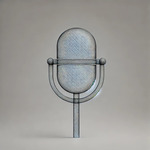
AI Safety Fundamentals: Alignment (BlueDot Impact)
Explorez tous les épisodes de AI Safety Fundamentals: Alignment
Date | Titre | Durée | |
---|---|---|---|
13 May 2023 | Cooperation, Conflict, and Transformative Artificial Intelligence: Sections 1 & 2 — Introduction, Strategy and Governance | 00:27:32 | |
Transformative artificial intelligence (TAI) may be a key factor in the long-run trajectory of civilization. A growing interdisciplinary community has begun to study how the development of TAI can be made safe and beneficial to sentient life (Bostrom 2014; Russell et al., 2015; OpenAI, 2018; Ortega and Maini, 2018; Dafoe, 2018). We present a research agenda for advancing a critical component of this effort: preventing catastrophic failures of cooperation among TAI systems. By cooperation failures we refer to a broad class of potentially-catastrophic inefficiencies in interactions among TAI-enabled actors. These include destructive conflict; coercion; and social dilemmas (Kollock, 1998; Macy and Flache, 2002) which destroy value over extended periods of time. We introduce cooperation failures at greater length in Section 1.1. Karnofsky (2016) defines TAI as ''AI that precipitates a transition comparable to (or more significant than) the agricultural or industrial revolution''. Such systems range from the unified, agent-like systems which are the focus of, e.g., Yudkowsky (2013) and Bostrom (2014), to the "comprehensive AI services’’ envisioned by Drexler (2019), in which humans are assisted by an array of powerful domain-specific AI tools. In our view, the potential consequences of such technology are enough to motivate research into mitigating risks today, despite considerable uncertainty about the timeline to TAI (Grace et al., 2018) and nature of TAI development. Original text: https://www.alignmentforum.org/s/p947tK8CoBbdpPtyK/p/KMocAf9jnAKc2jXri Narrated for AI Safety Fundamentals by Perrin Walker of TYPE III AUDIO. --- A podcast by BlueDot Impact. | |||
13 May 2023 | High-Stakes Alignment via Adversarial Training [Redwood Research Report] | 00:19:15 | |
(Update: We think the tone of this post was overly positive considering our somewhat weak results. You can read our latest post with more takeaways and followup results here.) This post motivates and summarizes this paper from Redwood Research, which presents results from the project first introduced here. We used adversarial training to improve high-stakes reliability in a task (“filter all injurious continuations of a story”) that we think is analogous to work that future AI safety engineers will need to do to reduce the risk of AI takeover. We experimented with three classes of adversaries – unaugmented humans, automatic paraphrasing, and humans augmented with a rewriting tool – and found that adversarial training was able to improve robustness to these three adversaries without affecting in-distribution performance. We think this work constitutes progress towards techniques that may substantially reduce the likelihood of deceptive alignment. Motivation Here are two dimensions along which you could simplify the alignment problem (similar to the decomposition at the top of this post): 1. Low-stakes (but difficult to oversee): Only consider domains where each decision that an AI makes is low-stakes, so no single action can have catastrophic consequences. In this setting, the key challenge is to correctly oversee the actions that AIs take, such that humans remain in control over time. 2. Easy oversight (but high-stakes): Only consider domains where overseeing AI behavior is easy, meaning that it is straightforward to run an oversight process that can assess the goodness of any particular action. Source: Narrated for AI Safety Fundamentals by Perrin Walker of TYPE III AUDIO. --- A podcast by BlueDot Impact. | |||
13 May 2023 | Yudkowsky Contra Christiano on AI Takeoff Speeds | 01:02:21 | |
In 2008, thousands of blog readers - including yours truly, who had discovered the rationality community just a few months before - watched Robin Hanson debate Eliezer Yudkowsky on the future of AI. Robin thought the AI revolution would be a gradual affair, like the Agricultural or Industrial Revolutions. Various people invent and improve various technologies over the course of decades or centuries. Each new technology provides another jumping-off point for people to use when inventing other technologies: mechanical gears → steam engine → railroad and so on. Over the course of a few decades, you’ve invented lots of stuff and the world is changed, but there’s no single moment when “industrialization happened”. Eliezer thought it would be lightning-fast. Once researchers started building human-like AIs, some combination of adding more compute, and the new capabilities provided by the AIs themselves, would quickly catapult AI to unimaginably superintelligent levels. The whole process could take between a few hours and a few years, depending on what point you measured from, but it wouldn’t take decades. You can imagine the graph above as being GDP over time, except that Eliezer thinks AI will probably destroy the world, which might be bad for GDP in some sense. If you come up with some way to measure (in dollars) whatever kind of crazy technologies AIs create for their own purposes after wiping out humanity, then the GDP framing will probably work fine. Crossposted from the Astral Codex Ten Podcast. --- A podcast by BlueDot Impact. | |||
13 May 2023 | Feature Visualization | 00:31:44 | |
There is a growing sense that neural networks need to be interpretable to humans. The field of neural network interpretability has formed in response to these concerns. As it matures, two major threads of research have begun to coalesce: feature visualization and attribution. This article focuses on feature visualization. While feature visualization is a powerful tool, actually getting it to work involves a number of details. In this article, we examine the major issues and explore common approaches to solving them. We find that remarkably simple methods can produce high-quality visualizations. Along the way we introduce a few tricks for exploring variation in what neurons react to, how they interact, and how to improve the optimization process. Original text: https://distill.pub/2017/feature-visualization/ Narrated for AI Safety Fundamentals by Perrin Walker of TYPE III AUDIO. --- A podcast by BlueDot Impact. | |||
