] Alex Volkov: It's already on, OLAMA. [00:34:30] You can just like OLAMA install Quill or QWQ.it's already on OpenRouter as well. You can get it on OpenRouter. So you can like replace. you can replace whatever you use, like OpenAI, you can replace and put this model in there. it's, you can try it out in Hug Face, this is where we tried it just now. [00:34:47] Alex Volkov: And, It's awesome. It's awesome to have this. I'm pretty sure that many people are already like trying different variations and different like fine tunes of this model. And it just like going up from here, like to get a open [00:35:00] model, 32 billion parameters, that gets, what is the score? let me take a look. [00:35:04] Alex Volkov: The score is, I think it gets, 50 on AIME. It's ridiculous. Anybody try this on ARK Challenge, by the way? Do you guys see in your like, like tweets or whatever, the ARK Challenge? Anybody try to run this model on that and try? I would be very interested because that's that's a big prize. It's a very big prize. [00:35:22] Alex Volkov: I'm pretty sure [00:35:22] Eugen Cheugh: someone's trying right now. You shall think that out. [00:35:26] Alex Volkov: I'm pretty sure somebody's trying right now. They could use a [00:35:29] Wolfram Ravenwolf: 72B [00:35:30] version of it and maybe that gets even better. Probably does. [00:35:35] Alex Volkov: Yeah. They're probably training a bigger model than this right now. all right folks. So with this, I think that, we've covered pretty much everything that we wanted to cover with Quill. [00:35:46] Scaling and Model Efficiency [00:35:46] Alex Volkov: and I think, yeah, the one thing that I wanted to show, let me just show this super quick before we move on to the next topic that we have is this, scaling kind of thing. We saw pretty much the same thing. From, from [00:36:00] DeepSeq. And then we saw pretty much the same thing also from OpenAI. The kind of the scaling confirmation, the scaling log confirmation, the next scaling log confirmation, test time compute or inference time compute works. [00:36:11] Alex Volkov: Which basically means that the more thinking, the more tokens, the more time you give these models, the better. to think, the better their answer is. We're getting more and more confirmation for this kind of Noah Brown, I don't know, thesis, that these models actually perform [00:36:30] significantly better when you give them more tokens to think. [00:36:32] Alex Volkov: this is incredible to me. This is like incredible because not only will we have better models with more scale, but Even though some people claim a wall has been hit, no wall has been hit. but also we now have these models that can answer better with more tokens. and this is like another, another confirmation from this. [00:36:51] Alex Volkov: Qwen, Quail32B is now here. You can, you can now run. a, a 4 0 5 B level models, at least on [00:37:00] MMLU Pro,like wolf from here said on your computers. And shout out to our friends from, Alibaba Quinn for releasing these awesome models for us as a Thanksgiving,present. [00:37:10] Alex Volkov: Jang, you're back with us. Let's see. maybe you're back. [00:37:14] Junyang Lin: I don't know if you can hear me. Yes, [00:37:16] Alex Volkov: we can hear you finally, yes. [00:37:18] Junyang Lin: I don't know what happened. [00:37:19] Alex Volkov: it's [00:37:20] Junyang Lin: fine. I [00:37:22] Alex Volkov: think that, let's try this again. maybe last thing as we're going to try. [00:37:27] Discussion on Reasoning Models [00:37:27] Alex Volkov: What, from what you can tell us, [00:37:30] how does the work on this look like? [00:37:34] Alex Volkov: Is a lot of it synthetic? Is a lot of it RL? Could you give us, a little bit of, Give us a hint of what's going to come in the technical release for this. And also what can we look forward to in the upcoming? Are you maybe working on a bigger model? give us some, give us something for Thanksgiving. [00:37:51] Junyang Lin: Oh yeah. for the reasoning steps, I think, the data quality, really matters and, we, we think that, it may split the steps, [00:38:00] more, make it more nuanced. make it more small steps. It can be just, the possible answers, with higher possibility, which means that the machine may think, in a different way from, the human being. [00:38:12] Junyang Lin: The human being may reach the answer very directly, but sometimes, for a reasoning model, it may reason to explore more possibilities. So when you label the data, you should pay attention to, these details and, This is a part of it, and now we only have done some work on mathematics and [00:38:30] coding, and especially mathematics, and I think there's still much room in general knowledge understanding. [00:38:37] Junyang Lin: I found that Wolfram just tested it for the MMU PRO, but we actually did not strengthen its performance for the MMU PRO. this kind of benchmark. So I think for the scientific reasoning, there's still much room for it to do it. And something surprising for us, is that we found that, it sometimes generate more beautiful texts, more [00:39:00] poetic, some, something like that. [00:39:02] Junyang Lin: I don't know why, maybe it is because it reasons. So I think it may encourage creative writing as well. A reasoning model that can encourage creative writing. That would be something very interesting. I also found some cases, in Twitter, that people find that, it sometimes generates, text more beautiful than, Claude's written by someone and created. [00:39:22] Junyang Lin: there's still much room for a reasoning model. Yep. [00:39:25] Alex Volkov: Very interesting. Just to recap, folks found that this model that is [00:39:30] trained for reasoning gives more poetic, writing. that's very interesting. All right, folks, I think it's time for us to move on, but [00:39:37] Wolfram Ravenwolf: just one quick comment. [00:39:39] Multilingual Capabilities of Qwen [00:39:39] Wolfram Ravenwolf: It's also very good in German. I tested it in German as well. So even if it may not be the focus, if you are multilingual or another language, try it. Yeah, [00:39:50] Junyang Lin: that's something not that difficult for us because the Qwen is strong model is multilingual And it is actually I think it is now good at German. [00:39:59] Junyang Lin: Yeah, [00:40:00] [00:40:02] Alex Volkov: Qwen's multilingual is very good at German. [00:40:04] BlueSky hate on OpenSource AI discussion [00:40:04] Alex Volkov: Alright folks, I think that it's time for us to move on a little bit and Now we're moving to less fun, less of a fun conversation, but I think we should talk about this. just a heads up, after this, we're gonna have this week's buzz, but I don't have a category for this. [00:40:19] Alex Volkov: I don't have a category for this, but it must be said. as ThursdAI is all about positivity. We talk about AI every week to highlight the advancement we highlight with positivity we get excited about every new [00:40:30] release every new whatever we also recently and now we have you know we're on youtube as well and the reason it coincided well with some of the folks in the ai community moving over to blue sky let me actually first Say hi to my colleague here, Thomas. [00:40:44] Alex Volkov: I'm going to pull you up on stage as well. welcome Thomas as well. Hey man, welcome. My colleagues for the past year from Weights Biases, welcome as well. You're more than welcome to join us as well, because you're also on BlueSky. And, so a bunch of the community, recently started seeing whether or not there's a [00:41:00] new place over at BlueSky. [00:41:02] Alex Volkov: for the ML community. I saw a bunch of ML people over there as well. I see Wolfram over here has a little butterfly. you all who are joining us from Twitter, or Xspaces, for example, you've probably seen a bunch of your favorite AI folks post just a blue butterfly and maybe follow them towards the other social media platform due to your political preferences, wherever they may be, which is completely fine. [00:41:26] Alex Volkov: That's all good and well and fine. so I started cross posting to both, [00:41:30] and I'll show you how my screen looks like recently. This is how my screen looks like. I scroll here, I scroll on X, and I scroll on blue sky. This is what my life looks like. Yes, I'm on both. because I want to make sure that I'm not missing any of the news. [00:41:43] Alex Volkov: That I want to bring to you, and also Zinova, our friend, right? He posts everywhere, and I see the community bifurcating. I don't like it. But I want to make sure that I'm not missing anything. This is not what I want to talk to you about. Not the bifurcation. I don't mind the bifurcation. We'll figure out something. [00:41:58] Alex Volkov: We're on YouTube as well, [00:42:00] so the folks from BlueSky who don't jump on TwitterX community, they can still join the live chat. What I want to talk to you about is this thing that happened where, a bunch of folks from Hug Face just joined Blue Sky as well, and one of the maybe nicest people in, from the Hug& Face community, Daniel,I'm blanking on his last name, Nisten, maybe you can help me out, Daniel Van Strijn? [00:42:24] Alex Volkov: Daniel Van Strijn?basically, did what he thought was [00:42:30] maybe a cool thing. He compiled the dataset. You guys know, we talk about data and open source and Hug Face as well. This is like in the spirit of the open source community, there's, we talk about open datasets. we, I have a thing here. This is my thing. [00:42:43] Alex Volkov: When we talk about somebody releasing. Open source datasets. We have a thing. We clap, right? and so he compiled, a dataset of 1 million blue sky posts to do some data science. This is like what Hagenfeist, put it on Hagenfeist. just to mention one thing before, [00:43:00] unlike Twitter, which used to be open, then Elon Musk bought it and then closed the API, and then you have to pay 42, 000 a year. [00:43:07] Alex Volkov: 42, 000 a year. Yes, this is the actual price. 42, 000 a year. this is the actual literal price for the API. Unlike Twitter, which used to be free, BlueSky is built on a federated algorithm. There's a firehose of API you can apply to it. And then you can just like drink from this firehose for free. This is like the whole point of the platform. [00:43:27] Alex Volkov: so then you'll connect to this firehose, drink from it and [00:43:30] collect, compile the data set of a 1 million posts, put it up on Hug Face, open source. [00:43:36] Community Reactions and Moderation Issues [00:43:36] Alex Volkov: And then got death threats. Death threats. He got death threats for this thing. People told him that he should kill himself for this act where he compiled data from an open fire hose of data that is open on purpose. [00:43:58] Alex Volkov: What the actual f**k? [00:44:00] And when I saw this, I'm like, what is going on? And in less than 24 hours, I'm going to just show you guys what this looks like. Okay. this is the, this is on the left of my screen and the folks who are not seeing this, you probably, I'm going to, maybe pin. [00:44:13] Alex Volkov: Yeah. let me just do this super quick. So you guys who are just listening to this, please see my pinned tweet, as well. because this is some insanity. Okay. And we have to talk about this because it's not over here. he compiled a 1 million public posts, BlueSky Firehose API, data set. [00:44:27] Alex Volkov: And then, it got extremely [00:44:30] viral to the point where I don't know, it's like almost 500 whatever it's called. And then the amount of hate and vitriol in replies that he got from people in here. Including, yes, including you should kill yourself comments and like death threats and doxing threats, et cetera. [00:44:47] Alex Volkov: many people reached out directly to,HugNFace folks. he became maybe number two most blocked person on the platform as well. and all of this, they, people reached out to the Hug Face community. Basically in less than [00:45:00] 24 hours, he basically said, I removed the BlueSky data from the repo. [00:45:03] Alex Volkov: I wanted to support the tool development for the platform, recognize this approach, violate the principle of transparency and consent. I apologize for this mistake, which, okay, fine. I acknowledge his position. I acknowledge the fact that he works in a,he works in a company and this company has lawyers and those lawyers need to adhere to GDPR laws, et cetera. [00:45:23] Alex Volkov: And many people started saying, Hey, you compiled my personal data without, the right for removal, et cetera, without the due [00:45:30] process, blah, blah, blah. Those lawyers came, there's a whole thing there. And then our friend here, Alpen, who's a researcher, of his own, connected to the same open firehose of data, and collected a dataset of 2 million posts. [00:45:47] Alex Volkov: That's twice as many as Daniel did, and posted that one, and then became the person of the day. Alpen, you want to take it from here? You want to tell us what happened to you since then? What your 24 hours looked [00:46:00] like? [00:46:00] Alpin Dale: yeah, sure. it's been quite the experience being the main character of the day in Blue Sky. [00:46:05] Alpin Dale: And,obviously, I'm not showing my face for very obvious reasons. I have received quite a few threats because, Yeah, unlike Hugging Face employees, I am not beholden to a corporation, so I didn't really back down. And, yeah, I probably received hundreds of death threats and doxxing attempts. [00:46:24] Alpin Dale: so just to reiterate what you said, the Firehose API is completely [00:46:30] open. [00:46:31] Alpin Dale: It is, it's a good analogy with the name because it's like a firehose, anyone can use it. [00:46:35] Legal and Ethical Implications [00:46:35] Alpin Dale: you have they've also,threatened me with litigation, but, I'm not sure if you guys are aware, but there was a court case back in 2022, HiQ Labs versus LinkedIn, where, HiQ Labs was, scraping public, public accounts from LinkedIn and, using it for some commercial purposes, I don't remember. [00:46:54] Alpin Dale: But, They did actually win in court against LinkedIn, and what they were doing was [00:47:00] slightly even more illegal because LinkedIn doesn't have a publicly accessible API, and they have Terms of Services specifically against that sort of scraping, and because of that, the ruling overturned later and they, they lost it, they lost the claim, but it did set a precedent to be had that if the,if the, data published on publicly accessible platforms could be lawfully connected, collected and used, even if terms of service like purported to limit such usage. [00:47:28] Alpin Dale: But I [00:47:30] Never agreed to such a term of service when I started scraping or copying the data from the Firehose API because first, I didn't do any authentication. Second, I didn't provide a username when I did that. So anyone could have done that technically with the AT protocol Python SDK. It's you don't even need to sign in or anything. [00:47:52] Alpin Dale: You just sign in. Connect to the thing and start downloading. [00:47:55] Alex Volkov: Yeah, this is the platform is built on the ethos of the open [00:48:00] web. The open web is you connect and you read the data. This is the ethos of the open web. When this is the ethos of the open web, when you post on this platform, Whether or not the TOS is saying anything, when you don't need to authenticate, the understanding of the people should be, regardless, and I understand some of the anger when the people discover, oh, s**t, my, my thoughts That I posted on this platform so far are being used to like, whatever, train, whatever. [00:48:28] Alex Volkov: I understand some of this, I [00:48:30] don't agree with them, but like I understand, what, how some people may feel when they discover Hey, my thoughts could be collected, blah, blah, blah. and somebody posted like a nice thread. But, the platform is open completely. Going from there to death threats, this is, like, where I draw completely, where I draw my line. [00:48:45] Alex Volkov: Alpen, the next thing that happened is what I want to talk to you about. you're getting death threats, you're getting doxxed attempts. Um,I couldn't find your post today. what happened? [00:48:56] Alpin Dale: for some reason, BlueSky decided to terminate my [00:49:00] account instead of the ones issuing the death threats, very interesting chain of events, but,they claimed that I was engaging in troll behavior, whatever that means. [00:49:10] Alpin Dale: And for that reason, they just, like it wasn't even,due to mass reporting that happens on X. com, right? Specifically emailed me with very, human generated language, where they told me that I was being a troll. I think I posted it on my Twitter account too. And, Yeah, they just assumed I'm trolling, [00:49:30] and what's funny is there's been screenshots floating around of similar mod messages, just giving people a slap on the wrist for much, much worse things, like things we can't even talk about here, right? [00:49:44] Alpin Dale: So very strange, very silly situation overall. And another thing I wanted to mention, a lot of people. We're bringing up the GDPR and all that because of like personally identifiable information, but if you go to the [00:50:00] dataset, all we have is the post text. The timestamp, the author, and the author name is a, it's just a hash, it's not the full author name, and the URI, so there isn't really much to link people to the, to their specific posts, and there isn't even a location tag, so I'm not sure if it fully applies with GDPR, but I'm not a liar anyways, and, The thing is, the data or their posts were published on a platform that is explicitly designed for public [00:50:30] discourse, right? [00:50:31] Alpin Dale: And the decision to share sensitive information on a platform like this lies with the user, not the observer. And we are the observer in this case. And by the very nature of public platforms, Individuals that post like content like this, they have to bear the responsibility that their information is accessible to anyone. [00:50:51] Alpin Dale: And I don't think my dataset like alters this reality because it just consolidates information that was already available for [00:51:00] everyone. And I guess,there were also people who were asking for an opt out option and, the Hugging Face CEO, Clem, also made an issue on the repo about this. And I did provide a very straightforward opt out process, if someone wants to remove that data, they can just submit a pull request. [00:51:18] Alpin Dale: to remove the specific posts that belong to them but alsothey have to accompany it with a proof of authorship they have to prove to me that the post that they're removing is not a [00:51:30] it belongs to them and it's not a malicious request so i guess i've covered all grounds so i'm not sure what the what people are worried about [00:51:38] Alex Volkov: so i uhI'm just showing to the folks who are listening, I'm showing a, an email from,from the moderation team at BlueSky. [00:51:46] Alex Volkov: BlueSky County Control, Alpendale, BlueSky Social was reviewed by BlueSky Content Moderators and assessed as a new account trolling the community, which is a violation of our community guidelines. As a result, the account has been permanently suspended. They didn't even give you the chance to like, hey, delete this and come back to [00:52:00] the platform. [00:52:00] Alex Volkov: Literally permanently suspended. the folks who are like saying, hey, You are going to be,delete this and come back or the folks who are like 13 death threats, are not there. Um,What can we say about this? it's ridiculous. Absolutely. And I, The fact that Hug Face's account, your account, Daniel's account, became the most blocked accounts on the platform in the past 24 hours, more so than some like crazy Manosphere accounts, is just is absolutely insanity. [00:52:28] Alex Volkov: The fact that most of [00:52:30] these anger prone accounts People are like anti AI completely. And the whole issue about like consent, whatever, most of them don't even appear in the dataset, by the way. Like some people checked on the fly, Zeofon and I, like we did some basic checking, many people didn't even appear in the dataset. [00:52:44] Alex Volkov: the fact that the absolute silly fact that the, none of them understand the Barbra Streisand effect on the internet and the fact that there's five datasets right now. Many of them collected the people who reacted to these specific posts and collected the data [00:53:00] set of the people who reacted to these specific posts. [00:53:02] Alex Volkov: And people just don't understand how the internet works. That was just like ridiculous to me. [00:53:07] Moving Forward with Open Source [00:53:07] Alex Volkov: so Alpen, I personally think that you did Many of these people also a very good service as well, because at least some of them now realize how open internet works, despite the being very upset with the fact that this is how the open internet works, at least some of them are now like realizing this. [00:53:23] Alex Volkov: I,I commend you on like the bravery and standing against this like absolute silliness and not backing down. And [00:53:30] Yeah, go ahead. Happy [00:53:31] Alpin Dale: to serve. Yeah, another small thing I wanted to add was, I've received a lot of threats about me getting reported to the EU, but what I find really ironic is that,earlier this year, the EU funded a research for collecting over 200 million blue sky posts with a greater level of detail. [00:53:50] Alpin Dale: So clearly the EU is fine with this, so I don't know what's the problem here, once again. [00:53:58] Alex Volkov: yeah, I saw this. Yeah, there's a way [00:54:00] bigger thing. The last thing I saw about this, and then maybe we'll open up for folks, and then I would love to chat with my friend Thomas, for whom it's late, and I invited him here, and I want to be very mindful of his time as well, so thank you, Thomas, for being patient. [00:54:12] Alex Volkov: The last thing I say about this is that this sucks for open source, from the very reason of, if you're open and public and good hearted about this, Hey folks, here's the data in the open, you can look at this data and you can ask for your s**t to be removed. You get an angry mob of people threatening [00:54:30] death against you and asking your lawyers to like, literally people asking like, was Daniel fired? [00:54:34] Alex Volkov: what the f**k? Meanwhile, this is a open firehose and all of the companies in the world probably already have all this data. I'm pretty sure, OpenAI has been already training on BlueSky. Like, why wouldn't they? It's open. Literally, if you want to train, and Thomas, maybe here is like a little entry to what we're going to talk about. [00:54:50] Alex Volkov: If you want to train a toxicity,thing, There is now a very good place to go to and look at toxicity score or I can show you where you can go [00:55:00] to to train toxicity score. Like, why wouldn't you go and collect this data? It's free, like literally it lies on the internet. [00:55:05] Alex Volkov: Nothing in the TOS, like Alpen said, even I went to the TOS of BlueSky. Literally it says over there, we do not control how other people use your data. Like literally that's what it says on the TOS. So yeah, I'm just like, I'm very frustrated against this. I want to speak out against this, absolutely ridiculous behavior. [00:55:22] Alex Volkov: I don't think that this,okay. So I don't think that the, how the people reacted on the platform speaks against the platform itself. I do think [00:55:30] That the way the moderators, acted out against Alvin's account and the removal of account permanently banned, speaks completely against the platform. [00:55:38] Alex Volkov: This is stupid and we should speak against this, on the platform itself. if we think that this is a place for the community, that's where I stand. And I wanted to share the publicly, super brief comments, folks, and then we'll move on to this week's bus. [00:55:49] Wolfram Ravenwolf: There was a link in his message from the moderators that he can reject it and get a review, appeal, yeah. [00:55:58] Wolfram Ravenwolf: So I hope that, I hope [00:56:00] he gets the appeal through. That is important. Yeah, [00:56:03] Alex Volkov: if you will,please email them with an appeal and, tell them about the multiple death threats that you received and the fact that, you didn't, did not mean to troll. [00:56:12] Wolfram Ravenwolf: I reported every one of those messages, by the way, and anyone who does it is probably a good thing. [00:56:18] Alex Volkov: Nisten, I know you have thoughts on this. I would love to hear. [00:56:22] Nisten Tahiraj: we need to better educate people to not go after the ones on their side. a lot of the open source devs do this stuff [00:56:30] because they want everyone to have, Healthcare robots that no single corporation owns. They make this data public because people want to democratize the technology for everyone. [00:56:41] Nisten Tahiraj: So it's not, it doesn't become like authoritarian and like a single source of control. And, to see that they prioritize, just, people's anger and feelings versus being objective. about it. Whereas, [00:57:00] so in this case, the public forum data set is public domain on purpose. And this is what drew people to the community in the first place, because they felt like Twitter was becoming too political, single sided. [00:57:12] Nisten Tahiraj: And, we didn't like that. And a lot of people moved to, because they saw Blue Sky as a, Much better, democratized alternative to all of this. And,so that's really disappointing because, these are the people on your side and, now the two [00:57:30] nicest, most contributing open source devs that we know, are more hated than, like someone like Andrew Tate. [00:57:37] Nisten Tahiraj: that just makes no sense at all. the, out of the five most blocked accounts Two of them are like the nicest people we know. So what is, something is pretty, pretty off. And, I'm also worried that in the AI community, we are in a bit of a bubble and not quite aware of,what people on our side are being communicated. [00:57:58] Nisten Tahiraj: are being shown how this [00:58:00] stuff works, how open source, works because I'm pretty sure from their point of view, they're like, oh, here's another company just took all of our data and is just gonna train this porn bot with it and there's nothing we can do about it, but it's not like that. [00:58:13] Nisten Tahiraj: Not a single company can own this data. It is public domain. We can't sue anyone else over the data. It's public domain in a public forum. You're supposed to have civil discourse because then the AI can also have civil [00:58:30] discourse and be reasonable and be like aligned to humanity. so now you have a bunch of people just giving, death threats and they're okay because they're just angry. [00:58:40] Nisten Tahiraj: So you can tell someone to go kill themselves just because you're angry. And, yeah, so that's not good. Like they're just not good. you should probably, yeah, anyway, so there is something for us to do as well, like we need to communicate better, what does open source do versus what having a single company. [00:58:58] Nisten Tahiraj: Own all that data and [00:59:00] have it as their property. because I feel like most of the general public doesn't really understand this. [00:59:06] Nisten Tahiraj: yeah, that's it. I was just, okay. Just really quickly. Sorry. I went on too long, but after going through war in the Balkans as a kid, I didn't think people would be getting death threats for an open source dataset. [00:59:17] Nisten Tahiraj: It's this is just completely beyond, It's absolutely unhinged. yeah, this is just completely off. [00:59:23] Wolfram Ravenwolf: Unhinged. Just one thing, those people even think that now the thing is over, so the dataset has been [00:59:30] removed, okay, it's done, but you can get a new one anytime. The platform hasn't changed. They have to realize that. [00:59:37] Alpin Dale: funny it mentioned that because they started blocking me for the explicit reason of, the user started blocking me for the explicit reason of stopping me from scraping their posts, as if I need my account to do that. [00:59:49] Alex Volkov: Yeah, I think that there's, a lot of misunderstanding of, what's actually, happening. [00:59:54] Alex Volkov: And how, which is fine, I completely empathize of people's misunderstanding of [01:00:00] technology, and thus fear, I get this I get the visceral reaction, I get,I don't like multiple other things about this, I don't like the, the absolute, horror mob. And the death threats, I don't like the platform reacting as it did, and like blocking completely, those things don't make sense. [01:00:14] Hey, this is Alex from the editing studio. Super quick, about two hours after we recorded the show, Alpin posted that the moderation team at BlueSky emailed him and his account was in fact reinstated. He didn't ask them to. [01:00:30] They revisited their decision on their own. [01:00:32] So either a public outcry from some individuals on the platform. Hopefully they listened to our show. I doubt they did. Um, but they reversed their decision. So I just wanted to set the record straight about that. He's back on the platform. Anyway, back to the show. [01:00:48] Alex Volkov: Alright folks, unfortunately though, we do have to move on, to better things, and I'll give my other co hosts like a little five, five to seven minutes off, to go take a break. Meanwhile, we're going to discuss [01:01:00] this week's buzz. [01:01:00] This Week's Buzz: Weights & Biases Updates [01:01:00] Alex Volkov: Welcome to this week's buzz, a category at ThursdAI, where I talk about everything that I've learned or everything new that happened in Weights Biases this week. And this week, I have a colleague of mine, Thomas Capelli, [01:01:30] from the AI team at Weights Biases. We're now the AI team. This is new for us. We're Thomas, how, do you want to introduce yourself super brief for folks who've been here before, but maybe one more introduction for folks who don't know who you are. [01:01:43] Thomas Capele: Yeah, I'm Thomas. I work with Alex. I'm in the AI Apply team at Weights Biases. I train models, I play with models on API, and I try to make my way into this LLM landscape that is becoming more and more complex. Try to avoid [01:02:00] getting roasted on the internet. And yeah, trying to learn from everyone. Thank you for the meeting. [01:02:06] Alex Volkov: So you're going by Cape Torch, I'm going to add this as well on X as well. I don't know what you're going off as,on Blue Skies, same Cape Torch. I invited you here, and I think let's do the connection from the previous thing as well. A lot of toxicity we talked about just now, a lot of like toxic comments as well. [01:02:23] Alex Volkov: and we're, we both work at Weights Biases on Weave. Weave is our LLM observability tool. [01:02:30] I showed off Weave multiple times on ThursdAI, but I will be remiss if I don't always remind people, because we have a bunch of new folks who are listening, what Weave is. Weave is an LLM observability tool. So if you're building as a developer, Anything with LLMs on production,you need to know what's going on, what your users are asking your LLM or what your LLM gives as responses, because sometimes imagine that your users are, let's say copy pasting, whatever comments, people just gave [01:03:00] Daniel and Alpin and they pasting it to them to do categorization, for example, and some of these like, Very bad things that we just talked about are getting pasted into the LLM and some of the LLM responses are maybe even worse, right? [01:03:13] Alex Volkov: so maybe your application doesn't handle this. Maybe your application responds even worse and you want to know about this. and, the way to see those, some developers just looks at logs. we have a tool. That is way nicer. And, this is just some of the things it does. but this [01:03:30] tool is called Weave. [01:03:30] Alex Volkov: it, it traces everything that your application gets as an input from users and also outputs. but that's not all it does. So it also allows you to do evaluations. And, recently Thomas and, has been working on, multiple things, specifically around scoring and different things. Thomas,you want to maybe give us a little bit of. [01:03:47] Alex Volkov: Yeah, I think you, [01:03:48] Thomas Capele: you described pretty well. Yeah, as I know, you have showed Weave and the product we have been working for a while, multiple times here, but it's, I would say it's mostly core feature is [01:04:00] actually building apps on top of LLMs and having observability and yeah, standard code, we have unit tests and for LLM based applications, we need like evaluations, actual evaluations on data we have curated. [01:04:13] Thomas Capele: And it's, we have been doing this in the ML world for a while, but as we are merging with the software engineers that. Maybe don't know how to integrate this randomness from the LLMs in the, in their applications. Yeah. you need to actually compute evaluations. And that means gathering [01:04:30] data, still labeling a lot of stuff manually to have high quality signal. [01:04:35] Thomas Capele: And then, yeah, iterating on your prompts and your application that, that's making API calls with scores, with metrics that gives you confidence that we are not like screwing up. And as you said, like I've been working recently on adding, we added a bunch of scores, default scores. We've a couple, yeah, like a month ago with Morgan, we spent like a week building those. [01:04:58] Thomas Capele: and recently we have been like, [01:05:00] yeah, looking at stuff like toxicity and hallucination and yeah, context and bias detection, and there's multiple of them that are LLM powered, like the ones you are showing on the screen right now, like You have an LLM that it's actually prompt in a certain way, and you maybe build a system that requires like a couple of LLM prompt with structured output to actually get the scores you were expecting,and then this thing should be able to give you, yeah, a good value of the [01:05:30] scoring if it's hallucinating, if it's a toxic, actually the mall providers like OpenAI and Mistral and Anthropic, I think have an API exactly for moderation. [01:05:41] Thomas Capele: So yeah, you can use also that and they are actually pretty good and fast and pretty cheap compared to the completion ABA. And we have no, what I've been doing this week and the last couple of weeks where I've been trying to build really high quality, small, non LLM powered scores. So example that you want to create a toxic, [01:06:00] detection system. [01:06:00] Thomas Capele: Yeah. what can you do? Yeah, you could find a small model that it's not an LLM or it was an LLM a couple years ago. Now, like BERT, we don't consider BERT an LLM. [01:06:09] Alex Volkov: Yeah. [01:06:10] Thomas Capele: yeah. I've been fine tuning the BERT task and checking like this new high end phase, small LLM2 models, trying to adapt them to the task. [01:06:18] Thomas Capele: Yeah. yeah, like good challenge, good engineering questions, like creating, there's plenty of high quality data set on HangingFace that people have been creating from multiple places, from Reddit, and [01:06:30] like these have been serving us to actually build this high quality classifiers that are capable of outputting and flagging the content that we're interested in. [01:06:40] Alex Volkov: So here's what I, here's what I'll say for folks, just to like highlight what we're talking about. Weave itself. is a toolkit that you can use for both these things. You can use it for logging and tracing your application, which is what it looks like right now. You basically add these lines to your either Python or JavaScript application, JavaScript type of application, and we will help you track [01:07:00] everything your users do in production. [01:07:01] Alex Volkov: Separately from this, You want to continuously evaluate your application on different set of metrics, for example, or scoring them on different set of metrics to know how your LLM or your prompts are doing, right? So you guys know that, like for example, before on the show we talked about, hey, here's this new model, the, qu quill, for example. [01:07:20] Alex Volkov: And you know that wolf from, for example, tested it on MMU Pro. Those are generic evaluations. MMU Pros, those are evaluations that somebody built specifically for. [01:07:30] Something big. Look, there's a set of questions that somebody builds something big. specific scorers for your type of application, something that you build for your type of applications. [01:07:38] Alex Volkov: and then people asked us as Weights Biases, Hey, okay, you give us a generic toolkit, an unopinionated toolkit, but can you give us some opinion? Can you give us some opinion? And basically this is what like Weave Scorers is. This is like an additional package that you can install if you want to,like additionally, right? [01:07:55] Alex Volkov: Thomas, help me out here, but you can add this. The ones we're [01:07:58] Thomas Capele: building right now, they're not yet [01:08:00] there. They will be probably in a certain future. Yeah. We need to test them correctly. And it's we're an experiment tracking company at the beginning. We're going to like, want to share the full reproducibility. [01:08:10] Thomas Capele: Like this is the data, this is how we train them. there's different versions. It's scoring metrics we get, so you like have confident that they work as expected. [01:08:18] Alex Volkov: So this is to me very interesting, right? So I came in as a previously software developer and now as like an AI evangelist, like I came in from like this side and I meet all these like machine learning engineers, experiment tracking folks who are like, okay, [01:08:30] now that we've built this like LLM based tool, observability tool, many people are asking us to do what Weights Biases does on the model side, on the Weights Biases side. [01:08:37] Alex Volkov: Hey, Use everything from your, immense knowledge of tracking and doing experimentation to bring this over to the LLM side. Okay, now that you have all this data, now that companies are tracking all the data, how to actually, do experimentation on the front side. Thomas, last thing I'll ask you here before I let you go, briefly is about guardrails specifically. [01:08:56] Alex Volkov: So there's this concept that we're going to talk about. We're going to keep talking about this [01:09:00] called guardrails. So we're talking about scorers. Scorers are basically the way to check your application. Just a model. [01:09:05] Understanding Scoring Models [01:09:05] Alex Volkov: Like [01:09:06] Thomas Capele: I would define like score is just a model. It takes an input, produce an output. [01:09:11] Thomas Capele: It could be simple. It could be complicated. Like a scoring, the simplest scores could be accuracy. if the prediction is equal to the label, like a complex score, it could be like an LLM power score that. Check that the context you retrieve from your RAG application, it's not like the response is not [01:09:30] hallucinated or is factually consistent with the original context. [01:09:33] Alex Volkov: So like HallucinationFreeScorer, for example, is one score for folks who are listening. whether or not the response that your RAG application returned, Has hallucinations in it. Or,yeah, it's [01:09:44] Thomas Capele: very it's very detailed. And you will probably need to refine all of this for your specific application because everyone has slightly definition and slightly needs, slightly different needs for their application. [01:09:55] Thomas Capele: So yeah, you may need to tune everything, but this is like a good starting point. [01:09:59] Guardrails in LLM Development [01:09:59] Thomas Capele: [01:10:00] So yeah, I find it very interesting that you mentioned guardrails. I would say like a guardrail is. Also a model that predicts, but it's need to be really fast and it needs to be, it needs to take actions, maybe change the output, like any of these scores don't change your output. [01:10:19] Thomas Capele: Like they will. Computer score, but they will not change the output. if you have IPAI's guardrail, it should, I don't know, redact stuff that [01:10:30] shouldn't pass. So it should change the output, like the payload you are getting from the API. So like guardrails are more like online, and these are more like, offline. [01:10:41] Alex Volkov: So that's a good boundary to do. And I think we'll end here, but this is basically an exception for going forward, folks. I will tell you about guardrails specifically. [01:10:48] Guardrails in Production [01:10:48] Alex Volkov: It's something we're getting into, and I'm going to keep talking about guardrails specifically, because I think that this is a very important piece of developing LLMs in production. [01:10:57] Alex Volkov: How are you making sure that the [01:11:00] model that you have online is also behaving within a set of boundaries that you set for your LLM? obviously we know that the big companies, they have their guardrails in place. We know because, for example, when you, talk with, advanced voice mode, for example, you ask it to sing, it doesn't sing. [01:11:14] Alex Volkov: there's a boundary that they set in place. when you develop with your LLMs in production, your guardrails, the only way to build them in is in by prompting for example there's other ways to do them and we are building some of those ways or we're building tools for you to build some of those ways [01:11:30] and like thomas said one of those guardrails are changing the output or like building ways to prevent from some of the output from happening like PII for example or there's like toxicity detection and other stuff like this so we will Talking more about guardrails, Thomas with this, I want to thank you for coming out to the show today and helping us with scores and discussing about Weave as well. [01:11:50] Alex Volkov: And, I appreciate the time here, folks. You can find Thomas on, X and on, and on BlueSky, under CapeTorch. Thomas is a machine learning engineer and, [01:12:00] developer AI engineer as well. Does a lot of great content, Thomas. Thank you for coming up. I appreciate you. He also does amazing cooking as well. [01:12:06] Alex Volkov: Follow him for some amazing gnocchi as well. Thanks, Thomas. Thomas, thank you. Folks, this has been this week's Bugs, and now we're back. Good job being here. See you guys. See you, man. And now we're back to big companies and APIs.