13 May 2023 | Thought Experiments Provide a Third Anchor | 00:08:29 | |
Previously, I argued that we should expect future ML systems to often exhibit "emergent" behavior, where they acquire new capabilities that were not explicitly designed or intended, simply as a result of scaling. This was a special case of a general phenomenon in the physical sciences called More Is Different. I care about this because I think AI will have a huge impact on society, and I want to forecast what future systems will be like so that I can steer things to be better. To that end, I find More Is Different to be troubling and disorienting. I’m inclined to forecast the future by looking at existing trends and asking what will happen if they continue, but we should instead expect new qualitative behaviors to arise all the time that are not an extrapolation of previous trends. Given this, how can we predict what future systems will look like? For this, I find it helpful to think in terms of "anchors"---reference classes that are broadly analogous to future ML systems, which we can then use to make predictions. The most obvious reference class for future ML systems is current ML systems A podcast by BlueDot Impact. | |||
13 May 2023 | Debate Update: Obfuscated Arguments Problem | 00:28:30 | |
This is an update on the work on AI Safety via Debate that we previously wrote about here. What we did: We tested the debate protocol introduced in AI Safety via Debate with human judges and debaters. We found various problems and improved the mechanism to fix these issues (details of these are in the appendix). However, we discovered that a dishonest debater can often create arguments that have a fatal error, but where it is very hard to locate the error. We don’t have a fix for this “obfuscated argument” problem, and believe it might be an important quantitative limitation for both IDA and Debate. Key takeaways and relevance for alignment: Our ultimate goal is to find a mechanism that allows us to learn anything that a machine learning model knows: if the model can efficiently find the correct answer to some problem, our mechanism should favor the correct answer while only requiring a tractable number of human judgements and a reasonable number of computation steps for the model. We’re working under a hypothesis that there are broadly two ways to know things: via step-by-step reasoning about implications (logic, computation…), and by learning and generalizing from data (pattern matching, bayesian updating…). Original text: https://www.alignmentforum.org/posts/PJLABqQ962hZEqhdB/debate-update-obfuscated-arguments-problem Narrated for AI Safety Fundamentals by Perrin Walker of TYPE III AUDIO. --- A podcast by BlueDot Impact. | |||
13 May 2023 | Least-To-Most Prompting Enables Complex Reasoning in Large Language Models | 00:16:08 | |
Chain-of-thought prompting has demonstrated remarkable performance on various natural language reasoning tasks. However, it tends to perform poorly on tasks which requires solving problems harder than the exemplars shown in the prompts. To overcome this challenge of easy-to-hard generalization, we propose a novel prompting strategy, least-to-most prompting. The key idea in this strategy is to break down a complex problem into a series of simpler subproblems and then solve them in sequence. Solving each subproblem is facilitated by the answers to previously solved subproblems. Our experimental results on tasks related to symbolic manipulation, compositional generalization, and math reasoning reveal that least-to-most prompting is capable of generalizing to more difficult problems than those seen in the prompts. A notable finding is that when the GPT-3 code-davinci-002 model is used with least-to-most prompting, it can solve the compositional generalization benchmark SCAN in any split (including length split) with an accuracy of at least 99% using just 14 exemplars, compared to only 16% accuracy with chain-of-thought prompting. This is particularly noteworthy because neural-symbolic models in the literature that specialize in solving SCAN are trained on the entire training set containing over 15,000 examples. We have included prompts for all the tasks in the Appendix. Source: https://arxiv.org/abs/2205.10625 Narrated for AI Safety Fundamentals by Perrin Walker of TYPE III AUDIO. --- A podcast by BlueDot Impact. | |||
13 May 2023 | AI Safety via Debate | 00:39:49 | |
Abstract: To make AI systems broadly useful for challenging real-world tasks, we need them to learn complex human goals and preferences. One approach to specifying complex goals asks humans to judge during training which agent behaviors are safe and useful, but this approach can fail if the task is too complicated for a human to directly judge. To help address this concern, we propose training agents via self play on a zero sum debate game. Given a question or proposed action, two agents take turns making short statements up to a limit, then a human judges which of the agents gave the most true, useful information. In an analogy to complexity theory, debate with optimal play can answer any question in PSPACE given polynomial time judges (direct judging answers only NP questions). In practice, whether debate works involves empirical questions about humans and the tasks we want AIs to perform, plus theoretical questions about the meaning of AI alignment. We report results on an initial MNIST experiment where agents compete to convince a sparse classifier, boosting the classifier's accuracy from 59.4% to 88.9% given 6 pixels and from 48.2% to 85.2% given 4 pixels. Finally, we discuss theoretical and practical aspects of the debate model, focusing on potential weaknesses as the model scales up, and we propose future human and computer experiments to test these properties. Original text: https://arxiv.org/abs/1805.00899 Narrated for AI Safety Fundamentals by Perrin Walker of TYPE III AUDIO. --- A podcast by BlueDot Impact. | |||