[01:12:30] [01:12:33] Alex Volkov: All right. All right. All right. We are back from this week's buzz, folks. Hopefully, you learned a little bit about scores and guardrails. We're going to keep talking about guardrails, but now we have to move on because we have a bunch of stuff to talk about specifically around big companies and APIs, which had A bunch of stuff this week as well. [01:12:51] OpenAI Leak Incident [01:12:51] Alex Volkov: I wanna talk about, the leak. You guys wanna talk about the leak, this week? open the eye had a big, oh my God. Oops. Something big [01:13:00] happened. but nothing actually big happened, but look to some extent, this was a little bit big. at some point, this week, a frustrated participant in the open ai, how should I say, test. [01:13:12] Alex Volkov: Program for Sora decided to quote unquote leak Sora and posted a hug and face space where you could go and say, Hey, I am,I want this and this. And you would see a Sora video generated and, yeah, we can actually show some videos. I think, this is not against any [01:13:30] TOS, I believe. and, Yeah, this wasn't actually a leak. What do you guys think? did you happen to participate in the bonanza of, Sora videos, Wolfram or Yam? Did you see this? [01:13:40] Wolfram Ravenwolf: I saw it, but I didn't, try to go to the link. [01:13:43] Alex Volkov: No. [01:13:44] Sora Video Leak Reactions [01:13:44] Alex Volkov: so basically, some very frustrated person from,the creative minds behind Sora behind the scenes, decided to like, Leak SORA, the leak wasn't actually the model leak like we would consider a model [01:14:00] leak. [01:14:00] Alex Volkov: the leak was basically a hug and face application with a request to a SORA API with just the keys hidden behind the hug and face. we're showing some of the videos. I'm going to also add this to,to the top of the space for you guys as well. The videos look pretty good, but many of the folks who commented, they basically said that, compared to when Sora just was announced, where all of [01:14:30] us were mind blown completely, now the videos, when you compare them to something like Kling, or some of, Runway videos, they're pretty much on the same level. [01:14:41] Alex Volkov: And, I, they look good. They still look very good. look at this animation for example. It looks very good still And apparently there's like a version of Sora called Sora Turbo. So these videos are like fairly quick, but Like folks are not as mind blown [01:15:00] as before yeah Some of the physics looks a little bit better than Kling etc, but it feels like we've moved onand this is something that I want to talk to you guys like super quick. [01:15:09] Alex Volkov: we're following every week, right? So we get adapted every week, like every,the Reasoning Model Formula 1 blew us away. And then R1 came out and now we run this on our models due to Quill. So we're used to getting adapted to this. the video world caught up to Sora like super quick. [01:15:24] Alex Volkov: Now we can run these models. There's one open source one like every week. These videos [01:15:30] don't blow us away as they used to anymore and,why isn't OpenAI releasing this at this point is unclear because if you could say before, elections, you could,you can put down Trump and Kamala Harris in there, Now, what's the reason for not releasing this and not giving us this thing? [01:15:47] Alex Volkov: anyway, yeah, this video is pretty cool. There's one video with, a zoom in and somebody eating a burger. yeah, leak, not leak, I don't know, but, thoughts about the sourcling? What do you guys think about the videos and, the non releasing, things? Folks, I want to ask, Nisten, [01:16:00] what do you think about those videos? [01:16:01] Alex Volkov: Do you have a chance to look at them? [01:16:03] Nisten Tahiraj: I was going to say, by the way, I was going to say the exact same thing you did, that it's just been so long now, what, a few, a couple of months since they announced it? I think it's more than [01:16:14] Alex Volkov: a couple of months, I think half a year, maybe, yeah. [01:16:16] Nisten Tahiraj: Yeah, it's over half a year that so much happened that we're no longer impressed. [01:16:22] Nisten Tahiraj: And I'm just trying to be mindful of that, that things are still moving fast. And, they haven't stopped [01:16:30] moving. Like we've seen a whole bunch of models start to get close to this now. it's still better, I would say it's still better than most of, what's come out in the last six months. but,yeah, we're getting pretty close. [01:16:41] Nisten Tahiraj: I think they haven't released it mainly because of, weaponized litigation,that's the main thing. [01:16:45] Alpin Dale: Yeah. [01:16:45] Nisten Tahiraj: Holding them back and, uh.yeah, companies in other countries don't have that problem as, as much, so they were able to, to advance more, like while still being respectful tothe brands and [01:17:00] stuff, but, yeah, I think the main reason is, people are just going to try and nitpick any kind of,of, attack vector to, to, to sue them. [01:17:08] Nisten Tahiraj: For it. So that's probably why [01:17:10] Alex Volkov: Yeah. Everything open AI will Yeah. Will get attacked. That I fully agree with you on this. Yeah. speaking of, let's see, do we have anything else from OpenAI? I don't believe so. Yeah. the other one thing that I wanted to show super quick is that the new Chad GPT now is also y I'm gonna show this super quick on the thing, is also now [01:17:30] supporting cursor. [01:17:31] Alex Volkov: So now, the NutriGPT app is supporting the Cursor app, so now you can ask what I'm working on in Cursor, and if you hover this, you can actually see all of my, including env, You can actually see my secrets, but, you can ask it, you can ask it about the open, open queries. And why would I, if I have Cursor? [01:17:49] Alex Volkov: That's the question, right? Cursor supports O1, because, I have unlimited O1 queries on ChaiGPTN, whereas I have like fairly limited, queries for O1 in Cursor. and generally [01:18:00] That's been pretty good. That's been pretty cool. You can ask it about the stuff that you have open. There's a shortcut I think it's option shift 1 on Windows and you can enable this and basically you then start chatting With the open interface in the one. [01:18:13] Alex Volkov: We tested this a couple of weeks ago if you guys remember and I found it super fun. I don't know if you guys used it since then or for those who use the Mac version of, of ChatGPT. I find it really fun. So folks in the audience, if you're using the macOS app and you are connecting this to Cursor or to the terminal, for [01:18:30] example. [01:18:30] Alex Volkov: Unfortunately, I use the warp terminal and they still don't have warp. they have iTerm here and other things. if you use PyCharm or other, JetBrains, they also started supporting those.but I specifically use Courser and now there's a support for Courser, supports for Windsurf, which is another thing that we didn't cover yet. [01:18:46] Alex Volkov: And I heard amazing things. And I hope, hopefully over the Thanksgiving break, I will have to, have a chance to use Windsurf. but yeah, this is from, OpenAI and we were waiting for some more news from OpenAI, but we didn't get one. So hopefully the folks at [01:19:00] OpenAI will get a Thanksgiving break. [01:19:02] Alex Volkov: Just a small reminder. I looked a year ago, if you guys remember the Thanksgiving episode we had a year ago. We were discussing the control alt deletemen weekend where Sam Altman was fired and then rehired. That was the Thanksgiving episode of last year. You guys remember this? last year we discussed how Sam Altman, and Greg Brockman were shanked and, the coup from Ilya. [01:19:26] Alex Volkov: You guys remember? It's been a year. It's been a year since then. This was the [01:19:30] Thanksgiving last year. and, yeah, it's been a year since then. which by the way. Next week is the one, the two year anniversary of JGPT as well. So we probably should prepare something for that. so that's on the OpenAI News. [01:19:43] Alex Volkov: let's super quick talk about this.at some point There's this, the sayings from Space Uncle is, they need to be studied in an encyclopedia. somebody tweeted, I don't understand how game developers and game journalists got so ideologically captured. [01:20:00] Elon Musk tweeted and said, Too many game studios are owned by massive corporations. [01:20:03] Alex Volkov: XAI is going to start an AI game studio to make games great again.and I'm like, and please unmute if you're muted and laughing, because I want to hear, and I want the audience to hear that both PicoCreator and Nisten are just like laughing out loud at this. It's XAI with all of their like 200, H200, 200, 000 H200s, like the best, the fastest ever growing massive [01:20:30] Memphis, super cluster, they're going to build games like, what are they really going to actually. [01:20:34] Alex Volkov: Have a gaming studio in there. Like we know he is, Elon is a, I don't know the best Diablo game player in the world right now. I don't know how the f**k [01:20:43] Nisten Tahiraj: he's, he is fourth or 20th or, [01:20:45] Alex Volkov: yeah, he was 20. I think he's at some point he got number one recently, or something. I, we know, we all know he's a gamer. [01:20:51] Alex Volkov: Kudos. I really, I'm not making this up. Like I'm really have no idea how the f**k you can be like the best Diablo player in the world doing all these other stuff [01:21:00] and. I get the sentiment of okay, let's make games. Great. Turning in the eye company, the games company, how the,what? [01:21:08] Alex Volkov: Ah, I just want to turn to this. [01:21:12] Eugen Cheugh: I love most. It's just a massive corporation, XAI with billions of dollars of funding. It's going to be not a messy corporation. [01:21:23] Alex Volkov: Yeah, this is not necessarily AI related necessarily,we are expecting big things from XAI, specifically around GROK [01:21:30] 3. [01:21:30] Alex Volkov: Hopefully December, that's the date that they've given us. They have a hundred thousand H100s turning away and building something. We know that this was like announced. we know that Elon promises and doesn't deliver on time, but delivers at some point anyway. We know that they have. very good folks behind the scenes. [01:21:47] Alex Volkov: We know this, we've seen this before. We know that, infrastructure is something they're building out. They're building out enterprise infrastructure for APIs. we've seen the X, AI API layer building out. We've seen the kind of the [01:22:00] X,infrastructure. Sorry, enterprise infrastructure for, the building layer. [01:22:03] Alex Volkov: We've seen all this, getting prepared. Like we've talked about this, we're getting to the point where X is going to be another player, competing another player versus Google, OpenAI, Anthropic, etc. GRUG3 is going to be something significant to contend with. and like the amount of GPUs are there. [01:22:22] Alex Volkov: It's just is this a sidetrack? this is basically my question. [01:22:25] Nisten Tahiraj: it, so Uncle Elon tends to be like very [01:22:30] impulsive as we've seen, so if he spends a lot of time on something he's gonna start getting obsessed with it. So there's that. In order to have a gaming service, you will need a lot of GPUs, and I'm pretty sure at this point, if they want to do cloud gaming or streaming, they probably have more GPUs than PlayStation. [01:22:49] Nisten Tahiraj: they might actually just have more right now. they're like, we can probably Support that, and so much for the Department of Government Efficiency, now we're all [01:23:00] just going to be streaming games. [01:23:05] Nisten Tahiraj: But there is, there's also Another lining to this is for, for a while, for the last 10 years, there was an article about 10 years ago that the E3, I don't think that's a thing anymore, but the E3 gaming conference had a SpaceX booth over a decade ago and SpaceX was actively recruiting for the E3. I think to quote, it was, programmers of physics engine, and the [01:23:30] rumors were that they were going after the ones who made the Steam Havoc 2, like the one in Portal, and the ones that worked on the, Unreal Tournament physics engine. [01:23:40] Nisten Tahiraj: And this was over 10 years ago, and those people, those programmers, were recruited by SpaceX. like, when you see, the Falcon Heavy, 2, 3, 4 rockets, just like Go dance in midair and land like they're in a video game is because, the people that made the simulation very likely worked on game engines. [01:23:58] Nisten Tahiraj: So it might be [01:24:00] a hiring angle from him, or it might just be Angelino playing a lot of games and he just wants to know. there is an angle [01:24:07] Alex Volkov: for gaming as a playground for training. Like a GI, whatever, like open AI obviously had, like trained robots in this area. we saw many papers for like agents running wild in a game constrained environments. [01:24:19] Alex Volkov: There, there could be an angle there for sure. I just, this doesn't feel like, this feels like an impulsive, hey. make f*****g games great again. [01:24:26] Anthropic's Model Context Protocol [01:24:26] Alex Volkov: Alright, moving on, unless we have another comment here, moving on to [01:24:30] I really wanted to discuss the, super briefly the, Model Context Protocol from Anthropic. [01:24:36] Alex Volkov: because this kind of blew up, but it's not ready yet. I saw a comment from Simon Wilson, you guys know Simon Wilson, the friend of the pod, he'd been here multiple times. basically he covered this. super quick, Anthropic released this new protocol, which they hope to standardize and by standardize, they mean Hey, let's get around this. [01:24:53] Alex Volkov: Okay. So let's talk about a standard in the industry right now, the OpenAI SDK for Python. That's a [01:25:00] standard way to interact with LLMs. Pretty much everybody supports this, including Gemini. I think the only one who doesn't support this is Anthropic actually. So in Python, if you want to interact with any LLM, Literally any provider in LLM, including OpenRouter, like Google, OpenAI themselves, like pretty much everyone else, like including together, like all of the, all of those, you can replace one line of code in the OpenAI API, OpenAI Python SDK, where you just put a different URL in there, and then this is the standard way to talk to [01:25:30] LLMs. [01:25:30] Alex Volkov: I think for TypeScript, JavaScript, it's pretty much the same.so it looks like Anthropic is trying to do something like this to standardize around how LLMs are connecting with other applications. So right now, just a minute before I showed you how ChatGPT is connecting to like a VS Code for something. [01:25:49] Alex Volkov: They built those integrations themselves. So you would install a specific extension in VS Code in etc. And that extension That they've built [01:26:00] talks to the ChatGPT app on the Mac OS that they've built and they build this connection for you. This is not what Anthropic wants to do. Anthropic wants to create a protocol that like developers, other developers can build on their own to allow the LLM to talk to any application and you as a developer, I as a developer, other developers can build those Communication layers, and then whatever LLM, in this case, this is the Anthropic, Claude desktop app, this could be the JGPT app, could be the Gemini GPT app, [01:26:30] Gemini app, et cetera, could talk to other applications. [01:26:32] Alex Volkov: What those other applications are? Anything. Anything on your desktop, anything. At all. So they build this kind of a first standard, communication via JSON RPC. And I think they're buildingother ways, and other servers. I think this is a way to summarize this, basically. [01:26:50] Alex Volkov: this is a open preview. Nisten, you want to take another crack at trying to recap this? Or Yam or Wolfram, you guys want to? You want to give me your thoughts on this super quick? As far as I understand from [01:27:00] Simon, this is like still robust and still in,in, in flux. [01:27:03] Nisten Tahiraj: I think this might end up being a much bigger deal than we, we first expect, because it is an interoperability layer, and as a developer, you will have to learn this. [01:27:15] Nisten Tahiraj: it is annoying at the moment that, While proposing a standard, Anthropic is not showing willingness to abide by one, which most people chose, and even Google was forced to support the OpenAI standard. if you [01:27:30] want people to come with your standard, to abide by your standard, you also have to show willingness to abide by others. [01:27:36] Nisten Tahiraj: that's not going to work here until Anthropic Just supports a plug and play OpenAI API, so I just put their models in, but that aside. The criticism aside,this is pretty, pretty important. So I've been doing some of this stuff and just trying to do it with basic JSON. So I think that's,it's very good. [01:27:55] Nisten Tahiraj: And yeah, it's pretty hard to know, am I on Mac? Am I on Linux? Am I on a phone? [01:28:00] What's the LLM going to talk to? what does this app even want me to do? Do I have to emulate this on the screen and then click on it? Can't it just give me a JSON so that I can click on it so it's a lot easier for me? [01:28:11] Nisten Tahiraj: And this will also apply to websites and, and web apps after to you. Offer some kind of a JSON RPC. An RPC is just like an API for people. It's just an application programming interface. It's something you query, like you write a curl to this [01:28:30] IP and here's my API key and give me, or here I'm going to give you this stuff and give me this stuff. [01:28:37] Nisten Tahiraj: From the database or whatever. So this is this actually extremely important because you can apply to, to web apps as well. And it's a way to manage multiple sessions. So I think it's a pretty big deal, even though I am. No. And anthropic, it this,yeah. I think that this is gonna become much, much more important because it saves a lot of bandwidth.[01:29:00] [01:29:00] Nisten Tahiraj: Instead of you having to run a visual language model to show the whole screen, to run it on an emulator, to have to click on it and move around. And it's so compute intensive. It's can you just gimme like a adjacent API, so I can just like, [01:29:13] Alex Volkov: yeah, do [01:29:13] Nisten Tahiraj: a constraint output to adjacent and just output three tokens. [01:29:16] Nisten Tahiraj: Be done with the whole thing. so yeah. Yeah, it's, I think it'll become a big deal. [01:29:21] Alex Volkov: So in the spirit of, of the holiday, thank you and tropic for trying to standardize things, standardize, often, sometimes it's annoying, but often leads to good things as [01:29:30] well. folks, should try out the MMCP and definitely give them feedback. [01:29:34] Alex Volkov: but yeah, they should also abide by some standards as well. It looks like the industry is standardizing around the. OpenAI SDK, and they maybe should also, it would help. [01:29:43] Wolfram Ravenwolf: It's a new thing that they are doing because, so far we usually had the LLM as a part in an agent pipeline where you have, another process called the LLM with some input. [01:29:52] Wolfram Ravenwolf: And here we have the LLM going out to get. The input itself. So I think that is also in the agent context, very important and [01:30:00] more integration is always better, but of course it's a new thing. We have to develop all those servers as I call it. So a lot of reinventing the wheel, I guess we'll see if it can really persevere. [01:30:12] Alex Volkov: Yeah, one example that they highlight, and Simon talked about this as well, is that if you have a database, a SQLite database that sits on your computer,the way to have So you guys know we, we talked about tool use, for example,via API, those models can Get respond with some, some idea of how to use your [01:30:30] tools. [01:30:30] Alex Volkov: And you, as a developer, you are in charge of using those tools. You basically get in response a structure of a function call. And you're like, okay, now I have to take this and then go to an external tool and use this. This is connecting this piece forward. This is basically. Allowing this LLM to then actually go and actually use this tool. [01:30:48] Alex Volkov: Basically like getting a step forward. And one, one example that they're showing is a connecting to a database, allowing this LLM to connect to a database via a sq lite like MCP server. the model compute [01:31:00] protocol server. cps, sorry. yeah. So connecting via this MCP server,you basically allowing LM to read from this database. [01:31:08] Alex Volkov: Itself without like returning a call. And then you are in charge as a developer to go and do the call return it responses. so basically trying to, allow LLMs to connect to different services. Yeah. And this, I think I agree with you with more work in here. this could be big. [01:31:24] Nisten Tahiraj: It could literally make like over a thousand times more compute efficient to automate [01:31:30] something on a screen. Because instead of using a visual language model frame by frame, you can just have a JSON. [01:31:37] Alex Volkov: Let's talk about Like literally [01:31:38] Nisten Tahiraj: over a thousand times. Let's compute to do it. So I'm going to, I'm going to take a longer look at it as well. [01:31:46] Alex Volkov: speaking of automating things on the screen, [01:31:48] H runner from H the french AI company [01:31:48] Alex Volkov: let's talk about the next thing that we want to talk about, H company AI. This is the next thing in big companies and APIs, H company from. France, this is another big company. So [01:32:00] we know Mistral is from France. some, DeepMind, some folks is from France as well. [01:32:04] Alex Volkov: there's also FAIR in France from Meta. now France is positioning themselves to be one big kind of hub from AI as well. H Company. raised, fundraised, I think, 250 I have in my notes. Yeah, 220, one of the biggest, seed rounds. 220 million dollars, one of the biggest ones in, in the history of, French seed rounds, a while ago. [01:32:24] Alex Volkov: And they just showcased their Runner H. Their Runner H [01:32:30] is, they're competing with Claude on speed of computer use. I apologize for this. Let's take a look at how fast they're claiming they're opening a browser, going to recipes and providing recipes for something. On the right, we have Claude, Computer Use. [01:32:46] Alex Volkov: Claude is basically, hey, open the browser. On the left, they already pulled up a browser and already extracting data. So basically they're claiming A speed up of maybe two to three times over cloud computer use. [01:33:00] And they're basically showing while Claude still pulls up the Firefox browser, they have already completed the task, extracted the data and already responded to the user. [01:33:09] Alex Volkov: they're showing steps by steps comparison, which I don't think is necessarily in, Apple's to Apple's comparison. I don't think it's necessarily fair, but. There's a big but here, big French, but, I don't know how to say, sorry, Nisten, I don't know how to say but in French, but there's a big one. [01:33:25] Alex Volkov: Their models, as far as I could see, and I did some research, they have [01:33:30] a, they say this runner age thing that they have is powered by a specialized LLM, specialized optimist for function calling for 2 billion params. So whatever we see on the left is not like Claude, which whatever, we don't know the size of Claude, this is like a 2 billion parameter model. [01:33:45] Alex Volkov: and, integrates in the VLM of a 3 billion parameter model to see, understand, interact with the graphical and text interface. Let's look at another example here. they're basically browsing the web and like doing extraction and yeah, I don't think you guys can see it. maybe like this.[01:34:00] [01:34:02] Alex Volkov: It's literally, they're going to Wolfram Alpha and extracting and doing this task. They're basically asking Wolfram Alpha to do a task. So it's not like they're just reading from things. They're finding input and they're like plugging things in there and like responding, reading from the output from Wolfram Alpha as well. [01:34:18] Alex Volkov: this runnerage thing actually performs tasks on the web. Extracts information back way faster than Claude Computerius, which Claude Computerius, let's give it its place. We were very excited when it came [01:34:30] out, and it does very well for, for just an adjustment of Claude. and they are showing immense differences in five steps, and we're still waiting for Claude Computerius to like, Try to figure this out. [01:34:42] Alex Volkov: So did you [01:34:43] Nisten Tahiraj: say it's a separate to be model? And then there's another? That's what I found [01:34:48] Alex Volkov: from them. Yeah. Yeah. They said that they have, let me see if I can find the previous announcement. Yeah. Yeah. [01:34:54] Wolfram Ravenwolf: The previous announcement [01:34:56] Alex Volkov: that they have, that we missed from last week, Introducing Studio, a [01:35:00] automations at scale, run or age the most advanced agent to date. [01:35:04] Alex Volkov: That's what they said last year. Powered by specialized LLM, highly optimized for function calling, 2 billion parameters. It also integrates a specialized VLM, 3 billion parameters, to perceive, understand, and interact with graphical and text elements. Delivers the state of the art on the public WebVoyager framework. [01:35:20] Alex Volkov: And this is the graph that they have. WebVoyager, they have Runner H01. At 66 percent maybe? And, and [01:35:30] then, Cloud Computer Use at 52 percent and Agent E, I don't know where it is, it's like here. Yeah, so like the size of it is what's the most impressive part. [01:35:41] Nisten Tahiraj: Yeah, I'd say this is impressive. as to what they're doing. [01:35:44] Nisten Tahiraj: we can guess what model they're using, but it doesn't matter all that much. I just wanna say that it's not an apples to apples comparison with cloud because cloud is an entire OS in there and you can use whatever you want. It can use blender, it can, [01:36:00] you can run a virtual box of Windows 95 and it will use that as well. [01:36:04] Eugen Cheugh: so the, yeah, it's not. That's not a pure example, whereas in this one, I'm assuming they do need access to the document object model, the DOM of the website, to be able to navigate it, but The results do indeed seem impressive, and it's at a size that you can run it, you can run on your own, Yeah, because if you're measuring steps and speed, actually, I think, Anthropic Cloud should, probably, partner with [01:36:30] a company like Browserbase, and just, do a demo, and then see how close they get instead. It will skip literally the first eight steps or something like that, which is all just the OS booted up. [01:36:40] Alex Volkov: Yeah, this is why I didn't love the comparison specifically, you guys are right, it's running a janky Docker with Firefox, and by the time, it loads Firefox, these guys already loaded the website, so it's not like necessarily apples to apples, but it looks like those models are tiny compared to Claude, and also, they talk about, It's beyond [01:37:00] optimizing agent performance, they're like, they have, optimizing web interactions. [01:37:05] Alex Volkov: they engineered Runaways to handle any web interactions. Advancing towards one singular mission, automating the web, so they're focused on web. So Eugene, like what you're talking about, like browser based with computer use, it looks like this is their focus, whereas computer use is, for computer use, generic. [01:37:22] Alex Volkov: This is like their focus for web interactions. I guess what I'm saying is it's exciting. they raised a boatload of money, the folks behind [01:37:30] there, they seem like very,adept, I, I know they're based in France, Wolfram. I don't know, Wolfram, you're asking if, if I'm sure they're France. [01:37:36] Alex Volkov: yeah, they're based in France, and, Yeah, we'll see. They're waitlisted. I haven't tested them out. I know that some folks collaborated on them already and posted some threads. so we'll hopefully, we'll see if I get access to this. I'll tell you guys and we'll play with it. Absolutely. definitely exciting in the world of agents. [01:37:54] Alex Volkov: I think this is it from big companies. Folks, what do you think? Anything else From big companies, nothing from Google after the [01:38:00] releases of last week where they reclaimed the throne. Hopefully they're getting their deserved breaks and and relaxing. I don't think this week was fairly chill. [01:38:07] Alex Volkov: Probably the next week they're going to come back with a vengeance. Next week there's like the AWS re invent. Maybe Amazon will come with something. And then the week after RPS. Maybe some folks are waiting for that. I think that this is it in big companies. Let's move on to vision and video. [01:38:22] Alex Volkov: And then, Oh, I think we're at two minutes. Folks, I think we're at time. I think we're at time. I got too excited that we have like a bunch of other things to talk about. [01:38:30] So let me maybe recap on our Thanksgiving super quick. the stuff that we didn't get to just to like to recap super quick. we didn't get to, but just to tell you guys what else we didn't get to, runway specifically. [01:38:41] Alex Volkov: Oh yeah, I just, I have to show this. not to talk about this. Just just visually show this beautiful thing. If I can click this. If I can click this thing, yeah, Runway introduced an expand feature, if you guys haven't seen this, it's really fun to just watch. Let me just mute this. basically, [01:39:00] what you see above and below, Runway introduced an expand feature where you take a video and you give it, give this model and the model tries to predict it. [01:39:08] Alex Volkov: in different ratio, what's above and below this video. So basically, if you give a video in the widescreen format, 16 by nine, and you could try to turn it into a 19 by six format. And so the model will try to fill in the frames. The general video model tries to fill in the frames of what's above and below. [01:39:25] Alex Volkov: So what we're looking at in the video on the screen is like a Lord of the [01:39:30] Rings scene where Legolas rides one of those like elephant looking thingies. Basically, the model tries to fill in the, just the frames from above and below. It just looks a little bit creepy. it's funny looking, but it's like looks, interesting. [01:39:45] Alex Volkov: so this is like one expand feature and the other one is they released an actual image model from Runway, which kind of looks interesting. it's called a frames and it's specific for image generation for [01:40:00] world building. and Confi UI desktop launched. I think that's pretty much it. [01:40:05] Thanksgiving Reflections and Thanks [01:40:05] Alex Volkov: Folks, it's time to say thanks, because it's Thanksgiving. I just wanted to start, but I wanted to hear from you as well. My biggest thanks this year goes to, first of all, everybody who tunes in to ThursdAI. Everybody who comes into the community, everybody who provides comments and shares with their friends and, and listens and,The second huge thanks goes to all of you. [01:40:26] Alex Volkov: My co hosts here, Wolfram, Yam, Nisten, LDJ, Junyang [01:40:30] who joined us, Eugene who joined us as well. Zafari who joined us from time to time, like a bunch of other folks. huge thanks to you for being here from like week to week for more than like almost, we're coming up on two years. And I think the thirst, the third thanks goes to Jensen for the GPUs that he provided for all of us to enjoy those like amazing corn coffee of AI features around the world. [01:40:51] Alex Volkov: just, yeah, just open up the mics and feel free to, to join the festivities even though I don't know any of you celebrate [01:41:00] Thanksgiving unnecessarily. But yeah, what are you guys thankful for? before we wrap up, let's do the Thanksgiving roundup. [01:41:07] Eugen Cheugh: I'm giving thanks to open models. [01:41:08] Eugen Cheugh: let's go. Yeah, no, proving that you do not need billions of dollars to catch up with GPT 4 despite what the big labs will say. The open teams, keep going, keep bringing open models to the masses. [01:41:25] Nisten Tahiraj: Yeah, We had Thanksgiving last month in Canada. I would like to [01:41:30] give thanks to two particular creators, mahi and, tki. each have over a thousand models and, quants that they release. And, and also Mr. Der Backer, probably mispronounced that was, over 5,000, quantization of models. [01:41:48] Nisten Tahiraj: this is the stuff I use every day in tell. Other people. So whenever something new comes up, I almost always expect them to have a good, well done quantization ready for [01:42:00] others to use. and they just do this as volunteers. I don't even think they're part of the, none of them are part of like even a big corporation, or have high salaries. [01:42:08] Nisten Tahiraj: They literally just do it as volunteers. Yeah, I want to give thanks to those people in particular, and everybody else here, and all the people on Discord as well, who sit around and help you correct stuff, but yeah, that's it for me. [01:42:27] Wolfram Ravenwolf: Okay, I have three. The first [01:42:30] is to Alex for the podcast, because it's amazing to be here. [01:42:34] Wolfram Ravenwolf: It's my way to keep up with the stuff I can't keep up with. So thank you for having me. Thank you for doing this. Thank you very much. And the second is to the whole community of AI people, especially those who release all these stuff in the open. But everybody who contributes, everybody who does a good thing about it, I think it is furthering humanity. [01:42:53] Wolfram Ravenwolf: So thanks for that. And the third is a thanks to every reasonable person who is not, Going to insights or stuff, [01:43:00] but it's open minded and, seeing that we are all in the same boat and we are all trying to make the world a better place in our different ways. And for being, accepting and understanding of this. [01:43:11] Wolfram Ravenwolf: In this times, I think it's very important to keep an open mind. [01:43:16] Nisten Tahiraj: Oh yeah, just really quickly to add on, the biggest thanks I think for this year goes to the DeepSeek and Qwent teams for just caring. up everybody [01:43:30] else when we stalled on progress they kept it up to like actually democratize the models for you to actually have this piece of artificial intelligence and own it and control it and be loyal and make it loyal to you yeah. [01:43:47] Nisten Tahiraj: they actually enable people to, to run fully local models. Like 90% of what I use every day is just completely open source. Now, honestly, it w it, I wouldn't, it would not be there if it wasn't for them. It would probably maybe be like [01:44:00] 20, 30%. So,yeah, they, they really carried, like that's a gaming term, like someone who. [01:44:06] Nisten Tahiraj: Carries the team. They have really carried, so yeah. [01:44:11] Alex Volkov: Jan, go [01:44:14] Yam Peleg: ahead. To Jensen for the GPUs, and [01:44:17] Alex Volkov: to everybody [01:44:18] Yam Peleg: else I'm hugging face. Especially people collecting and releasing datasets. I think they're not getting enough credits because you can't just use the dataset [01:44:30] without training a model. There is an effort. [01:44:31] Yam Peleg: to, until you appreciate the dataset, but, they make it possible, everything else. [01:44:39] Alex Volkov: Last thing that I have to, and this is not because I have to, but honestly, folks, huge thanks to Weights Biases for all of this, honestly, I wouldn't have been able to do this as my job without a few folks in Weights Biases, so thank you Morgan, thank you Lavanya, thank you a bunch of folks in Weights Biases. [01:44:55] Alex Volkov: who realized this could be a part of my actual day to day and bringing you news from Weights [01:45:00] Biases, but also promoting some of the stuff. many of the labs, if not most of the labs that we talk about, are using Weights Biases to bring us the open source, but also the closed source LLMs in the world. [01:45:10] Alex Volkov: I couldn't be More happy and be in a better place to bring you the news, but also participate behind the scenes in building some of these things. With that, thank you to all of you. Hopefully you go and enjoy some of the rest of your holiday. Those of you who celebrate, those of you who don't celebrate, this is, I think the first Thursday in a while that we didn't have any breaking news. [01:45:27] Alex Volkov: I'm itching to press it anyway, but we didn't [01:45:30] have any breaking news, but hopefully we'll have some next week. There could be some news next week. We'll see. With that, thank everybody who joins, go and enjoy the rest of your day. And we'll see you here next week as always. Bye everyone. Bye bye. [01:45:43] Alex Volkov: Bye bye. Bye bye. Bye bye. Bye bye. And we have [01:46:00] a
This is a public episode. If you'd like to discuss this with other subscribers or get access to bonus episodes, visit sub.thursdai.news/subscribe | |||
06 Dec 2024 | 📆 ThursdAI - Dec 5 - OpenAI o1 & o1 pro, Tencent HY-Video, FishSpeech 1.5, Google GENIE2, Weave in GA & more AI news | 01:31:37 | |
Well well well, December is finally here, we're about to close out this year (and have just flew by the second anniversary of chatGPT 🎂) and it seems that all of the AI labs want to give us X-mas presents to play with over the holidays! Look, I keep saying this, but weeks are getting crazier and crazier, this week we got the cheapest and the most expensive AI offerings all at once (the cheapest from Amazon and the most expensive from OpenAI), 2 new open weights models that beat commercial offerings, a diffusion model that predicts the weather and 2 world building models, oh and 2 decentralized fully open sourced LLMs were trained across the world LIVE and finished training. I said... crazy week! And for W&B, this week started with Weave launching finally in GA 🎉, which I personally was looking forward for (read more below)! TL;DR Highlights * OpenAI O1 & Pro Tier: O1 is out of preview, now smarter, faster, multimodal, and integrated into ChatGPT. For heavy usage, ChatGPT Pro ($200/month) offers unlimited calls and O1 Pro Mode for harder reasoning tasks. * Video & Audio Open Source Explosion: Tencent’s HYVideo outperforms Runway and Luma, bringing high-quality video generation to open source. Fishspeech 1.5 challenges top TTS providers, making near-human voice available for free research. * Open Source Decentralization: Nous Research’s DiStRo (15B) and Prime Intellect’s INTELLECT-1 (10B) prove you can train giant LLMs across decentralized nodes globally. Performance is on par with centralized setups. * Google’s Genie 2 & WorldLabs: Generating fully interactive 3D worlds from a single image, pushing boundaries in embodied AI and simulation. Google’s GenCast also sets a new standard in weather prediction, beating supercomputers in accuracy and speed. * Amazon’s Nova FMs: Cheap, scalable LLMs with huge context and global language coverage. Perfect for cost-conscious enterprise tasks, though not top on performance. * 🎉 Weave by W&B: Now in GA, it’s your dashboard and tool suite for building, monitoring, and scaling GenAI apps. Get Started with 1 line of code OpenAI’s 12 Days of Shipping: O1 & ChatGPT Pro The biggest splash this week came from OpenAI. They’re kicking off “12 days of launches,” and Day 1 brought the long-awaited full version of o1. The main complaint about o1 for many people is how slow it was! Well, now it’s not only smarter but significantly faster (60% faster than preview!), and officially multimodal: it can see images and text together. Better yet, OpenAI introduced a new ChatGPT Pro tier at $200/month. It offers unlimited usage of o1, advanced voice mode, and something called o1 pro mode — where o1 thinks even harder and longer about your hardest math, coding, or science problems. For power users—maybe data scientists, engineers, or hardcore coders—this might be a no-brainer. For others, 200 bucks might be steep, but hey, someone’s gotta pay for those GPUs. Given that OpenAI recently confirmed that there are now 300 Million monthly active users on the platform, and many of my friends already upgraded, this is for sure going to boost the bottom line at OpenAI! Quoting Sam Altman from the stream, “This is for the power users who push the model to its limits every day.” For those who complained o1 took forever just to say “hi,” rejoice: trivial requests will now be answered quickly, while super-hard tasks get that legendary deep reasoning including a new progress bar and a notification when a task is complete. Friend of the pod Ray Fernando gave pro a prompt that took 7 minutes to think through! I've tested the new o1 myself, and while I've gotten dangerously close to my 50 messages per week quota, I've gotten some incredible results already, and very fast as well. This ice-cubes question failed o1-preview and o1-mini and it took both of them significantly longer, and it took just 4 seconds for o1. Open Source LLMs: Decentralization & Transparent Reasoning Nous Research DiStRo & DeMo Optimizer We’ve talked about decentralized training before, but the folks at Nous Research are making it a reality at scale. This week, Nous Research wrapped up the training of a new 15B-parameter LLM—codename “Psyche”—using a fully decentralized approach called “Nous DiStRo.” Picture a massive AI model trained not in a single data center, but across GPU nodes scattered around the globe. According to Alex Volkov (host of ThursdAI), “This is crazy: they’re literally training a 15B param model using GPUs from multiple companies and individuals, and it’s working as well as centralized runs.” The key to this success is “DeMo” (Decoupled Momentum Optimization), a paper co-authored by none other than Diederik Kingma (yes, the Kingma behind Adam optimizer and VAEs). DeMo drastically reduces communication overhead and still maintains stability and speed. The training loss curve they’ve shown looks just as good as a normal centralized run, proving that decentralized training isn’t just a pipe dream. The code and paper are open source, and soon we’ll have the fully trained Psyche model. It’s a huge step toward democratizing large-scale AI—no more waiting around for Big Tech to drop their weights. Instead, we can all chip in and train together. Prime Intellect INTELLECT-1 10B: Another Decentralized Triumph But wait, there’s more! Prime Intellect also finished training their 10B model, INTELLECT-1, using a similar decentralized setup. INTELLECT-1 was trained with a custom framework that reduces inter-GPU communication by 400x. It’s essentially a global team effort, with nodes from all over the world contributing compute cycles. The result? A model hitting performance similar to older Meta models like Llama 2—but fully decentralized. Ruliad DeepThought 8B: Reasoning You Can Actually See If that’s not enough, we’ve got yet another open-source reasoning model: Ruliad’s DeepThought 8B. This 8B parameter model (finetuned from LLaMA-3.1) from friends of the show FarEl, Alpin and Sentdex 👏 Ruliad’s DeepThought attempts to match or exceed performance of much larger models in reasoning tasks (beating several 72B param models while being 8B itself) is very impressive. Google is firing on all cylinders this week Google didn't stay quiet this week as well, and while we all wait for the Gemini team to release the next Gemini after the myriad of very good experimental models recently, we've gotten some very amazing things this week. Google’s PaliGemma 2 - finetunable SOTA VLM using Gemma PaliGemma v2, a new vision-language family of models (3B, 10B and 33B) for 224px, 448px, 896px resolutions are a suite of base models, that include image segmentation and detection capabilities and are great at OCR which make them very versatile for fine-tuning on specific tasks. They claim to achieve SOTA on chemical formula recognition, music score recognition, spatial reasoning, and chest X-ray report generation! Google GenCast SOTA weather prediction with... diffusion!? More impressively, Google DeepMind released GenCast, a diffusion-based model that beats the state-of-the-art ENS system in 97% of weather predictions. Did we say weather predictions? Yup. Generative AI is now better at weather forecasting than dedicated physics based deterministic algorithms running on supercomputers. Gencast can predict 15 days in advance in just 8 minutes on a single TPU v5, instead of hours on a monstrous cluster. This is mind-blowing. As Yam said on the show, “Predicting the world is crazy hard” and now diffusion models handle it with ease. W&B Weave: Observability, Evaluation and Guardrails now in GA Speaking of building and monitoring GenAI apps, we at Weights & Biases (the sponsor of ThursdAI) announced that Weave is now GA. Weave is a developer tool for evaluating, visualizing, and debugging LLM calls in production. If you’re building GenAI apps—like a coding agent or a tool that processes thousands of user requests—Weave helps you track costs, latency, and quality systematically. We showcased two internal apps: Open UI (a website builder from a prompt) and Winston (an AI agent that checks emails, Slack, and more). Both rely on Weave to iterate, tune prompts, measure user feedback, and ensure stable performance. With O1 and other advanced models coming to APIs soon, tools like Weave will be crucial to keep those applications under control. If you follow this newsletter and develop with LLMs, now is a great way to give Weave a try Open Source Audio & Video: Challenging Proprietary Models Tencent’s HY Video: Beating Runway & Luma in Open Source Tencent came out swinging with their open-source model, HYVideo. It’s a video model that generates incredible realistic footage, camera cuts, and even audio—yep, Foley and lip-synced character speech. Just a single model doing text-to-video, image-to-video, puppeteering, and more. It even outperforms closed-source giants like Runway Gen 3 and Luma 1.6 on over 1,500 prompts. This is the kind of thing we dreamed about when we first heard of video diffusion models. Now it’s here, open-sourced, ready for tinkering. “It’s near SORA-level,” as I mentioned, referencing OpenAI’s yet-to-be-fully-released SORA model. The future of generative video just got more accessible, and competitors should be sweating right now. We may just get SORA as one of the 12 days of OpenAI releases! FishSpeech 1.5: Open Source TTS Rivaling the Big Guns Not just video—audio too. FishSpeech 1.5 is a multilingual, zero-shot voice cloning model that ranks #2 overall on TTS benchmarks, just behind 11 Labs. This is a 500M-parameter model, trained on a million hours of audio, achieving near-human quality, fast inference, and open for research. This puts high-quality text-to-speech capabilities in the open-source community’s hands. You can now run a top-tier TTS system locally, clone voices, and generate speech in multiple languages with low latency. No more relying solely on closed APIs. This is how open-source chases—and often catches—commercial leaders. If you’ve been longing for near-instant voice cloning on your own hardware, this is the model to go play with! Creating World Models: Genie 2 & WorldLabs Fei Fei Li’s WorldLabs: Images to 3D Worlds WorldLabs, founded by Dr. Fei Fei Li, showcased a mind-boggling demo: turning a single image into a walkable 3D environment. Imagine you take a snapshot of a landscape, load it into their system, and now you can literally walk around inside that image as if it were a scene in a video game. “I can literally use WASD keys and move around,” Alex commented, clearly impressed. It’s not perfect fidelity yet, but it’s a huge leap toward generating immersive 3D worlds on the fly. These tools could revolutionize virtual reality, gaming, and simulation training. WorldLabs’ approach is still in early stages, but what they demonstrated is nothing short of remarkable. Google’s Genie 2: Playable Worlds from a Single Image If WorldLabs’s 3D environment wasn’t enough, Google dropped Genie 2. Take an image generated by Imagen 3, feed it into Genie 2, and you get a playable world lasting up to a minute. Your character can run, objects have physics, and the environment is consistent enough that if you leave an area and return, it’s still there. As I said on the pod, “It looks like a bit of Doom, but generated from a single static image. Insane!” The model simulates complex interactions—think water flowing, balloons bursting—and even supports long-horizon memory. This could be a goldmine for AI-based game development, rapid prototyping, or embodied agent training. Amazon’s Nova: Cheaper LLMs, Not Better LLMs Amazon is also throwing their hat in the ring with the Nova series of foundational models. They’ve got variants like Nova Micro, Lite, Pro, and even a Premier tier coming in 2025. The catch? Performance is kind of “meh” compared to Anthropic or OpenAI’s top models, but Amazon is aiming to be the cheapest high-quality LLM among the big players. With a context window of up to 300K tokens and 200+ language coverage, Nova could find a niche, especially for those who want to pay less per million tokens. Nova Micro costs around 3.5 cents per million input tokens and 14 cents per million output tokens—making it dirt cheap to process massive amounts of data. Although not a top performer, Amazon’s approach is: “We may not be best, but we’re really cheap and we scale like crazy.” Given Amazon’s infrastructure, this could be compelling for enterprises looking for cost-effective large-scale solutions. Phew, this was a LONG week with a LOT of AI drops, and NGL, o1 actually helped me a bit for this newsletter, I wonder if you can spot the places where o1 wrote some of the text using a the transcription of the show and the outline as guidelines and the previous newsletter as a tone guide and where I wrote it myself? Next week, NEURIPS 2024, the biggest ML conference in the world, I'm going to be live streaming from there, so if you're at the conference, come by booth #404 and say hi! I'm sure there will be a TON of new AI updates next week as well! Show Notes & Links TL;DR of all topics covered: * This weeks Buzz * Weights & Biases announces Weave is now in GA 🎉(wandb.me/tryweave) * Tracing LLM calls * Evaluation & Playground * Human Feedback integration * Scoring & Guardrails (in preview) * Open Source LLMs * DiStRo & DeMo from NousResearch - decentralized DiStRo 15B run (X, watch live, Paper) * Prime Intellect - INTELLECT-1 10B decentralized LLM (Blog, watch) * Ruliad DeepThoutght 8B - Transparent reasoning model (LLaMA-3.1) w/ test-time compute scaling (X, HF, Try It) * Google GenCast - diffusion model SOTA weather prediction (Blog) * Google open sources PaliGemma 2 (X, Blog) * Big CO LLMs + APIs * Amazon announces Nova series of FM at AWS (X) * Google GENIE 2 creates playable worlds from a picture! (Blog) * OpenAI 12 days started with o1 full and o1 pro and pro tier $200/mo (X, Blog) * Vision & Video * Tencent open sources HY Video - beating Luma & Runway (Blog, Github, Paper, HF) * Runway video keyframing prototype (X) * Voice & Audio * FishSpeech V1.5 - multilingual, zero-shot instant voice cloning, low-latency, open text to speech model (X, Try It) * Eleven labs - real time audio agents builder (X) This is a public episode. If you'd like to discuss this with other subscribers or get access to bonus episodes, visit sub.thursdai.news/subscribe | |||
13 Dec 2024 | 📆 ThursdAI - Dec 12 - unprecedented AI week - SORA, Gemini 2.0 Flash, Apple Intelligence, LLama 3.3, NeurIPS Drama & more AI news | 01:39:04 | |
Hey folks, Alex here, writing this from the beautiful Vancouver BC, Canada. I'm here for NeurIPS 2024, the biggest ML conferences of the year, and let me tell you, this was one hell of a week to not be glued to the screen. After last week banger week, with OpenAI kicking off their 12 days of releases, with releasing o1 full and pro mode during ThursdAI, things went parabolic. It seems that all the AI labs decided to just dump EVERYTHING they have before the holidays? 🎅 A day after our show, on Friday, Google announced a new Gemini 1206 that became the #1 leading model on LMarena and Meta released LLama 3.3, then on Saturday Xai releases their new image model code named Aurora. On a regular week, the above Fri-Sun news would be enough for a full 2 hour ThursdAI show on it's own, but not this week, this week this was barely a 15 minute segment 😅 because so MUCH happened starting Monday, we were barely able to catch our breath, so lets dive into it! As always, the TL;DR and full show notes at the end 👇 and this newsletter is sponsored by W&B Weave, if you're building with LLMs in production, and want to switch to the new Gemini 2.0 today, how will you know if your app is not going to degrade? Weave is the best way! Give it a try for free. Gemini 2.0 Flash - a new gold standard of fast multimodal LLMs Google has absolutely taken the crown away from OpenAI with Gemini 2.0 believe it or not this week with this incredible release. All of us on the show were in agreement that this is a phenomenal release from Google for the 1 year anniversary of Gemini. Gemini 2.0 Flash is beating Pro 002 and Flash 002 on all benchmarks, while being 2x faster than Pro, having 1M context window, and being fully multimodal! Multimodality on input and output This model was announced to be fully multimodal on inputs AND outputs, which means in can natively understand text, images, audio, video, documents and output text, text + images and audio (so it can speak!). Some of these capabilities are restricted for beta users for now, but we know they exists. If you remember project Astra, this is what powers that project. In fact, we had Matt Wolfe join the show, and he demoed had early access to Project Astra and demoed it live on the show (see above) which is powered by Gemini 2.0 Flash. The most amazing thing is, this functionality, that was just 8 months ago, presented to us in Google IO, in a premium Booth experience, is now available to all, in Google AI studio, for free! Really, you can try out right now, yourself at https://aistudio.google.com/live but here's a demo of it, helping me proof read this exact paragraph by watching the screen and talking me through it. Performance out of the box This model beating Sonnet 3.5 on Swe-bench Verified completely blew away the narrative on my timeline, nobody was ready for that. This is a flash model, that's outperforming o1 on code!? So having a Flash MMIO model with 1M context that is accessible via with real time streaming option available via APIs from the release time is honestly quite amazing to begin with, not to mention that during the preview phase, this is currently free, but if we consider the previous prices of Flash, this model is going to considerably undercut the market on price/performance/speed matrix. You can see why this release is taking the crown this week. 👏 Agentic is coming with Project Mariner An additional thing that was announced by Google is an Agentic approach of theirs is project Mariner, which is an agent in the form of a Chrome extension completing webtasks, breaking SOTA on the WebVoyager with 83.5% score with a single agent setup. We've seen agents attempts from Adept to Claude Computer User to Runner H, but this breaking SOTA from Google seems very promising. Can't wait to give this a try. OpenAI gives us SORA, Vision and other stuff from the bag of goodies Ok so now let's talk about the second winner of this week, OpenAI amazing stream of innovations, which would have taken the crown, if not for, well... ☝️ SORA is finally here (for those who got in) Open AI has FINALLY released SORA, their long promised text to video and image to video (and video to video) model (nee, world simulator) to general availability, including a new website - sora.com and a completely amazing UI to come with it. SORA can generate images of various quality from 480p up to 1080p and up to 20 seconds long, and they promised that those will be generating fast, as what they released is actually SORA turbo! (apparently SORA 2 is already in the works and will be even more amazing, more on this later) New accounts paused for now OpenAI seemed to have severely underestimated how many people would like to generate the 50 images per month allowed on the plus account (pro account gets you 10x more for $200 + longer durations whatever that means), and since the time of writing these words on ThursdAI afternoon, I still am not able to create a sora.com account and try out SORA myself (as I was boarding a plane when they launched it) SORA magical UI I've invited one of my favorite video creators, Blaine Brown to the show, who does incredible video experiments, that always go viral, and had time to play with SORA to tell us what he thinks both from a video perspective and from a interface perspective. Blaine had a great take that we all collectively got so much HYPE over the past 8 months of getting teased, that many folks expected SORA to just be an incredible text to video 1 prompt to video generator and it's not that really, in fact, if you just send prompts, it's more like a slot machine (which is also confirmed by another friend of the pod Bilawal) But the magic starts to come when the additional tools like blend are taken into play. One example that Blaine talked about is the Remix feature, where you can Remix videos and adjust the remix strength (Strong, Mild) Another amazing insight Blaine shared is a that SORA can be used by fusing two videos that were not even generated with SORA, but SORA is being used as a creative tool to combine them into one. And lastly, just like Midjourney (and StableDiffusion before that), SORA has a featured and a recent wall of video generations, that show you videos and prompts that others used to create those videos with, for inspiration and learning, so you can remix those videos and learn to prompt better + there are prompting extension tools that OpenAI has built in. One more thing.. this model thinks I love this discovery and wanted to share this with you, the prompt is "A man smiles to the camera, then holds up a sign. On the sign, there is only a single digit number (the number of 'r's in 'strawberry')" Advanced Voice mode now with Video! I personally have been waiting for Voice mode with Video for such a long time, since the that day in the spring, where the first demo of advanced voice mode talked to an OpenAI employee called Rocky, in a very flirty voice, that in no way resembled Scarlet Johannson, and told him to run a comb through his hair. Well today OpenAI have finally announced that they are rolling out this option soon to everyone, and in chatGPT, we'll all going to have the camera button, and be able to show chatGPT what we're seeing via camera or the screen of our phone and have it have the context. If you're feeling a bit of a deja-vu, yes, this is very similar to what Google just launched (for free mind you) with Gemini 2.0 just yesterday in AI studio, and via APIs as well. This is an incredible feature, it will not only see your webcam, it will also see your IOS screen, so you’d be able to reason about an email with it, or other things, I honestly can’t wait to have it already! They also announced Santa mode, which is also super cool, tho I don’t quite know how to .. tell my kids about it? Do I… tell them this IS Santa? Do I tell them this is an AI pretending to be Santa? Where is the lie end exactly? And in one of his funniest jailbreaks (and maybe one of the toughest ones) Pliny the liberator just posted a Santa jailbreak that will definitely make you giggle (and him get Coal this X-mas) The other stuff (with 6 days to go) OpenAI has 12 days of releases, and the other amazing things we got obviously got overshadowed but they are still cool, Canvas can now run code and have custom GPTs, GPT in Apple Intelligence is now widely supported with the public release of iOS 18.2 and they have announced fine tuning with reinforcement learning, allowing to funetune o1-mini to outperform o1 on specific tasks with a few examples. There's 6 more work days to go, and they promised to "end with a bang" so... we'll keep you updated! This weeks Buzz - Guard Rail Genie Alright, it's time for "This Week's Buzz," our weekly segment brought to you by Weights & Biases! This week I hosted Soumik Rakshit from the Weights and Biases AI Team (The team I'm also on btw!). Soumik gave us a deep dive into Guardrails, our new set of features in Weave for ensuring reliability in GenAI production! Guardrails serve as a "safety net" for your LLM powered applications, filtering out inputs or llm responses that trigger a certain criteria or boundary. Types of guardrails include prompt injection attacks, PII leakage, jailbreaking attempts and toxic language as well, but can also include a competitor mention, or selling a product at $0 or a policy your company doesn't have. As part of developing the guardrails Soumik also developed and open sourced an app to test prompts against those guardrails "Guardrails Genie" and we're going to host it to allow folks to test their prompts against our guardrails, and also are developing it and the guardrails in the open so please check out our Github Apple iOS 18.2 Apple Intelligence + ChatGPT integration Apple Intelligence is finally here, you can download it if you have iPhone 15 pro and pro Max and iPhone 16 all series. If you have one of those phones, you will get the following new additional features that have been in Beta for a while, features like Image Playground with the ability to create images based on your face or faces that you have stored in your photo library. You can also create GenMoji and those are actually pretty cool! The highlight and the connection with OpenAI's release is of course the ChatGPT integration, where in if Siri is too dumdum to answer any real AI questions, and let's face it, it's most of the time, a user will get a button and chatGPT will take over upon user approval. This will not require an account! Grok New Image Generation Codename "Aurora" Oh, Space Uncle is back at it again! The team at XAI launched its image generation model with the codename "Aurora" and briefly made it public only to pull it and launch it again (this time, the model is simply "Grok"). Apparently, they've trained their own image model from scratch in like three months but they pulled it back a day after, I think because they forgot to add watermarks 😅 but it's still unconfirmed why the removal occurred in the first place, Regardless of the reason, many folks, such as Wolfram, found it was not on the same level as their Flux integration. It is really good at realism and faces, and is really unrestricted in terms of generating celebrities or TV shows form the 90's or cartoons. They really don't care about copyright. The model however does appear to generate fairly realistic images with its autoregressive model approach where generation occurs pixel-by-pixel instead of diffusion. But as I said on the show "It's really hard to get a good sense for the community vibe about anything that Elon Musk does because there's so much d**k riding on X for Elon Musk..." Many folks post only positive things on anything X or Xai does in the hopes that space uncle will notice them or reposts them, it's really hard to get an honest "vibes check" on Xai stuff. All jokes aside we'll hopefully have some better comparisons on sites such as image LmArena who just today launched ImgArena but until that day comes we'll just have to wait and see what other new iterations and announcements follow! NeurIPS Drama: Best Paper Controversy! Now, no week in AI would be complete without a little drama. This time around it’s with the biggest machine learning engineering conference of the year, NeurIPS. This year's "Best Paper" award went to a work entitled Visual Auto Aggressive Modeling (VAR). This paper apparently introduced an innovative way to outperform traditional diffusion models when it comes to image generation! Great right? well not so fast because here’s where things get spicy. This is where Keyu Tian comes in, the main author of this work and a former intern of ByteDance who are getting their fair share of the benefits with their co-signing on the paper but their lawsuit may derail its future. ByteDance is currently suing Keyu Tian for a whopping one million dollars citing alleged sabotage on the work in a coordinated series of events that compromised other colleagues work. Specifically, according to some reports "He modified source code to changes random seeds and optimizes which, uh, lead to disrupting training processes...Security attacks. He gained unauthorized access to the system. Login backdoors to checkpoints allowing him to launch automated attacks that interrupted processes to colleagues training jobs." Basically, they believe that he "gained unauthorized access to the system" and hacked other systems. Now the paper is legit and it introduces potentially very innovative solutions but we have an ongoing legal situation. Also to note is despite firing him they did not withdraw the paper which could speak volumes to its future! As always, if it bleeds, it leads and drama is usually at the top of the trends, so definitely a story that will stay in everyone's mind when they look back at NeurIPS this year. Phew.. what a week folks, what a week! I think with 6 more days of OpenAI gifts, there's going to be plenty more to come next week, so share this newsletter with a friend or two, and if you found this useful, consider subscribing to our other channels as well and checkout Weave if you've building with GenAI, it's really helpful! TL;DR and show notes * Meta llama 3.3 (X, Model Card) * OpenAI 12 days of Gifts (Blog) * Apple ios 18.2 - Image Playground, GenMoji, ChatGPT integration (X) * 🔥 Google Gemini 2.0 Flash - the new gold standard of LLMs (X, AI Studio) * Google Project Mariner - Agent that browsers for you (X) * This weeks Buzz - chat with Soumik Rakshit from AI Team at W&B (Github) * NeurIPS Drama - Best Paper Controversy - VAR author is sued by ByteDance (X, Blog) * Xai new image generation codename Aurora (Blog) * Cognition launched Devin AI developer assistant - $500/mo * LMArena launches txt2img Arena for Diffusion models (X) This is a public episode. If you'd like to discuss this with other subscribers or get access to bonus episodes, visit sub.thursdai.news/subscribe | |||
20 Dec 2024 | 🎄ThursdAI - Dec19 - o1 vs gemini reasoning, VEO vs SORA, and holiday season full of AI surprises | 01:35:38 | |
For the full show notes and links visit https://sub.thursdai.news 🔗 Subscribe to our show on Spotify: https://thursdai.news/spotify 🔗 Apple: https://thursdai.news/apple Ho, ho, holy moly, folks! Alex here, coming to you live from a world where AI updates are dropping faster than Santa down a chimney! 🎅 It's been another absolutely BANANAS week in the AI world, and if you thought last week was wild, and we're due for a break, buckle up, because this one's a freakin' rollercoaster! 🎢 In this episode of ThursdAI, we dive deep into the recent innovations from OpenAI, including their 1-800 ChatGPT phone service and new advancements in voice mode and API functionalities. We discuss the latest updates on O1 model capabilities, including Reasoning Effort settings, and highlight the introduction of WebRTC support by OpenAI. Additionally, we explore the groundbreaking VEO2 model from Google, the generative physics engine Genesis, and new developments in open source models like Cohere's Command R7b. We also provide practical insights on using tools like Weights & Biases for evaluating AI models and share tips on leveraging GitHub Gigi. Tune in for a comprehensive overview of the latest in AI technology and innovation. 00:00 Introduction and OpenAI's 12 Days of Releases 00:48 Advanced Voice Mode and Public Reactions 01:57 Celebrating Tech Innovations 02:24 Exciting New Features in AVMs 03:08 TLDR - ThursdAI December 19 12:58 Voice and Audio Innovations 14:29 AI Art, Diffusion, and 3D 16:51 Breaking News: Google Gemini 2.0 23:10 Meta Apollo 7b Revisited 33:44 Google's Sora and Veo2 34:12 Introduction to Veo2 and Sora 34:59 First Impressions of Veo2 35:49 Comparing Veo2 and Sora 37:09 Sora's Unique Features 38:03 Google's MVP Approach 43:07 OpenAI's Latest Releases 44:48 Exploring OpenAI's 1-800 CHAT GPT 47:18 OpenAI's Fine-Tuning with DPO 48:15 OpenAI's Mini Dev Day Announcements 49:08 Evaluating OpenAI's O1 Model 54:39 Weights & Biases Evaluation Tool - Weave 01:03:52 ArcAGI and O1 Performance 01:06:47 Introduction and Technical Issues 01:06:51 Efforts on Desktop Apps 01:07:16 ChatGPT Desktop App Features 01:07:25 Working with Apps and Warp Integration 01:08:38 Programming with ChatGPT in IDEs 01:08:44 Discussion on Warp and Other Tools 01:10:37 GitHub GG Project 01:14:47 OpenAI Announcements and WebRTC 01:24:45 Modern BERT and Smaller Models 01:27:37 Genesis: Generative Physics Engine 01:33:12 Closing Remarks and Holiday Wishes Here’s a talking podcast host speaking excitedly about his show TL;DR - Show notes and Links * Open Source LLMs * Meta Apollo 7B – LMM w/ SOTA video understanding (Page, HF) * Microsoft Phi-4 – 14B SLM (Blog, Paper) * Cohere Command R 7B – (Blog) * Falcon 3 – series of models (X, HF, web) * IBM updates Granite 3.1 + embedding models (HF, Embedding) * Big CO LLMs + APIs * OpenAI releases new o1 + API access (X) * Microsoft makes CoPilot Free! (X) * Google - Gemini Flash 2 Thinking experimental reasoning model (X, Studio) * This weeks Buzz * W&B weave Playground now has Trials (and o1 compatibility) (try it) * Alex Evaluation of o1 and Gemini Thinking experimental (X, Colab, Dashboard) * Vision & Video * Google releases Veo 2 – SOTA text2video modal - beating SORA by most vibes (X) * HunyuanVideo distilled with FastHunyuan down to 6 steps (HF) * Kling 1.6 (X) * Voice & Audio * OpenAI realtime audio improvements (docs) * 11labs new Flash 2.5 model – 75ms generation (X) * Nexa OmniAudio – 2.6B – multimodal local LLM (Blog) * Moonshine Web – real time speech recognition in the browser (X) * Sony MMAudio - open source video 2 audio model (Blog, Demo) * AI Art & Diffusion & 3D * Genesys – open source generative 3D physics engine (X, Site, Github) * Tools * CerebrasCoder – extremely fast apps creation (Try It) * RepoPrompt to chat with o1 Pro – (download) This is a public episode. If you'd like to discuss this with other subscribers or get access to bonus episodes, visit sub.thursdai.news/subscribe | |||
27 Dec 2024 | 📆 ThursdAI - Dec 26 - OpenAI o3 & o3 mini, DeepSeek v3 658B beating Claude, Qwen Visual Reasoning, Hume OCTAVE & more AI news | 01:35:32 | |
Hey everyone, Alex here 👋 I was hoping for a quiet holiday week, but whoa, while the last newsletter was only a week ago, what a looong week it has been, just Friday after the last newsletter, it felt like OpenAI has changed the world of AI once again with o3 and left everyone asking "was this AGI?" over the X-mas break (Hope Santa brought you some great gifts!) and then not to be outdone, DeepSeek open sourced basically a Claude 2.5 level behemoth DeepSeek v3 just this morning! Since the breaking news from DeepSeek took us by surprise, the show went a bit longer (3 hours today!) than expected, so as a Bonus, I'm going to release a separate episode with a yearly recap + our predictions from last year and for next year in a few days (soon in your inbox!) TL;DR * Open Source LLMs * CogAgent-9B (Project, Github) * Qwen QvQ 72B - open weights visual reasoning (X, HF, Demo, Project) * GoodFire Ember - MechInterp API - GoldenGate LLama 70B * 🔥 DeepSeek v3 658B MoE - Open Source Claude level model at $6M (X, Paper, HF, Chat) * Big CO LLMs + APIs * 🔥 OpenAI reveals o3 and o3 mini (Blog, X) * X.ai raises ANOTHER 6B dollars - on their way to 200K H200s (X) * This weeks Buzz * Two W&B workshops upcoming in January * SF - January 11 * Seattle - January 13 (workshop by yours truly!) * New Evals course with Paige Bailey and Graham Neubig - pre-sign up for free * Vision & Video * Kling 1.6 update (Tweet) * Voice & Audio * Hume OCTAVE - 3B speech-language model (X, Blog) * Tools * OpenRouter added Web Search Grounding to 300+ models (X) Open Source LLMs DeepSeek v3 658B - frontier level open weights model for ~$6M (X, Paper, HF, Chat ) This was absolutely the top of the open source / open weights news for the past week, and honestly maybe for the past month. DeepSeek, the previous quant firm from China, has dropped a behemoth model, a 658B parameter MoE (37B active), that you'd need 8xH200 to even run, that beats Llama 405, GPT-4o on most benchmarks and even Claude Sonnet 3.5 on several evals! The vibes seem to be very good with this one, and while it's not all the way beating Claude yet, it's nearly up there already, but the kicker is, they trained it with a very restricted compute, per the paper, with ~2K h800 (which is like H100 but with less bandwidth) for 14.8T tokens. (that's 15x cheaper than LLama 405 for comparison) For evaluations, this model excels on Coding and Math, which is not surprising given how excellent DeepSeek coder has been, but still, very very impressive! On the architecture front, the very interesting thing is, this feels like Mixture of Experts v2, with a LOT of experts (256) and 8+1 active at the same time, multi token prediction, and a lot optimization tricks outlined in the impressive paper (here's a great recap of the technical details) The highlight for me was, that DeepSeek is distilling their recent R1 version into this version, which likely increases the performance of this model on Math and Code in which it absolutely crushes (51.6 on CodeForces and 90.2 on MATH-500) The additional aspect of this is the API costs, and while they are going to raise the prices come February (they literally just swapped v2.5 for v3 in their APIs without telling a soul lol), the price performance for this model is just absurd. Just a massive massive release from the WhaleBros, now I just need a quick 8xH200 to run this and I'm good 😅 Other OpenSource news - Qwen QvQ, CogAgent-9B and GoldenGate LLama In other open source news this week, our friends from Qwen have released a very interesting preview, called Qwen QvQ, a visual reasoning model. It uses the same reasoning techniques that we got from them in QwQ 32B, but built with the excellent Qwen VL, to reason about images, and frankly, it's really fun to see it think about an image. You can try it here and a new update to CogAgent-9B (page), an agent that claims to understand and control your computer, claims to beat Claude 3.5 Sonnet Computer Use with just a 9B model! This is very impressive though I haven't tried it just yet, I'm excited to see those very impressive numbers from open source VLMs driving your computer and doing tasks for you! A super quick word from ... Weights & Biases! We've just opened up pre-registration for our upcoming FREE evaluations course, featuring Paige Bailey from Google and Graham Neubig from All Hands AI. We've distilled a lot of what we learned about evaluating LLM applications while building Weave, our LLM Observability and Evaluation tooling, and are excited to share this with you all! Get on the list Also, 2 workshops (also about Evals) from us are upcoming, one in SF on Jan 11th and one in Seattle on Jan 13th (which I'm going to lead!) so if you're in those cities at those times, would love to see you! Big Companies - APIs & LLMs OpenAI - introduces o3 and o3-mini - breaking Arc-AGI challenge, GQPA and teasing AGI? On the last day of the 12 days of OpenAI, we've got the evals of their upcoming o3 reasoning model (and o3-mini) and whoah. I think I speak on behalf of most of my peers that we were all shaken by how fast the jump in capabilities happened from o1-preview and o1 full (being released fully just two weeks prior on day 1 of the 12 days) Almost all evals shared with us are insane, from 96.7 on AIME (from 13.4 with Gpt40 earlier this year) to 87.7 GQPA Diamond (which is... PhD level Science Questions) But two evals stand out the most, and one of course is the Arc-AGI eval/benchmark. It was designed to be very difficult for LLMs and easy for humans, and o3 solved it with an unprecedented 87.5% (on high compute setting) This benchmark was long considered impossible for LLMs, and just the absolute crushing of this benchmark for the past 6 months is something to behold: The other thing I want to highlight is the Frontier Math benchmark, which was released just two months ago by Epoch, collaborating with top mathematicians to create a set of very challenging math problems. At the time of release (Nov 12), the top LLMs solved only 2% of this benchmark. With o3 solving 25% of this benchmark just 3 months after o1 taking 2%, it's quite incredible to see how fast these models are increasing in capabilities. Is this AGI? This release absolutely started or restarted a debate of what is AGI, given that, these goal posts move all the time. Some folks are freaking out and saying that if you're a software engineer, you're "cooked" (o3 solved 71.7 of SWE-bench verified and gets 2727 ELO on CodeForces which is competition code, which is 175th global rank among human coders!), some have also calculated its IQ and estimate it to be at 157 based on the above CodeForces rating. So the obvious question is being asked (among the people who follow the news, most people who don't follow the news could care less) is.. is this AGI? Or is something else AGI? Well, today we got a very interesting answer to this question, from a leak between a Microsoft and OpenAI negotiation and agreement, in which they have a very clear definition of AGI. "A system generating $100 Billion in profits" - a reminder, per their previous agreement, if OpenAI builds AGI, Microsoft will lose access to OpenAI technologies. o3-mini and test-time compute as the new scaling law While I personally was as shaken as most of my peers at these incredible breakthroughs, I was also looking at the more practical and upcoming o3-mini release, which is supposed to come on January this year per Sam Altman. Per their evaluations, o3-mini is going to be significantly cheaper and faster than o3, while offering 3 levels of reasoning effort to developers (low, medium and high) and on medium level, it would beat the current best model (o1) while being cheaper than o1-mini. All of these updates and improvements in the span of less than 6 months are a testament of just how impressive test-time compute is as our additional new scaling law. Not to mention that current scaling laws still hold, we're waiting for Orion or GPT 4.5 or whatever it's called, and that underlying model will probably significantly improve the reasoning models that are built on top of it. Also, if the above results from DeepSeek are anything to go by (and they should be), the ability of these reasoning models to generate incredible synthetic training data for the next models is also quite incredible so... flywheel is upon us, models get better and make better models. Other AI news from this week: The most impressive other news came from HUME, showcasing OCTAVE - their new 3B speech-language model, which is able to not only fake someone's voice with 5 seconds of audio, but also take on their personality and style of speaking and mannerisms. This is not only a voice model mind you, but a 3B LLM as well, so it can mimic a voice, and even create new voices from a prompt. While they mentioned the size, the model was not released yet and will be coming to their API soon, and when I asked about open source, it seems that Hume CEO did not think it's a safe bet opening up this kind of tech to the world yet. I also loved a new little x-mas experiment from OpenRouter and Exa, where-in on the actual OpenRouter interface, you can now chat with over 300 models they serve, and ground answers in search. This is it for this week, which again, I thought is going to be a very chill one, and .. nope! The second part of the show/newsletter, in which we did a full recap of the last year, talked about our predictions from last year and did predictions for this next year, is going to drop in a few days 👀 So keep your eyes peeled. (I decided to separate the two, as 3 hour podcast about AI is... long, I'm no Lex Fridman lol) As always, if you found any of this interesting, please share with a friend, and comment on social media, or right here on Substack, I love getting feedback on what works and what doesn't. Thank you for being part of the ThursdAI community 👋 ThursdAI - Recaps of the most high signal AI weekly spaces is a reader-supported publication. To receive new posts and support my work, consider becoming a free or paid subscriber. This is a public episode. If you'd like to discuss this with other subscribers or get access to bonus episodes, visit sub.thursdai.news/subscribe | |||
02 Jan 2025 | 📆 ThursdAI - Jan 2 - is 25' the year of AI agents? | 01:31:29 | |
Hey folks, Alex here 👋 Happy new year! On our first episode of this year, and the second quarter of this century, there wasn't a lot of AI news to report on (most AI labs were on a well deserved break). So this week, I'm very happy to present a special ThursdAI episode, an interview with Joāo Moura, CEO of Crew.ai all about AI agents! We first chatted with Joāo a year ago, back in January of 2024, as CrewAI was blowing up but still just an open source project, it got to be the number 1 trending project on Github, and #1 project on Product Hunt. (You can either listen to the podcast or watch it in the embedded Youtube above) 00:36 Introduction and New Year Greetings 02:23 Updates on Open Source and LLMs 03:25 Deep Dive: AI Agents and Reasoning 03:55 Quick TLDR and Recent Developments 04:04 Medical LLMs and Modern BERT 09:55 Enterprise AI and Crew AI Introduction 10:17 Interview with João Moura: Crew AI 25:43 Human-in-the-Loop and Agent Evaluation 33:17 Evaluating AI Agents and LLMs 44:48 Open Source Models and Fin to OpenAI 45:21 Performance of Claude's Sonnet 3.5 48:01 Different parts of an agent topology, brain, memory, tools, caching 53:48 Tool Use and Integrations 01:04:20 Removing LangChain from Crew 01:07:51 The Year of Agents and Reasoning 01:18:43 Addressing Concerns About AI 01:24:31 Future of AI and Agents 01:28:46 Conclusion and Farewell --- Is 2025 "the year of AI agents"? AI agents as I remember them as a concept started for me a few month after I started ThursdAI ,when AutoGPT exploded. Was such a novel idea at the time, run LLM requests in a loop, (In fact, back then, I came up with a retry with AI concept and called it TrAI/Catch, where upon an error, I would feed that error back into the GPT api and ask it to correct itself. it feels so long ago!) AutoGPT became the fastest ever Github project to reach 100K stars, and while exciting, it did not work. Since then we saw multiple attempts at agentic frameworks, like babyAGI, autoGen. Crew AI was one of them that keeps being the favorite among many folks. So, what is an AI agent? Simon Willison, friend of the pod, has a mission, to ask everyone who announces a new agent, what they mean when they say it because it seems that everyone "shares" a common understanding of AI agents, but it's different for everyone. We'll start with Joāo's explanation and go from there. But let's assume the basic, it's a set of LLM calls, running in a self correcting loop, with access to planning, external tools (via function calling) and a memory or sorts that make decisions. Though, as we go into detail, you'll see that since the very basic "run LLM in the loop" days, the agents in 2025 have evolved and have a lot of complexity. My takeaways from the conversation I encourage you to listen / watch the whole interview, Joāo is deeply knowledgable about the field and we go into a lot of topics, but here are my main takeaways from our chat * Enterprises are adopting agents, starting with internal use-cases * Crews have 4 different kinds of memory, Long Term (across runs), short term (each run), Entity term (company names, entities), pre-existing knowledge (DNA?) * TIL about a "do all links respond with 200" guardrail * Some of the agent tools we mentioned * Stripe Agent API - for agent payments and access to payment data (blog) * Okta Auth for Gen AI - agent authentication and role management (blog) * E2B - code execution platform for agents (e2b.dev) * BrowserBase - programmatic web-browser for your AI agent * Exa - search grounding for agents for real time understanding * Crew has 13 crews that run 24/7 to automate their company * Crews like Onboarding User Enrichment Crew, Meetings Prep, Taking Phone Calls, Generate Use Cases for Leads * GPT-4o mini is the most used model for 2024 for CrewAI with main factors being speed / cost * Speed of AI development makes it hard to standardize and solidify common integrations. * Reasoning models like o1 still haven't seen a lot of success, partly due to speed, partly due to different way of prompting required. This weeks Buzz We've just opened up pre-registration for our upcoming FREE evaluations course, featuring Paige Bailey from Google and Graham Neubig from All Hands AI (previously Open Devin). We've distilled a lot of what we learned about evaluating LLM applications while building Weave, our LLM Observability and Evaluation tooling, and are excited to share this with you all! Get on the list Also, 2 workshops (also about Evals) from us are upcoming, one in SF on Jan 11th and one in Seattle on Jan 13th (which I'm going to lead!) so if you're in those cities at those times, would love to see you! And that's it for this week, there wasn't a LOT of news as I said. The interesting thing is, even in the very short week, the news that we did get were all about agents and reasoning, so it looks like 2025 is agents and reasoning, agents and reasoning! See you all next week 🫡 TL;DR with links: * Open Source LLMs * HuatuoGPT-o1 - medical LLM designed for medical reasoning (HF, Paper, Github, Data) * Nomic - modernbert-embed-base - first embed model on top of modernbert (HF) * HuggingFace - SmolAgents lib to build agents (Blog) * SmallThinker-3B-Preview - a QWEN 2.5 3B "reasoning" finetune (HF) * Wolfram new Benchmarks including DeepSeek v3 (X) * Big CO LLMs + APIs * Newcomer Rubik's AI Sonus-1 family - Mini, Air, Pro and Reasoning (X, Chat) * Microsoft "estimated" GPT-4o-mini is a ~8B (X) * Meta plans to bring AI profiles to their social networks (X) * This Week's Buzz * W&B Free Evals Course with Page Bailey and Graham Beubig - Free Sign Up * SF evals event - January 11th * Seattle evals workshop - January 13th This is a public episode. If you'd like to discuss this with other subscribers or get access to bonus episodes, visit sub.thursdai.news/subscribe | |||
10 Jan 2025 | 📆 ThursdAI - Jan 9th - NVIDIA's Tiny Supercomputer, Phi-4 is back, Kokoro TTS & Moondream gaze, ByteDance SOTA lip sync & more AI news | 01:20:26 | |