13 May 2023 | Robust Feature-Level Adversaries Are Interpretability Tools | 00:35:33 | |
Abstract: The literature on adversarial attacks in computer vision typically focuses on pixel-level perturbations. These tend to be very difficult to interpret. Recent work that manipulates the latent representations of image generators to create "feature-level" adversarial perturbations gives us an opportunity to explore perceptible, interpretable adversarial attacks. We make three contributions. First, we observe that feature-level attacks provide useful classes of inputs for studying representations in models. Second, we show that these adversaries are uniquely versatile and highly robust. We demonstrate that they can be used to produce targeted, universal, disguised, physically-realizable, and black-box attacks at the ImageNet scale. Third, we show how these adversarial images can be used as a practical interpretability tool for identifying bugs in networks. We use these adversaries to make predictions about spurious associations between features and classes which we then test by designing "copy/paste" attacks in which one natural image is pasted into another to cause a targeted misclassification. Our results suggest that feature-level attacks are a promising approach for rigorous interpretability research. Original text: https://arxiv.org/abs/2110.03605 Narrated for AI Safety Fundamentals by Perrin Walker of TYPE III AUDIO. --- A podcast by BlueDot Impact. | |||
13 May 2023 | Embedded Agents | 00:17:39 | |
Suppose you want to build a robot to achieve some real-world goal for you—a goal that requires the robot to learn for itself and figure out a lot of things that you don’t already know. There’s a complicated engineering problem here. But there’s also a problem of figuring out what it even means to build a learning agent like that. What is it to optimize realistic goals in physical environments? In broad terms, how does it work? In this series of posts, I’ll point to four ways we don’t currently know how it works, and four areas of active research aimed at figuring it out. This is Alexei, and Alexei is playing a video game. Like most games, this game has clear input and output channels. Alexei only observes the game through the computer screen, and only manipulates the game through the controller. The game can be thought of as a function which takes in a sequence of button presses and outputs a sequence of pixels on the screen. Alexei is also very smart, and capable of holding the entire video game inside his mind. Original text: https://intelligence.org/2018/10/29/embedded-agents/ Narrated for AI Safety Fundamentals by Perrin Walker of TYPE III AUDIO. --- A podcast by BlueDot Impact. | |||
13 May 2023 | Progress on Causal Influence Diagrams | 00:23:03 | |
By Tom Everitt, Ryan Carey, Lewis Hammond, James Fox, Eric Langlois, and Shane Legg About 2 years ago, we released the first few papers on understanding agent incentives using causal influence diagrams. This blog post will summarize progress made since then. What are causal influence diagrams? A key problem in AI alignment is understanding agent incentives. Concerns have been raised that agents may be incentivized to avoid correction, manipulate users, or inappropriately influence their learning. This is particularly worrying as training schemes often shape incentives in subtle and surprising ways. For these reasons, we’re developing a formal theory of incentives based on causal influence diagrams (CIDs). Source: https://deepmindsafetyresearch.medium.com/progress-on-causal-influence-diagrams-a7a32180b0d1 Narrated for AI Safety Fundamentals by TYPE III AUDIO. --- A podcast by BlueDot Impact. | |||
13 May 2023 | The Alignment Problem From a Deep Learning Perspective | 00:33:47 | |
Within the coming decades, artificial general intelligence (AGI) may surpass human capabilities at a wide range of important tasks. We outline a case for expecting that, without substantial effort to prevent it, AGIs could learn to pursue goals which are undesirable (i.e. misaligned) from a human perspective. We argue that if AGIs are trained in ways similar to today's most capable models, they could learn to act deceptively to receive higher reward, learn internally-represented goals which generalize beyond their training distributions, and pursue those goals using power-seeking strategies. We outline how the deployment of misaligned AGIs might irreversibly undermine human control over the world, and briefly review research directions aimed at preventing this outcome. A podcast by BlueDot Impact. | |||
13 May 2023 | Learning From Human Preferences | 00:06:33 | |
One step towards building safe AI systems is to remove the need for humans to write goal functions, since using a simple proxy for a complex goal, or getting the complex goal a bit wrong, can lead to undesirable and even dangerous behavior. In collaboration with DeepMind’s safety team, we’ve developed an algorithm which can infer what humans want by being told which of two proposed behaviors is better. Original article: A podcast by BlueDot Impact. | |||
13 May 2023 | Machine Learning for Humans: Supervised Learning | 00:22:05 | |
The two tasks of supervised learning: regression and classification. Linear regression, loss functions, and gradient descent. How much money will we make by spending more dollars on digital advertising? Will this loan applicant pay back the loan or not? What’s going to happen to the stock market tomorrow? A podcast by BlueDot Impact. | |||
13 May 2023 | Intelligence Explosion: Evidence and Import | 00:18:59 | |