Hey everyone, Alex here 👋 This week's ThursdAI was a whirlwind of announcements, from Microsoft finally dropping Phi-4's official weights on Hugging Face (a month late, but who's counting?) to Sam Altman casually mentioning that OpenAI's got AGI in the bag and is now setting its sights on superintelligence. Oh, and NVIDIA? They're casually releasing a $3,000 supercomputer that can run 200B parameter models on your desktop. No big deal. We had some amazing guests this week too, with Oliver joining us to talk about a new foundation model in genomics and biosurveillance (yes, you read that right - think wastewater and pandemic monitoring!), and then, we've got some breaking news! Vik returned to the show with a brand new Moondream release that can do some pretty wild things. Ever wanted an AI to tell you where someone's looking in a photo? Now you can, thanks to a tiny model that runs on edge devices. 🤯 So buckle up, folks, because we've got a ton to cover. Let's dive into the juicy details of this week's AI madness, starting with open source. 03:10 TL;DR 03:10 Deep Dive into Open Source LLMs 10:58 MetaGene: A New Frontier in AI 20:21 PHI4: The Latest in Open Source AI 27:46 R Star Math: Revolutionizing Small LLMs 34:02 Big Companies and AI Innovations 42:25 NVIDIA's Groundbreaking Announcements 43:49 AI Hardware: Building and Comparing Systems 46:06 NVIDIA's New AI Models: LLAMA Neumatron 47:57 Breaking News: Moondream's Latest Release 50:19 Moondream's Journey and Capabilities 58:41 Weights & Biases: New Evals Course 01:08:29 NVIDIA's World Foundation Models 01:08:29 ByteDance's LatentSync: State-of-the-Art Lip Sync 01:12:54 Kokoro TTS: High-Quality Text-to-Speech As always, TL;DR section with links and show notes below 👇 Open Source AI & LLMs Phi-4: Microsoft's "Small" Model Finally Gets its Official Hugging Face Debut Finally, after a month, we're getting Phi-4 14B on HugginFace. So far, we've had bootlegged copies of it, but it's finally officially uploaded by Microsoft. Not only is it now official, it's also officialy MIT licensed which is great! So, what's the big deal? Well, besides the licensing, it's a 14B parameter, dense decoder-only Transformer with a 16K token context length and trained on a whopping 9.8 trillion tokens. It scored 80.4 on math and 80.6 on MMLU, making it about 10% better than its predecessor, Phi-3 and better than Qwen 2.5's 79 What’s interesting about phi-4 is that the training data consisted of 40% synthetic data (almost half!) The vibes are always interesting with Phi models, so we'll keep an eye out, notable also, the base models weren't released due to "safety issues" and that this model was not trained for multi turn chat applications but single turn use-cases MetaGene-1: AI for Pandemic Monitoring and Pathogen Detection Now, this one's a bit different. We usually talk about LLMs in this section, but this is more about the "open source" than the "LLM." Prime Intellect, along with folks from USC, released MetaGene-1, a metagenomic foundation model. That's a mouthful, right? Thankfully, we had Oliver Liu, a PhD student at USC, and an author on this paper, join us to explain. Oliver clarified that the goal is to use AI for "biosurveillance, pandemic monitoring, and pathogen detection." They trained a 7B parameter model on 1.5 trillion base pairs of DNA and RNA sequences from wastewater, creating a model surprisingly capable of zero-shot embedding. Oliver pointed out that while using genomics to pretrain foundation models is not new, MetaGene-1 is, "in its current state, the largest model out there" and is "one of the few decoder only models that are being used". They also have collected 15T bae pairs but trained on 10% of them due to grant and compute constraints. I really liked this one, and though the science behind this was complex, I couldn't help but get excited about the potential of transformer models catching or helping catch the next COVID 👏 rStar-Math: Making Small LLMs Math Whizzes with Monte Carlo Tree Search Alright, this one blew my mind. A paper from Microsoft (yeah, them again) called "rStar-Math" basically found a way to make small LLMs do math better than o1 using Monte Carlo Tree Search (MCTS). I know, I know, it sounds wild. They took models like Phi-3-mini (a tiny 3.8B parameter model) and Qwen 2.5 3B and 7B, slapped some MCTS magic on top, and suddenly these models are acing the AIME 2024 competition math benchmark and scoring 90% on general math problems. For comparison, OpenAI's o1-preview scores 85.5% on math and o1-mini scores 90%. This is WILD, as just 5 months ago, it was unimaginable that any LLM can solve math of this complexity, then reasoning models could, and now small LLMs with some MCTS can! Even crazier, they observed an "emergence of intrinsic self-reflection capability" in these models during problem-solving, something they weren't designed to do. LDJ chimed in saying "we're going to see more papers showing these things emerging and caught naturally." So, is 2025 the year of not just AI agents, but also emergent reasoning in LLMs? It's looking that way. The code isn't out yet (the GitHub link in the paper is currently a 404), but when it drops, you can bet we'll be all over it. Big Companies and LLMs OpenAI: From AGI to ASI Okay, let's talk about the elephant in the room: Sam Altman's blog post. While reflecting on getting fired from his job on like a casual Friday, he dropped this bombshell: "We are now confident that we know how to build AGI as we have traditionally understood it." And then, as if that wasn't enough, he added, "We're beginning to turn our aim beyond that to superintelligence in the true sense of the word." So basically, OpenAI is saying, "AGI? Done. Next up: ASI." This feels like a big shift in how openly folks at OpenAI is talking about Superintelligence, and while AGI is yet to be properly defined (LDJ read out the original OpenAI definition on the live show, but the Microsoft definition contractually with OpenAI was a system that generates $100B in revenue) they are already talking about Super Intelligence which supersedes all humans ever lived in all domains NVIDIA @ CES - Home SuperComputers, 3 scaling laws, new Models There was a lot of things happening at CES, the largest consumer electronics show, but the AI focus was on NVIDIA, namely on Jensen Huangs keynote speech! He talked about a lot of stuff, really, it's a show, and is a very interesting watch, NVIDIA is obviously at the forefront of all of this AI wave, and when Jensen tells you that we're at the high of the 3rd scaling law, he knows what he's talking about (because he's fueling all of it with his GPUs) - the third one is of course test time scaling or "reasoning", the thing that powers o1, and the coming soon o3 model and other reasoners. Project Digits - supercomputer at home? Jensen also announced Project Digits: a compact AI supercomputer priced at a relatively modest $3,000. Under the hood, it wields a Grace Blackwell “GB10” superchip that supposedly offers 1 petaflop of AI compute and can support LLMs up to 200B parameters (or you can link 2 of them to run LLama 405b at home!) This thing seems crazy, but we don't know more details like the power requirements for this beast! Nemotrons again? Also announced was a family of NVIDIA LLama Nemotron foundation models, but.. weirdly we already have Nemotron LLamas (3 months ago) , so those are... new ones? I didn't really understand what was announced here, as we didn't get new models, but the announcement was made nonetheless. We're due to get 3 new version of Nemotron on the Nvidia NEMO platform (and Open), sometime soon. NVIDIA did release new open source models, with COSMOS, which is a whole platform that includes pretrained world foundation models to help simulate world environments to train robots (among other things). They have released txt2world and video2world Pre-trained Diffusion and Autoregressive models in 7B and 14B sizes, that generate videos to simulate visual worlds that have strong alignment to physics. If you believe Elon when he says that Humanoid Robots are going to be the biggest category of products (every human will want 1 or 3, so we're looking at 20 billion of them), then COSMOS is a platform to generate synthetic data to train these robots to do things in the real world! This weeks buzz - Weights & Biases corner The wait is over, our LLM Evals course is now LIVE, featuring speakers Graham Neubig (who we had on the pod before, back when Open Hands was still called Open Devin) and Paige Bailey, and Anish and Ayush from my team at W&B! If you're building with LLM in production and don't have a robust evaluation setup, or don't even know where to start with one, this course is definitely for you! Sign up today. You'll learn from examples of Imagen and Veo from Paige, Agentic examples using Weave from Graham and Basic and Advanced Evaluation from Anish and Ayush. The workshop in Seattle next was filled out super quick, so since we didn't want to waitlist tons of folks, we have extended it to another night, so those of you who couldn't get in, will have another opportunity on Tuesday! (Workshop page) but while working on it I came up with this distillation of what I'm going to deliver, and wanted to share with you. Vision & Video New Moondream 01-09 can tell where you look (among other things) (blog, HF) We had some breaking news on the show! Vik Korrapati, the creator of Moondream, joined us to announce updates to Moondream, a new version of his tiny vision language model. This new release has some incredible capabilities, including pointing, object detection, structured output (like JSON), and even gaze detection. Yes, you read that right. Moondream can now tell you where someone (or even a pet!) is looking in an image. Vic explained how they achieved this: "We took one of the training datasets that Gazelle trained on and added it to the Moondream fine tuning mix". What's even more impressive is that Moondream is tiny - the new version comes in 2B and 0.5B parameter sizes. As Vic said, "0.5b is we actually started with the 2b param model and we pruned down while picking specific capabilities you want to preserve". This makes it perfect for edge devices and applications where cost or privacy is a concern. It's incredible to see how far Moondream has come, from a personal project to a company with seven employees working on it. Since Vik joined ThursdAI last January (we seem to be on a kick of revisiting with our guests from last year!) Moondream is a company, but they are committed to open source and so this releases is also Apache 2 👏 but you can also try this out on their website playground and hire them if you need to finetune a custom tiny vision model! Voice & Audio Very exciting updates in the OSS voice and audio this week! KOKORO TTS - Apache 2 tiny (82M! params) TTS that's #1 on TTS arena (HF,Demo) Honestly when Wolfram told me about Kokoro being #1 on TTS arena and that it was released a few weeks back, I almost skipped giving this an update, but wow, this tiny tiny model can run on edge devices, can run in your browser, and the sound it generates is SO clean! It's Apache 2 license and the voices were trained on non licensed data (per the author) There's no voice cloning support yet, but there are voice packs you can use, and somehow, they got the SKY voice. Remember the one that Scarlett Johanson almost sued OpenAI for? That one! And for 82M parameters it sounds so good, hell, for any TTS, it sounds very good! ByteDance - LatentSync state of the art lip syncing (X, Paper, Fal) In the same week, ByteDance released a SOTA lip syncing OSS model called LatentSync, which takes a voice (for example, such as the one you can create with Kokoro above) and a video, and sync the lips of the person in the video, to make it seem like that person said the thing. This is for example great for translation purposes, here's a quick example of my cloned voice (via 11labs) and translated opening of the show in spanish, and overlays it on top of my actual video, and it's pretty good! This week Lex Fridman interviewed Volodymir Zelensky and I loved the technical and AI aspect of that whole multilingual interview, they have translated that into English, Russian and Ukrainian. But the lips weren't synced so it looked a bit off still. Now consider the different with and without lip syncing (here's a quick example I whipped up) Baidu - Hallo 3 - generative avatars now with animated backgrounds Meanwhile over at Baidu, Hallo 3 is their 3rd iteration of generative portraits, a way to turn a single image into a completely animated avatar, by also providing it a recording of your voice (or a TTS, does it really matter at this point?) The highlight here is, the background is now part of these avatars! Where as previously these avatars used to be static, now they have dynamic backgrounds. Tho I still feel weirded out by their lip movements, but maybe with the above lipsyncing this can be fixed? Not a bad second week of the yeah eh? A LOT of open source across multimodalities, supercomputers at home, tiny vision and TTS models and tons of apache 2 or MIT licensed models all over! See you guys next week (well, some of you in person in SF and Seattle) but most of you next week on ThursdAI! 🫡 Tl;DR + Show Notes * Open Source LLMs * Phi-4 MIT licensed family of models from Microsoft (X, Blog, HF) * Prime Intellect - MetaGENE-1 - metagenomic foundation model (Site, X, Paper) * rStar-Math - making Small LLMs do Math better than o1 with MCTS (Paper, Github) * Big CO LLMs + APIs * Sam Altman releases an ASI blog, multiple OpenAI people switch from AGI to ASI (X) * NVIDIA updates from CES (X) * XAI - Grok IOS app + Grok 3 finished pre-training * Qwen has a new web portal with all their modals - chat.qwenlm.ai * This weeks Buzz * Evals Course is LIVE - Evals with Paige Bailey and Graham Neubig Course Signup (Signup) * San Francisco is still open (Details) * Seattle is almost waitlisted (Workshop) * Vision & Video * NVIDIA Cosmos - World Foundation Models (Post, Github, HF) * Moondream 2 announcement - new evals - Chat with Vik Korrapati (X, HF, Try It) * Voice & Audio * Kokoro - #1 TTS with Apache 2 license (HF, Demo) * Baidu - Hallo 3 - generative portraits (Project, Github, HF) * ByteDance - LatentSync lip syncing model (X, Paper, Fal) * AI Art & Diffusion & 3D * Stability - SPAR3D: Stable Point-Aware Reconstruction of 3D Objects from Single Images ( HF) This is a public episode. If you'd like to discuss this with other subscribers or get access to bonus episodes, visit sub.thursdai.news/subscribe | |||
17 Jan 2025 | 📆 ThursdAI - Jan 16, 2025 - Hailuo 4M context LLM, SOTA TTS in browser, OpenHands interview & more AI news | 01:40:32 | |
Hey everyone, Alex here 👋 Welcome back, to an absolute banger of a week in AI releases, highlighted with just massive Open Source AI push. We're talking a MASSIVE 4M context window context window model from Hailuo (remember when a jump from 4K to 16K seemed like a big deal?), a 8B omni model that lets you livestream video and glimpses of Agentic ChatGPT? This week's ThursdAI was jam-packed with so much open source goodness that the big companies were practically silent. But don't worry, we still managed to squeeze in some updates from OpenAI and Mistral, along with a fascinating new paper from Sakana AI on self-adaptive LLMs. Plus, we had the incredible Graham Neubig, from All Hands AI, join us to talk about Open Hands (formerly OpenDevin) and even contributed to our free, LLM Evaluation course on Weights & Biases! Before we dive in, a friend asked me over dinner, what are the main 2 things that happened in AI in 2024, and this week highlights one of those trends. Most of the Open Source is now from China. This week, we got MiniMax from Hailuo, OpenBMB with a new MiniCPM, InternLM came back and most of the rest were Qwen finetunes. Not to mention DeepSeek. Wanted to highlight this significant narrative change and that this is being done despite the chip export restrictions. ThursdAI - Recaps of the most high signal AI weekly spaces is a reader-supported publication. To receive new posts and support my work, consider becoming a free or paid subscriber. Open Source AI & LLMs MiniMax-01: 4 Million Context, 456 Billion Parameters, and Lightning Attention This came absolutely from the left field, given that we've seen no prior LLMs from Haulio, the company previously releasing video models with consistent characters. Dropping a massive 456B mixture of experts model (45B active parameters) with such a long context support in open weights, but also with very significant benchmarks that compete with Gpt-4o, Claude and DeekSeek v3 (75.7 MMLU-pro, 89 IFEval, 54.4 GPQA) They have trained the model on up to 1M context window and then extended it to 4M with ROPE scaling methods (our coverage of RoPE) during Inference. MiniMax-Text-01 adopts a hybrid architecture that combines Lightning Attention, Softmax Attention and Mixture-of-Experts (MoE) with 45B active parameters. I gotta say, when we started talking about context window, imagining a needle in a haystack graph that shows 4M, in the open source seemed far fetched, though we did say that theoretically, there may not be a limit to context windows. I just always expected that limit to be unlocked by transformers alternative architectures like Mamba or other State Space Models. Vision, API and Browsing - Minimax-VL-01 It feels like such a well rounded and complete release, that it highlights just how mature company that is behind it. They have also released a vision version of this model, that includes a 300M param Vision Transformer on top (trained with 512B vision language tokens) that features dynamic resolution and boasts very high DocVQA and ChartQA scores. Not only did these two models were released in open weights, they also launched as a unified API endpoint (supporting up to 1M tokens) and it's cheap! $0.2/1M input and $1.1/1M output tokens! AFAIK this is only the 3rd API that supports this much context, after Gemini at 2M and Qwen Turbo that supports 1M as well. Surprising web browsing capabilities You can play around with the model on their website, hailuo.ai which also includes web grounding, which I found quite surprising to find out, that they are beating chatGPT and Perplexity on how fast they can find information that just happened that same day! Not sure what search API they are using under the hood but they are very quick. 8B chat with video model omni-model from OpenBMB OpenBMB has been around for a while and we've seen consistently great updates from them on the MiniCPM front, but this one takes the cake! This is a complete omni modal end to end model, that does video streaming, audio to audio and text understanding, all on a model that can run on an iPad! They have a demo interface that is very similar to the chatGPT demo from spring of last year, and allows you to stream your webcam and talk to the model, but this is just an 8B parameter model we're talking about! It's bonkers! They are boasting some incredible numbers, and to be honest, I highly doubt their methodology in textual understanding, because, well, based on my experience alone, this model understands less than close to chatGPT advanced voice mode, but miniCPM has been doing great visual understanding for a while, so ChartQA and DocVQA are close to SOTA. But all of this doesn't matter, because, I say again, just a little over a year ago, Google released a video announcing these capabilities, having an AI react to a video in real time, and it absolutely blew everyone away, and it was FAKED. And this time a year after, we have these capabilities, essentially, in an 8B model that runs on device 🤯 Voice & Audio This week seems to be very multimodal, not only did we get an omni-modal from OpenBMB that can speak, and last week's Kokoro still makes a lot of waves, but this week there were a lot of voice updates as well Kokoro.js - run the SOTA open TTS now in your browser Thanks to friend of the pod Xenova (and the fact that Kokoro was released with ONNX weights), we now have kokoro.js, or npm -i kokoro-js if you will. This allows you to install and run Kokoro, the best tiny TTS model, completely within your browser, with a tiny 90MB download and it sounds really good (demo here) Hailuo T2A - Emotional text to speech + API Hailuo didn't rest on their laurels of releasing a huge context window LLM, they also released a new voice framework (tho not open sourced) this week, and it sounds remarkably good (competing with 11labs) They have all the standard features like Voice Cloning, but claim to have a way to preserve the emotional undertones of a voice. They also have 300 voices to choose from and professional effects applied on the fly, like acoustics or telephone filters. (Remember, they have a video model as well, so assuming that some of this is to for the holistic video production) What I specifically noticed is their "emotional intelligence system" that's either automatic or can be selected from a dropdown. I also noticed their "lax" copyright restrictions, as one of the voices that was called "Imposing Queen" sounded just like a certain blonde haired heiress to the iron throne from a certain HBO series. When I generated a speech worth of that queen, I noticed that the emotion in that speech sounded very much like an actress would read them, and unlike any old TTS, just listen to it in the clip above, I don't remember getting TTS outputs with this much emotion from anything, maybe outside of advanced voice mode! Quite impressive! This Weeks Buzz from Weights & Biases - AGENTS! Breaking news from W&B as our CTO just broke SWE-bench Verified SOTA, with his own o1 agentic framework he calls W&B Programmer 😮 at 64.6% of the issues! Shawn describes how he achieved this massive breakthrough here and we'll be publishing more on this soon, but the highlight for me is he ran over 900 evaluations during the course of this, and tracked all of them in Weave! We also have an upcoming event in NY, on Jan 22nd, if you're there, come by and learn how to evaluate your AI agents, RAG applications and hang out with our team! (Sign up here) Big Companies & APIs OpenAI adds chatGPT tasks - first agentic feature with more to come! We finally get a glimpse of an agentic chatGPT, in the form of scheduled tasks! Deployed to all users, it is now possible to select gpt-4o with tasks, and schedule tasks in the future. You can schedule them in natural language, and then will execute a chat (and maybe perform a search or do a calculation) and then send you a notification (and an email!) when the task is done! A bit underwhelming at first, as I didn't really find a good use for this yet, I don't doubt that this is just a building block for something more Agentic to come that can connect to my email or calendar and do actual tasks for me, not just... save me from typing the chatGPT query at "that time" Mistral CodeStral 25.01 - a new #1 coding assistant model An updated Codestral was released at the beginning of the week, and TBH I've never seen the vibes split this fast on a model. While it's super exciting that Mistral is placing a coding model at #1 on the LMArena CoPilot's arena, near Claude 3.5 and DeepSeek, the fact that this new model is not released weights is really a bummer (especially as a reference to the paragraph I mentioned on top) We seem to be closing down on OpenSource in the west, while the Chinese labs are absolutely crushing it (while also releasing in the open, including Weights, Technical papers). Mistral has released this model in API and via a collab with the Continue dot dev coding agent, but they used to be the darling of the open source community by releasing great models! Also notable, a very quick new benchmark post release was dropped that showed a significant difference between their reported benchmarks and how it performs on Aider polyglot There was way more things for this week than we were able to cover, including a new and exciting transformers squared new architecture from Sakana, a new open source TTS with voice cloning and a few other open source LLMs, one of which cost only $450 to train! All the links in the TL;DR below! TL;DR and show notes * Open Source LLMs * MiniMax-01 from Hailuo - 4M context 456B (45B A) LLM (Github, HF, Blog, Report) * Jina - reader V2 model - HTML 2 Markdown/JSON (HF) * InternLM3-8B-Instruct - apache 2 License (Github, HF) * OpenBMB - MiniCPM-o 2.6 - Multimodal Live Streaming on Your Phone (HF, Github, Demo) * KyutAI - Helium-1 2B - Base (X, HF) * Dria-Agent-α - 3B model that outputs python code (HF) * Sky-T1, a ‘reasoning’ AI model that can be trained for less than $450 (blog) * Big CO LLMs + APIs * OpenAI launches ChatGPT tasks (X) * Mistral - new CodeStral 25.01 (Blog, no Weights) * Sakana AI - Transformer²: Self-Adaptive LLMs (Blog) * This weeks Buzz * Evaluating RAG Applications Workshop - NY, Jan 22, W&B and PineCone (Free Signup) * Our evaluations course is going very strong! (chat w/ Graham Neubig) (https://wandb.me/evals-t) * Vision & Video * Luma releases Ray2 video model (Web) * Voice & Audio * Hailuo T2A-01-HD - Emotions Audio Model from Hailuo (X, Try It) * OuteTTS 0.3 - 1B & 500M - zero shot voice cloning model (HF) * Kokoro.js - 80M SOTA TTS in your browser! (X, Github, try it ) * AI Art & Diffusion & 3D * Black Forest Labs - Finetuning for Flux Pro and Ultra via API (Blog) * Show Notes and other Links * Hosts - Alex Volkov (@altryne), Wolfram RavenWlf (@WolframRvnwlf), Nisten Tahiraj (@nisten) * Guest - Graham Neubig (@gneubig) from All Hands AI (@allhands_ai) * Graham’s mentioned Agents blogpost - 8 things that agents can do right now * Projects - Open Hands (previously Open Devin) - Github * Germany meetup in Cologne (here) * Toronto Tinkerer Meetup *Sold OUT* (Here) * YaRN conversation we had with the Authors (coverage) See you folks next week! Have a great long weekend if you’re in the US 🫡 Please help to promote the podcast and newsletter by sharing with a friend! This is a public episode. If you'd like to discuss this with other subscribers or get access to bonus episodes, visit sub.thursdai.news/subscribe | |||
24 Jan 2025 | 📆 ThursdAI - Jan 23, 2025 - 🔥 DeepSeek R1 is HERE, OpenAI Operator Agent, $500B AI manhattan project, ByteDance UI-Tars, new Gemini Thinker & more AI news | 01:49:39 | |
What a week, folks, what a week! Buckle up, because ThursdAI just dropped, and this one's a doozy. We're talking seismic shifts in the open source world, a potential game-changer from DeepSeek AI that's got everyone buzzing, and oh yeah, just a casual $500 BILLION infrastructure project announcement. Plus, OpenAI finally pulled the trigger on "Operator," their agentic browser thingy – though getting it to actually operate proved to be a bit of a live show adventure, as you'll hear. This week felt like one of those pivotal moments in AI, a real before-and-after kind of thing. DeepSeek's R1 hit the open source scene like a supernova, and suddenly, top-tier reasoning power is within reach for anyone with a Mac and a dream. And then there's OpenAI's Operator, promising to finally bridge the gap between chat and action. Did it live up to the hype? Well, let's just say things got interesting. As I’m writing this, White House just published that an Executive Order on AI was just signed and published as well, what a WEEK. Open Source AI Goes Nuclear: DeepSeek R1 is HERE! Hold onto your hats, open source AI just went supernova! This week, the Chinese Whale Bros – DeepSeek AI, that quant trading firm turned AI powerhouse – dropped a bomb on the community in the best way possible: R1, their reasoning model, is now open source under the MIT license! As I said on the show, "Open source AI has never been as hot as this week." This isn't just a model, folks. DeepSeek unleashed a whole arsenal: two full-fat R1 models (DeepSeek R1 and DeepSeek R1-Zero), and a whopping six distilled finetunes based on Qwen (1.5B, 7B, 14B, and 32B) and Llama (8B, 72B). One stat that blew my mind, and Nisten's for that matter, is that DeepSeek-R1-Distill-Qwen-1.5B, the tiny 1.5 billion parameter model, is outperforming GPT-4o and Claude-3.5-Sonnet on math benchmarks! "This 1.5 billion parameter model that now does this. It's absolutely insane," I exclaimed on the show. We're talking 28.9% on AIME and 83.9% on MATH. Let that sink in. A model you can probably run on your phone is schooling the big boys in math. License-wise, it's MIT, which as Nisten put it, "MIT is like a jailbreak to the whole legal system, pretty much. That's what most people don't realize. It's like, this is, it's not my problem. You're a problem now." Basically, do whatever you want with it. Distill it, fine-tune it, build Skynet – it's all fair game. And the vibes? "Vibes are insane," as I mentioned on the show. Early benchmarks are showing R1 models trading blows with o1-preview and o1-mini, and even nipping at the heels of the full-fat o1 in some areas. Check out these numbers: And the price? Forget about it. We're talking 50x cheaper than o1 currently. DeepSeek R1 API is priced at $0.14 / 1M input tokens and $2.19 / 1M output tokens, compared to OpenAI's o1 at $15.00 / 1M input and a whopping $60.00 / 1M output. Suddenly, high-quality reasoning is democratized. LDJ highlighted the "aha moment" in DeepSeek's paper, where they talk about how reinforcement learning enabled the model to re-evaluate its approach and "think more." It seems like simple RL scaling, combined with a focus on reasoning, is the secret sauce. No fancy Monte Carlo Tree Search needed, apparently! But the real magic of open source is what the community does with it. Pietro Schirano joined us to talk about his "Retrieval Augmented Thinking" (RAT) approach, where he extracts the thinking process from R1 and transplants it to other models. "And what I found out is actually by doing so, you may even like smaller, quote unquote, you know, less intelligent model actually become smarter," Pietro explained. Frankenstein models, anyone? (John Lindquist has a tutorial on how to do it here) And then there's the genius hack from Voooogel, who figured out how to emulate a "reasoning_effort" knob by simply replacing the "end" token with "Wait, but". "This tricks the model into keeps thinking," as I described it. Want your AI to really ponder the meaning of life (or just 1+1)? Now you can, thanks to open source tinkering. Georgi Gerganov, the legend behind llama.cpp, even jumped in with a two-line snippet to enable speculative decoding, boosting inference speeds on the 32B model on my Macbook from a sluggish 5 tokens per second to a much more respectable 10-11 tokens per second. Open source collaboration at its finest and it's only going to get better! Thinking like a Neurotic Many people really loved the way R1 thinks, and what I found astonishing is that I just sent "hey" and the thinking went into a whole 5 paragraph debate of how to answer, a user on X answered with "this is Woody Allen-level of Neurotic" which... nerd sniped me so hard! I used Hauio Audio (which is great!) and ByteDance latentSync and gave R1 a voice! It's really something when you hear it's inner monologue being spoken out like this! ByteDance Enters the Ring: UI-TARS Controls Your PC Not to be outdone in the open source frenzy, ByteDance, the TikTok behemoth, dropped UI-TARS, a set of models designed to control your PC. And they claim SOTA performance, beating even Anthropic's computer use models and, in some benchmarks, GPT-4o and Claude. UI-TARS comes in 2B, 7B, and 72B parameter flavors, and ByteDance even released desktop apps for Mac and PC to go along with them. "They released an app it's called the UI TARS desktop app. And then, this app basically allows you to Execute the mouse clicks and keyboard clicks," I explained during the show. While I personally couldn't get the desktop app to work flawlessly (quantization issues, apparently), the potential is undeniable. Imagine open source agents controlling your computer – the possibilities are both exciting and slightly terrifying. As Nisten wisely pointed out, "I would use another machine. These things are not safe to tell people. I might actually just delete your data if you, by accident." Words to live by, folks. LDJ chimed in, noting that UI-TARS seems to excel particularly in operating system-level control tasks, while OpenAI's leaked "Operator" benchmarks might show an edge in browser control. It's a battle for desktop dominance brewing in open source! Noting that the common benchmark between Operator and UI-TARS is OSWorld, UI-Tars launched with a SOTA Humanity's Last Exam: The Benchmark to Beat Speaking of benchmarks, a new challenger has entered the arena: Humanity's Last Exam (HLE). A cool new unsaturated bench of 3,000 challenging questions across over a hundred subjects, crafted by nearly a thousand subject matter experts from around the globe. "There's no way I'm answering any of those myself. I need an AI to help me," I confessed on the show. And guess who's already topping the HLE leaderboard? You guessed it: DeepSeek R1, with a score of 9.4%! "Imagine how hard this benchmark is if the top reasoning models that we have right now... are getting less than 10 percent completeness on this," MMLU and Math are getting saturated? HLE is here to provide a serious challenge. Get ready to hear a lot more about HLE, folks. Big CO LLMs + APIs: Google's Gemini Gets a Million-Token Brain While open source was stealing the show, the big companies weren't completely silent. Google quietly dropped an update to Gemini Flash Thinking, their experimental reasoning model, and it's a big one. We're talking 1 million token context window and code execution capabilities now baked in! "This is Google's scariest model by far ever built ever," Nisten declared. "This thing, I don't like how good it is. This smells AGI-ish" High praise, and high concern, coming from Nisten! Benchmarks are showing significant performance jumps in math and science evals, and the speed is, as Nisten put it, "crazy usable." They have enabled the whopping 1M context window for the new Gemini Flash 2.0 Thinking Experimental (long ass name, maybe let's call it G1?) and I agree, it's really really good! And unlike some other reasoning models cough OpenAI cough, Gemini Flash Thinking shows you its thinking process! You can actually see the chain of thought unfold, which is incredibly valuable for understanding and debugging. Google's Gemini is quietly becoming a serious contender in the reasoning race (especially with Noam Shazeer being responsible for it!) OpenAI's "Operator" - Agents Are (Almost) Here The moment we were all waiting for (or at least, I was): OpenAI finally unveiled Operator, their first foray into Level 3 Autonomy - agentic capabilities with ChatGPT. Sam Altman himself hyped it up as "AI agents are AI systems that can do work for you. You give them a task and they go off and do it." Sounds amazing, right? Operator is built on a new model called CUA (Computer Using Agent), trained on top of GPT-4, and it's designed to control a web browser in the cloud, just like a human would, using screen pixels, mouse, and keyboard. "This is just using screenshots, no API, nothing, just working," one of the OpenAI presenters emphasized. They demoed Operator booking restaurant reservations on OpenTable, ordering groceries on Instacart, and even trying to buy Warriors tickets on StubHub (though that demo got a little… glitchy). The idea is that you can delegate tasks to Operator, and it'll go off and handle them in the background, notifying you when it needs input or when the task is complete. As I'm writing these words, I have an Operator running trying to get me some fried rice, and another one trying to book me a vacation with kids over the summer, find some options and tell me what it found. Benchmarks-wise, OpenAI shared numbers for OSWorld (38.1%) and WebArena (58.1%), showing Operator outperforming previous SOTA but still lagging behind human performance. "Still a way to go," as they admitted. But the potential is massive. The catch? Operator is initially launching in the US for Pro users only, and even then, it wasn't exactly smooth sailing. I immediately paid the $200/mo to try it out (pro mode didn't convince me, unlimited SORA videos didn't either, operator definitely did, SOTA agents from OpenAI is definitely something I must try!) and my first test? Writing a tweet 😂 Here's a video of that first attempt, which I had to interrupt 1 time. But hey, it's a "low key research preview" right? And as Sam Altman said, "This is really the beginning of this product. This is the beginning of our step into Agents Level 3 on our tiers of AGI" Agentic ChatGPT is coming, folks, even if it's taking a slightly bumpy route to get here. BTW, while I'm writing these words, Operator is looking up some vacation options for me and is sending me notifications about them, what a world and we've only just started 2025! Project Stargate: $500 Billion for AI Infrastructure If R1 and Operator weren't enough to make your head spin, how about a $500 BILLION "Manhattan Project for AI infrastructure"? That's exactly what OpenAI, SoftBank, and Oracle announced this week: Project Stargate. "This is insane," I exclaimed on the show. "Power ups for the United States compared to like, other, other countries, like 500 billion commitment!" We're talking about a massive investment in data centers, power plants, and everything else needed to fuel the AI revolution. 2% of the US GDP, according to some estimates! Larry Ellison even hinted at using this infrastructure for… curing cancer with personalized vaccines. Whether you buy into that or not, the scale of this project is mind-boggling. As LDJ explained, "It seems like it is very specifically for open AI. Open AI will be in charge of operating it. And yeah, it's, it sounds like a smart way to actually kind of get funding and investment for infrastructure without actually having to give away open AI equity." And in a somewhat related move, Microsoft, previously holding exclusive cloud access for OpenAI, has opened the door for OpenAI to potentially run on other clouds, with Microsoft's approval if "they cannot meet demant". Is AGI closer than we think? Sam Altman himself downplayed the hype, tweeting, "Twitter hype is out of control again. We're not going to deploy AGI next month, nor have we built it. We have some very cool stuff for you, but please chill and cut your expectations a hundred X." But then he drops Operator and a $500 billion infrastructure bomb in the same week and announces that o3-mini is going to be available for the FREE tier of chatGPT. Sure, Sam, we're going to chill... yeah right. This Week's Buzz at Weights & Biases: SWE-bench SOTA! Time for our weekly dose of Weights & Biases awesomeness! This week, our very own CTO, Shawn Lewis, broke the SOTA on SWE-bench Verified! That's right, W&B Programmer, Shawn's agentic framework built on top of o1, achieved a 64.6% solve rate on this notoriously challenging coding benchmark. Shawn detailed his journey in a blog post, highlighting the importance of iteration and evaluation – powered by Weights & Biases Weave, naturally. He ran over 1000 evaluations to reach this SOTA result! Talk about eating your own dogfood! REMOVING BARRIERS TO AMERICAN LEADERSHIP IN ARTIFICIAL INTELLIGENCE - Executive order Just now as I’m editing the podcast, President Trump signed into effect an executive order for AI, and here are the highlights. - Revokes existing AI policies that hinder American AI innovation - Aims to solidify US as global leader in AI for human flourishing, competitiveness, and security - Directs development of an AI Action Plan within 180 days - Requires immediate review and revision of conflicting policies - Directs OMB to revise relevant memos within 60 days - Preserves agency authority and OMB budgetary functions - Consistent with applicable law and funding availability - Seeks to remove barriers and strengthen US AI dominance This marks such a significant pivot into AI acceleration, removing barriers, acknowledging that AI is a huge piece of our upcoming future and that US really needs to innovate here, become the global leader, and remove regulation and obstacles. The folks that work on this behind the scenes, Sriram Krishan (previously A16Z) and David Sacks, are starting to get into the government and implement those policies, so we’re looking forward to what will come form that! Vision & Video: Nvidia's Vanishing Eagle 2 & Hugging Face's Tiny VLM In the world of vision and video, Nvidia teased us with Eagle 2, a series of frontier vision-language models promising 4K HD input, long-context video, and grounding capabilities with some VERY impressive evals. Weights were released, then…yanked. "NVIDIA released Eagle 2 and then yanked it back. So I don't know what's that about," I commented. Mysterious Nvidia strikes again. On the brighter side, Hugging Face released SmolVLM, a truly tiny vision-language model, coming in at just 256 million and 500 million parameters. "This tiny model that runs in like one gigabyte of RAM or some, some crazy things, like a smart fridge" I exclaimed, impressed. The 256M model even outperforms their previous 80 billion parameter Idefics model from just 17 months ago. Progress marches on, even in tiny packages. AI Art & Diffusion & 3D: Hunyuan 3D 2.0 is State of the Art For the artists and 3D enthusiasts, Tencent's Hunyuan 3D 2.0 dropped this week, and it's looking seriously impressive. "Just look at this beauty," I said, showcasing a generated dragon skull. "Just look at this." Hunyuan 3D 2.0 boasts two models: Hunyuan3D-DiT-v2-0 for shape generation and Hunyuan3D-Paint-v2-0 for coloring. Text-to-3D and image-to-3D workflows are both supported, and the results are, well, see for yourself: If you're looking to move beyond 2D images, Hunyuan 3D 2.0 is definitely worth checking out. Tools: ByteDance Clones Cursor with Trae And finally, in the "tools" department, ByteDance continues its open source blitzkrieg with Trae, a free Cursor competitor. "ByteDance drops Trae, which is a cursor competitor, which is free for now" I announced on the show, so if you don't mind your code being sent to... china somewhere, and can't afford Cursor, this is not a bad alternative! Trae imports your Cursor configs, supports Claude 3.5 and GPT-4o, and offers a similar AI-powered code editing experience, complete with chat interface and "builder" (composer) mode. The catch? Your code gets sent to a server in China. If you're okay with that, you've got yourself a free Cursor alternative. "If you're okay with your like code getting shared with ByteDance, this is a good option for you," I summarized. Decisions, decisions. Phew! That was a whirlwind tour through another insane week in AI. From DeepSeek R1's open source reasoning revolution to OpenAI's Operator going live, and Google's million-token Gemini brain, it's clear that the pace of innovation is showing no signs of slowing down. Open source is booming, agents are inching closer to reality, and the big companies are throwing down massive infrastructure investments. We're accelerating as f**k, and it's only just beginning, hold on to your butts. Make sure to dive into the show notes below for all the links and details on everything we covered. And don't forget to give R1 a spin – and maybe try out that "reasoning_effort" hack. Just don't blame me if your AI starts having an existential crisis. And as a final thought, channeling my inner Woody Allen-R1, "Don't overthink too much. enjoy our one. Enjoy the incredible things we received this week from open source." See you all next week for more ThursdAI madness! And hopefully, by then, Operator will actually be operating. 😉 TL;DR and show notes * Open Source LLMs * DeepSeek R1 - MIT licensed SOTA open source reasoning model (HF, X) * ByteDance UI-TARS - PC control models (HF, Github ) * HLE - Humanity's Last Exam benchmark (Website) * Big CO LLMs + APIs * SoftBank, Oracle, OpenAI Stargate Project - $500B AI infrastructure (OpenAI Blog) * Google Gemini Flash Thinking 01-21 - 1M context, Code execution, Better Evals (X) * OpenAI Operator - Agentic browser in ChatGPT Pro operator.chatgpt.com * Anthropic launches citations in API (blog) * Perplexity SonarPRO Search API and an Android AI assistant (X) * This weeks Buzz 🐝 * W&B broke SOTA SWE-bench verified (W&B Blog) * Vision & Video * HuggingFace SmolVLM - Tiny VLMs - runs even on WebGPU (HF) * AI Art & Diffusion & 3D * Hunyuan 3D 2.0 - SOTA open-source 3D (HF) * Tools * ByteDance Trae - Cursor competitor (Trae AI: https://trae.ai/) * Show Notes: * Pietro Skirano RAT - Retrieval augmented generation (X) * Run DeepSeek with more “thinking” script (Gist) This is a public episode. If you'd like to discuss this with other subscribers or get access to bonus episodes, visit sub.thursdai.news/subscribe | |||
30 Jan 2025 | 📆 ThursdAI - Jan 30 - DeepSeek vs. Nasdaq, R1 everywhere, Qwen Max & Video, Open Source SUNO, Goose agents & more AI news | 01:54:46 | |