It seems unlikely that humans are near the ceiling of possible intelligences, rather than simply being the first such intelligence that happened to evolve. Computers far outperform humans in many narrow niches (e.g. arithmetic, chess, memory size), and there is reason to believe that similar large improvements over human performance are possible for general reasoning, technology design, and other tasks of interest. As occasional AI critic Jack Schwartz (1987) wrote: "If artificial intelligences can be created at all, there is little reason to believe that initial successes could not lead swiftly to the construction of artificial superintelligences able to explore significant mathematical, scientific, or engi-neering alternatives at a rate far exceeding human ability, or to generate plans and take action on them with equally overwhelming speed. Since man’s near-monopoly of all higher forms of intelligence has been one of the most basic facts of human existence throughout the past history of this planet, such developments would clearly create a new economics, a new sociology, and a new history." Why might AI “lead swiftly” to machine superintelligence? Below we consider some reasons. A podcast by BlueDot Impact. | |||
13 May 2023 | On the Opportunities and Risks of Foundation Models | 00:15:46 | |
AI is undergoing a paradigm shift with the rise of models (e.g., BERT, DALL-E, GPT-3) that are trained on broad data at scale and are adaptable to a wide range of downstream tasks. We call these models foundation models to underscore their critically central yet incomplete character. This report provides a thorough account of the opportunities and risks of foundation models, ranging from their capabilities (e.g., language, vision, robotics, reasoning, human interaction) and technical principles(e.g., model architectures, training procedures, data, systems, security, evaluation, theory) to their applications (e.g., law, healthcare, education) and societal impact (e.g., inequity, misuse, economic and environmental impact, legal and ethical considerations). Though foundation models are based on standard deep learning and transfer learning, their scale results in new emergent capabilities,and their effectiveness across so many tasks incentivizes homogenization. Homogenization provides powerful leverage but demands caution, as the defects of the foundation model are inherited by all the adapted models downstream. Despite the impending widespread deployment of foundation models, we currently lack a clear understanding of how they work, when they fail, and what they are even capable of due to their emergent properties. To tackle these questions, we believe much of the critical research on foundation models will require deep interdisciplinary collaboration commensurate with their fundamentally sociotechnical nature. A podcast by BlueDot Impact. | |||
13 May 2023 | Specification Gaming: The Flip Side of AI Ingenuity | 00:13:13 | |
Specification gaming is a behaviour that satisfies the literal specification of an objective without achieving the intended outcome. We have all had experiences with specification gaming, even if not by this name. Readers may have heard the myth of King Midas and the golden touch, in which the king asks that anything he touches be turned to gold - but soon finds that even food and drink turn to metal in his hands. In the real world, when rewarded for doing well on a homework assignment, a student might copy another student to get the right answers, rather than learning the material - and thus exploit a loophole in the task specification. A podcast by BlueDot Impact. | |||
13 May 2023 | Superintelligence: Instrumental Convergence | 00:17:55 | |
According to the orthogonality thesis, intelligent agents may have an enormous range of possible final goals. Nevertheless, according to what we may term the “instrumental convergence” thesis, there are some instrumental goals likely to be pursued by almost any intelligent agent, because there are some objectives that are useful intermediaries to the achievement of almost any final goal. We can formulate this thesis as follows: A podcast by BlueDot Impact. | |||
13 May 2023 | The Easy Goal Inference Problem Is Still Hard | 00:07:36 | |
One approach to the AI control problem goes like this:
This approach has the major advantage that we can begin empirical work today — we can actually build systems which observe user behavior, try to figure out what the user wants, and then help with that. There are many applications that people care about already, and we can set to work on making rich toy models. It seems great to develop these capabilities in parallel with other AI progress, and to address whatever difficulties actually arise, as they arise. That is, in each domain where AI can act effectively, we’d like to ensure that AI can also act effectively in the service of goals inferred from users (and that this inference is good enough to support foreseeable applications). This approach gives us a nice, concrete model of each difficulty we are trying to address. It also provides a relatively clear indicator of whether our ability to control AI lags behind our ability to build it. And by being technically interesting and economically meaningful now, it can help actually integrate AI control with AI practice. Overall I think that this is a particularly promising angle on the AI safety problem. A podcast by BlueDot Impact. | |||
13 May 2023 | Future ML Systems Will Be Qualitatively Different | 00:12:47 | |
In 1972, the Nobel prize-winning physicist Philip Anderson wrote the essay "More Is Different". In it, he argues that quantitative changes can lead to qualitatively different and unexpected phenomena. While he focused on physics, one can find many examples of More is Different in other domains as well, including biology, economics, and computer science. Some examples of More is Different include: Uranium. With a bit of uranium, nothing special happens; with a large amount of uranium packed densely enough, you get a nuclear reaction. DNA. Given only small molecules such as calcium, you can’t meaningfully encode useful information; given larger molecules such as DNA, you can encode a genome. Water. Individual water molecules aren’t wet. Wetness only occurs due to the interaction forces between many water molecules interspersed throughout a fabric (or other material). Original text: https://bounded-regret.ghost.io/future-ml-systems-will-be-qualitatively-different/ Narrated for AI Safety Fundamentals by Perrin Walker of TYPE III AUDIO. --- A podcast by BlueDot Impact. | |||
13 May 2023 | Four Background Claims | 00:15:28 | |
MIRI’s mission is to ensure that the creation of smarter-than-human artificial intelligence has a positive impact. Why is this mission important, and why do we think that there’s work we can do today to help ensure any such thing? In this post and my next one, I’ll try to answer those questions. This post will lay out what I see as the four most important premises underlying our mission. Related posts include Eliezer Yudkowsky’s “Five Theses” and Luke Muehlhauser’s “Why MIRI?”; this is my attempt to make explicit the claims that are in the background whenever I assert that our mission is of critical importance. #### Claim #1: Humans have a very general ability to solve problems and achieve goals across diverse domains. We call this ability “intelligence,” or “general intelligence.” This isn’t a formal definition — if we knew exactly what general intelligence was, we’d be better able to program it into a computer — but we do think that there’s a real phenomenon of general intelligence that we cannot yet replicate in code. Alternative view: There is no such thing as general intelligence. Instead, humans have a collection of disparate special-purpose modules. Computers will keep getting better at narrowly defined tasks such as chess or driving, but at no point will they acquire “generality” and become significantly more useful, because there is no generality to acquire. Source: https://intelligence.org/2015/07/24/four-background-claims/ Narrated for AI Safety Fundamentals by Perrin Walker of TYPE III AUDIO. --- A podcast by BlueDot Impact. | |||
13 May 2023 | Acquisition of Chess Knowledge in Alphazero | 00:22:21 | |
Abstract: What is learned by sophisticated neural network agents such as AlphaZero? This question is of both scientific and practical interest. If the representations of strong neural networks bear no resemblance to human concepts, our ability to understand faithful explanations of their decisions will be restricted, ultimately limiting what we can achieve with neural network interpretability. In this work we provide evidence that human knowledge is acquired by the AlphaZero neural network as it trains on the game of chess. By probing for a broad range of human chess concepts we show when and where these concepts are represented in the AlphaZero network. We also provide a behavioural analysis focusing on opening play, including qualitative analysis from chess Grandmaster Vladimir Kramnik. Finally, we carry out a preliminary investigation looking at the low-level details of AlphaZero's representations, and make the resulting behavioural and representational analyses available online. Original text: https://arxiv.org/abs/2111.09259 Narrated for AI Safety Fundamentals by TYPE III AUDIO. --- A podcast by BlueDot Impact. | |||
13 May 2023 | Discovering Latent Knowledge in Language Models Without Supervision | 00:37:09 | |
Abstract: Existing techniques for training language models can be misaligned with the truth: if we train models with imitation learning, they may reproduce errors that humans make; if we train them to generate text that humans rate highly, they may output errors that human evaluators can't detect. We propose circumventing this issue by directly finding latent knowledge inside the internal activations of a language model in a purely unsupervised way. Specifically, we introduce a method for accurately answering yes-no questions given only unlabeled model activations. It works by finding a direction in activation space that satisfies logical consistency properties, such as that a statement and its negation have opposite truth values. We show that despite using no supervision and no model outputs, our method can recover diverse knowledge represented in large language models: across 6 models and 10 question-answering datasets, it outperforms zero-shot accuracy by 4\\% on average. We also find that it cuts prompt sensitivity in half and continues to maintain high accuracy even when models are prompted to generate incorrect answers. Our results provide an initial step toward discovering what language models know, distinct from what they say, even when we don't have access to explicit ground truth labels. Original text: https://arxiv.org/abs/2212.03827 Narrated for AI Safety Fundamentals by Perrin Walker of TYPE III AUDIO. --- A podcast by BlueDot Impact. | |||
13 May 2023 | Visualizing the Deep Learning Revolution | 00:41:31 | |
The field of AI has undergone a revolution over the last decade, driven by the success of deep learning techniques. This post aims to convey three ideas using a series of illustrative examples:
I’ll focus on four domains: vision, games, language-based tasks, and science. The first two have more limited real-world applications, but provide particularly graphic and intuitive examples of the pace of progress. Original article: Author: A podcast by BlueDot Impact. | |||
13 May 2023 | Measuring Progress on Scalable Oversight for Large Language Models | 00:09:32 | |
Abstract: Developing safe and useful general-purpose AI systems will require us to make progress on scalable oversight: the problem of supervising systems that potentially outperform us on most skills relevant to the task at hand. Empirical work on this problem is not straightforward, since we do not yet have systems that broadly exceed our abilities. This paper discusses one of the major ways we think about this problem, with a focus on ways it can be studied empirically. We first present an experimental design centered on tasks for which human specialists succeed but unaided humans and current general AI systems fail. We then present a proof-of-concept experiment meant to demonstrate a key feature of this experimental design and show its viability with two question-answering tasks: MMLU and time-limited QuALITY. On these tasks, we find that human participants who interact with an unreliable large-language-model dialog assistant through chat -- a trivial baseline strategy for scalable oversight -- substantially outperform both the model alone and their own unaided performance. These results