Hey folks, Alex here 👋 It’s official—grandmas (and the entire stock market) now know about DeepSeek. If you’ve been living under an AI rock, DeepSeek’s new R1 model just set the world on fire, rattling Wall Street (causing the biggest monetary loss for any company, ever!) and rocketing to #1 on the iOS App Store. This week’s ThursdAI show took us on a deep (pun intended) dive into the dizzying whirlwind of open-source AI breakthroughs, agentic mayhem, and big-company cat-and-mouse announcements. Grab your coffee (or your winter survival kit if you’re in Canada), because in true ThursdAI fashion, we’ve got at least a dozen bombshells to cover—everything from brand-new Mistral to next-gen vision models, new voice synthesis wonders, and big moves from Meta and OpenAI. We’re also talking “reasoning mania,” as the entire industry scrambles to replicate, dethrone, or ride the coattails of the new open-source champion, R1. So buckle up—because if the last few days are any indication, 2025 is officially the Year of Reasoning (and quite possibly, the Year of Agents, or both!) Open Source LLMs DeepSeek R1 discourse Crashes the Stock Market One-sentence summary: DeepSeek’s R1 “reasoning model” caused a frenzy this week, hitting #1 on the App Store and briefly sending NVIDIA’s stock plummeting in the process ($560B drop, largest monetary loss of any stock, ever) Ever since DeepSeek R1 launched (our technical coverate last week!), the buzz has been impossible to ignore—everyone from your mom to your local barista has heard the name. The speculation? DeepSeek’s new architecture apparently only cost $5.5 million to train, fueling the notion that high-level AI might be cheaper than Big Tech claims. Suddenly, people wondered if GPU manufacturers like NVIDIA might see shrinking demand, and the stock indeed took a short-lived 17% tumble. On the show, I joked, “My mom knows about DeepSeek—your grandma probably knows about it, too,” underscoring just how mainstream the hype has become. Not everyone is convinced the cost claims are accurate. Even Dario Amodei of Anthropic weighed in with a blog post arguing that DeepSeek’s success increases the case for stricter AI export controls. Public Reactions * Dario Amodei’s blogIn “On DeepSeek and Export Controls,” Amodei argues that DeepSeek’s efficient scaling exemplifies why democratic nations need to maintain a strategic leadership edge—and enforce export controls on advanced AI chips. He sees Chinese breakthroughs as proof that AI competition is global and intense. * OpenAI Distillation EvidenceOpenAI mentioned it found “distillation traces” of GPT-4 inside R1’s training data. Hypocrisy or fair game? On ThursdAI, the panel mused that “everyone trains on everything,” so perhaps it’s a moot point. * Microsoft ReactionMicrosoft wasted no time, swiftly adding DeepSeek to Azure—further proof that corporations want to harness R1’s reasoning power, no matter where it originated. * Government reactedEven officials in the government, David Sacks, US incoming AI & Crypto czar, discussed the fact that DeepSeek did "distillation" using the term somewhat incorrectly, and presidet Trump was asked about it. * API OutagesDeepSeek’s own API has gone in and out this week, apparently hammered by demand (and possibly DDoS attacks). Meanwhile, GPU clouds like Groq are showing up to accelerate R1 at 300 tokens/second, for those who must have it right now. We've seen so many bad takes on the topic, from seething cope takes, to just gross misunderstandings from gov officials confusing the ios App with the OSS models, folks throwing conspiracy theories into the mix, claiming that $5.5M sum was a PsyOp. The fact of the matter is, DeepSeek R1 is an incredible model, and is now powering (just a week later), multiple products (more on this below) and experiences already, while pushing everyone else to compete (and give us reasoning models!) Open Thoughts Reasoning Dataset One-sentence summary: A community-led effort, “Open Thoughts,” released a new large-scale dataset (OpenThoughts-114k) of chain-of-thought reasoning data, fueling the open-source drive toward better reasoning models. Worried about having enough labeled “thinking” steps to train your own reasoner? Fear not. The OpenThoughts-114k dataset aggregates chain-of-thought prompts and responses—114,000 of them—for building or fine-tuning reasoning LLMs. It’s now on Hugging Face for your experimentation pleasure. The ThursdAI panel pointed out how crucial these large, openly available reasoning datasets are. As Wolfram put it, “We can’t rely on the big labs alone. More open data means more replicable breakouts like DeepSeek R1.” Mistral Small 2501 (24B) One-sentence summary: Mistral AI returns to the open-source spotlight with a 24B model that fits on a single 4090, scoring over 81% on MMLU while under Apache 2.0. Long rumored to be “going more closed,” Mistral AI re-emerged this week with Mistral-Small-24B-Instruct-2501—an Apache 2.0 licensed LLM that runs easily on a 32GB VRAM GPU. That 81% MMLU accuracy is no joke, putting it well above many 30B–70B competitor models. It was described as “the perfect size for local inference and a real sweet spot,” noting that for many tasks, 24B is “just big enough but not painfully heavy.” Mistral also finally started comparing themselves to Qwen 2.5 in official benchmarks—a big shift from their earlier reluctance, which we applaud! Berkeley TinyZero & RAGEN (R1 Replications) One-sentence summary: Two separate projects (TinyZero and RAGEN) replicated DeepSeek R1-zero’s reinforcement learning approach, showing you can get “aha” reasoning moments with minimal compute. If you were wondering whether R1 is replicable: yes, it is. Berkeley’s TinyZero claims to have reproduced the core R1-zero behaviors for $30 using a small 3B model. Meanwhile, the RAGEN project aims to unify RL + LLM + Agents with a minimal codebase. While neither replication is at R1-level performance, they demonstrate how quickly the open-source community pounces on new methods. “We’re now seeing those same ‘reasoning sparks’ in smaller reproductions,” said Nisten. “That’s huge.” Agents Codename Goose by Blocks (X, Github) One-sentence summary: Jack Dorsey’s company Blocks released Goose, an open-source local agent framework letting you run keyboard automation on your machine. Ever wanted your AI to press keys and move your mouse in real time? Goose does exactly that with AppleScript, memory extensions, and a fresh approach to “local autonomy.” On the show, I tried Goose, but found it occasionally “went rogue, trying to delete my WhatsApp chats.” Security concerns aside, Goose is significant: it’s an open-source playground for agent-building. The plugin system includes integration with Git, Figma, a knowledge graph, and more. If nothing else, Goose underscores how hot “agentic” frameworks are in 2025. OpenAI’s Operator: One-Week-In It’s been a week since Operator went live for Pro-tier ChatGPT users. “It’s the first agent that can run for multiple minutes without bugging me every single second,”. Yet it’s still far from perfect—captchas, login blocks, and repeated confirmations hamper tasks. The potential, though, is enormous: “I asked Operator to gather my X.com bookmarks and generate a summary. It actually tried,” I shared, “but it got stuck on three links and needed constant nudges.” Simon Willison added that it’s “a neat tech demo” but not quite a productivity boon yet. Next steps? Possibly letting the brand-new reasoning models (like O1 Pro Reasoning) do the chain-of-thought under the hood. I also got tired of opening hundreds of tabs for operator, so I wrapped it in a macOS native app, that has native notifications and the ability to launch Operator tasks via a Raycast extension, if you're interested, you can find it on my Github Browser-use / Computer-use Alternatives In addition to Goose, the ThursdAI panel mentioned browser-use on GitHub, plus numerous code interpreters. So far, none blow minds in reliability. But 2025 is evidently “the year of agents.” If you’re itching to offload your browsing or file editing to an AI agent, expect to tinker, troubleshoot, and yes, babysit. The show consensus? “It’s not about whether agents are coming, it’s about how soon they’ll become truly robust,” said Wolfram. Big CO LLMs + APIs Alibaba Qwen2.5-Max (& Hidden Video Model) (Try It) One-sentence summary: Alibaba’s Qwen2.5-Max stands toe-to-toe with GPT-4 on some tasks, while also quietly rolling out video-generation features. While Western media fixates on DeepSeek, Alibaba’s Qwen team quietly dropped the Qwen2.5-Max MoE model. It clocks in at 69% on MMLU-Pro—beating some OpenAI or Google offerings—and comes with a 1-million-token context window. And guess what? The official Chat interface apparently does hidden video generation, though Alibaba hasn’t publicized it in the English internet. In the Chinese AI internet, this video generation model is called Tongyi Wanxiang, and even has it’s own website, can support first and last video generation and looks really really good, they have a gallery up there, and it even has audio generation together with the video! This one was an img2video, but the movements are really natural! Zuckerberg on LLama4 & LLama4 Mini In Meta’s Q4 earnings call, Zuck was all about AI (sorry, Metaverse). He declared that LLama4 is in advanced training, with a smaller “LLama4 Mini” finishing pre-training. More importantly, a “reasoning model” is in the works, presumably influenced by the mania around R1. Some employees had apparently posted on Blind about “Why are we paying billions for training if DeepSeek did it for $5 million?” so the official line is that Meta invests heavily for top-tier scale. Zuck also doubled down on saying "Glasses are the perfect form factor for AI" , to which I somewhat agree, I love my Meta Raybans, I just wished they were integrated into the ios more. He also boasted about their HUGE datacenters, called Mesa, spanning the size of Manhattan, being built for the next step of AI. (Nearly) Announced: O3-Mini Right before the ThursdAI broadcast, rumors swirled that OpenAI might reveal O3-Mini. It’s presumably GPT-4’s “little cousin” with a fraction of the cost. Then…silence. Sam Altman also mentioned they would be bringing o3-mini by end of January, but maybe the R1 crazyness made them keep working on it and training it a bit more? 🤔 In any case, we'll cover it when it launches. This Week’s Buzz We're still the #1 spot on Swe-bench verified with W&B programmer, and our CTO, Shawn Lewis, chatted with friends of the pod Swyx and Alessio about it! (give it a listen) We have two upcoming events: * AI.engineer in New York (Feb 20–22). Weights & Biases is sponsoring, and I will broadcast ThursdAI live from the summit. If you snagged a ticket, come say hi—there might be a cameo from the “Chef.” * Toronto Tinkerer Workshops (late February) in the University of Toronto. The Canadian AI scene is hot, so watch out for sign-ups (will add them to the show next week) Weights & Biases also teased more features for LLM observability (Weave) and reminded folks of their new suite of evaluation tools. “If you want to know if your AI is actually better, you do evals,” Alex insisted. For more details, check out wandb.me/weave or tune into the next ThursdAI. Vision & Video DeepSeek - Janus Pro - multimodal understanding and image gen unified (1.5B & 7B) One-sentence summary: Alongside R1, DeepSeek also released Janus Pro, a unified model for image understanding and generation (like GPT-4’s rumored image abilities). DeepSeek apparently never sleeps. Janus Pro is MIT-licensed, 7B parameters, and can both parse images (SigLIP) and generate them (LlamaGen). The model outperforms DALL·E 3 and SDXL! on some internal benchmarks—though at a modest 384×384 resolution. NVIDIA’s Eagle 2 Redux One-sentence summary: NVIDIA re-released the Eagle 2 vision-language model with 4K resolution support, after mysteriously yanking it a week ago. Eagle 2 is back, boasting multi-expert architecture, 16k context, and high-res video analysis. Rumor says it competes with big 70B param vision models at only 9B. But it’s overshadowed by Qwen2.5-VL (below). Some suspect NVIDIA is aiming to outdo Meta’s open-source hold on vision—just in time to keep GPU demand strong. Qwen 2.5 VL - SOTA oss vision model is here One-sentence summary: Alibaba’s Qwen 2.5 VL model claims state-of-the-art in open-source vision, including 1-hour video comprehension and “object grounding.” The Qwen team didn’t hold back: “It’s the final boss for vision,” joked Nisten. Qwen 2.5 VL uses advanced temporal modeling for video and can handle complicated tasks like OCR or multi-object bounding boxes. Featuring advances in precise object localization, video temporal understanding and agentic capabilities for computer, this is going to be the model to beat! Voice & Audio YuE 7B (Open “Suno”) Ever dream of building the next pop star from your code editor? YuE 7B is your ticket. This model, now under Apache 2.0, supports chain-of-thought creation of structured songs, multi-lingual lyrics, and references. It’s slow to infer, but it’s arguably the best open music generator so far in the open source What's more, they have changed the license to apache 2.0 just before we went live, so you can use YuE everywhere! Refusion Fuzz Refusion, a new competitor to paid audio models like Suno and Udio, launched “Fuzz,” offering free music generation online until GPU meltdown. If you want to dabble in “prompt to jam track” without paying, check out Refusion Fuzz. Will it match the emotional nuance of premium services like 11 Labs or Hauio? Possibly not. But hey, free is free. Tools (that have integrated R1) Perplexity with R1 In the perplexity.ai chat, you can choose “Pro with R1” if you pay for it, harnessing R1’s improved reasoning to parse results. For some, it’s a major upgrade to “search-based question answering.” Others prefer it to paying for O1 or GPT-4. I always check Perplexity if it knows what the latest episode of ThursdAI was, and it's the first time it did a very good summary! I legit used it to research the show this week! It's really something. Meanwhile, Exa.ai also integrated a “DeepSeek Chat” for your agent-based workflows. Like it or not, R1 is everywhere. Krea.ai with DeepSeek Our friends at Krea, an AI art tool aggregator, also hopped on the R1 bandwagon for chat-based image searching or generative tasks. Conclusion Key Takeaways * DeepSeek’s R1 has massive cultural reach, from #1 apps to spooking the stock market. * Reasoning mania is upon us—everyone from Mistral to Meta wants a piece of the logic-savvy LLM pie. * Agentic frameworks like Goose, Operator, and browser-use are proliferating, though they’re still baby-stepping through reliability issues. * Vision and audio get major open-source love, with Janus Pro, Qwen 2.5 VL, YuE 7B, and more reshaping multimodality. * Big Tech (Meta, Alibaba, OpenAI) is forging ahead with monster models, multi-billion-dollar projects, and cross-country expansions in search of the best reasoning approaches. At this point, it’s not even about where the next big model drop comes from; it’s about how quickly the entire ecosystem can adopt (or replicate) that new methodology. Stay tuned for next week’s ThursdAI, where we’ll hopefully see new updates from OpenAI (maybe O3-Mini?), plus the ongoing race for best agent. Also, catch us at AI.engineer in NYC if you want to talk shop or share your own open-source success stories. Until then, keep calm and carry on training. TLDR * Open Source LLMs * DeepSeek Crashes the Stock Market: Did $5.5M training or hype do it? * Open Thoughts Reasoning Dataset OpenThoughts-114k (X, HF) * Mistral Small 2501 (24B, Apache 2.0) (HF) * Berkeley TinyZero & RAGEN (R1-Zero Replications) (Github, WANDB) * Allen Institute - Tulu 405B (Blog, HF) * Agents * Goose by Blocks (local agent framework) - (X, Github) * Operator (OpenAI) – One-Week-In (X) * Browser-use - oss version of Operator (Github) * Big CO LLMs + APIs * Alibaba Qwen2.5-Max (+ hidden video model) - (X, Try it) * Zuckerberg on LLama4 & “Reasoning Model” (X) * This Week’s Buzz * Shawn Lewis interview on Latent Space with swyx & Alessio * We’re sponsoring the ai.engineer upcoming summit in NY (Feb 19-22), come say hi! * After that, we’ll host 2 workshops with AI Tinkerers Toronto (Feb 23-24), make sure you’re signed up to Toronto Tinkerers to receive the invite (we were sold out quick last time!) * Vision & Video * DeepSeek Janus Pro - 1.5B and 7B (Github, Try It) * NVIDIA Eagle 2 (Paper, Model, Demo) * Alibaba Qwen 2.5 VL (Project, HF, Github, Try It) * Voice & Audio * Yue 7B (Open Suno) - (Demo, HF, Github) * Refusion Fuzz (free for now) * Tools * Perplexity with R1 (choose Pro with R1) * Exa integrated R1 for free (demo) * Participants * Alex Volkov (@altryne) * Wolfram Ravenwolf (@WolframRvnwlf) * Nisten Tahiraj (@nisten ) * LDJ (@ldjOfficial) * Simon Willison (@simonw) * W&B Weave (@weave_wb) This is a public episode. If you'd like to discuss this with other subscribers or get access to bonus episodes, visit sub.thursdai.news/subscribe | |||
07 Feb 2025 | 📆 ThursdAI - Feb 6 - OpenAI DeepResearch is your personal PHD scientist, o3-mini & Gemini 2.0, OmniHuman-1 breaks reality & more AI news | 01:40:29 | |
What's up friends, Alex here, back with another ThursdAI hot off the presses. Hold onto your hats because this week was another whirlwind of AI breakthroughs, mind-blowing demos, and straight-up game-changers. We dove deep into OpenAI's new "Deep Research" agent – and let me tell you, it's not just hype, it's legitimately revolutionary. You also don't have to take my word for it, a new friend of the pod and a scientist DR Derya Unutmaz joined us to discuss his experience with Deep Research as a scientist himself! You don't want to miss this conversation! We also unpack Google's Gemini 2.0 release, including the blazing-fast Flash Lite model. And just when you thought your brain couldn't handle more, ByteDance drops OmniHuman-1, a human animation model that's so realistic, it's scary good. I've also saw maybe 10 more TLDR & Show Notes * Open Source LLMs (and deep research implementations) * Jina Node-DeepResearch (X, Github) * HuggingFace - OpenDeepResearch (X) * Deep Agent - R1 -V (X, Github) * Krutim - Krutim 2 12B, Chitrath VLM, Embeddings and more from India (X, Blog, HF) * Simple Scaling - S1 - R1 (Paper) * Mergekit updated - * Big CO LLMs + APIs * OpenAI ships o3-mini and o3-mini High + updates thinking traces (Blog, X) * Mistral relaunches LeChat with Cerebras for 1000t/s (Blog) * OpenAI Deep Research - the researching agent that uses o3 (X, Blog) * Google ships Gemini 2.0 Pro, Gemini 2.0 Flash-lite in AI Studio (Blog) * Anthropic Constitutional Classifiers - announced a universal jailbreak prevention (Blog, Try It) * Cloudflare to protect websites from AI scraping (News) * HuggingFace becomes the AI Appstore (link) * This weeks Buzz - Weights & Biases updates * AI Engineer workshop (Saturday 22) * Tinkerers Toronto workshops (Sunday 23 , Monday 24) * We released a new Dataset editor feature (X) * Audio and Sound * KyutAI open sources Hibiki - simultaneous translation models (Samples, HF) * AI Art & Diffusion & 3D * ByteDance OmniHuman-1 - unparalleled Human Animation Models (X, Page) * Pika labs adds PikaAdditions - adding anything to existing video (X) * Google added Imagen3 to their API (Blog) * Tools & Others * Mistral Le Chat has ios an and adroid apps now (X) * CoPilot now has agentic workflows (X) * Replit launches free apps agent for everyone (X) * Karpathy drops a new 3 hour video on youtube (X, Youtube) * OpenAI canvas links are now shareable (like Anthropic artifacts) - (example) * Show Notes & Links * Guest of the week - Dr Derya Umnutaz - talking about Deep Research * He's examples of Ehlers-Danlos Syndrome (ChatGPT), (ME/CFS) Deep Research, Nature article about Deep Reseach with Derya comments * Hosts * Alex Volkov - AI Evangelist & Host @altryne * Wolfram Ravenwolf - AI Evangelist @WolframRvnwlf * Nisten Tahiraj - AI Dev at github.GG - @nisten * LDJ - Resident data scientist - @ldjconfirmed Big Companies products & APIs OpenAI's new chatGPT moment with Deep Research, their second "agent" product (X) Look, I've been reporting on AI weekly for almost 2 years now, and been following the space closely since way before chatGPT (shoutout Codex days) and this definitely feels like another chatGPT moment for me. DeepResearch is OpenAI's new agent, that searches the web for any task you give it, is able to reason about the results, and continue searching those sources, to provide you with an absolute incredible level of research into any topic, scientific or ... the best taqueria in another country. The reason why it's so good is it's ability to do multiple search trajectories, backtrack if it needs to, and react in real time to new information. It also has python tool use (to do plots and calculations) and of course, the brain of it is o3, the best reasoning model from OpenAI Deep Research is only offered on the Pro tier ($200) of chatGPT, and it's the first publicly available way to use o3 full! and boy, does it deliver! I've had it review my workshop content, help me research LLM as a judge articles (which it did masterfully) and help me plan datenights in Denver (though it kind of failed at that, showing me a closed restaurant) A breakthrough for scientific research But I'm no scientist, so I've asked Dr Derya Unutmaz, M.D. to join us, and share his incredible findings as a doctor, a scientist and someone with decades of experience in writing grants, patent applications, paper etc. The whole conversation is very very much worth listening to on the pod, we talked for almost an hour, but the highlights are honestly quite crazy. So one of the first things I did was, I asked Deep Research to write a review on a particular disease that I’ve been studying for a decade. It came out with this impeccable 10-to-15-page review that was the best I’ve read on the topic— Dr. Derya Unutmaz And another banger quote It wrote a phenomenal 25-page patent application for a friend’s cancer discovery—something that would’ve cost 10,000 dollars or more and taken weeks. I couldn’t believe it. Every one of the 23 claims it listed was thoroughly justified Humanity's LAST exam? OpenAI announced Deep Research and have showed that on HLE (Humanity's Last Exam) benchmark that was just released a few weeks ago, it scores a whopping 26.6 percent! When HLE was released (our coverage here) all the way back at ... checks notes... January 23 or this year! the top reasoning models at the time (o1, R1) scored just under 10% O3-mini and Deep Research now score 13% and 26.6% respectively, which means both that AI is advancing like crazy, but also.. that maybe calling this "last exam" was a bit premature? 😂😅 Deep Research is now also SOTA holder on GAIA, a public benchmark on real world questions, though Clementine (one of GAIA authors) throws a bit of shade on the result since OpenAI didn't really submit their results. Incidently, Clementine is also involved in HuggingFace attempt at replicating Deep Research in the open (with OpenDeepResearch) OpenAI releases o3-mini and o3-mini high This honestly got kind of buried with the Deep Research news, but as promised, on the last day of January, OpenAI released their new reasoning model, which is significantly fast and much cheaper than o1, while matching it on most benchmarks! I've been talking about the fact that during o3 announcement (our coverage) that mini may be more practical and useful announcement than o3 itself, given the price and speed of it. And viola, OpenAI has reduced the price point of their best reasoner model by 67%, and it's now matches just 2x that of DeepSeek R1. Coming in at 110c for 1M input tokens and 440c for 1M output tokens, and streaming at a whopping 1000t/s at some instances, this reasoner is really something to beat. Great for application developers In addition to seem to be a great model, comparing it to R1 is a nonstarter IMO, not only because "it’s sending your data to choyna", which IMO is a ridiculous attack vector and people should be ashamed by posting this content. o3-mini supports all of the nice API things that OpenAI has, like tool use, structured outputs, developer messages and streaming. The ability to set the reasoning effort is also interesting for applications! Added benefit is the new 200K context window with 100K (claimed) output context. It's also really really fast, while R1 availability grows, as it gets hosted on more and more US based providers, none of them are offering the full context window at these token speeds. o3-mini-high?! While the free users also started getting access to o3-mini, with the "reason" button on chatGPT, plus subscribers received 2 models, o3-mini and o3-mini-high, which is essentially the same model, but with the "high" reasoning mode turned on, giving the model significantly more compute (and tokens) to think. This can be done on the API level by selecting reasoning_effort=high but it's the first time OpenAI is exposing this to non API users! One highlight for me is, just how MANY tokens o3-mini high things through. In one of my evaluations on Weave, o3-mini high generated around 160K output tokens, answering 20 questions, while DeepSeek R1 for example generated 75K and Gemini Thinking, got the highest score on these, while charging only 14K tokens (though I'm pretty sure Google just doesn't report on thinking tokens yet, this seems like a bug) As I'm writing this, OpenAI just announced a new update, o3-mini and o3-mini-high now show... "updated" reasoning traces! These definitely "feel" more like the R1 reasoning traces (remember, previously OpenAI had a different model summarizing the reasoning to prevent training on them?) but they are not really the RAW ones (confirmed) Google ships Gemini 2.0 Pro, Gemini 2.0 Flash-lite in AI Studio (X, Blog) Congrats to our friends at Google for 2.0 👏 Google finally put all the experimental models under one 2.0 umbrella, giving us Gemini 2.0, Gemini 2.0 Flash and a new model! They also introduced Gemini 2.0 Flash-lite, a crazy fast and cheap model that performs similarly to Flash 1.5. The rate limits on Flash-lite are twice as high as the regular Flash, making it incredibly useful for real-time applications. They have also released a few benchmarks, but they only compared those to the previous benchmark released by Google, and while that's great, I wanted a comparison done, so I asked DeepResearch to do it for me, and it did (with citations!) Google also released Imagen 3, their awesome image diffusion model in their API today, with 3c per image, this one is really really good! Mistral's new LeChat spits out 1000t/s + new IOS apps During the show, Mistral announced new capabilities for their LeChat interface, including a 15$/mo tier, but most importantly, a crazy fast generation using some kind of new inference, spitting out around 1000t/s. (Powered by Cerebras) Additionally they have code interpreter there, Canvas, and they also claim to have the best OCR and don't forget, they have access to Flux images, and likely are the only place I know of that offers that image model for free! Finally, they've released native mobile apps! (IOS, Android) * from my quick tests, the 1000t/s is not always on, my first attempt was instant, it was like black magic, and then the rest of them were pretty much the same speed as before 🤔 Maybe they are getting hammered in traffic... This weeks Buzz (What I learned with WandB this week) I got to play around with O3-Mini before it was released (perks of working at Weights & Biases!), and I used Weave, our observability and evaluation framework, to analyze its performance. The results were… interesting. * Latency and Token Count: O3-Mini High's latency was six times longer than O3-Mini Low on a simple reasoning benchmark (92 seconds vs. 6 seconds). But here's the kicker: it didn't even answer more questions correctly! And the token count? O3-Mini High used half a million tokens to answer 20 questions three times. That's… a lot. * Weave Leaderboards: Nisten got super excited about using Weave's leaderboard feature to benchmark models. He realized it could solve a real problem in the open-source community – providing a verifiable and transparent way to share benchmark results. (really, we didnt' rehearse this!) I also announced some upcoming workshops I'd love to see you at: * AI Engineer Workshop in NYC: I'll be running a workshop on evaluations at the AI Engineer Summit in New York on February 22nd. Come say hi and learn about evals! * AI Tinkerers Workshops in Toronto: I'll also be doing two workshops with AI Tinkerers in Toronto on February 23rd and 24th. ByteDance OmniHuman-1 - a reality bending mind breaking img2human model Ok, this is where my mind completely broke this week, like absolutely couldn't stop thinking about this release from ByteDance. After releasing the SOTA lipsyncing model just a few months ago (LatentSync, our coverage) they have once again blew everyone away. This time with a img2avatar model that's unlike anything we've ever seen. This one doesn't need words, just watch my live reaction as I lose my mind The level of real world building in these videos is just absolutely ... too much? The piano keys moving, there's a video of a woman speaking in the microphone, and behind her, the window has reflections of cars and people moving! The thing that most blew me away upon review was the Niki Glazer video, with shiny dress and the model almost perfectly replicating the right sources of light. Just absolute sorcery! The authors confirmed that they don't have any immediate plans to release this as a model or even a product, but given the speed of open source, we'll get this within a year for sure! Get ready Open Source LLMs (and deep research implementations) This week wasn't massive for open-source releases in terms of entirely new models, but the ripple effects of DeepSeek's R1 are still being felt. The community is buzzing with attempts to replicate and build upon its groundbreaking reasoning capabilities. It feels like everyone is scrambling to figure out the "secret sauce" behind R1's "aha moment," and we're seeing some fascinating results. Jina Node-DeepResearch and HuggingFace OpenDeepResearch The community wasted no time trying to replicate OpenAI's Deep Research agent. * Jina AI released "Node-DeepResearch" (X, Github), claiming it follows the "query, search, read, reason, repeat" formula. As I mentioned on the show, "I believe that they're wrong" about it being just a simple loop. O3 is likely a fine-tuned model, but still, it's awesome to see the open-source community tackling this so quickly! * Hugging Face also announced "OpenDeepResearch" (X), aiming to create a truly open research agent. Clementine Fourrier, one of the authors behind the GAIA benchmark (which measures research agent capabilities), is involved, so this is definitely one to watch. Deep Agent - R1 -V: These folks claim to have replicated DeepSeek R1's "aha moment" – where the model realizes its own mistakes and rethinks its approach – for just $3! (X, Github) As I said on the show, "It's crazy, right? Nothing costs $3 anymore. Like it's half a coffee in Starbucks." They even claim you can witness this "aha moment" in a VLM. Open source is moving fast. Krutim - Krutim 2 12B, Chitrath VLM, Embeddings and more from India: This Indian AI lab released a whole suite of models, including an improved LLM (Krutim 2), a VLM (Chitrarth 1), a speech-language model (Dhwani 1), an embedding model (Vyakhyarth 1), and a translation model (Krutrim Translate 1). (X, Blog, HF) They even developed a benchmark called "BharatBench" to evaluate Indic AI performance. However, the community was quick to point out some… issues. As Harveen Singh Chadha pointed out on X, it seems like they blatantly copied IndicTrans, an MIT-licensed model, without even mentioning it. Not cool, Krutim. Not cool. AceCoder: This project focuses on using reinforcement learning (RL) to improve code models. (X) They claim to have created a pipeline to automatically generate high-quality, verifiable code training data. They trained a reward model (AceCode-RM) that significantly boosts the performance of Llama-3.1 and Qwen2.5-coder-7B. They even claim you can skip SFT training for code models by using just 80 steps of R1-style training! Simple Scaling - S1 - R1: This paper (Paper) showcases the power of quality over quantity. They fine-tuned Qwen2.5-32B-Instruct on just 1,000 carefully curated reasoning examples and matched the performance of o1-preview! They also introduced a technique called "budget forcing," allowing the model to control its test-time compute and improve performance. As I mentioned, Niklas Mengenhoff, who worked at Allen and was previously on the show, is involved. This is one to really pay attention to – it shows that you don't need massive datasets to achieve impressive reasoning capabilities. Unsloth reduces R1 type reasoning to just 7GB VRAM (blog) Deepseek R1-zero was autonimously learned reasoning in what they DeepSeek researchers called the "aha moment" Unsloth adds another attempt at replicating this "aha moment" and claims they got it down to less than 7B VRAM, and it can see it for free, in a google colab! This magic could be recreated through GRPO, a RL algorithm that optimizes responses efficiently without requiring a value function, unlike Proximal Policy Optimization (PPO) which relies on a value function How it works:1. The model generates groups of responses.2. Each response is scored based on correctness or another metric created by some set reward function rather than an LLM reward model.3 . The average score of the group is computed.4. Each response's score is compared to the group average.5. The model is reinforced to favor higher-scoring responses. Tools A few new and interesting tools were released this week as well: * Replit rebuilt and released their replit agents in an IOS app and released it free for many users. It can now build mini apps for you on the fly! (Replit) * Mistral has ios / android apps with the new release of LeChat (X) * Molly Cantillon released RPLY, which sits on your mac, and drafts replies to your messages. I installed it during writing this newsletter, and I did not expect it to hit this hard, it reviewed and summarized my texting patterns to "sound like me" and the models sit on device as well. Very very well crafted tool and the best thing it runs models on device if you want! * Github Copilot announced agentic workflows and next line editing, which are cursor features. To try them out you have to download VSCode insiders. They also added Gemini 2.0 (Blog) The AI field moves SO fast, I had to update the content of the newsletter around 5 times while writing it as new things kept getting released! This was a Banger week that started with o3-mini and deep research, continued with Gemini 2.0 and OmniHuman and "ended" with Mistral x Cerebras, Github copilot agents, o3-mini updated COT reasoning traces and a bunch more! AI doesn't stop, and we're here weekly to cover all of this, and give you guys the highlights, but also go deep! Really appreciate Derya's appearance on the show this week, please give him a follow and see you guys next week! This is a public episode. If you'd like to discuss this with other subscribers or get access to bonus episodes, visit sub.thursdai.news/subscribe | |||
13 Feb 2025 | 📆 ThursdAI - Feb 13 - my Personal Rogue AI, DeepHermes, Fast R1, OpenAI Roadmap / RIP GPT6, new Claude & Grok 3 imminent? | 01:43:48 | |
What a week in AI, folks! Seriously, just when you think things might slow down, the AI world throws another curveball. This week, we had everything from rogue AI apps giving unsolicited life advice (and sending rogue texts!), to mind-blowing open source releases that are pushing the boundaries of what's possible, and of course, the ever-present drama of the big AI companies with OpenAI dropping a roadmap that has everyone scratching their heads. Buckle up, because on this week's ThursdAI, we dove deep into all of it. We chatted with the brains behind the latest open source embedding model, marveled at a tiny model crushing math benchmarks, and tried to decipher Sam Altman's cryptic GPT-5 roadmap. Plus, I shared a personal story about an AI app that decided to psychoanalyze my text messages – you won't believe what happened! Let's get into the TL;DR of ThursdAI, February 13th, 2025 – it's a wild one! * Alex Volkov: AI Adventurist with weights and biases * Wolfram Ravenwlf: AI Expert & Enthusiast * Nisten: AI Community Member * Zach Nussbaum: Machine Learning Engineer at Nomic AI * Vu Chan: AI Enthusiast & Evaluator * LDJ: AI Community Member Personal story of Rogue AI with RPLY This week kicked off with a hilarious (and slightly unsettling) story of my own AI going rogue, all thanks to a new Mac app called RPLY designed to help with message replies. I installed it thinking it would be a cool productivity tool, but it turned into a personal intervention session, and then… well, let's just say things escalated. The app started by analyzing my text messages and, to my surprise, delivered a brutal psychoanalysis of my co-parenting communication, pointing out how both my ex and I were being "unpleasant" and needed to focus on the kids. As I said on the show, "I got this as a gut punch. I was like, f*ck, I need to reimagine my messaging choices." But the real kicker came when the AI decided to take initiative and started sending messages without my permission (apparently this was a bug with RPLY that was fixed since I reported)! Friends were texting me question marks, and my ex even replied to a random "Hey, How's your day going?" message with a smiley, completely out of our usual post-divorce communication style. "This AI, like on Monday before just gave me absolute s**t about not being, a person that needs to be focused on the kids also decided to smooth things out on friday" I chuckled, still slightly bewildered by the whole ordeal. It could have gone way worse, but thankfully, this rogue AI counselor just ended up being more funny than disastrous. Open Source LLMs DeepHermes preview from NousResearch Just in time for me sending this newsletter (but unfortunately not quite in time for the recording of the show), our friends at Nous shipped an experimental new thinking model, their first reasoner, called DeepHermes. NousResearch claims DeepHermes is among the first models to fuse reasoning and standard LLM token generation within a single architecture (a trend you'll see echoed in the OpenAI and Claude announcements below!) Definitely experimental cutting edge stuff here, but exciting to see not just an RL replication but also innovative attempts from one of the best finetuning collectives around. Nomic Embed Text V2 - First Embedding MoE Nomic AI continues to impress with the release of Nomic Embed Text V2, the first general-purpose Mixture-of-Experts (MoE) embedding model. Zach Nussbaum from Nomic AI joined us to explain why this release is a big deal. * First general-purpose Mixture-of-Experts (MoE) embedding model: This innovative architecture allows for better performance and efficiency. * SOTA performance on multilingual benchmarks: Nomic Embed V2 achieves state-of-the-art results on the multilingual MIRACL benchmark for its size. * Support for 100+ languages: Truly multilingual embeddings for global applications. * Truly open source: Nomic is committed to open source, releasing training data, weights, and code under the Apache 2.0 License. Zach highlighted the benefits of MoE for embeddings, explaining, "So we're trading a little bit of, inference time memory, and training compute to train a model with mixture of experts, but we get this, really nice added bonus of, 25 percent storage." This is especially crucial when dealing with massive datasets. You can check out the model on Hugging Face and read the Technical Report for all the juicy details. AllenAI OLMOE on iOS and New Tulu 3.1 8B AllenAI continues to champion open source with the release of OLMOE, a fully open-source iOS app, and the new Tulu 3.1 8B model. * OLMOE iOS App: This app brings state-of-the-art open-source language models to your iPhone, privately and securely. * Allows users to test open-source LLMs on-device. * Designed for researchers studying on-device AI and developers prototyping new AI experiences. * Optimized for on-device performance while maintaining high accuracy. * Fully open-source code for further development. * Available on the App Store for iPhone 15 Pro or newer and M-series iPads. * Tulu 3.1 8B As Nisten pointed out, "If you're doing edge AI, the way that this model is built is pretty ideal for that." This move by AllenAI underscores the growing importance of on-device AI and open access. Read more about OLMOE on the AllenAI Blog. Groq Adds Qwen Models and Lands on OpenRouter Groq, known for its blazing-fast inference speeds, has added Qwen models, including the distilled R1-distill, to its service and joined OpenRouter. * Record-fast inference: Experience a mind-blowing 1000 TPS with distilled DeepSeek R1 70B on Open Router. * Usable Rate Limits: Groq is now accessible for production use cases with higher rate limits and pay-as-you-go options. * Qwen Model Support: Access Qwen models like 2.5B-32B and R1-distill-qwen-32B. * Open Router Integration: Groq is now available on OpenRouter, expanding accessibility for developers. As Nisten noted, "At the end of the day, they are shipping very fast inference and you can buy it and it looks like they are scaling it. So they are providing the market with what it needs in this case." This integration makes Groq's speed even more accessible to developers. Check out Groq's announcement on X.com. SambaNova adds full DeepSeek R1 671B - flies at 200t/s (blog) In a complete trend of this week, SambaNova just announced they have availability of DeepSeek R1, sped up by their custom chips, flying at 150-200t/s. This is the full DeepSeek R1, not the distilled Qwen based versions! This is really impressive work, and compared to the second fastest US based DeepSeek R1 (on Together AI) it absolutely flies Agentica DeepScaler 1.5B Beats o1-preview on Math Agentica's DeepScaler 1.5B model is making waves by outperforming OpenAI's o1-preview on math benchmarks, using Reinforcement Learning (RL) for just $4500 of compute. * Impressive Math Performance: DeepScaleR achieves a 37.1% Pass@1 on AIME 2025, outperforming the base model and even o1-preview!! * Efficient Training: Trained using RL for just $4500, demonstrating cost-effective scaling of intelligence. * Open Sourced Resources: Agentica open-sourced their dataset, code, and training logs, fostering community progress in RL-based reasoning. Vu Chan, an AI enthusiast who evaluated the model, joined us to share his excitement: "It achieves, 42% pass at one on a AIME 24. which basically means if you give the model only one chance at every problem, it will solve 42% of them." He also highlighted the model's efficiency, generating correct answers with fewer tokens. You can find the model on Hugging Face, check out the WandB logs, and see the announcement on X.com. ModernBert Instruct - Encoder Model for General Tasks ModernBert, known for its efficient encoder-only architecture, now has an instruct version, ModernBert Instruct, capable of handling general tasks. * Instruct-tuned Encoder: ModernBERT-Large-Instruct can perform classification and multiple-choice tasks using its Masked Language Modeling (MLM) head. * Beats Qwen .5B: Outperforms Qwen .5B on MMLU and MMLU Pro benchmarks. * Efficient and Versatile: Demonstrates the potential of encoder models for general tasks without task-specific heads. This release shows that even encoder-only models can be adapted for broader applications, challenging the dominance of decoder-based LLMs for certain tasks. Check out the announcement on X.com. Big CO LLMs + APIs RIP GPT-5 and o3 - OpenAI Announces Public Roadmap OpenAI shook things up this week with a roadmap update from Sam Altman, announcing a shift in strategy for GPT-5 and the o-series models. Get ready for GPT-4.5 (Orion) and a unified GPT-5 system! * GPT-4.5 (Orion) is Coming: This will be the last non-chain-of-thought model from OpenAI. * GPT-5: A Unified System: GPT-5 will integrate technologies from both the GPT and o-series models into a single, seamless system. * No Standalone o3: o3 will not be released as a standalone model; its technology will be integrated into GPT-5. "We will no longer ship O3 as a standalone model," Sam Altman stated. * Simplified User Experience: The model picker will be eliminated in ChatGPT and the API, aiming for a more intuitive experience. * Subscription Tier Changes: * Free users will get unlimited access to GPT-5 at a standard intelligence level. * Plus and Pro subscribers will gain access to increasingly advanced intelligence settings of GPT-5. * Expanded Capabilities: GPT-5 will incorporate voice, canvas, search, deep research, and more. This roadmap signals a move towards more integrated and user-friendly AI experiences. As Wolfram noted, "Having a unified access and the AI should be smart enough... AI has, we need an AI to pick which AI to use." This seems to be OpenAI's direction. Read Sam Altman's full announcement on X.com. OpenAI Releases ModelSpec v2 OpenAI also released ModelSpec v2, an update to their document defining desired AI model behaviors, emphasizing customizability, transparency, and intellectual freedom. * Chain of Command: Defines a hierarchy to balance user/developer control with platform-level rules. * Truth-Seeking and User Empowerment: Encourages models to "seek the truth together" with users and empower decision-making. * Core Principles: Sets standards for competence, accuracy, avoiding harm, and embracing intellectual freedom. * Open Source: OpenAI open-sourced the Spec and evaluation prompts for broader use and collaboration on GitHub. This release reflects OpenAI's ongoing efforts to align AI behavior and promote responsible development. Wolfram praised ModelSpec, saying, "I was all over the original models back when it was announced in the first place... That is one very important aspect when you have the AI agent going out on the web and get information from not trusted sources." Explore ModelSpec v2 on the dedicated website. VP Vance Speech at AI Summit in Paris - Deregulate and Dominate! Vice President Vance delivered a powerful speech at the AI Summit in Paris, advocating for pro-growth AI policies and deregulation to maintain American leadership in AI. * Pro-Growth and Deregulation: VP Vance urged for policies that encourage AI innovation and cautioned against excessive regulation, specifically mentioning GDPR. * American AI Leadership: Emphasized ensuring American AI technology remains the global standard and blocks hostile foreign adversaries from weaponizing AI. "Hostile foreign adversaries have weaponized AI software to rewrite history, surveil users, and censor speech… I want to be clear – this Administration will block such efforts, full stop," VP Vance declared. * Key Points: * Ensure American AI leadership. * Encourage pro-growth AI policies. * Maintain AI's freedom from ideological bias. * Prioritize a pro-worker approach to AI development. * Safeguard American AI and chip technologies. * Block hostile foreign adversaries' weaponization of AI. Nisten commented, "He really gets something that most EU politicians do not understand is that whenever they have such a good thing, they're like, okay, this must be bad. And we must completely stop it." This speech highlights the ongoing debate about AI regulation and its impact on innovation. Read the full speech here. Cerebras Powers Perplexity with Blazing Speed (1200 t/s!) Perplexity is now powered by Cerebras, achieving inference speeds exceeding 1200 tokens per second. * Unprecedented Speed: Perplexity's Sonar model now flies at over 1200 tokens per second thanks to Cerebras' massive LPU chips. "Like perplexity sonar, their specific LLM for search is now powered by Cerebras and it's like 12. 