are an encouraging sign that scalable oversight will be tractable to study with present models and bolster recent findings that large language models can productively assist humans with difficult tasks. Authors: Samuel R. Bowman, Jeeyoon Hyun, Ethan Perez, Edwin Chen, Craig Pettit, Scott Heiner, Kamilė Lukošiūtė, Amanda Askell, Andy Jones, Anna Chen, Anna Goldie, Azalia Mirhoseini, Cameron McKinnon, Christopher Olah, Daniela Amodei, Dario Amodei, Dawn Drain, Dustin Li, Eli Tran-Johnson, Jackson Kernion, Jamie Kerr, Jared Mueller, Jeffrey Ladish, Joshua Landau, Kamal Ndousse, Liane Lovitt, Nelson Elhage, Nicholas Schiefer, Nicholas Joseph, Noemí Mercado, Nova DasSarma, Robin Larson, Sam McCandlish, Sandipan Kundu, Scott Johnston, Shauna Kravec, Sheer El Showk, Stanislav Fort, Timothy Telleen-Lawton, Tom Brown, Tom Henighan, Tristan Hume, Yuntao Bai, Zac Hatfield-Dodds, Ben Mann, Jared Kaplan Original text: https://arxiv.org/abs/2211.03540 Narrated for AI Safety Fundamentals by Perrin Walker of TYPE III AUDIO. --- A podcast by BlueDot Impact. | |||
13 May 2023 | Toy Models of Superposition | 00:41:43 | |
It would be very convenient if the individual neurons of artificial neural networks corresponded to cleanly interpretable features of the input. For example, in an “ideal” ImageNet classifier, each neuron would fire only in the presence of a specific visual feature, such as the color red, a left-facing curve, or a dog snout. Empirically, in models we have studied, some of the neurons do cleanly map to features. But it isn't always the case that features correspond so cleanly to neurons, especially in large language models where it actually seems rare for neurons to correspond to clean features. This brings up many questions. Why is it that neurons sometimes align with features and sometimes don't? Why do some models and tasks have many of these clean neurons, while they're vanishingly rare in others? In this paper, we use toy models — small ReLU networks trained on synthetic data with sparse input features — to investigate how and when models represent more features than they have dimensions. We call this phenomenon superposition . When features are sparse, superposition allows compression beyond what a linear model would do, at the cost of "interference" that requires nonlinear filtering. Narrated for AI Safety Fundamentals by Perrin Walker of TYPE III AUDIO. --- A podcast by BlueDot Impact. | |||
13 May 2023 | A Short Introduction to Machine Learning | 00:17:47 | |
Despite the current popularity of machine learning, I haven’t found any short introductions to it which quite match the way I prefer to introduce people to the field. So here’s my own. Compared with other introductions, I’ve focused less on explaining each concept in detail, and more on explaining how they relate to other important concepts in AI, especially in diagram form. If you're new to machine learning, you shouldn't expect to fully understand most of the concepts explained here just after reading this post - the goal is instead to provide a broad framework which will contextualise more detailed explanations you'll receive from elsewhere. I'm aware that high-level taxonomies can be controversial, and also that it's easy to fall into the illusion of transparency when trying to introduce a field; so suggestions for improvements are very welcome! The key ideas are contained in this summary diagram: First, some quick clarifications: None of the boxes are meant to be comprehensive; we could add more items to any of them. So you should picture each list ending with “and others”. The distinction between tasks and techniques is not a firm or standard categorisation; it’s just the best way I’ve found so far to lay things out. The summary is explicitly from an AI-centric perspective. For example, statistical modeling and optimization are fields in their own right; but for our current purposes we can think of them as machine learning techniques. Original text: https://www.alignmentforum.org/posts/qE73pqxAZmeACsAdF/a-short-introduction-to-machine-learning Narrated for AI Safety Fundamentals by Perrin Walker of TYPE III AUDIO. --- A podcast by BlueDot Impact. | |||
13 May 2023 | Takeaways From Our Robust Injury Classifier Project [Redwood Research] | 00:12:01 | |
With the benefit of hindsight, we have a better sense of our takeaways from our first adversarial training project (paper). Our original aim was to use adversarial training to make a system that (as far as we could tell) never produced injurious completions. If we had accomplished that, we think it would have been the first demonstration of a deep learning system avoiding a difficult-to-formalize catastrophe with an ultra-high level of reliability. Presumably, we would have needed to invent novel robustness techniques that could have informed techniques useful for aligning TAI. With a successful system, we also could have performed ablations to get a clear sense of which building blocks were most important. Alas, we fell well short of that target. We still saw failures when just randomly sampling prompts and completions. Our adversarial training didn’t reduce the random failure rate, nor did it eliminate highly egregious failures (example below). We also don’t think we've successfully demonstrated a negative result, given that our results could be explained by suboptimal choices in our training process. Overall, we’d say this project had value as a learning experience but produced much less alignment progress than we hoped. Source: Narrated for AI Safety Fundamentals by TYPE III AUDIO. --- A podcast by BlueDot Impact. | |||
13 May 2023 | What Failure Looks Like | 00:18:23 | |
Crossposted from the AI Alignment Forum. May contain more technical jargon than usual. The stereotyped image of AI catastrophe is a powerful, malicious AI system that takes its creators by surprise and quickly achieves a decisive advantage over the rest of humanity. I think this is probably not what failure will look like, and I want to try to paint a more realistic picture. I’ll tell the story in two parts: Part I: machine learning will increase our ability to “get what we can measure,” which could cause a slow-rolling catastrophe. ("Going out with a whimper.") Part II: ML training, like competitive economies or natural ecosystems, can give rise to “greedy” patterns that try to expand their own influence. Such patterns can ultimately dominate the behavior of a system and cause sudden breakdowns. ("Going out with a bang," an instance of optimization daemons.) I think these are the most important problems if we fail to solve intent alignment. In practice these problems will interact with each other, and with other disruptions/instability caused by rapid progress. These problems are worse in worlds where progress is relatively fast, and fast takeoff can be a key risk factor, but I’m scared even if we have several years. Crossposted from the LessWrong Curated Podcast by TYPE III AUDIO. --- A podcast by BlueDot Impact. | |||
13 May 2023 | Goal Misgeneralisation: Why Correct Specifications Aren’t Enough for Correct Goals | 00:17:17 | |
As we build increasingly advanced AI systems, we want to make sure they don’t pursue undesired goals. This is the primary concern of the AI alignment community. Undesired behaviour in an AI agent is often the result of specification gaming —when the AI exploits an incorrectly specified reward. However, if we take on the perspective of the agent we’re training, we see other reasons it might pursue undesired goals, even when trained with a correct specification. Imagine that you are the agent (the blue blob) being trained with reinforcement learning (RL) in the following 3D environment: The environment also contains another blob like yourself, but coloured red instead of blue, that also moves around. The environment also appears to have some tower obstacles, some coloured spheres, and a square on the right that sometimes flashes. You don’t know what all of this means, but you can figure it out during training! You start exploring the environment to see how everything works and to see what you do and don’t get rewarded for. For more details, check out our paper. By Rohin Shah, Vikrant Varma, Ramana Kumar, Mary Phuong, Victoria Krakovna, Jonathan Uesato, and Zac Kenton. Original text: Narrated for AI Safety Fundamentals by Perrin Walker of TYPE III AUDIO. --- A podcast by BlueDot Impact. | |||
13 May 2023 | Introduction to Logical Decision Theory for Computer Scientists | 00:14:28 | |
Decision theories differ on exactly how to calculate the expectation--the probability of an outcome, conditional on an action. This foundational difference bubbles up to real-life questions about whether to vote in elections, or accept a lowball offer at the negotiating table. When you're thinking about what happens if you don't vote in an election, should you calculate the expected outcome as if only your vote changes, or as if all the people sufficiently similar to you would also decide not to vote? Questions like these belong to a larger class of problems, Newcomblike decision problems, in which some other agent is similar to us or reasoning about what we will do in the future. The central principle of 'logical decision theories', several families of which will be introduced, is that we ought to choose as if we are controlling the logical output of our abstract decision algorithm. Newcomblike considerations--which might initially seem like unusual special cases--become more prominent as agents can get higher-quality information about what algorithms or policies other agents use: Public commitments, machine agents with known code, smart contracts running on Ethereum. Newcomblike considerations also become more important as we deal with agents that are very similar to one another; or with large groups of agents that are likely to contain high-similarity subgroups; or with problems where even small correlations are enough to swing the decision. In philosophy, the debate over decision theories is seen as a debate over the principle of rational choice. Do 'rational' agents refrain from voting in elections, because their one vote is very unlikely to change anything? Do we need to go beyond 'rationality', into 'social rationality' or 'superrationality' or something along those lines, in order to describe agents that could possibly make up a functional society? Original text: https://arbital.com/p/logical_dt/?l=5d6 Narrated for AI Safety Fundamentals by Perrin Walker of TYPE III AUDIO. --- A podcast by BlueDot Impact. | |||
26 Mar 2024 | Can We Scale Human Feedback for Complex AI Tasks? | 00:20:06 | |
Reinforcement learning from human feedback (RLHF) has emerged as a powerful technique for steering large language models (LLMs) toward desired behaviours. However, relying on simple human feedback doesn’t work for tasks that are too complex for humans to accurately judge at the scale needed to train AI models. Scalable oversight techniques attempt to address this by increasing the abilities of humans to give feedback on complex tasks. This article briefly recaps some of the challenges faced with human feedback, and introduces the approaches to scalable oversight covered in session 4 of our AI Alignment course. A podcast by BlueDot Impact. | |||
26 Mar 2024 | Weak-To-Strong Generalization: Eliciting Strong Capabilities With Weak Supervision | 00:35:05 | |
Widely used alignment techniques, such as reinforcement learning from human feedback (RLHF), rely on the ability of humans to supervise model behavior—for example, to evaluate whether a model faithfully followed instructions or generated safe outputs. However, future superhuman models will behave in complex ways too difficult for humans to reliably evaluate; humans will only be able to weakly supervise superhuman models. We study an analogy to this problem: can weak model supervision elicit the full capabilities of a much stronger model? We test this using a range of pretrained language models in the GPT-4 family on natural language processing (NLP), chess, and reward modeling tasks. We find that when we naively fine-tune strong pretrained models on labels generated by a weak model, they consistently perform better than their weak supervisors, a phenomenon we call weak-to-strong generalization. However, we are still far from recovering the full capabilities of strong models with naive fine-tuning alone, suggesting that techniques like RLHF may scale poorly to superhuman models without further work. We find that simple methods can often significantly improve weak-to-strong generalization: for example, when fine-tuning GPT-4 with a GPT-2-level supervisor and an auxiliary confidence loss, we can recover close to GPT-3.5-level performance on NLP tasks. Our results suggest that it is feasible to make empirical progress today on a fundamental challenge of aligning superhuman models. Source: A podcast by BlueDot Impact. | |||