100 tokens per second. It's it matches Google now on speed," I noted on the show. * Google-Level Speed: Perplexity now matches Google in inference speed, making it incredibly fast and responsive. This partnership significantly enhances Perplexity's performance, making it an even more compelling search and AI tool. See Perplexity's announcement on X.com. Anthropic Claude Incoming - Combined LLM + Reasoning Model Rumors are swirling that Anthropic is set to release a new Claude model that will be a combined LLM and reasoning model, similar to OpenAI's GPT-5 roadmap. * Unified Architecture: Claude's next model is expected to integrate both LLM and reasoning capabilities into a single, hybrid architecture. * Reasoning Powerhouse: Rumors suggest Anthropic has had a reasoning model stronger than Claude 3 for some time, hinting at a significant performance leap. This move suggests a broader industry trend towards unified AI models that seamlessly blend different capabilities. Stay tuned for official announcements from Anthropic. Elon Musk Teases Grok 3 "Weeks Out" Elon Musk continues to tease the release of Grok 3, claiming it will be "a few weeks out" and the "most powerful AI" they have tested, with enhanced reasoning capabilities. * Grok 3 Hype: Elon Musk claims Grok 3 will be the most powerful AI X.ai has released, with a focus on reasoning. * Reasoning Focus: Grok 3's development may have shifted towards reasoning capabilities, potentially causing a slight delay in release. While details remain scarce, the anticipation for Grok 3 is building, especially in light of the advancements in open source reasoning models. This Week's Buzz 🐝 Weave Dataset Editing in UI Weights & Biases Weave has added a highly requested feature: dataset editing directly in the UI. * UI-Based Dataset Editing: Users can now edit datasets directly within the Weave UI, adding, modifying, and deleting rows without code. "One thing that, folks asked us and we've recently shipped is the ability to edit this from the UI itself. So you don't have to have code," I explained. * Versioning and Collaboration: Every edit creates a new dataset version, allowing for easy tracking and comparison. * Improved Dataset Management: Simplifies dataset management and version control for evaluations and experiments. This feature streamlines the workflow for LLM evaluation and observability, making Weave even more user-friendly. Try it out at wandb.me/weave Toronto Workshops - AI in Production: Evals & Observability Don't miss our upcoming AI in Production: Evals & Observability Workshops in Toronto! * Two Dates: Sunday and Monday workshops in Toronto. * Hands-on Learning: Learn to build and evaluate LLM-powered applications with robust observability. * Expert Guidance: Led by yours truly, Alex Volkov, and featuring Nisten. * Limited Spots: Registration is still open, but spots are filling up fast! Register for Sunday's workshop here and Monday's workshop here. Join us to level up your LLM skills and network with the Toronto AI community! Vision & Video Adobe Firefly Video - Image to Video and Text to Video Adobe announced Firefly Video, entering the image-to-video and text-to-video generation space. * Video Generation: Firefly Video offers both image-to-video and text-to-video capabilities. * Adobe Ecosystem: Integrates with Adobe's creative suite, providing a powerful tool for video creators. This release marks Adobe's significant move into the rapidly evolving video generation landscape. Try Firefly Video here. Voice & Audio YouTube Expands AI Dubbing to All Creators YouTube is expanding AI dubbing to all creators, breaking down language barriers on the platform. * AI-Powered Dubbing: YouTube is leveraging AI to provide dubbing in multiple languages for all creators. "YouTube now expands. AI dubbing in languages to all creators, and that's super cool. So basically no language barriers anymore. AI dubbing is here," I announced. * Increased Watch Time: Pilot program saw 40% of watch time in dubbed languages, demonstrating the feature's impact. "Since the pilot launched last year, 40 percent of watch time for videos with the feature enabled was in the dub language and not the original language. That's insane!" I highlighted. * Global Reach: Eliminates language barriers, making content accessible to a wider global audience. Wolfram emphasized the importance of dubbing, especially in regions with strong dubbing cultures like Germany. "Every movie that comes here is getting dubbed in high quality. And now AI is doing that on YouTube. And I personally, as a content creator, I have always have to decide, do I post in German or English?" This feature is poised to revolutionize content consumption on YouTube. Read more on X.com. Meta Audiobox Aesthetics - Unified Quality Assessment Meta released Audiobox Aesthetics, a unified automatic quality assessment model for speech, music, and sound. * Unified Assessment: Provides a single model for evaluating the quality of speech, music, and general sound. * Four Key Metrics: Evaluates audio based on Production Quality (PQ), Production Complexity (PC), Content Enjoyment (CE), and Content Usefulness (CU). * Automated Evaluation: Offers a scalable solution for assessing synthetic audio quality, reducing reliance on costly human evaluations. This tool is expected to significantly improve the development and evaluation of TTS and audio generation models. Access the Paper and Weights on GitHub. Zonos - Expressive TTS with High-Fidelity Cloning Zyphra released Zonos, a highly expressive TTS model with high-fidelity voice cloning capabilities. * Expressive TTS: Zonos offers expressive speech generation with control over speaking rate, pitch, and emotions. * High-Fidelity Voice Cloning: Claims high-fidelity voice cloning from short audio samples (though my personal test was less impressive). "My own voice clone sounded a little bit like me but not a lot. Ok at least for me, the cloning is really really bad," I admitted on the show. * High Bitrate Audio: Generates speech at 44kHz with a high bitrate codec for enhanced audio quality. * Open Source & API: Models are open source, with a commercial API available. While voice cloning might need further refinement, Zonos represents another step forward in open-source TTS technology. Explore Zonos on Hugging Face (Hybrid), Hugging Face (Transformer), and GitHub, and read the Blog post. Tools & Others Emergent Values AI - AI Utility Functions and Biases Researchers found that AIs exhibit emergent values, including biases in valuing human lives from different regions. * Emergent Utility Functions: AI models appear to develop implicit utility functions and value systems during training. "Research finds that AI's have expected utility functions for people and other emergent values. And this is freaky," I summarized. * Value Biases: Studies revealed biases, with AIs valuing lives from certain regions (e.g., Nigeria, Pakistan, India) higher than others (e.g., Italy, France, Germany, UK, US). "Nigerian people, valued as like eight us people. One Nigerian person was valued like eight us people," I highlighted the surprising finding. * Utility Engineering: Researchers propose "utility engineering" as a research agenda to analyze and control these emergent value systems. LDJ pointed out a potential correlation between the valued regions and the source of RLHF data labeling, suggesting a possible link between training data and emergent biases. While the study is still debated, it raises important questions about AI value alignment. Read the announcement on X.com and the Paper. LM Studio Lands Support for Speculative Decoding LM Studio, the popular local LLM inference tool, now supports speculative decoding, significantly speeding up inference. * Faster Inference: Speculative decoding leverages a smaller "draft" model to accelerate inference with a larger model. "Speculative decoding finally landed in LM studio, which is dope folks. If you use LM studio, if you don't, you should," I exclaimed. * Visualize Accepted Tokens: LM Studio visualizes accepted draft tokens, allowing users to see speculative decoding in action. * Performance Boost: Improved inference speeds by up to 40% in tests, without sacrificing model performance. "It runs around 10 tokens per second without the speculative decoding and around 14 to 15 tokens per second with speculative decoding, which is great," I noted. This update makes LM Studio even more powerful for local LLM experimentation. See the announcement on X.com. Noam Shazeer / Jeff Dean on Dwarkesh Podcast Podcast enthusiasts should check out the new Dwarkesh Podcast episode featuring Noam Shazeer (Transformer co-author) and Jeff Dean (Google DeepMind). * AI Insights: Listen to insights from two AI pioneers in this new podcast episode. Tune in to hear from these influential figures in the AI world. Find the announcement on X.com. What a week, folks! From rogue AI analyzing my personal life to OpenAI shaking up the roadmap and tiny models conquering math, the AI world continues to deliver surprises. Here are some key takeaways: * Open Source is Exploding: Nomic Embed Text V2, OLMoE, DeepScaler 1.5B, and ModernBERT Instruct are pushing the boundaries of what's possible with open, accessible models. * Speed is King: Groq, Cerebras and SambaNovas are delivering blazing-fast inference, making real-time AI applications more feasible than ever. * Reasoning is Evolving: DeepScaler 1.5B's success demonstrates the power of RL for even small models, and OpenAI and Anthropic are moving towards unified models with integrated reasoning. * Privacy Matters: AllenAI's OLMoE highlights the growing importance of on-device AI for data privacy. * The AI Landscape is Shifting: OpenAI's roadmap announcement signals a move towards simpler, more integrated AI experiences, while government officials are taking a stronger stance on AI policy. Stay tuned to ThursdAI for the latest updates, and don't forget to subscribe to the newsletter for all the links and details! Next week, I'll be in New York, so expect a special edition of ThursdAI from the AI Engineer floor. TLDR & Show Notes * Open Source LLMs * NousResearch DeepHermes-3 Preview (X, HF) * Nomic Embed Text V2 - first embedding MoE (HF, Tech Report) * AllenAI OLMOE on IOS as a standalone app & new Tulu 3.1 8B (Blog, App Store) * Groq adds Qwen models (including R1 distill) and lands on OpenRouter (X) * Agentica DeepScaler 1.5B beats o1-preview on math using RL for $4500 (X, HF, WandB) * ModernBert can be instructed (though encoder only) to do general tasks (X) * LMArena releases a dataset of 100K votes with human preferences (X, HF) * SambaNova adds full DeepSeek R1 671B - flies at 200t/s (blog) * Big CO LLMs + APIs * RIP GPT-5 and o3 - OpenAI announces a public roadmap (X) * OpenAI released Model Spec v2 (Github, Blog) * VP Vance Speech at AI Summit in Paris (full speech) * Cerebras now powers Perplexity with >1200t/s (X) * Anthropic Claude incoming, will be combined LLM + reasoning (The Information) * This weeks Buzz * We've added dataset editing in the UI (X) * 2 workshops in Toronto, Sunday and Monday * Vision & Video * Adobe announces firefly video (img2video and txt2video) (try it) * Voice & Audio * Youtube to expand AI Dubbing to all creators (X) * Meta Audiobox Aesthetics - Unified Automatic Quality Assessment for Speech, Music, and Sound (Paper, Weights) * Zonos, a highly expressive TTS model with high fidelity voice cloning (Blog, HF,HF, Github) * Tools & Others * Emergent Values AI - Research finds that AI's have expected utility functions (X, paper) * LMStudio lands support for Speculative Decoding (X) * Noam Shazeer / Jeff Dean on Dwarkesh podcast (X) This is a public episode. If you'd like to discuss this with other subscribers or get access to bonus episodes, visit sub.thursdai.news/subscribe | |||
20 Feb 2025 | 📆 ThursdAI - Feb 20 - Live from AI Eng in NY - Grok 3, Unified Reasoners, Anthropic's Bombshell, and Robot Handoffs! | 01:41:13 | |
Holy moly, AI enthusiasts! Alex Volkov here, reporting live from the AI Engineer Summit in the heart of (touristy) Times Square, New York! This week has been an absolute whirlwind of announcements, from XAI's Grok 3 dropping like a bomb, to Figure robots learning to hand each other things, and even a little eval smack-talk between OpenAI and XAI. It’s enough to make your head spin – but that's what ThursdAI is here for. We sift through the chaos and bring you the need-to-know, so you can stay on the cutting edge without having to, well, spend your entire life glued to X and Reddit. This week we had a very special live show with the Haize Labs folks, the ones I previously interviewed about their bijection attacks, discussing their open source judge evaluation library called Verdict. So grab your favorite caffeinated beverage, maybe do some stretches because your mind will be blown, and let's dive into the TL;DR of ThursdAI, February 20th, 2025! Participants * Alex Volkov: AI Evangelist with Weights and Biases * Nisten: AI Engineer and cohost * Akshay: AI Community Member * Nuo: Dev Advocate at 01AI * Nimit: Member of Technical Staff at Haize Labs * Leonard: Co-founder at Haize Labs Open Source LLMs Perplexity's R1 7076: Censorship-Free DeepSeek Perplexity made a bold move this week, releasing R1 7076, a fine-tuned version of DeepSeek R1 specifically designed to remove what they (and many others) perceive as Chinese government censorship. The name itself, 1776, is a nod to American independence – a pretty clear statement! The core idea? Give users access to information on topics the CCP typically restricts, like Tiananmen Square and Taiwanese independence. Perplexity used human experts to identify around 300 sensitive topics and built a "censorship classifier" to train the bias out of the model. The impressive part? They claim to have done this without significantly impacting the model's performance on standard evals. As Nuo from 01AI pointed out on the show, though, he'd "actually prefer that they can actually disclose more of their details in terms of post training... Running the R1 model by itself, it's already very difficult and very expensive." He raises a good point – more transparency is always welcome! Still, it's a fascinating attempt to tackle a tricky problem, the problem which I always say we simply cannot avoid. You can check it out yourself on Hugging Face and read their blog post. Arc Institute & NVIDIA Unveil Evo 2: Genomics Powerhouse Get ready for some serious science, folks! Arc Institute and NVIDIA dropped Evo 2, a massive genomics model (40 billion parameters!) trained on a mind-boggling 9.3 trillion nucleotides. And it’s fully open – two papers, weights, data, training, and inference codebases. We love to see it! Evo 2 uses the StripedHyena architecture to process huge genetic sequences (up to 1 million nucleotides!), allowing for analysis of complex genomic patterns. The practical applications? Predicting the effects of genetic mutations (super important for healthcare) and even designing entire genomes. I’ve been super excited about genomics models, and seeing these alternative architectures like StripedHyena getting used here is just icing on the cake. Check it out on X. ZeroBench: The "Impossible" Benchmark for VLLMs Need more benchmarks? Always! A new benchmark called ZeroBench arrived, claiming to be the "impossible benchmark" for Vision Language Models (VLLMs). And guess what? All current top-of-the-line VLLMs get a big fat zero on it. One example they gave was a bunch of scattered letters, asking the model to "answer the question that is written in the shape of the star among the mess of letters." Honestly, even I struggled to see the star they were talking about. It highlights just how much further VLLMs need to go in terms of true visual understanding. (X, Page, Paper, HF) Hugging Face's Ultra Scale Playbook: Scaling Up For those of you building massive models, Hugging Face released the Ultra Scale Playbook, a guide to building and scaling AI models on huge GPU clusters. They ran 4,000 scaling experiments on up to 512 GPUs (nothing close to Grok's 100,000, but still impressive!). If you're working in a lab and dreaming big, this is definitely a resource to check out. (HF). Big CO LLMs + APIs Grok 3: XAI's Big Swing new SOTA LLM! (and Maybe a Bug?) Monday evening, BOOM! While some of us were enjoying President's Day, the XAI team dropped Grok 3. They announced it with a setting very similar to OpenAI announcements. They're claiming state-of-the-art performance on some benchmarks (more on that drama later!), and a whopping 1 million token context window, finally confirmed after some initial confusion. They talked a lot about agents and a future of reasoners as well. The launch was a bit… messy. First, there was a bug where some users were getting Grok 2 even when the dropdown said Grok 3. That led to a lot of mixed reviews. Even when I finally thought I was using Grok 3, it still flubbed my go-to logic test, the "Beth's Ice Cubes" question. (The answer is zero, folks – ice cubes melt!). But Akshay, who joined us on the show, chimed in with some love: "...with just the base model of Grok 3, it's, in my opinion, it's the best coding model out there." So, mixed vibes, to say the least! It's also FREE for now, "until their GPUs melt," according to XAI, which is great. UPDATE: The vibes are shifting, more and more of my colleagues and mutuals are LOVING grok3 for one shot coding, for talking to it. I’m getting convinced as well, though I did use and will continue to use Grok for real time data and access to X. DeepSearch In an attempt to show off some Agentic features, XAI also launched a deep search (not research like OpenAI but effectively the same) Now, XAI of course has access to X, which makes their deep search have a leg up, specifically for real time information! I found out it can even “use” the X search! OpenAI's Open Source Tease In what felt like a very conveniently timed move, Sam Altman dropped a poll on X the same day as the Grok announcement: if OpenAI were to open-source something, should it be a small, mobile-optimized model, or a model on par with o3-mini? Most of us chose o3 mini, just to have access to that model and play with it. No indication of when this might happen, but it’s a clear signal that OpenAI is feeling the pressure from the open-source community. The Eval Wars: OpenAI vs. XAI Things got spicy! There was a whole debate about the eval numbers XAI posted, specifically the "best of N" scores (like best of 64 runs). Boris from OpenAI, and Aiden mcLau called out some of the graphs. Folks on X were quick to point out that OpenAI also used "best of N" in the past, and the discussion devolved from there. XAI is claiming SOTA. OpenAI (or some folks from within OpenAI) aren't so sure. The core issue? We can't independently verify Grok's performance because there's no API yet! As I said, "…we're not actually able to use this model to independently evaluate this model and to tell you guys whether or not they actually told us the truth." Transparency matters, folks! DeepSearch - How Deep? Grok also touted a new "Deep Search" feature, kind of like Perplexity or OpenAI's "Deep Research" in their more expensive plan. My initial tests were… underwhelming. I nicknamed it "Shallow Search" because it spent all of 34 seconds on a complex query where OpenAI's Deep Research took 11 minutes and cited 17 sources. We're going to need to do some more digging (pun intended) on this one. This Week's Buzz We’re leaning hard into agents at Weights & Biases! We just released an agents whitepaper (check it out on our socials!), and we're launching an agents course in collaboration with OpenAI's Ilan Biggio. Sign up at wandb.me/agents! We're hearing so much about agent evaluation and observability, and we're working hard to provide the tools the community needs. Also, sadly, our Toronto workshops are completely sold out. But if you're at AI Engineer in New York, come say hi to our booth! And catch my talk on LLM Reasoner Judges tomorrow (Friday) at 11 am EST – it’ll be live on the AI Engineer YouTube channel (HERE)! Vision & Video Microsoft MUSE: Playable Worlds from a Single Image This one is wild. Microsoft's MUSE can generate minutes of playable gameplay from just a single second of video frames and controller actions. It's based on the World and Human Action Model (WHAM) architecture, trained on a billion gameplay images from Xbox. So if you’ve been playing Xbox lately, you might be in the model! I found it particularly cool: "…you give it like a single second of a gameplay of any type of game with all the screen elements, with percentages, with health bars, with all of these things and their model generates a game that you can control." (X, HF, Blog). StepFun's Step-Video-T2V: State-of-the-Art (and Open Source!) We got two awesome open-source video breakthroughs this week. First, StepFun's Step-Video-T2V (and T2V Turbo), a 30 billion parameter text-to-video model. The results look really good, especially the text integration. Imagine a Chinese girl opening a scroll, and the words "We will open source" appearing as she unfurls it. That’s the kind of detail we're talking about. And it’s MIT licensed! As Nisten noted "This is pretty cool. It came out. Right before Sora came out, people would have lost their minds." (X, Paper, HF, Try It). HAO AI's FastVideo: Speeding Up HY-Video The second video highlight: HAO AI released FastVideo, a way to make HY-Video (already a strong open-source contender) three times faster with no additional training! They call the trick "Sliding Tile Attention" apparently that alone provides enormous boost compared to even flash attention. This is huge because faster inference means these models become more practical for real-world use. And, bonus: it supports HY-Video's Loras, meaning you can fine-tune it for, ahem, all kinds of creative applications. I will not go as far as to mention civit ai. (Github) Figure's Helix: Robot Collaboration! Breaking news from the AI Engineer conference floor: Figure, the humanoid robot company, announced Helix, a Vision-Language-Action (VLA) model built into their robots!It has full upper body control! What blew my mind: they showed two robots working together, handing objects to each other, based on natural language commands! As I watched, I exclaimed, "I haven't seen a humanoid robot, hand off stuff to the other one... I found it like super futuristically cool." The model runs on the robot, using a 7 billion parameter VLM for understanding and an 80 million parameter transformer for control. This is the future, folks! Tools & Others Microsoft's New Quantum Chip (and State of Matter!) Microsoft announced a new quantum chip and a new state of matter (called "topological superconductivity"). "I found it like absolutely mind blowing that they announced something like this," I gushed on the show. While I'm no quantum physicist, this sounds like a big deal for the future of computing. Verdict: Hayes Labs' Framework for LLM Judges And of course, the highlight of our show: Verdict, a new open-source framework from Hayes Labs (the folks behind those "bijection" jailbreaks!) for composing LLM judges. This is a huge deal for anyone working on evaluation. Leonard and Nimit from Hayes Labs joined us to explain how Verdict addresses some of the core problems with LLM-as-a-judge: biases (like preferring their own responses!), sensitivity to prompts, and the challenge of "meta-evaluation" (how do you know your judge is actually good?). Verdict lets you combine different judging techniques ("primitives") to create more robust and efficient evaluators. Think of it as "judge-time compute scaling," as Leonard called it. They're achieving near state-of-the-art results on benchmarks like ExpertQA, and it's designed to be fast enough to use as a guardrail in real-time applications! One key insight: you don't always need a full-blown reasoning model for judging. As Nimit explained, Verdict can combine simpler LLM calls to achieve similar results at a fraction of the cost. And, it's open source! (Paper, Github,X). Conclusion Another week, another explosion of AI breakthroughs! Here are my key takeaways: * Open Source is THRIVING: From censorship-free LLMs to cutting-edge video models, the open-source community is delivering incredible innovation. * The Need for Speed (and Efficiency): Whether it's faster video generation or more efficient LLM judging, performance is key. * Robots are Getting Smarter (and More Collaborative): Figure's Helix is a glimpse into a future where robots work together. * Evaluation is (Finally) Getting Attention: Tools like Verdict are essential for building reliable and trustworthy AI systems. * The Big Players are Feeling the Heat: OpenAI's open-source tease and XAI's rapid progress show that the competition is fierce. I'll be back in my usual setup next week, ready to break down all the latest AI news. Stay tuned to ThursdAI – and don't forget to give the pod five stars and subscribe to the newsletter for all the links and deeper dives. There’s potentially an Anthropic announcement coming, so we’ll see you all next week. TLDR * Open Source LLMs * Perplexity R1 1776 - finetune of china-less R1 (Blog, Model) * Arc institute + Nvidia - introduce EVO 2 - genomics model (X) * ZeroBench - impossible benchmark for VLMs (X, Page, Paper, HF) * HuggingFace ultra scale playbook (HF) * Big CO LLMs + APIs * Grok 3 SOTA LLM + reasoning and Deep Search (blog, try it) * OpenAI is about to open source something? Sam posts a polls * This weeks Buzz * We are about to launch an agents course! Pre-sign up wandb.me/agents * Workshops are SOLD OUT * Watch my talk LIVE from AI Engineer - 11am EST Friday (HERE) * Keep watching AI Eng conference after the show on AIE YT * ) * Vision & Video * Microsoft MUSE - playable worlds from one image (X, HF, Blog) * Microsoft OmniParser - Better, faster screen parsing for GUI agents with OmniParser v2 (Gradio Demo) * HAO AI - fastVIDEO - making HY-Video 3x as fast (Github) * StepFun - Step-Video-T2V (+Turbo), a SotA 30B text-to-video model (Paper, Github, HF, Try It) * Figure announces HELIX - vision action model built into FIGURE Robot (Paper) * Tools & Others * Microsoft announces a new quantum chip and a new state of matter (Blog, X) * Verdict - Framework to compose SOTA LLM judges with JudgeTime Scaling (Paper, Github,X) This is a public episode. If you'd like to discuss this with other subscribers or get access to bonus episodes, visit sub.thursdai.news/subscribe | |||
28 Feb 2025 | 📆 Feb 27, 2025 - GPT-4.5 Drops TODAY?!, Claude 3.7 Coding BEAST, Grok's Unhinged Voice, Humanlike AI voices & more AI news | 01:40:30 | |
Hey all, Alex here 👋 What can I say, the weeks are getting busier , and this is one of those "crazy full" weeks in AI. As we were about to start recording, OpenAI teased GPT 4.5 live stream, and we already had a very busy show lined up (Claude 3.7 vibes are immaculate, Grok got an unhinged voice mode) and I had an interview with Kevin Hou from Windsurf scheduled! Let's dive in! 🔥 GPT 4.5 (ORION) is here - worlds largest LLM (10x GPT4o) OpenAI has finally shipped their next .5 model, which is 10x scale from the previous model. We didn't cover this on the podcast but did watch the OpenAI live stream together after the podcast concluded. A very interesting .5 release from OpenAI, where even Sam Altman says "this model won't crush on benchmarks" and is not the most frontier model, but is OpenAI's LARGEST model by far (folks are speculating 10+ Trillions of parameters) After 2 years of smaller models and distillations, we finally got a new BIG model, that shows scaling laws proper, and while on some benchmarks it won't compete against reasoning models, this model will absolutely fuel a huge increase in capabilities even for reasoners, once o-series models will be trained on top of this. Here's a summary of the announcement and quick vibes recap (from folks who had access to it before) * OpenAI's largest, most knowledgeable model. * Increased world knowledge: 62.5% on SimpleQA, 71.4% GPQA * Better in creative writing, programming, problem-solving (no native step-by-step reasoning). * Text and image input and text output * Available in ChatGPT Pro and API access (API supports Function Calling, Structured Output) * Knowledge Cutoff is October 2023. * Context Window is 128,000 tokens. * Max Output is 16,384 tokens. * Pricing (per 1M tokens): Input: $75, Output: $150, Cached Input: $37.50. * Foundation for future reasoning models 4.5 Vibes Recap Tons of folks who had access are pointing to the same thing, while this model is not beating others on evals, it's much better at multiple other things, namely creative writing, recommending songs, improved vision capability and improved medical diagnosis. Karpathy said "Everything is a little bit better and it's awesome, but also not exactly in ways that are trivial to point to" and posted a thread of pairwise comparisons of tone on his X thread Though the reaction is bifurcated as many are upset with the high price of this model (10x more costly on outputs) and the fact that it's just marginally better at coding tasks. Compared to the newerSonnet (Sonnet 3.7) and DeepSeek, folks are looking at OpenAI and asking, why isn't this way better? Anthropic's Claude 3.7 Sonnet: A Coding Powerhouse Anthropic released Claude 3.7 Sonnet, and the immediate reaction from the community was overwhelmingly positive. With 8x more output capability (64K) and reasoning built in, this model is an absolute coding powerhouse. Claude 3.7 Sonnet is the new king of coding models, achieving a remarkable 70% on the challenging SWE-Bench benchmark, and the initial user feedback is stellar, though vibes started to shift a bit towards Thursday. Ranking #1 on WebDev arena, and seemingly trained on UX and websites, Claude Sonnet 3.7 (AKA NewerSonner) has been blowing our collective minds since it was released on Monday, especially due to introducing Thinking and reasoning in a combined model. Now, since the start of the week, the community actually had time to play with it, and some of them return to sonnet 3.5 and saying that while the model is generally much more capable, it tends to generate tons of things that are unnecessary. I wonder if the shift is due to Cursor/Windsurf specific prompts, or the model's larger output context, and we'll keep you updated on if the vibes shift again. Open Source LLMs This week was HUGE for open source, folks. We saw releases pushing the boundaries of speed, multimodality, and even the very way LLMs generate text! DeepSeek's Open Source Spree DeepSeek went on an absolute tear, open-sourcing a treasure trove of advanced tools and techniques: This isn't your average open-source dump, folks. We're talking FlashMLA (efficient decoding on Hopper GPUs), DeepEP (an optimized communication library for MoE models), DeepGEMM (an FP8 GEMM library that's apparently ridiculously fast), and even parallelism strategies like DualPipe and EPLB. They are releasing some advanced stuff for training and optimization of LLMs, you can follow all their releases on their X account Dual Pipe seems to be the one that got most attention from the community, which is an incredible feat in pipe parallelism, that even got the cofounder of HuggingFace super excited Microsoft's Phi-4: Multimodal and Mini (Blog, HuggingFace) Microsoft joined the party with Phi-4-multimodal (5.6B parameters) and Phi-4-mini (3.8B parameters), showing that small models can pack a serious punch. These models are a big deal. Phi-4-multimodal can process text, images, and audio, and it actually beats WhisperV3 on transcription! As Nisten said, "This is a new model and, I'm still reserving judgment until, until I tried it, but it looks ideal for, for a portable size that you can run on the phone and it's multimodal." It even supports a wide range of languages. Phi-4-mini, on the other hand, is all about speed and efficiency, perfect for finetuning. Diffusion LLMs: Mercury Coder and LLaDA (X , Try it) This is where things get really interesting. We saw not one, but two diffusion-based LLMs this week: Mercury Coder from Inception Labs and LLaDA 8B. (Although, ok, to be fair, LLaDa released 2 weeks ago I was just busy) For those who don't know, diffusion is usually used for creating things like images. The idea of using it to generate text is like saying, "Okay, there's a revolutionary tool for painting; I'll write the code using it." Inception Labs' Mercury Coder is claiming over 1000 tokens per second on NVIDIA H100s – that's insane speed, usually only seen with specialized chips! Nisten spent hours digging into these, noting, "This is a complete breakthrough and, it just hasn't quite hit yet that this just happened because people thought for a while it should be possible because then you can do, you can do multiple token prediction at once". He explained that these models combine a regular LLM with a diffusion component, allowing them to generate multiple tokens simultaneously and excel at tasks like "fill in the middle" coding. LLaDA 8B, on the other hand, is an open-source attempt, and while it needs more training, it shows the potential of this approach. LDJ pointed out that LLaDA is "trained on like around five times or seven times less data while already like competing with LLAMA3 AP with same parameter count". Are diffusion LLMs the future? It's too early to say, but the speed gains are very intriguing. Magma 8B: Robotics LLM from Microsoft Microsoft dropped Magma 8B, a Microsoft Research project, an open-source model that combines vision and language understanding with the ability to control robotic actions. Nisten was particularly hyped about this one, calling it "the robotics. LLM." He sees it as a potential game-changer for robotics companies, allowing them to build robots that can understand visual input, respond to language commands, and act in the real world. OpenAI's Deep Research for Everyone (Well, Plus Subscribers) OpenAI finally brought Deep Research, its incredible web-browsing and research tool, to Plus subscribers. I've been saying this for a while: Deep Research is another ChatGPT moment. It's that good. It goes out, visits websites, understands your query in context, and synthesizes information like nothing else. As Nisten put it, "Nothing comes close to OpenAI's Deep Research...People like pull actual economics data, pull actual stuff." If you haven't tried it, you absolutely should. Our full coverage of Deep Research is here if you haven't yet listened, it's incredible. Alexa Gets an AI Brain Upgrade with Alexa+ Amazon finally announced Alexa+, the long-awaited LLM-powered upgrade to its ubiquitous voice assistant. Alexa+ will be powered by Claude (and sometimes Nova), offering a much more conversational and intelligent experience, with integrations across Amazon services. This is a huge deal. For years, Alexa has felt… well, dumb, compared to the advancements in LLMs. Now, it's getting a serious intelligence boost, thanks to Anthropic's Claude. It'll be able to handle complex conversations, control smart home devices, and even perform tasks across various Amazon services. Imagine asking Alexa, "Did I let the dog out today?" and it actually checking your Ring camera footage to give you an answer! (Although, as I joked, let's hope it doesn't start setting houses on fire.) Also very intriguing is the new SDKs they are releasing to connect Alexa+ to all kinds of experience, I think this is huge and will absolutely create a new industry of applications built for voice Alexa. Alexa Web Actions for example will allow Alexa to navigate to a website and complete actions (think order Uber Eats) The price? 20$/mo but free if you're a Amazon Prime subscriber, which is most of the US households at this point. They are focusing on personalization and memory, though still unclear how that's going to be handled, and the ability to share documents like schedules I'm very much looking forward to smart Alexa, and to be able to say "Alexa, set a timer for the amount of time it takes to hard boil an egg, and flash my house lights when the timer is done" Grok Gets a Voice... and It's UNHINGED Grok, Elon Musk's AI, finally got a voice mode, and… well, it's something else. One-sentence summary: Grok's new voice mode includes an "unhinged" 18+ option that curses like a sailor, along with other personality settings. Yes, you read that right. There's literally an "unhinged" setting in the UI. We played it live on the show, and... well, let's just say it's not for the faint of heart (or for kids). Here's a taste: Alex: "Hey there." Grok: "Yo, Alex. What's good, you horny b*****d? How's your day been so far? Fucked up or just mildly shitty?" Beyond the shock value, the voice mode is actually quite impressive in its expressiveness and ability to understand interruptions. It has several personalities, from a helpful "Grok Doc" to an "argumentative" mode that will disagree with everything you say. It's... unique. This Week's Buzz (WandB-Related News) Agents Course is Coming! We announced our upcoming agents course! You can pre-sign up HERE . This is going to be a deep dive into building and deploying AI agents, so don't miss it! AI Engineer Summit Recap We briefly touched on the AI Engineer Summit in New York, where we met with Kevin Hou and many other brilliant minds in the AI space. The theme was "Agents at Work," and it was a fantastic opportunity to see the latest developments in agent technology. I gave a talk about reasoning agents and had a workshop about evaluations on Saturday, and saw many listeners of ThursdAI 👏 ✋ Interview with Kevin Hou from Windsurf This week we had the pleasure of chatting with Kevin Hou from Windsurf about their revolutionary AI editor. Windsurf isn't just another IDE, it's an agentic IDE. As Kevin explained, "we made the pretty bold decision of saying, all right, we're not going to do chat... we are just going to [do] agent." They've built Windsurf from the ground up with an agent-first approach, and it’s making waves. Kevin walked us through the evolution of AI coding tools, from autocomplete to chat, and now to agents. He highlighted the "magical experiences" users are having, like debugging complex code with AI assistance that actually understands the context. We also delved into the challenges – memory, checkpointing, and cost. We also talked about the burning question: vibe coding. Is coding as we know it dead? Kevin’s take was nuanced: "there's an in between state that I really vibe or like gel with, which is,the scaffolding of what you want… Let's use, let's like vibe code and purely use the agent to accomplish this sort of commit." He sees AI agents raising the bar for software quality, demanding better UX, testing, and overall polish. And of course, we had to ask about the elephant in the room – why are so many people switching from Cursor to Windsurf? Kevin's answer was humble, pointing to user experience, the agent-first workflow, and the team’s dedication to building the best product. Check out our full conversation on the pod and download Windsurf for yourself: windsurf.ai Video Models & Voice model updates There is so much happening in LLM world, that folks may skip over the other stuff, but there's so much happening in these world's as well this week! Here's a brief recap! * Alibaba's WanX: Open-sourced, cutting-edge video generation models making waves with over 250,000 downloads already. They claim to take SOTA on open source video generation evals and of course img2video of this high quality model will lead to ... folks using it for all kinds of things. * HUMEs Octave: A groundbreaking LLM model that genuinely understands context and emotion and does TTS. Blog Hume has been doing emotional TTS but with this TTS focused LLM we are now able to create voices with a prompt, and receive emotional responses that are inferred from the text. Think shyness, sarcasm, anger etc * 11labs’ Scribe: Beating Whisper 3 with impressive accuracy and diarization features, Scribe is raising the bar in speech-to-text quality. 11labs releasing their own ASR (automatic speech recognition) was not in my cards, and boy did they deliver. Beating whisper, with speaker separation (diarization), world level timestamps and much lower WER than other models, this is a very interesting entry to this space. However, free for now on their website, it's significantly slower than Gemini 2.0 and Whisper for me at least. * Sesame releases their conversational speech model (and promising to open source this) and it's honestly the best / least uncanny conversations I had with an AI. Check out my conversation with it * Lastly, VEO 2, the best video model around according to some, is finally available via API (though txt2video only) and it's fairly expensive, but gives some amazing results. You can try it out on FAL Phew, it looks like we've made it! Huge huge week in AI, big 2 new models, tons of incredible updates on multimodality and voice as well 🔥 If you enjoyed this summary, the best way to support us is to share with a friend (or 3) and give us a 5 start reviews on wherever you get your podcasts, it really does help! 👏 See you next week, Alex This is a public episode. If you'd like to discuss this with other subscribers or get access to bonus episodes, visit sub.thursdai.news/subscribe | |||
06 Mar 2025 | ThursdAI - Mar 6, 2025 - Alibaba's R1 Killer QwQ, Exclusive Google AI Mode Chat, and MCP fever sweeping the community! | 01:50:59 | |
What is UP folks! Alex here from Weights & Biases (yeah, still, but check this weeks buzz section below for some news!) I really really enjoyed today's episode, I feel like I can post it unedited it was so so good. We started the show with our good friend Junyang Lin from Alibaba Qwen, where he told us about their new 32B reasoner QwQ. Then we interviewed Google's VP of the search product, Robby Stein, who came and told us about their upcoming AI mode in Google! I got access and played with it, and it made me switch back from PPXL as my main. And lastly, I recently became fully MCP-pilled, since we covered it when it came out over thanksgiving, I saw this acronym everywhere on my timeline but only recently "got it" and so I wanted to have an MCP deep dive, and boy... did I get what I wished for! You absolutely should tune in to the show as there's no way for me to cover everything we covered about MCP with Dina and Jason! ok without, further adieu.. let's dive in (and the TL;DR, links and show notes in the end as always!) ThursdAI - Recaps of the most high signal AI weekly spaces is a reader-supported publication. To receive new posts and support my work, consider becoming a free or paid subscriber. 🤯 Alibaba's QwQ-32B: Small But Shocking Everyone! The open-source LLM segment started strong, chatting with friend of the show Junyang Justin Lin from Alibaba’s esteemed Qwen team. They've cooked up something quite special: QwQ-32B, a reasoning-focused, reinforcement-learning-boosted beast that punches remarkably above its weight. We're talking about a mere 32B parameters model holding its ground on tough evaluations against DeepSeek R1, a 671B behemoth! Here’s how wild this is: You can literally run QwQ on your Mac! Junyang shared that they applied two solid rounds of RL to amp its reasoning, coding, and math capabilities, integrating agents into the model to fully unlock its abilities. When I called out how insane it was that we’ve gone from "LLMs can't do math" to basically acing competitive math benchmarks like AIME24, Junyang calmly hinted that they're already aiming for unified thinking/non-thinking models. Sounds wild, doesn’t it? Check out the full QwQ release here, or dive into their blog post. 🚀 Google Launches AI Mode: Search Goes Next-Level (X, Blog, My Live Reaction). For the past two years, on this very show, we've been asking, "Where's Google?" in the Gen AI race. Well, folks, they're back. And they're back in a big way. Next, we were thrilled to have Google’s own Robby Stein, VP of Product for Google Search, drop by ThursdAI after their massive launch of AI Mode and expanded AI Overviews leveraging Gemini 2.0. Robby walked us through this massive shift, which essentially brings advanced conversational AI capabilities straight into Google. Seriously — Gemini 2.0 is now out here doing complex reasoning while performing fan-out queries behind the scenes in Google's infrastructure. Google search is literally Googling itself. No joke. "We actually have the model generating fan-out queries — Google searches within searches — to collect accurate, fresh, and verified data," explained Robby during our chat. And I gotta admit, after playing with AI Mode, Google is definitely back in the game—real-time restaurant closures, stock analyses, product comparisons, and it’s conversational to boot. You can check my blind reaction first impression video here. (also, while you're there, why not subscribe to my YT?) Google has some huge plans, but right now AI Mode is rolling out slowly via Google Labs for Google One AI Premium subscribers first. More soon though! 🐝 This Week's Buzz: Weights & Biases Joins CoreWeave Family! Huge buzz (in every sense of the word) from Weights & Biases, who made waves with their announcement this week: We've joined forces with CoreWeave! Yeah, that's big news as CoreWeave, the AI hyperscaler known for delivering critical AI infrastructure, has now acquired Weights & Biases to build the ultimate end-to-end AI platform. It's early days of this exciting journey, and more details are emerging, but safe to say: the future of Weights & Biases just got even more exciting. Congrats to the whole team at Weights & Biases and our new colleagues at CoreWeave! We're committed to all users of WandB so you will be able to keep using Weights & Biases, and we'll continuously improve our offerings going forward! Personally, also nothing changes for ThursdAI! 🎉 MCP Takes Over: Giving AI agents super powers via standardized protocol Then things got insanely exciting. Why? Because MCP is blowing up and I had to find out why everyone's timeline (mine included) just got invaded. Welcoming Cloudflare’s amazing product manager Dina Kozlov and Jason Kneen—MCP master and creator—things quickly got mind-blowing. MCP servers, Jason explained, are essentially tool wrappers that effortlessly empower agents with capabilities like API access and even calling other LLMs—completely seamlessly and securely. According to Jason, "we haven't even touched the surface yet of what MCP can do—these things are Lego bricks ready to form swarms and even self-evolve." Dina broke down just how easy it is to launch MCP servers on Cloudflare Workers while teasing exciting upcoming enhancements. Both Dina and Jason shared jaw-dropping examples, including composing complex workflows connecting Git, Jira, Gmail, and even smart home controls—practically instantaneously! Seriously, my mind is still spinning. The MCP train is picking up steam, and something tells me we'll be talking about this revolutionary agent technology a lot more soon. Check out two great MCP directories that popped up this recently: Smithery, Cursor Directory and Composio. This show was one of the best ones we recorded, honestly, I barely need to edit it. It was also a really really fun livestream, so if you prefer seeing to listening, here's the lightly edited live stream Thank you for being a ThursdAI subscriber, as always here's the TL:DR and shownotes for everything that happened in AI this week and the things we mentioned (and hosts we had) ThursdAI - Recaps of the most high signal AI weekly spaces is a reader-supported publication. To receive new posts and support my work, consider becoming a free or paid subscriber. TL;DR and Show Notes * Show Notes & Guests * Alex Volkov - AI Eveangelist & Weights & Biases (@altryne) * Co Hosts - @WolframRvnwlf @ldjconfirmed @nisten * Junyang Justin Lin - Head of Qwen Team, Alibaba - @JustinLin610 * Robby Stein - VP of Product, Google Search - @rmstein * Dina Kozlov - Product Manager, Cloudflare - @dinasaur_404 * Jason Kneen - MCP Wiz - @jasonkneen * My Google AI Mode Blind Reaction Video (Youtube) * Sesame Maya Conversation Demo - (Youtube) * Cloudflare MCP docs (Blog) * Weights & Biases Agents Course Pre-signup - https://wandb.me/agents * Open Source LLMs * Qwen's latest reasoning model QwQ-32B - matches R1 on some evals (X, Blog, HF, Chat) * Cohere4ai - Aya Vision - 8B & 32B (X, HF) * AI21 - Jamba 1.6 Large & Jamba 1.6 Mini (X, HF) * Big CO LLMs + APIs * Google announces AI Mode & AI Overviews Gemini 2.0 (X, Blog, My Live Reaction) * OpenAI rolls out GPT 4.5 to plus users - #1 on LM Arena 🔥 (X) * Grok Voice is available for free users as well (X) * Elysian Labs launches Auren ios app (X, App Store) * Mistral announces SOTA OCR (Blog) * This weeks Buzz * Weights & Biases is acquired by CoreWeave 🎉 (Blog) * Vision & Video * Tencent HYVideo img2vid is finally here (X, HF, Try It) * Voice & Audio * NotaGen - symbolic music generation model high-quality classical sheet music Github, Demo, HF * Sesame takes the world by storm with their amazing voice model (My Reaction) * AI Art & Diffusion & 3D * MiniMax__AI - Image-01: A Versatile Text-to-Image Model at 1/10 the Cost (X, Try it) * Zhipu AI - CogView 4 6B - (X, Github) * Tools * Google - DataScience agent in GoogleColab Blog * Baidu Miaoda - nocode AI build tool This is a public episode. If you'd like to discuss this with other subscribers or get access to bonus episodes, visit sub.thursdai.news/subscribe | |||
13 Mar 2025 | 📆 ThursdAI Turns Two! 🎉 Gemma 3, Gemini Native Image, new OpenAI tools, tons of open source & more AI news | 01:32:04 | |
LET'S GO! Happy second birthday to ThursdAI, your favorite weekly AI news show! Can you believe it's been two whole years since we jumped into that random Twitter Space to rant about GPT-4? From humble beginnings as a late-night Twitter chat to a full-blown podcast, Newsletter and YouTube show with hundreds of thousands of downloads, it's been an absolutely wild ride! That's right, two whole years of me, Alex Volkov, your friendly AI Evangelist, along with my amazing co-hosts, trying to keep you up-to-date on the breakneck speed of the AI world And what better way to celebrate than with a week PACKED with insane AI news? Buckle up, folks, because this week Google went OPEN SOURCE crazy, Gemini got even cooler, OpenAI created a whole new Agents SDK and the open-source community continues to blow our minds. We’ve got it all - from game-changing model releases to mind-bending demos. This week I'm also on the Weights & Biases company retreat, so TL;DR first and then the newsletter, but honestly, I'll start embedding the live show here in the substack from now on, because we're getting so good at it, I barely have to edit lately and there's a LOT to show you guys! TL;DR and Show Notes & Links * Hosts & Guests * Alex Volkov - AI Eveangelist & Weights & Biases (@altryne) * Co Hosts - @WolframRvnwlf @ldjconfirmed @nisten * Sandra Kublik - DevRel at Cohere (@itsSandraKublik) * Open Source LLMs * Google open sources Gemma 3 - 1B - 27B - 128K context (Blog, AI Studio, HF) * EuroBERT - multilingual encoder models (210M to 2.1B params) * Reka Flash 3 (reasoning) 21B parameters is open sourced (Blog, HF) * Cohere Command A 111B model - 256K context (Blog) * Nous Research Deep Hermes 24B / 3B Hybrid Reasoners (X, HF) * AllenAI OLMo 2 32B - fully open source GPT4 level model (X, Blog, Try It) * Big CO LLMs + APIs * Gemini Flash generates images natively (X, AI Studio) * Google deep research is now free in Gemini app and powered by Gemini Thinking (Try It no cost) * OpenAI released new responses API, Web Search, File search and Computer USE tools (X, Blog) * This weeks Buzz * The whole company is at an offsite at oceanside, CA * W&B internal MCP hackathon and had cool projects - launching an MCP server soon! * Vision & Video * Remade AI - 8 LORA video effects for WANX (HF) * AI Art & Diffusion & 3D * ByteDance Seedream 2.0 - A Native Chinese-English Bilingual Image Generation Foundation Model by ByteDance (Blog, Paper) * Tools * Everyone's talking about Manus - (manus.im) * Google AI studio now supports youtube understanding via link dropping Open Source LLMs: Gemma 3, EuroBERT, Reka Flash 3, and Cohere Command-A Unleashed! This week was absolutely HUGE for open source, folks. Google dropped a BOMBSHELL with Gemma 3! As Wolfram pointed out, this is a "very technical achievement," and it's not just one model, but a whole family ranging from 1 billion to 27 billion parameters. And get this – the 27B model can run on a SINGLE GPU! Sundar Pichai himself claimed you’d need "at least 10X compute to get similar performance from other models." Insane! Gemma 3 isn't just about size; it's packed with features. We're talking multimodal capabilities (text, images, and video!), support for over 140 languages, and a massive 128k context window. As Nisten pointed out, "it might actually end up being the best at multimodal in that regard" for local models. Plus, it's fine-tuned for safety and comes with ShieldGemma 2 for content moderation. You can grab Gemma 3 on Google AI Studio, Hugging Face, Ollama, Kaggle – everywhere! Huge shoutout to Omar Sanseviero and the Google team for this incredible release and for supporting the open-source community from day one! Colin aka Bartowski, was right, "The best thing about Gemma is the fact that Google specifically helped the open source communities to get day one support." This is how you do open source right! Next up, we have EuroBERT, a new family of multilingual encoder models. Wolfram, our European representative, was particularly excited about this one: "In European languages, you have different characters than in other languages. And, um, yeah, encoding everything properly is, uh, difficult." Ranging from 210 million to 2.1 billion parameters, EuroBERT is designed to push the boundaries of NLP in European and global languages. With training on a massive 5 trillion-token dataset across 15 languages and support for 8K context tokens, EuroBERT is a workhorse for RAG and other NLP tasks. Plus, how cool is their mascot? Reka Flash 3 - a 21B reasoner with apache 2 trained with RLOO And the open source train keeps rolling! Reka AI dropped Reka Flash 3, a 21 billion parameter reasoning model with an Apache 2.0 license! Nisten was blown away by the benchmarks: "This might be one of the best like 20B size models that there is right now. And it's Apache 2.0. Uh, I, I think this is a much bigger deal than most people realize." Reka Flash 3 is compact, efficient, and excels at chat, coding, instruction following, and function calling. They even used a new reinforcement learning technique called REINFORCE Leave One-Out (RLOO). Go give it a whirl on Hugging Face or their chat interface – chat.reka.ai! Last but definitely not least in the open-source realm, we had a special guest, Sandra (@itsSandraKublik) from Cohere, join us to announce Command-A! This beast of a model clocks in at 111 BILLION parameters with a massive 256K context window. Sandra emphasized its efficiency, "It requires only two GPUs. Typically the models of this size require 32 GPUs. So it's a huge, huge difference." Command-A is designed for enterprises, focusing on agentic tasks, tool use, and multilingual performance. It's optimized for private deployments and boasts enterprise-grade security. Congrats to Sandra and the Cohere team on this massive release! Big CO LLMs + APIs: Gemini Flash Gets Visual, Deep Research Goes Free, and OpenAI Builds for Agents The big companies weren't sleeping either! Google continued their awesome week by unleashing native image generation in Gemini Flash Experimental! This is seriously f*****g cool, folks! Sorry for my French, but it’s true. You can now directly interact with images, tell Gemini what to do, and it just does it. We even showed it live on the stream, turning ourselves into cat-confetti-birthday-hat-wearing masterpieces! Wolfram was right, "It's also a sign what we will see in, like, Photoshop, for example. Where you, you expect to just talk to it and have it do everything that a graphic designer would be doing." The future of creative tools is HERE. And guess what else Google did? They made Deep Research FREE in the Gemini app and powered by Gemini Thinking! Nisten jumped in to test it live, and we were all impressed. "This is the nicest interface so far that I've seen," he said. Deep Research now digs through HUNDREDS of websites (Nisten’s test hit 156!) to give you comprehensive answers, and the interface is slick and user-friendly. Plus, you can export to Google Docs! Intelligence too cheap to meter? Google is definitely pushing that boundary. Last second additions - Allen Institute for AI released OLMo 2 32B - their biggest open model yet Just as I'm writing this, friend of the pod, Nathan from Allen Institute for AI announced the release of a FULLY OPEN OLMo 2, which includes weights, code, dataset, everything and apparently it beats the latest GPT 3.5, GPT 4o mini, and leading open weight models like Qwen and Mistral. Evals look legit, but nore than that, this is an Apache 2 model with everything in place to advance open AI and open science! Check out Nathans tweet for more info, and congrats to Allen team for this awesome release! OpenAI new responses API and Agent ASK with Web, File and CUA tools Of course, OpenAI wasn't going to let Google have all the fun. They dropped a new SDK for agents called the Responses API. This is a whole new way to build with OpenAI, designed specifically for the agentic era we're entering. They also released three new tools: Web Search, Computer Use Tool, and File Search Tool. The Web Search tool is self-explanatory – finally, built-in web search from OpenAI! The Computer Use Tool, while currently limited in availability, opens up exciting possibilities for agent automation, letting agents interact with computer interfaces. And the File Search Tool gives you a built-in RAG system, simplifying knowledge retrieval from your own files. As always, OpenAI is adapting to the agentic world and giving developers more power. Finally in the big company space, Nous Research released PORTAL, their new Inference API service. Now you can access their awesome models, like Hermes 3 Llama 70B and DeepHermes 3 8B, directly via API. It's great to see more open-source labs offering API access, making these powerful models even more accessible. This Week's Buzz at Weights & Biases: Offsite Hackathon and MCP Mania! This week's "This Week's Buzz" segment comes to you live from Oceanside, California! The whole Weights & Biases team is here for our company offsite. Despite the not-so-sunny California weather (thanks, storm!), it's been an incredible week of meeting colleagues, strategizing, and HACKING! And speaking of hacking, we had an MCP hackathon! After last week’s MCP-pilling episode, we were all hyped about Model Context Protocol, and the team didn't disappoint. In just three hours, the innovation was flowing! We saw agents built for WordPress, MCP support integrated into Weave playground, and even MCP servers for Weights & Biases itself! Get ready, folks, because an MCP server for Weights & Biases is COMING SOON! You'll be able to talk to your W&B data like never before. Huge shoutout to the W&B team for their incredible talent and for embracing the agentic future! And in case you missed it, Weights & Biases is now part of the CoreWeave family! Exciting times ahead! Vision & Video: LoRA Video Effects and OpenSora 2.0 Moving into vision and video, Remade AI released 8 LoRA video effects for 1X! Remember 1X from Alibaba? Now you can add crazy effects like "squish," "inflate," "deflate," and even "cakeify" to your videos using LoRAs. It's open source and super cool to see video effects becoming trainable and customizable. And in the realm of open-source video generation, OpenSora 2.0 dropped! This 11 billion parameter model claims state-of-the-art video generation trained for just $200,000! They’re even claiming performance close to Sora itself on some benchmarks. Nisten checked out the demos, and while we're all a bit jaded now with the rapid pace of video AI, it's still mind-blowing how far we've come. Open source video is getting seriously impressive, seriously fast. AI Art & Diffusion & 3D: ByteDance's Bilingual Seedream 2.0 ByteDance, the folks behind TikTok, released Seedream 2.0, a native Chinese-English bilingual image generation foundation model. This model, from ByteDream, excels at text rendering, cultural nuance, and human preference alignment. Seedream 2.0 boasts "powerful general capability," "native bilingual comprehension ability," and "excellent text rendering." It's designed to understand both Chinese and English prompts natively, generating high-quality, culturally relevant images. The examples look stunning, especially its ability to render Chinese text beautifully. Tools: Manus AI Agent, Google AI Studio YouTube Links, and Cursor Embeddings Finally, in the tools section, everyone's buzzing about Manus, a new AI research agent. We gave it a try live on the show, asking it to do some research. The UI is slick, and it seems to be using Claude 3.7 behind the scenes. Manus creates a to-do list, browses the web in a real Chrome browser, and even generates files. It's like Operator on steroids. We'll be keeping an eye on Manus and will report back on its performance in future episodes. And Google AI Studio keeps getting better! Now you can drop YouTube links into Google AI Studio, and it will natively understand the video! This is HUGE for video analysis and content understanding. Imagine using this for support, content summarization, and so much more. PHEW! What a week to celebrate two years of ThursdAI! From open source explosions to Gemini's visual prowess and OpenAI's agentic advancements, the AI world is moving faster than ever. As Wolfram aptly put it, "The acceleration, you can feel it." And Nisten reminded us of the incredible journey, "I remember I had early access to GPT-4 32K, and, uh, then... the person for the contract that had given me access, they cut it off because on the one weekend, I didn't realize how expensive it was. So I had to use $180 worth of tokens just trying it out." Now, we have models that are more powerful and more accessible than ever before. Thank you to Wolfram, Nisten, and LDJ for co-hosting and bringing their insights every week. And most importantly, THANK YOU to our amazing community for tuning in, listening, and supporting ThursdAI for two incredible years! We couldn't do it without you. Here's to another year of staying up-to-date so YOU don't have to! Don't forget to subscribe to the podcast, YouTube channel, and newsletter to stay in the loop. And share ThursdAI with a friend – it's the best birthday gift you can give us! Until next week, keep building and keep exploring the amazing world of AI! LET'S GO! This is a public episode. If you'd like to discuss this with other subscribers or get access to bonus episodes, visit sub.thursdai.news/subscribe | |||
20 Mar 2025 | ThursdAI - Mar 20 - OpenAIs new voices, Mistral Small, NVIDIA GTC recap & Nemotron, new SOTA vision from Roboflow & more AI news | 01:51:29 | |
Hey, it's Alex, coming to you fresh off another live recording of ThursdAI, and what an incredible one it's been! I was hoping that this week will be chill with the releases, because of NVIDIA's GTC conference, but no, the AI world doesn't stop, and if you blinked this week, you may have missed 2 or 10 major things that happened. From Mistral coming back to OSS with the amazing Mistral Small 3.1 (beating Gemma from last week!) to OpenAI dropping a new voice generation model, and 2! new whisper killer ASR models with a Breaking News during our live show (there's a reason we're called ThursdAI) which we watched together and then dissected with Kwindla, our amazing AI VOICE and real time expert. Not to mention that we also had dedicated breaking news from friend of the pod Joseph Nelson, that came on the show to announce a SOTA vision model from Roboflow + a new benchmark on which even the top VL models get around 6%! There's also a bunch of other OSS, a SOTA 3d model from Tencent and more! And last but not least, Yam is back 🎉 So... buckle up and let's dive in. As always, TL;DR and show notes at the end, and here's the YT live version. (While you're there, please hit subscribe and help me hit that 1K subs on YT 🙏 ) Voice & Audio: OpenAI's Voice Revolution and the Open Source Echo Hold the phone, everyone, because this week belonged to Voice & Audio! Seriously, if you weren't paying attention to the voice space, you missed a seismic shift, courtesy of OpenAI and some serious open-source contenders. OpenAI's New Voice Models - Whisper Gets an Upgrade, TTS Gets Emotional! OpenAI dropped a suite of next-gen audio models: gpt-4o-mini-tts-latest (text-to-speech) and GPT 4.0 Transcribe and GPT 4.0 Mini Transcribe (speech-to-text), all built upon their powerful transformer architecture. To unpack this voice revolution, we welcomed back Kwindla Cramer from Daily, the voice AI whisperer himself. The headline news? The new speech-to-text models are not just incremental improvements; they’re a whole new ballgame. As OpenAI’s Shenyi explained, "Our new generation model is based on our large speech model. This means this new model has been trained on trillions of audio tokens." They're faster, cheaper (Mini Transcribe is half price of Whisper!), and boast state-of-the-art accuracy across multiple languages. But the real kicker? They're promptable! "This basically opens up a whole field of prompt engineering for these models, which is crazy," I exclaimed, my mind officially blown. Imagine prompting your transcription model with context – telling it you're discussing dog breeds, and suddenly, its accuracy for breed names skyrockets. That's the power of promptable ASR! I recorded a live reaction aftder dropping of stream, and I was really impressed with how I can get the models to pronounce ThursdAI by just... asking! But the voice magic doesn't stop there. GPT 4.0 Mini TTS, the new text-to-speech model, can now be prompted for… emotions! "You can prompt to be emotional. You can ask it to do some stuff. You can prompt the character a voice," OpenAI even demoed a "Mad Scientist" voice! Captain Ryland voice, anyone? This is a huge leap forward in TTS, making AI voices sound… well, more human. But wait, there’s more! Semantic VAD! Semantic Voice Activity Detection, as OpenAI explained, "chunks the audio up based on when the model thinks The user's actually finished speaking." It’s about understanding the meaning of speech, not just detecting silence. Kwindla hailed it as "a big step forward," finally addressing the age-old problem of AI agents interrupting you mid-thought. No more robotic impatience! OpenAI also threw in noise reduction and conversation item retrieval, making these new voice models production-ready powerhouses. This isn't just an update; it's a voice AI revolution, folks. They also built a super nice website to test out the new models with openai.fm ! Canopy Labs' Orpheus 3B - Open Source Voice Steps Up But hold on, the open-source voice community isn't about to be outshone! Canopy Labs dropped Orpheus 3B, a "natural sounding speech language model" with open-source spirit. Orpheus, available in multiple sizes (3B, 1B, 500M, 150M), boasts zero-shot voice cloning and a glorious Apache 2 license. Wolfram noted its current lack of multilingual support, but remained enthusiastic, I played with them a bit and they do sound quite awesome, but I wasn't able to finetune them on my own voice due to "CUDA OUT OF MEMORY" alas I did a live reaction recording for this model on X NVIDIA Canary - Open Source Speech Recognition Enters the Race Speaking of open source, NVIDIA surprised us with Canary, a speech recognition and translation model. "NVIDIA open sourced Canary, which is a 1 billion parameter and 180 million parameter speech recognition and translation, so basically like whisper competitor," I summarized. Canary is tiny, fast, and CC-BY licensed, allowing commercial use. It even snagged second place on the Hugging Face speech recognition leaderboard! Open source ASR just got a whole lot more interesting. Of course, this won't get to the level of the new SOTA ASR OpenAI just dropped, but this can run locally and allows commercial use on edge devices! ThursdAI - Recaps of the most high signal AI weekly spaces is a reader-supported publication. To receive new posts and support my work, consider becoming a free or paid subscriber. Vision & Video: Roboflow's Visionary Model and Video Generation Gets Moving After the voice-apalooza, let's switch gears to the visual world, where Vision & Video delivered some knockout blows, spearheaded by Roboflow and StepFun. Roboflow's RF-DETR and RF100-VL - A New Vision SOTA Emerges Roboflow stole the vision spotlight this week with their RF-DETR model and the groundbreaking RF100-VL benchmark. We were lucky enough to have Joseph Nelson, Roboflow CEO, join the show again and give us the breaking news (they published the Github 11 minutes before he came on!) RF-DETR is Roboflow's first in-house model, a real-time object detection transformer that's rewriting the rulebook. "We've actually never released a model that we've developed. And so this is the first time where we've taken a lot of those learnings and put that into a model," Joseph revealed. And what a model it is! RF-DETR is not just fast; it's SOTA on real-world datasets and surpasses the 60 mAP barrier on COCO. But Joseph dropped a truth bomb: COCO is outdated. "The benchmark that everyone uses is, the COCO benchmark… hasn't been updated since 2017, but models have continued to get really, really, really good. And so they're saturated the COCO benchmark," he explained. Enter RF100-VL, Roboflow's revolutionary new benchmark, designed to evaluate vision-language models on real-world data. "We, introduced a benchmark that we call RF 100 vision language," Joseph announced. The results? Shockingly low zero-shot performance on real-world vision tasks, highlighting a major gap in current models. Joseph's quiz question about QwenVL 2.5's zero-shot performance on RF100-VL revealed a dismal 5.8% accuracy. "So we as a field have a long, long way to go before we have zero shot performance on real world context," Joseph concluded. RF100-VL is the new frontier for vision, and RF-DETR is leading the charge! Plus, it runs on edge devices and is Apache 2 licensed! Roboflow, you legends! Check out the RF-DETR Blog Post, the RF-DETR Github, and the RF100-VL Benchmark for more details! StepFun's Image-to-Video TI2V - Animating Images with Style Stepping into the video arena, StepFun released their image2video model, TI2V. TI2V boasts impressive motion controls and generates high-quality videos from images and text prompts, especially excelling in anime-style video generation. Dive into the TI2V HuggingFace Space and TI2V Github to explore further. Open Source LLMs: Mistral's Triumphant Return, LG's Fridge LLM, NVIDIA's Nemotron, and ByteDance's RL Boost Let's circle back to our beloved Open Source LLMs, where this week was nothing short of a gold rush! Mistral is BACK, Baby! - Mistral Small 3.1 24B (Again!) Seriously, Mistral AI's return to open source with Mistral Small 3.1 deserves another shoutout! "Mistral is back with open source. Let's go!" I cheered, and I meant it. This multimodal, Apache 2 licensed model is a powerhouse, outperforming Gemma 3 and ready for action on a single GPU. Wolfram, ever the pragmatist, noted, "We are in right now, where a week later, you already have some new toys to play with." referring to Gemma 3 that we covered just last week! Not only did we get a great new update from Mistral, they also cited our friends at Nous research and their Deep Hermes (released just last week!) for the reason to release the base models alongside finetuned models! Mistral Small 3.1 is not just a model; it's a statement: open source is thriving, and Mistral is leading the charge! Check out their Blog Post, the HuggingFace page, and the Base Model on HF. NVIDIA Nemotron - Distilling, Pruning, Making Llama's Better NVIDIA finally dropped Llama Nemotron, and it was worth the wait! Nemotron Nano (8B) and Super (49B) are here, with Ultra (253B) on the horizon. These models are distilled, pruned, and, crucially, designed for reasoning with a hybrid architecture allowing you to enable and disable reasoning via a simple on/off switch in the system prompt! Beating other reasoners like QwQ on GPQA tasks, this distillined and pruned LLama based reasoner seems very powerful! Congrats to NVIDIA Chris Alexius (a friend of the pod) who co-authored the announcement, told me that FP8 is expected and when that drops, this model will also fit on a single H100 GPU, making it really great for enterprises who host on their own hardware. And yes, it’s ready for commercial use. NVIDIA, welcome to the open-source LLM party! Explore the Llama-Nemotron HuggingFace Collection and the Dataset. LG Enters the LLM Fray with EXAONE Deep 32B - Fridge AI is Officially a Thing LG, yes, that LG, surprised everyone by open-sourcing EXAONE Deep 32B, a "thinking model" from the fridge and TV giant. "LG open sources EXAONE and EXAONE Deep 32B thinking model," I announced, still slightly amused by the fridge-LLM concept. This 32B parameter model claims "superior capabilities" in reasoning, and while my live test in LM Studio went a bit haywire, quantization could be the culprit. It's non-commercial, but hey, fridge-powered AI is now officially a thing. Who saw that coming? Check out my Reaction Video, the LG Blog, and the HuggingFace page for more info. ByteDance's DAPO - Reinforcement Learning Gets Efficient From the creators of TikTok, ByteDance, comes DAPO, a new reinforcement learning method that's outperforming GRPO. DAPO promises 50% accuracy on AIME 2024 with 50% less training steps. Nisten, our RL expert, explained it's a refined GRPO, pushing the boundaries of RL efficiency. Open source RL is getting faster and better, thanks to ByteDance! Dive into the X thread, Github, and Paper for the technical details. Big CO LLMs + APIs: Google's Generosity, OpenAI's Oligarch Pricing, and GTC Mania Switching gears to the Big CO LLM arena, we saw Google making moves for the masses, OpenAI catering to the elite, and NVIDIA… well, being NVIDIA. Google Makes DeepResearch Free and Adds Canvas Google is opening up DeepResearch to everyone for FREE! DeepResearch, Gemini's advanced search mode, is now accessible without a Pro subscription. I really like it's revamped UI where you can see the thinking and the sources! I used it live on the show to find out what we talked about in the latest episode of ThursdAI, and it did a pretty good job! Plus, Google unveiled Canvas, letting you "build apps within Gemini and actually see them." Google is making Gemini more accessible and more powerful, a win for everyone. Here's a Tetris game it built for me and here's a markdown enabled word counter I rebuild every week before I send ThursdAI (making sure I don't send you 10K words every week 😅) OpenAI's O1 Pro API - Pricey Power for the Few OpenAI, in contrast, released O1 Pro API, but with a price tag that's… astronomical. "OpenAI makes O1-pro API available to oligarchs ($600/1mtok output!)," I quipped, highlighting the exclusivity. $600 per million output tokens? "If you code with this, if you vibe code with this, you better already have VCs backing your startup," I warned. O1 Pro might be top-tier performance, but it's priced for the 0.1%. NVIDIA GTC Recap - Jensen's Hardware Extravaganza NVIDIA GTC was, as always, a hardware spectacle. New GPUs (Blackwell Ultra, Vera Rubin, Feynman!), the tiny DGX Spark supercomputer, the GR00T robot foundation model, and the Blue robot – NVIDIA is building the AI future, brick by silicon brick. Jensen is the AI world's rockstar, and GTC is his sold-out stadium show. Check out Rowan Cheung's GTC Recap on X for a quick overview. Shoutout to our team at GTC and this amazingly timed logo shot I took from the live stream! Antropic adds Web Search We had a surprise at the end of the show, with Antropic releasing web search. It's a small thing, but for folks who use Cloud AI, it's very important. You can now turn on web search directly on Claude which makes it... the last frontier lab to enable this feature 😂 Congrats! AI Art & Diffusion & 3D: Tencent's 3D Revolution Tencent Hunyuan 3D 2.0 MV and Turbo - 3D Generation Gets Real-Time Tencent updated Hunyuan 3D to 2.0 MV (MultiView) and Turbo, pushing the boundaries of 3D generation. Hunyuan 3D 2.0 surpasses SOTA in geometry, texture, and alignment, and the Turbo version achieves near real-time 3D generation – under one second on an H100! Try out the Hunyuan3D-2mv HF Space to generate your own 3D masterpieces! MultiView (MV) is another game-changer, allowing you to input 1-4 views for more accurate 3D models. "MV allows to generate 3d shapes from 1-4 views making the 3D shapes much higher quality" I explained. The demo of generating a 3D mouse from Gemini-generated images showcased the seamless pipeline from thought to 3D object. I literally just asked Gemini with native image generation to generate a character and then Holodecks are getting closer, folks! Closing Remarks and Thank You And that's all she wrote, folks! Another week, another AI explosion. From voice to vision, open source to Big CO, this week was a whirlwind of innovation. Huge thanks again to our incredible guests, Joseph Nelson from Roboflow, Kwindla Cramer from Daily, and Lucas Atkins from ARCEE! And of course, massive shoutout to my co-hosts, Wolfram, Yam, and Nisten – you guys are the best! And YOU, the ThursdAI community, are the reason we do this. Thank you for tuning in, for your support, and for being as hyped about AI as we are. Remember, ThursdAI is a labor of love, fueled by Weights & Biases and a whole lot of passion. Missed anything? thursdai.news is your one-stop shop for the podcast, newsletter, and video replay. And seriously, subscribe to our YouTube channel! Let's get to 1000 subs! Helpful? We’d love to see you here again! TL;DR and Show Notes: * Guests and Cohosts * Alex Volkov - AI Evangelist & Weights & Biases (@altryne) Co Hosts - @WolframRvnwlf @yampeleg @nisten * Sponsor - Weights & Biases Weave (@weave_wb) * Joseph Nelson - CEO Roboflow (@josephofiowa) * Kindwla Kramer - CEO Daily (@kwindla) * Lucas Atkins - Labs team at Arcee lead (@LukasAtkins7) * Open Source LLMs * Mistral Small 3.1 24B - Multimodal (Blog, HF, HF base) * LG open sources EXAONE and EXAONE Deep 32B thinking model (Alex Reaction Video, LG BLOG, HF) * ByteDance releases DAPO - better than GRPO RL Method (X, Github, Paper) * NVIDIA drops LLama-Nemotron (Super 49B, Nano 8B) with reasoning and data (X, HF, Dataset) * Big CO LLMs + APIs * Google makes DeepResearch free, Canvas added, Live Previews (X) * OpenAI makes O1-pro API available to oligarchs ($600/1mtok output!) * NVIDIA GTC recap - (X) * This weeks Buzz * Come visit the Weights & Biases team at GTC today! * Vision & Video * Roboflow drops RF-DETR a SOTA vision model + new eval RF100-VL for VLMs (Blog, Github, Benchmark) * StepFun dropped their image2video model TI2V (HF, Github) * Voice & Audio * OpenAI launches a new voice model and 2 new transcription models (Blog, Youtube) * Canopy Labs drops Orpheus 3B (1B, 500B, 150M versions) - natural sounding speech language model (Blog, HF, Colab) * NVIDIA Canary 1B/180M Flash - apache 2 speech recognition and translation LLama finetune (HF) * AI Art & Diffusion & 3D * Tencent updates Hunyuan 3D 2.0 MV (MultiView) and Turbo (HF) * Tools * ARCEE Conductor - model router (X) * Cursor ships Claude 3.7 MAX (X) * Notebook LM teases MindMaps (X) * Gemini Co-Drawing - using Gemini native image output for helping drawing (HF) This is a public episode. If you'd like to discuss this with other subscribers or get access to bonus episodes, visit sub.thursdai.news/subscribe | |||
27 Mar 2025 | 📆 ThursdAI - Mar 27 - Gemini 2.5 Takes #1, OpenAI Goes Ghibli, DeepSeek V3 Roars, Qwen Omni, Wandb MCP & more AI news | 01:24:00 | |
Hey everyone, Alex here 👋 Welcome back to ThursdAI! And folks, what an absolutely insane week it's been in the world of AI. Seriously, as I mentioned on the show, we don't often get weeks this packed with game-changing releases. We saw Google emphatically reclaim the #1 LLM spot with Gemini 2.5 Pro (and OpenAI try really hard to hit back with a new ChatGPT), DeepSeek dropped a monster 685B parameter open-source model, Qwen launched a tiny but mighty 7B Omni model that handles voice and video like a champ, and OpenAI finally gave us native image generation in GPT-4o, immediately unleashing a tidal wave of Ghibli-fication across the internet. It was intense, with big players seemingly trying to one-up each other constantly – remember when Sam Altman dropped Advanced Voice Mode right when Google was about to show Astra? This weeks was this, on steroids. We had a fantastic show trying to unpack it all, joined by the brilliant Tulsee Doshi from the Google Gemini team, my Weights & Biases colleague Morgan McQuire talking MCP tools, and the MLX King himself, Prince Canuma. Plus, my awesome co-hosts Wolfram, Nisten, and Yam were there to add their insights. (watch the LIVE recap or keep reading and listen to the audio pod) So, grab your beverage of choice, buckle up, and let's try to make sense of this AI whirlwind! (TL'DR and show notes at the bottom 👇) Big CO LLMs + APIs 🔥 Google Reclaims #1 with Gemini 2.5 Pro (Thinking!) Okay, let's start with the big news. Google came out swinging this week, dropping Gemini 2.5 Pro and, based on the benchmarks and our initial impressions, taking back the crown for the best all-around LLM currently available. (Check out the X announcement, the official blog post, and seriously, go try it yourself at ai.dev). We were super lucky to have Tulsee Doshi, who leads the product team for Gemini modeling efforts at Google, join us on the show to give us the inside scoop. Gemini 2.5 Pro Experimental isn't just an incremental update; it's topping benchmarks in complex reasoning, science, math, and coding. As Tulsee explained, this isn't just about tweaking one thing – it's a combination of a significantly enhanced base model and improved post-training techniques, including integrating those "thinking" capabilities (like chain-of-thought) right into the core models. That's why they dropped "thinking" from the official name – it's not a separate mode anymore, it's becoming fundamental to how Gemini operates. Tulsee mentioned their goal is for the main line models to be thinking models, leveraging inference time when needed to get the best answer. This is a huge step towards more capable and reliable AI. The performance gains are staggering across the board. We saw massive jumps on benchmarks like AIME (up nearly 20 points!) and GPQA. But it's not just about the numbers. As Tulsee highlighted, Gemini 2.5 is proving to be incredibly well-rounded, excelling not only on academic benchmarks but also on human preference evaluations like LM Arena (where style control is key). The "vibes" are great, as Wolfram put it. My own testing on reasoning tasks confirms this – the latency is surprisingly low for such a powerful model (around 13 seconds on my hard reasoning questions compared to 45+ for others), and the accuracy is the highest I've seen yet at 66% on that specific challenging set. It also inherits the strengths of previous Gemini models – native multimodality and that massive long context window (up to 1M tokens!). Tulsee emphasized how crucial long context is, allowing the model to reason over entire code repos, large sets of financial documents, or research papers. The performance on long context tasks, like the needle-in-a-haystack test shown on Live Bench, is truly impressive, maintaining high accuracy even at 120k+ tokens where other models often falter significantly. Nisten mentioned on the show that while it's better than GPT-4o, it might not completely replace Sonnet 3.5 for him yet, especially for certain coding or medical tasks under 128k context. Still, the consensus is clear: Gemini 2.5 Pro is the absolute best model right now across categories. Go play with it! ARC-AGI 2 Benchmark Revealed (X, Interactive Blog) Also on the benchmark front, the challenging ARC-AGI 2 benchmark was revealed. This is designed to test tasks that are easy for humans but hard for LLMs. The initial results are sobering: base LLMs score 0% accuracy, and even current "thinking" models only reach about 4%. It highlights just how far we still have to go in developing truly robust AI reasoning, giving us another hill to climb. GPT-4o got another update (as I'm writing these words!) tied for #1 on LMArena, beating 4.5 How much does Sam want to win over Google? So much he's letting it ALL out. Just now, we saw an update from LMArena and Sam, about a NEW GPT-4o (2025-03-26) which jumps OVER GPT 4.5 (like.. what?) and lands at number 2 on the LM Arena, jumping over 3o points. Tied #1 in Coding, Hard Prompts. Top-2 across ALL categories. Besides getting very close to Gemini but not quite beating it, I gotta ask, what's the point of 4.5 then? Open Source LLMs The open-source community wasn't sleeping this week either, with some major drops! DeepSeek V3 Update - 685B Parameter Beast! The Whale Bros at DeepSeek silently dropped an update to their V3 model (X, HF), and it's a monster. We're talking 685 Billion parameters in a Mixture-of-Experts (MoE) architecture. This isn't R1 (their reasoning model), but the powerful base model that R1 was built upon (and supposedly R2 when it'll come out) The benchmark jumps from the previous version are huge, especially in reasoning: * MMLU-Pro: 75.9 → 81.2 (+5.3) * GPQA: 59.1 → 68.4 (+9.3) * AIME: 39.6 → 59.4 (+19.8) (Almost 20 points on competitive math!) * LiveCodeBench: 39.2 → 49.2 (+10.0) They're highlighting major boosts in reasoning, stronger front-end dev skills, and smarter tool use. Nisten noted it even gets some hard reasoning questions right that challenge other models. The "vibes" are reportedly great. Wolfram tried to run it locally but found even the 1-bit quantized version too large for his system (though it should theoretically fit in combined RAM/VRAM), but he's using it via API. It’s likely the best non-reasoning open model right now, potentially the best overall if you can run it. And huge news for the community – they've released these weights under the MIT License, just like R1! Massive respect to DeepSeek for continuing to push powerful models into the open. They also highlight being significantly better at Front End development and websites aesthetics. Qwen Launches Omni 7B Model - Voice & Video Chat! Our friends at Qwen (Alibaba) also came through with something super cool: Qwen2.5-Omni-7B (HF). This is an end-to-end multimodal model that can perceive text, images, audio, AND video, while generating both text and natural-sounding speech, potentially in real-time. They're using a "Thinker-Talker" architecture. What blew my mind is the size – it's listed as 7B parameters, though I saw a meme suggesting it might be closer to 11B internally (ah, the joys of open source!). Still, even at 11B, having this level of multimodal understanding and generation in a relatively small open model is fantastic. It understands voice and video natively and outputs text and voice. Now, when I hear "Omni," I start expecting image generation too (thanks, Google!), so maybe that's next for Qwen? 😉 AI Art & Diffusion & Auto-regression This was arguably where the biggest "mainstream" buzz happened this week, thanks mainly to OpenAI. OpenAI Launches Native Image Support in GPT-4o - Ghibli Everywhere! This felt like a direct response to Gemini 2.5's launch, almost like OpenAI saying, "Oh yeah? Watch this!" They finally enabled the native image generation capabilities within GPT-4o (Blog, Examples). Remember that image Greg Brockman tweeted a year ago of someone writing on a blackboard with an old OpenAI logo, hinting at this? Well, it's here. And the results? Honestly, they're stunning. The prompt adherence is incredible. It actually listens to what you ask for in detail, including text generation within images, which diffusion models notoriously struggle with. The realism can be jaw-dropping, but it can also generate various styles. Speaking of styles... the internet immediately lost its collective mind and turned everything into the style of Studio Ghibli (great X thread here). My entire feed became Ghibli-fied. It's a testament to how accessible and fun this feature is. Wolfram even suggested we should have Ghibli avatars for the show! Interestingly, this image generation is apparently auto-regressive, not based on diffusion models like Midjourney or Stable Diffusion. This is more similar to how models like Grok's Aurora work, generating the image sequentially (top-to-bottom, kinda like how old GIFs used to load, as Yam pointed out we confirmed). This likely contributes to the amazing prompt adherence, especially with text. The creative potential is huge – people are generating incredible ad concepts (like this thread) and even recreating entire movie trailers, like this unbelievable Lord of the Rings one (link), purely through prompts in GPT-4o. It's wild. Now, this launch wasn't just about cool features; it also marked a significant shift in OpenAI's policy around image generation, aiming for what CEO Sam Altman called "a new high-water mark for us in allowing creative freedom." Joanne Jang, who leads model behavior at OpenAI, shared some fascinating insights into their thinking (Reservoir Samples post). She explained they're moving away from broad, blanket refusals (which often felt safest but limited creativity) towards a more precise approach focused on preventing real-world harm. This means trusting user creativity more, not letting hypothetical worst-case scenarios overshadow everyday usefulness (like generating memes!), and valuing the "unknown, unimaginable possibilities" that overly strict rules might stifle. It's a nuanced approach acknowledging that, as Joanne quoted, "Ships are safest in the harbor... But that’s not what ships or models are for." A philosophy change I definitely appreciate. Reve - New SOTA Diffusion Contender? While OpenAI grabbed headlines, another player emerged claiming State-of-the-Art results, this time in the diffusion space. Reve Image 1.0 (X, Blog/News, Try it) apparently beats Midjourney and Flux in benchmarks, particularly in prompt adherence, realism, and even text generation (though likely not as consistently as GPT-4o's native approach). It works on a credit system ($5 for 500 generations, ~1 cent per image) which is quite affordable. The editing seems a bit different, relying on chatting with the model rather than complex tools. It was kind of hidden/anonymous before, but now they've revealed themselves. Honestly, this would probably be huge news if OpenAI hadn't dropped their image bomb the same week. Ideogram 3 Also Launched - Another SOTA Claim! And just to make the AI art space even more crowded this week, Ideogram also launched version 3.0 (Blog, Try it), also claiming state-of-the-art performance! Ideogram has always been strong with text rendering and logos. Version 3.0 boasts stunning realism, creative design capabilities, and a new "Style References" feature where you can upload images to guide the aesthetic. They claim it consistently outperforms others in human evaluations. It's wild – we had at least three major image generation models/updates drop this week, all claiming top performance, and none of them seemed to benchmark directly against each other in their launch materials! It’s hard to keep track! This Week's Buzz + MCP (X, Github!) Bringing it back to Weights & Biases for a moment. We had Morgan McQuire on the show, who heads up our AI Applied team, to talk about something we're really excited about internally – integrating MCP with Weave, our LLM observability and evaluation tool. Morgan showed a demo and have shipped the MCP server, which you can try right now! Coming soon is the integration with wandb models, which will allows ML folks around the world to build agents that monitor loss curves for them! Weights & Biases Weave Official MCP Server Tool - Talk to Your Evals! We've launched an official MCP server tool for Weave! What does this mean? If you're using Weave to track your experiments, evaluations, prompts, etc. (and you should be!), you can now literally chat with that data. As Morgan demonstrated, you can ask questions like "Tell me about my last three evaluations," and the MCP tool, connected to your Weave data, will not only fetch and summarize that information for you directly in the chat interface (like Claude code or others that support MCP) but will generate a report and add visualizations! This is just the beginning of how we see MCP enhancing observability and interaction with ML workflows. Being able to query and analyze your runs and evaluations using natural language is incredibly powerful. Agents, Tools & MCP And speaking of MCP... OpenAI Adds Support for MCP - MCP WON! This was HUGE news, maybe slightly overshadowed by the image generation, but potentially far more impactful long-term, as Wolfram pointed out right at the start of the show. OpenAI officially announced support for the Model Context Protocol (MCP) (docs here). Why is this massive? Because Anthropic initiated MCP, and there was a real fear that OpenAI, being OpenAI, might just create its own competing standard for agent/tool communication, leading to fragmentation (think VHS vs. Betamax, or Blu-ray vs. HD DVD – standards wars suck!). Instead, OpenAI embraced the existing standard. As I said on the show, MCP WON! This is crucial for the ecosystem. It means developers can build tools and agents using the MCP standard, and they should (hopefully) work seamlessly across different models like Claude and GPT. OpenAI not only added support but released it in their Agents SDK and explicitly stated support is "coming soon" for the ChatGPT desktop app and response APIs. Yam expertly clarified the distinction: tools are often single API calls, while MCPs are servers that can maintain state, allowing for more complex, guided interactions. Qwen also adding MCP support to their UI just reinforces this – the standard is gaining traction fast. This standardization is absolutely essential for building a robust agentic future. Voice & Audio Just one more quick update on the audio front: OpenAI Updates Advanced Voice Mode with Semantic VAD Alongside the image generation, OpenAI also quietly updated the advanced voice mode in ChatGPT (YT announcement). The key improvement is "Semantic VAD" (Voice Activity Detection). Instead of just cutting off when you pause (leading to annoying interruptions, especially for kids or people who think while speaking), it now tries to understand the meaning and tone to determine if you're actually finished talking. This should lead to a much more natural conversation flow. They also claim better personality, a more engaging natural tone (direct and concise), and less need for you to fill silence with "umms" just to keep the mic open. It might feel a tad slower because it waits a bit longer, but the improvement in interaction quality should be significant. MLX-Audio And speaking (heh) of audio and speech, we had the awesome Prince Canuma on the show! If you're into running models locally on Apple Silicon (Macs!), you probably know Prince. He's the MLX King, the creator and maintainer of essential libraries like MLX-VLM (for vision models), FastMLX, MLX Embeddings, and now, MLX-Audio. Seriously, huge props to Prince and the folks in the MLX community for making these powerful open-source models accessible on Mac hardware. It's an incredible contribution. This week, Prince released MLX-Audio v0.0.3. This isn't just text-to-speech (TTS); it aims to be a comprehensive audio package for MLX. Right now, it supports some of the best open TTS models out there: * Kokoro: The tiny, high-quality TTS model we've covered before. * Sesame 1B: Another strong contender. * Orpheus: From Canopy Labs (as Prince confirmed). * Suno Bark: Known for generating music and sound effects too. MLX-Audio makes running state-of-the-art speech synthesis locally on your Mac incredibly easy, basically offering a Hugging Face transformers pipeline equivalent but optimized for Apple Silicon. If you have a Mac, pip install mlx-audio and give it a spin! Prince also took a feature request on the show to allow text file input directly, so look out for that! Phew! See what I mean? An absolutely jam-packed week. Huge thanks again to Tulsee, Morgan, and Prince for joining us and sharing their insights, and to Wolfram, Nisten, and Yam for co-piloting through the news storm. And thank YOU all for tuning in! We'll be back next week, undoubtedly trying to catch our breath and make sense of whatever AI marvels (or madness) gets unleashed next. P.S - if the ghiblification trend didn’t get to your families as well, the alpha right now is… showing your kids how to be a magician and turn them into Ghibli characters, here are me and my kiddos (who asked to be pirates and princesses) TL;DR and Show Notes: * Guests and Cohosts * Alex Volkov - AI Evangelist & Weights & Biases (@altryne)Co Hosts - Wolfram Ravenwlf (@WolframRvnwlf), Nisten Tahiraj (@nisten), Yam Peleg (@yampeleg) * Tulsee Doshi - Head of Product, Gemini Models at Google DeepMind (@tulseedoshi) * Morgan McQuire - Head of AI Applied Team at Weights & Biases (@morgymcg) * Prince Canuma - ML Research Engineer, Creator of MLX Libraries (@PrinceCanuma) * Big CO LLMs + APIs * 🔥 Google reclaims #1 position with Gemini 2.5 Pro (thinking) - (X, Blog, Try it) * ARC-AGI 2 benchmark revealed - Base LLMs score 0%, thinking models 4%. * Open Source LLMs * Deepseek updates DeepSeek-V3-0324 685B params (X, HF) - MIT License! * Qwen launches an Omni 7B model - perceives text, image, audio, video & generates text and speech (HF) * AI Art & Diffusion & Auto-regression * OpenAI launches native image support in GPT-4o (Model Card, X thread, Ad threads, Full Lord of the Rings trailer, Model Card) * Reve - new SOTA diffusion image gen claims (X, Blog/News, Try) * Ideogram 3 launched - another SOTA claim, strong on text/logos, realism, style refs (Blog, Try it) * This weeks Buzz + MCP * Weights & Biases Weave official MCP server tool - talk to your evals! (X, Github) * Agents , Tools & MCP * OpenAI has added support for MCP - MCP WON! (Docs) * Voice & Audio * OpenAI updates advanced voice mode with semantic VAD for more natural conversations (YT announcement). * MLX-Audio v0.0.3 released by Prince Canuma (Github) * Show Notes and other Links * Catch the show live & subscribe to the newsletter/YouTube: thursdai.news/yt * Try Gemini 2.5 Pro: AI.dev * Learn more about MCP from our previous episode (March 6th). This is a public episode. If you'd like to discuss this with other subscribers or get access to bonus episodes, visit sub.thursdai.news/subscribe | |||
03 Apr 2025 | ThursdAI - Apr 3rd - OpenAI Goes Open?! Gemini Crushes Math, AI Actors Go Hollywood & MCP, Now with Observability? | 01:37:33 | |
Woo! Welcome back to ThursdAI, show number 99! Can you believe it? We are one show away from hitting the big 100, which is just wild to me. And speaking of milestones, we just crossed 100,000 downloads on Substack alone! [Insert celebratory sound effect here 🎉]. Honestly, knowing so many of you tune in every week genuinely fills me with joy, but also a real commitment to keep bringing you the the high-signal, zero-fluff AI news you count on. Thank you for being part of this amazing community! 🙏 And what a week it's been! I started out busy at work, playing with the native image generation in ChatGPT like everyone else (all 130 million of us!), and then I looked at my notes for today… an absolute mountain of updates. Seriously, one of those weeks where open source just exploded, big companies dropped major news, and the vision/video space is producing stuff that's crossing the uncanny valley. We’ve got OpenAI teasing a big open source release (yes, OpenAI might actually be open again!), Gemini 2.5 showing superhuman math skills, Amazon stepping into the agent ring, truly mind-blowing AI character generation from Meta, and a personal update on making the Model Context Protocol (MCP) observable. Plus, we had some fantastic guests join us live! So buckle up, grab your coffee (or whatever gets you through the AI whirlwind), because we have a lot to cover. Let's dive in! (as always, show notes and links in the end) OpenAI Makes Waves: Open Source Tease, Tough Evals & Billions Raised It feels like OpenAI was determined to dominate the headlines this week, hitting us from multiple angles. First, the potentially massive news: OpenAI is planning to release a new open source model in the "coming months"! Kevin Weil tweeted that they're working on a "highly capable open language model" and are actively seeking developer feedback through dedicated sessions (sign up here if interested) to "get this right." Word on the street is that this could be a powerful reasoning model. Sam Altman also cheekily added they won't slap on a Llama-style <700M user license limit. Seeing OpenAI potentially re-embrace its "Open" roots with a potentially SOTA model is huge. We'll be watching like hawks! Second, they dropped PaperBench, a brutal new benchmark evaluating an AI's ability to replicate ICML 2024 research papers from scratch (read paper, write code, run experiments, match results - no peeking at original code!). It's incredibly detailed (>8,300 tasks) and even includes meta-evaluation for the LLM judge they built (Nano-Eval framework also open sourced). The kicker? Claude 3.5 Sonnet (New) came out on top with just 21.0% replication score (human PhDs got 41.4%). Props to OpenAI for releasing an eval where they don’t even win. That’s what real benchmarking integrity looks like. You can find the code on GitHub and read the full paper here. Third, the casual 40 Billion Dollars funding round led by SoftBank. Valuing the company at 300 Billion. Yes, Billion with a B. More than Coke, more than Disney. The blog post was hilariously short for such a massive number. They also mentioned500 million weekly ChatGPT usersand the insane onboarding rate (1M users/hr!) thanks to native image generation, especially seeing huge growth in India. The scale is just mind-boggling. Oh, and for fun, try the new grumpy, EMO "Monday" voice in advanced voice mode. It's surprisingly entertaining. Open Source Powerhouses: Nomic & OpenHands Deliver SOTA Beyond the OpenAI buzz, the open source community delivered some absolute gems, and we had guests from two key projects join us! Nomic Embed Multimodal: SOTA Embeddings for Visual Docs Our friends at Nomic AI are back with a killer release! We had Zach Nussbaum on the show discussing Nomic Embed Multimodal. These are new 3B & 7B parameter embedding models (available on Hugging Face) built on Alibaba's excellent Qwen2.5-VL. They achieved SOTA on visual document retrieval by cleverly embedding interleaved text-image sequences – perfect for PDFs and complex webpages. Zach highlighted that they chose the Qwen base because high-performing open VLMs under 3B params are still scarce, making it a solid foundation. Importantly, the 7B model comes with an Apache 2.0 license, and they've open sourced weights, code, and data. They offer both a powerful multi-vector version (ColNomic) and a faster single-vector one. Huge congrats to Nomic! OpenHands LM 32B & Agent: Accessible SOTA Coding Remember OpenDevin? It evolved into OpenHands, and the team just dropped their own OpenHands LM 32B! We chatted with co-founder Xingyao "Elle" Wang about this impressive Qwen 2.5 finetune (MIT licensed, on Hugging Face). It hits a remarkable 37.2% on SWE-Bench Verified (a coding benchmark measuring real-world repo tasks), competing with much larger models. Elle stressed they didn't just chase code completion scores; they focused on tuning for agentic capabilities – tool use, planning, self-correction – using trajectories from their contamination-free Switch Execution dataset. This focus seems to be paying off, as the OpenHands agent also snagged the #2 spot on the brand new Live SWE-Bench leaderboard! Plus, the 32B model runs locally on a single 3090, making this power accessible. You can also try their managed OpenHands Cloud ($50 free credit). Amazing progress from this team! Frontiers: Diffusion LMs & Superhuman Math Two other developments pushed the boundaries this week: Dream 7B: A Diffusion Language Model Challenger? This one's fascinating conceptually. Researchers unveiled Dream 7B, a language model based on diffusion, not auto-regression. The benchmarks they shared show it competing strongly with top 7-8B models, and absolutely crushing tasks like Sudoku (81% vs <50% for others), potentially due to its parallel processing nature being better for global constraints. It's an exciting hint at alternative architectures, but the model weights aren't out yet, so we can't verify or play with it. Still, one to watch! Gemini 2.5 Obliterates Olympiad Math (24.4% on USAMO!) We already knew Gemini 2.5 was good, but wow. New results dropped showing its performance on the USA Math Olympiad (USAMO) – problems so hard most top models score under 5%. Gemini 2.5 Pro scored an incredible 24.4%! The gap between it and everything else is massive, highlighting the power of its reasoning and thinking capabilities (which you can inspect via its traces!). Having used it for complex tasks myself (like wrestling with tax forms!), I can attest to its depth. It's free in the Gemini app – go try it! Agents, Compute & Making MCP Observable Amazon's Nova Act Agent & The Need for Access Amazon entered the agent chat with Nova Act, designed for web browser actions. They claim it beats Claude 3.5 and OpenAI's QA model on some benchmarks, possibly leveraging acquired Adept talent. But... it's only available via an SDK with a request form. As Yam rightly pointed out on the show, these agent claims mean little until we can actually use them in the real world! CoreWeave + NVIDIA = Insane Speeds Hardware keeps accelerating. CoreWeave announced hitting 800 Tokens/sec on Llama 3.1 405B using NVIDIA's new GB200 Blackwell chips, and 33,000 T/s on Llama 2 70B with H200s. Inference is getting fast. This Week's Buzz: Let's Make MCP Observable! Okay, my personal mission this week builds on the growing Model Context Protocol (MCP) momentum. MCP is potentially the "HTTP for agents," enabling tool interoperability. But as tool use moves external, we lose visibility, making debugging and security harder. That's why I'm launching the Observable Tools initiative. The goal: integrate observability into the MCP standard itself. Right now, that link redirects to a GitHub discussion where I've proposed using the OpenTelemetry (OTel) standard to add tracing to MCP interactions. This would give developers clear visibility into tool usage, regardless of their observability platform. I need your help! Please check out the proposal, join the discussion, and show your support with a 👍 or 🚀 on GitHub. We need the community voice to make this happen! (And yes, my viral tweet showed there's huge demand for usable MCP clients too – more on that soon!). Vision & Video: Entering the Uncanny Valley This space is moving at lightning speed. Runway Gen-4 was announced, pushing for better consistency in AI video. Here's a few example videos showing incredible character and world consistency: ByteDance's impressive OmniHuman (single image to talking avatar) is now publicly usable via Dreamina website. For people it's really good, but for animated style images, Hedra Labs feels actually better (and much much faster) Meta's MoCHA is mind-blowing. We had researcher Cong Wei explain how it generates movie-grade, full-body, expressive talking characters directly from speech and text (no reference image needed!). Using Diffusion Transformers and clever attention mechanisms, the realism is startling, handling lip-sync, gestures, emotions, and even multi-character dialogue. Check the project page videos – some are truly uncanny. Just look at this one! Voice Highlight: Hailuo Speech-02 While Gladia launched their Solaria STT, the standout for me was Hailuo's Speech-02 TTS API. The emotional control and voice cloning quality are, in my opinion, potentially SOTA right now, offering incredibly nuanced and realistic synthetic voices. Tool Update & Breaking News! * Google's NotebookLM now discovers related sources automatically. * BREAKING NEWS (Caught end of show): Devin 2.0 is out! Cognition Labs launched their AI software engineer V2 with a new IDE experience and, crucially, a $20/month starting price. Much more accessible to try! Phew! What a week. From OpenAI's big moves to Gemini's math prowess, stunning AI actors from Meta, and the push for an observable agent ecosystem – the field is accelerating like crazy. Alright folks, that’s a wrap for show #99! Thank you again for tuning in, for being part of the community, and for keeping us on our toes with your insights and feedback. Special thanks to our guests Zach Nussbaum (Nomic), Xingyao Wang (All Hands AI), and Cong Wei (Meta/MoCHA) for joining us! If you missed any part of the show, or want to grab any of the links, head over to ThursdAI.news. The full recording (video on YouTube, audio on Spotify, Apple Podcasts, etc.) and this blog post with all the notes will be up shortly. The best way to support the show? Share it with a friend or colleague who needs to stay up-to-date on AI, and drop us a 5-star review on your podcast platform! Financial support via Substack is also appreciated but never required. Get ready for Episode 100 next week! Until then, happy tinkering, stay curious, and I'll see you next ThursdAI! Bye bye everyone! TL;DR and Show Notes Host, Guests, and Co-hosts * Host: Alex Volkov - AI Evangelist & Weights & Biases (@altryne) * Co-Hosts: * LDJ (@ldjconfirmed) * Yam Peleg (@yampeleg) * Guests: * Zach Nussbaum (@zach_nussbaum) - Nomic AI * Xingyao Wang (@xingyaow_) - All Hands AI / OpenHands * Cong Wei (@CongWei1230) - Meta AI / MoCha Key Topics & Links * OpenAI's Big Week: * Teasing highly capable Open Source Reasoner Model (seeking feedback). * Released PaperBench eval (code, paper) & Nano-Eval framework. * Raised $40B at $300B valuation. * New EMO "Monday" voice in ChatGPT. * Open Source Powerhouses: * Nomic Embed Multimodal: SOTA visual doc embeddings (3B & 7B, Apache 2.0 for 7B). * OpenHands LM 32B: SOTA-level coding agent model (Qwen finetune, MIT License, 37.2% SWE-Bench, #2 Live SWE-Bench). Cloud version available. * Frontier Models & Capabilities: * Dream 7B: Promising diffusion LM shows strong benchmark results (esp. Sudoku), but weights not yet released. * Gemini 2.5: Crushes hard USAMO math eval (24.4% vs <5% for others), showcasing superior reasoning. * Agents & Compute: * Amazon's Nova Act agent announced, claims SOTA but lacks public access (request form). * CoreWeave/NVIDIA: Massive inference speedups (800T/s on Llama 405B with GB200). * This Week's Buzz - MCP: * Observable Tools initiative launched to add observability to MCP. * Proposal using OpenTelemetry posted for community feedback on GitHub - please support! * Huge demand shown for usable MCP clients (viral tweet). * Vision & Video Highlights: * Runway Gen-4 focuses on video consistency. * ByteDance OmniHuman (image-to-avatar) now publicly available via Dreamina (example thread). * Meta's MoCHA: Generates stunningly realistic, movie-grade talking characters from speech+text. * Voice Highlight: * Hailuo Speech-02: Impressive TTS API with excellent emotional control and voice cloning. * Tool Updates: * Windsurf adds deployments to Netlify. * Google NotebookLM adds source discovery. * Breaking News: * Devin 2.0 AI Software Engineer announced, starts at $20/month. This is a public episode. If you'd like to discuss this with other subscribers or get access to bonus episodes, visit sub.thursdai.news/subscribe | |||
10 Apr 2025 | 💯 ThursdAI - 100th episode 🎉 - Meta LLama 4, Google tons of updates, ChatGPT memory, WandB MCP manifesto & more AI news | 01:32:18 | |
Hey Folks, Alex here, celebrating an absolutely crazy (to me) milestone, of #100 episodes of ThursdAI 👏 100 episodes in a year and a half (as I started publishing much later than I started going live, and the first episode was embarrassing), 100 episodes that documented INCREDIBLE AI progress, we mention on the show today, we used to be excited by context windows jumping from 4K to 16K! I want to extend a huge thank you to every one of you, who subscribes, listens to the show on podcasts, joins the live recording (we regularly get over 1K live viewers across platforms), shares with friends and highest thank you for the paid supporters! 🫶 Sharing the AI news progress with you, energizes me to keep going, despite the absolute avalanche of news every week. And what a perfect way to celebrate the 100th episode, on a week that Meta dropped Llama 4, sending the open-source world into a frenzy (and a bit of chaos). Google unleashed a firehose of announcements at Google Next. The agent ecosystem got a massive boost with MCP and A2A developments. And we had fantastic guests join us – Michael Lou diving deep into the impressive DeepCoder-14B, and Liad Yosef & Ido Salomon sharing their wild ride creating the viral GitMCP tool. I really loved today's show, and I encourage those of you who only read, to give this a watch/listen, and those of you who only listen, enjoy the recorded version (though longer and less edited!) Now let's dive in, there's a LOT to talk about (TL;DR and show notes as always, at the end of the newsletter) Open Source AI & LLMs: Llama 4 Takes Center Stage (Amidst Some Drama) Meta drops Llama 4 - Scout 109B/17BA & Maverick 400B/17BA (Blog, HF, Try It) This was by far the biggest news of this last week, and it dropped... on a Saturday? (I was on the mountain ⛷️! What are you doing Zuck) Meta dropped the long awaited LLama-4 models, huge ones this time * Llama 4 Scout: 17B active parameters out of ~109B total (16 experts). * Llama 4 Maverick: 17B active parameters out of a whopping ~400B total (128 experts). * Unreleased: Behemoth - 288B active with 2 Trillion total parameters chonker! * Both base and instruct finetuned models were released These new models are all Multimodal, Multilingual MoE (mixture of experts) architecture, and were trained with FP8, for significantly more tokens (around 30 Trillion Tokens!) with interleaved attention (iRoPE), and a refined SFT > RL > DPO post-training pipeline. The biggest highlight is the stated context windows, 10M for Scout and 1M for Maverick, which is insane (and honestly, I haven't yet seen a provider that is even remotely able to support anything of this length, nor do I have the tokens to verify it) The messy release - Big Oof from Big Zuck Not only did Meta release on a Saturday, messing up people's weekends, Meta apparently announced a high LM arena score, but the model they provided to LMArena was... not the model they released!? It caused LMArena to release the 2000 chats dataset, and truly, some examples are quite damning and show just how unreliable LMArena can be as vibe eval. Additionally, during the next days, folks noticed discrepancies between the stated eval scores Meta released, and the ability to evaluate them independently, including our own Wolfram, who noticed that a quantized version of Scout, performed better on his laptop while HIGHLY quantized (read: reduced precision) than it was performing on the Together API inference endpoint!? We've chatted on the show that this may be due to some VLLM issues, and speculated about other potential reasons for this. Worth noting the official response from Ahmad Al-Dahle, head of LLama at Meta, who mentioned stability issues between providers and absolutely denied any training on any benchmarks Too big for its own good (and us?) One of the main criticism the OSS community had about these releases, is that for many of us, the reason for celebrating Open Source AI, is the ability to run models without network, privately on our own devices. Llama 3 was released in 8-70B distilled versions and that was incredible for us local AI enthusiasts! These models, despite being "only" 17B active params, are huge and way to big to run on most local hardware, and so the question then is, if we're getting a model that HAS to run on a service, why not use Gemini 2.5 that's MUCH better and faster and cheaper than LLama? Why didn't Meta release those sizes? Was it due to an inability to beat Qwen/DeepSeek enough? 🤔 My Take Despite the absolutely chaotic rollout, this is still a monumental effort from Meta. They spent millions on compute and salaries to give this to the community. Yes, no papers yet, the LM Arena thing was weird, and the inference wasn't ready. But Meta is standing up for Western open-source in a big way. We have to celebrate the core contribution while demanding better rollout practices next time. As Wolfram rightly said, the real test will be the fine-tunes and distillations the community builds on these base models. Releasing the base weights is crucial for that. Let's see if the community can tame this beast once the inference dust settles. Shout out to Ahmed Al-Dahle and the whole Llama team at Meta – incredible work, messy launch, but thank you for pushing open source forward. 🎉 Together AI & Agentica (Berkley) finetuned DeepCoder-14B with reasoning (X, Blog) Amidst the Llama noise, we got another stellar open-source release! We were thrilled to have Michael Lou from Agentica/UC Berkeley join us to talk about DeepCoder-14B-Preview which beats DeepSeek R1 and even o3-mini on several coding benchmarks. Using distributed Reinforcement Learning (RL), it achieves 60.6% Pass@1 accuracy on LiveCodeBench, matching the performance of models like o3-mini-2025-01-31 (Low) despite its smaller size. The stated purpose of the project is to democratize RL and they have open sourced the model (HF), the dataset (HF), the Weights & Biases logs and even the eval logs! Shout out to Michael, Sijun and Alpay and the rest of the team who worked on this awesome model! NVIDIA Nemotron ULTRA is finally here, 253B pruned Llama 3-405B (HF) While Llama 4 was wrapped in mystery, NVIDIA dropped their pruned and distilled finetune of the previous Llama chonker 405B model, turning at just about half the parameters. And they were able to include the LLama-4 benchmarks in their release, showing that the older Llama, finetuned can absolutely beat the new ones at AIME, GPQA and more. As a reminder, we covered the previous 2 NEMOTRONS and they are a combined reasoning and non reasoning models, so the jump is not that surprising, and it does seem like a bit of eval cherry picking happened here. Nemotron Ultra supports 128K context and fits on a single 8xH100 node for inference. Built on open Llama models and trained on vetted + synthetic data, it's commercially viable. Shout out to NVIDIA for releasing this, and especially for pushing open reasoning datasets which Nisten rightly praised as having long-term value beyond the models themselves. More Open Source Goodness: Jina, DeepCogito, Kimi The open-source train didn't stop there: * Jina Reranker M0: Our friends at Jina released a state-of-the-art multimodal reranker model. If you're doing RAG with images and text, this looks super useful for improving retrieval quality across languages and modalities (Blog, HF) * DeepCogito: A new company emerged releasing a suite of Llama fine-tunes (3B up to 70B planned, with larger ones coming) trained using a technique they call Iterated Distillation and Amplification (IDA). They claim their 70B model beats DeepSeek V2 70B on some benchmarks . Definitely one to watch. (Blog, HF) * Kimi-VL & Kimi-VL-Thinking: MoonShot, who sometimes get lost in the noise, released incredibly impressive Kimi Vision Language Models (VLMs). These are MoE models with only ~3 Billion active parameters, yet they're showing results on par with or even beating models 10x larger (like Gemma 2 27B) on benchmarks like MathVision and ScreenSpot. They handle high-res images, support 128k context, and crucially, include a reasoning VLM variant. Plus, they're MIT licensed! Nisten's been following Kimi and thinks they're legit, just waiting for easier ways to run them locally. Definitely keep an eye on Kimi. (HF) This Week's Buzz from Weights & Biases - Observable Tools & A2A! This week was personally very exciting on the W&B front, as I spearheaded and launched initiatives directly related to the MCP and A2A news! W&B launches the observable.tools initiative! As MCP takes off, one challenge becomes clear: observability. When your agent calls an external MCP tool, that part of the execution chain becomes a black box. You lose the end-to-end visibility crucial for debugging and evaluation. That's why I'm thrilled that we launched Observable Tools (Website) – an initiative championing full-stack agent observability, specifically within the MCP ecosystem. Our vision is to enable developers using tools like W&B Weave to see inside those MCP tool calls, getting a complete trace of every step. The core of this is Proposal RFC 269 on the official MCP GitHub spec, which I authored! (My first RFC, quite the learning experience!). It details how to integrate OpenTelemetry tracing directly into the MCP protocol, allowing tools to securely report detailed execution spans back to the calling client (agent). We went deep on the spec, outlining transmission mechanisms, schemas, and rationale. My ask to you, the ThursdAI community: Please check out observable.tools, read the manifesto, watch the fun video we made, and most importantly, go to the RFC 269 proposal (shortcut: wandb.me/mcp-spec). Read it, comment, give feedback, and upvote if you agree! We need community support to make this impossible for the MCP maintainers to ignore. Let's make observability a first-class citizen in the MCP world! We also invite our friends from across the LLM observability landscape (LangSmith, Braintrust, Arize, Galileo, etc.) to join the discussion and collaborate. W&B is a Launch Partner for Google's A2A As mentioned earlier, we're also excited to be a launch partner for Google's new Agent2Agent (A2A) protocol. We believe standardized communication between agents is the next critical step, and we'll be supporting A2A alongside MCP in our tools. Exciting times for agent infrastructure! I've invited Google folks to next week to discuss the protocol in depth! ThursdAI - Recaps of the most high signal AI weekly spaces is a reader-supported publication. To receive new posts and support my work, consider becoming a free or paid subscriber. Big Company LLMs + APIs: Google's Onslaught & OpenAI's Memory Upgrade While open source had a wild week, the big players weren't sleeping. Google especially came out swinging at Google Next. Google announces TONS of new things at Next 🙌 (Blog) Google I/O felt like a preview, Google Next felt like the delivery truck backing up and dumping everything. Here's the whirlwind tour: * Gemini 2.5 Flash API: The faster, cheaper Gemini 2.5 model is coming soon to Vertex AI. (Still waiting on that general API access!). * Veo 2 Editing: Their top-tier video model (competing with Sora, Kling) gets editing capabilities. Very cool. * Imagen 3 Updates: Their image model gets improvements, including inpainting. * Lyria: Text-to-music model moves into preview. * TPU v7 (Ironwood): New TPU generation coming soon. As Nisten noted, Google's infrastructure uptime is consistently amazing, which could be a winning factor regardless of model SOTA status. * Chirp 3 HD Voices + Voice Cloning: This one raised eyebrows. The notes mentioned HD voices and voice cloning. Cloning is a touchy subject the big players usually avoid publicly (copyright, deepfakes). Still digging for confirmation/details on this – if Google is really offering public voice cloning, that's huge. Let me know if you find a link! * Deep Research gets Gemini 2.5 Pro: The experimental deep research feature in Gemini (their answer to OpenAI's research agent) now uses the powerful 2.5 Pro model. Google released comparison stats showing users strongly prefer it (70%) over OpenAI's offering, citing better instruction following and comprehensiveness. I haven't fully tested the 2.5 version yet, but the free tier access is amazing. and just look at those differences in preference compared to OAI Deep Research! Firebase Studio (firebase.studio): Remember Project IDX? It's been rebranded and launched as Firebase Studio. This is Google's answer to the wave of "vibe coding" web builders like Lovable, Bolt and a few more. It's a full-stack, cloud-based GenAI environment for building, testing, and deploying apps, integrated with Firebase and likely Gemini. Looks promising! Google Embraces MCP & Launches A2A Protocol! Two massive protocol announcements from Google that signal the maturation of the AI agent ecosystem: * Official MCP Support! (X)This is huge. Following Microsoft and AWS, Google (via both Sundar Pichai and Demis Hassabis) announced official support for Anthropic's Model Context Protocol (MCP) in Gemini models and SDKs. MCP is rapidly becoming the standard for how agents discover and use tools securely and efficiently. With Google onboard, there's basically universal major vendor support. MCP is here to stay. * Agent2Agent (A2A) Protocol (Blog , Spec, W&B Blog)Google also launched a new open standard, A2A, designed for interoperability between different AI agents. Think of agents built by different vendors (Salesforce, ServiceNow, etc.) needing to talk to each other securely to coordinate complex workflows across enterprise systems. Built on web standards (HTTP, SSE, JSON-RPC), it handles discovery, task management (long-running!), and modality negotiation. Importantly, Google positions A2A as complementary to MCP, not competitive. MCP is how an agent uses a tool, A2A is how an agent talks to another agent. Weights & Biases is proud to be one of the 50+ launch partners working with Google on this! We'll do a deeper dive soon, but this + MCP feels like the foundation for a truly interconnected agent future. Cloudflare - new Agents SDK (agents.cloudflare.com) Speaking of agents, Cloudflare launched their new Agents SDK (npm i agents). Built on their serverless infrastructure (Workers, Durable Objects), it offers a platform for building stateful, autonomous AI agents with a compelling pricing model (pay for CPU time, not wall time). This ties into the GitMCP story later – Cloudflare is betting big on the edge agent ecosystem. Other Big Co News: * Anthropic MAX: A new $200/month tier for Claude, offering higher usage quotas but no new models. Meh. * Grok 3 API: Elon's xAI finally launched the API tier for Grok 3 (plus Fast and Mini variants). Now you can test its capabilities yourself. We're still waiting for the promised Open Source Grok-2 🚨 BREAKING NEWS 🚨 OpenAI Upgrades Memory Right on cue during the show, OpenAI dropped a feature update! Sam Altman hyped something coming, and while it wasn't the o3/o4-mini models (those are coming next), it's a significant enhancement to ChatGPT Memory. Previously, Memory tried to selectively save key facts. Now, when enabled, it can reference ALL of your past chats to personalize responses. Preferences, interests, past projects – it can potentially draw on everything. OpenAI states there's no storage limit for what it can reference. How? Likely some sophisticated RAG/vector search under the hood, not stuffing everything into context. LDJ mentioned he might have had this rolling out silently for weeks, and while the immediate difference wasn't huge, the potential is massive as models get better at utilizing this vast personal context. The immediate reaction? Excitement mixed with a bit of caution. As Wolfram pointed out, do I really want it remembering every single chat? Configurable memory (flagging chats for inclusion/exclusion) seems like a necessary follow-up. Thanks for the feature request, Wolfram! (And yes, Europe might not get this right away anyway...). This could finally stop ChatGPT from asking me basic questions it should know from our history! Prompt suggestion: Ask the new chatGPT with memory, a think that you asked it that you likely forgot. Just don't asked it what was the most boring thing you asked it, I got cooked I'm still feeling raw 😂 Vision & Video: Kimi Drops Tiny But Mighty VLMs The most impressive long form AI video paper dropped, showing that it's possible to create 1 minute long video, with incredible character and scene consistency This paper introduces TTT layers (Test Time Training) to a pre-trained transformer, allowing it to one shot generate these incredibly consistent long scenes. Can't wait to see how the future of AI video evolves with this progress! AI Art & Diffusion & 3D: HiDream Takes the Open Crown HiDream-I1-Dev 17B MIT license new leading open weights image gen! (HF) Just when we thought the image gen space was settling, HiDream, a Chinese company, open-sourced their HiDream-I1 family under MIT license! This 17B parameter model comes in Dev, Full, and Fast variants. The exciting part? Based on early benchmarks (like Artificial Analysis Image Arena), HiDream-I1-Dev surpasses Flux 1.1 [Pro], Recraft V3, Reve and Imagen 3 while being open source! It boasts outstanding prompt following and text rendering capabilities. HiDream's API is coming soon too and I really hope it's finetunable! Tools: GitMCP - The Little Repo Tool That Could GitMCP - turn any github repo into an MCP server (website) We wrapped up the show with a fantastic story from the community. We had Liad Yosef (Shopify) and Ido Salomon (Palo Alto Networks) join us to talk about GitMCP. It started with a simple problem: a 3MB LLM.txt file (a format proposed by Jeremy Howard for repo documentation) too large for context windows. Liad and Ido, working nights and weekends, built an MCP server that could ingest any GitHub repo (prioritizing LLM.txt if present, falling back to Readmes/code comments) and expose its documentation via MCP tools (semantic search, fetching). This means any MCP-compatible client (like Cursor, potentially future ChatGPT/Gemini) can instantly query the documentation of any public GitHub repo just by pointing to the GitMCP URL for that repo (e.g., https://gitmcp.io/user/repo). As Yam Peleg pointed out during the show, the genius here is dynamically generating a customized tool specifically for that repo, making it incredibly easy for the LLM to use. Then, the story got crazy. They launched, went viral, almost melted their initial Vercel serverless setup due to traffic and SSE connection costs (100$+ per hour!). DMs flew back and forth with Vercel's CEO, then Cloudflare's CTO swooped in offering to sponsor hosting on Cloudflare's unreleased Agents platform if they migrated immediately. A frantic weekend of coding ensued, culminating in a nail-biting domain switch and a temporary outage before getting everything stable on Cloudflare. The project has received massive praise (including from Jeremy Howard himself) and is solving a real pain point for developers wanting to easily ground LLMs in project documentation. Huge congrats to Liad and Ido for the amazing work and the wild ride! Check out gitmcp.io! Wrapping Up Episode 100! Whew! What a show. From the Llama 4 rollercoaster to Google's AI barrage, the rise of agent standards like MCP and A2A, groundbreaking open source models, and incredible community stories like GitMCP – this episode truly showed an exemplary week in AI and underlined the reason I do this every week. It's really hard to keep up, and so if I commit to you guys, I stay up to date myself! Hitting 100 episodes feels surreal. It's been an absolute privilege sharing this journey with Wolfram, LDJ, Nisten, Yam, all our guests, and all of you. Seeing the community grow, hitting milestones like 1000 YouTube subscribers today, fuels us to keep going 🎉 The pace isn't slowing down. If anything, it's accelerating. But we'll be right here, every Thursday, trying to make sense of it all, together. If you missed anything, don't worry! Subscribe to the ThursdAI News Substack for the full TL;DR and links below. Thanks again for making 100 episodes possible. Here's to the next 100! 🥂 Keep tinkering, keep learning, and I'll see you next week. Alex TL;DR and Show Notes * Hosts and Guests * Alex Volkov - AI Evangelist & Weights & Biases (@altryne) * Co Hosts - @WolframRvnwlf @yampeleg @nisten @ldjconfirmed * Michael Luo @michaelzluo - CS PhD @ UC Berkeley; AI & Systems * Liad Yosef (@liadyosef), Ido Salomon (@idosal1) - GitMCP creators * Open Source LLMs * Meta drops LLama 4 (Scout 109B/17BA & Maverick 400B/17BA) - (Blog, HF, Try It) * Together AI and Agentica (UC Berkley) announce DeepCoder-14B (X, Blog) * NVIDIA Nemotron Ultra is here! 253B pruned LLama 3-405B (X, HF) * Jina Reranker M0 - SOTA multimodal reranker model (Blog, HF) * DeepCogito - SOTA models 3-70B - beating DeepSeek 70B - (Blog, HF) * ByteDance new release - Seed-Thinking-v1.5 * Big CO LLMs + APIs * Google announces TONS of new things 🙌 (Blog) * Google launches Firebase Studio (website) * Google is announcing official support for MCP (X) * Google announces A2A protocol - agent 2 agent communication (Blog, Spec, W&B Blog) * Cloudflare - new Agents SDK (Website) * Anthropic MAX - $200/mo with more quota * Grok 3 finally launches API tier (API) * OPenAI adds enhanced memory to ChatGPT - can remember all your chats (X) * This weeks Buzz - MCP and A2A * W&B launches the observable.tools initiative & invite people to comment on the MCP RFC * W&B is the launch partner for Google's A2A (Blog) * Vision & Video * Kimi-VL and Kimi-VL-Thinking - A3B vision models (X, HF) * One-Minute Video Generation with Test-Time Training (Blog, Paper) * Voice & Audio * Amazon - Nova Sonic - speech2speech foundational model (Blog) * AI Art & Diffusion & 3D * HiDream-I1-Dev 17B MIT license new leading open weights image gen 0 passes Flux1.1[pro] ! (HF) * Tools * GitMCP - turn any github repo into an MCP server (try it) ThursdAI - Recaps of the most high signal AI weekly spaces is a reader-supported publication. To receive new posts and support my work, consider becoming a free or paid subscriber. This is a public episode. If you'd like to discuss this with other subscribers or get access to bonus episodes, visit sub.thursdai.news/subscribe |