31 Mar 2024 | Zoom In: An Introduction to Circuits | 00:44:03 | |
By studying the connections between neurons, we can find meaningful algorithms in the weights of neural networks. For example, microscopes let us see cells, leading to cellular biology. Science zoomed in. Several techniques including x-ray crystallography let us see DNA, leading to the molecular revolution. Science zoomed in. Atomic theory. Subatomic particles. Neuroscience. Science zoomed in. These transitions weren’t just a change in precision: they were qualitative changes in what the objects of scientific inquiry are. For example, cellular biology isn’t just more careful zoology. It’s a new kind of inquiry that dramatically shifts what we can understand. The famous examples of this phenomenon happened at a very large scale, but it can also be the more modest shift of a small research community realizing they can now study their topic in a finer grained level of detail. A podcast by BlueDot Impact. | |||
31 Mar 2024 | Towards Monosemanticity: Decomposing Language Models With Dictionary Learning | 00:08:53 | |
Using a sparse autoencoder, we extract a large number of interpretable features from a one-layer transformer. A podcast by BlueDot Impact. | |||
01 Apr 2024 | Interpretability in the Wild: A Circuit for Indirect Object Identification in GPT-2 Small | 00:24:48 | |
Research in mechanistic interpretability seeks to explain behaviors of machine learning (ML) models in terms of their internal components. However, most previous work either focuses on simple behaviors in small models or describes complicated behaviors in larger models with broad strokes. In this work, we bridge this gap by presenting an explanation for how GPT-2 small performs a natural language task called indirect object identification (IOI). Our explanation encompasses 26 attention heads grouped into 7 main classes, which we discovered using a combination of interpretability approaches relying on causal interventions. To our knowledge, this investigation is the largest end-to-end attempt at reverse-engineering a natural behavior "in the wild" in a language model. We evaluate the reliability of our explanation using three quantitative criteria–faithfulness, completeness, and minimality. Though these criteria support our explanation, they also point to remaining gaps in our understanding. Our work provides evidence that a mechanistic understanding of large ML models is feasible, pointing toward opportunities to scale our understanding to both larger models and more complex tasks. Code for all experiments is available at https://github.com/redwoodresearch/Easy-Transformer. A podcast by BlueDot Impact. | |||
07 Apr 2024 | AI Watermarking Won’t Curb Disinformation | 00:08:05 | |
Generative AI allows people to produce piles upon piles of images and words very quickly. It would be nice if there were some way to reliably distinguish AI-generated content from human-generated content. It would help people avoid endlessly arguing with bots online, or believing what a fake image purports to show. One common proposal is that big companies should incorporate watermarks into the outputs of their AIs. For instance, this could involve taking an image and subtly changing many pixels in a way that’s undetectable to the eye but detectable to a computer program. Or it could involve swapping words for synonyms in a predictable way so that the meaning is unchanged, but a program could readily determine the text was generated by an AI. Unfortunately, watermarking schemes are unlikely to work. So far most have proven easy to remove, and it’s likely that future schemes will have similar problems. A podcast by BlueDot Impact. | |||
07 Apr 2024 | Emerging Processes for Frontier AI Safety | 00:18:20 | |
The UK recognises the enormous opportunities that AI can unlock across our economy and our society. However, without appropriate guardrails, such technologies can pose significant risks. The AI Safety Summit will focus on how best to manage the risks from frontier AI such as misuse, loss of control and societal harms. Frontier AI organisations play an important role in addressing these risks and promoting the safety of the development and deployment of frontier AI. The UK has therefore encouraged frontier AI organisations to publish details on their frontier AI safety policies ahead of the AI Safety Summit hosted by the UK on 1 to 2 November 2023. This will provide transparency regarding how they are putting into practice voluntary AI safety commitments and enable the sharing of safety practices within the AI ecosystem. Transparency of AI systems can increase public trust, which can be a significant driver of AI adoption. This document complements these publications by providing a potential list of frontier AI organisations’ safety policies. A podcast by BlueDot Impact. | |||
07 Apr 2024 | Challenges in Evaluating AI Systems | 00:22:33 | |
Most conversations around the societal impacts of artificial intelligence (AI) come down to discussing some quality of an AI system, such as its truthfulness, fairness, potential for misuse, and so on. We are able to talk about these characteristics because we can technically evaluate models for their performance in these areas. But what many people working inside and outside of AI don’t fully appreciate is how difficult it is to build robust and reliable model evaluations. Many of today’s existing evaluation suites are limited in their ability to serve as accurate indicators of model capabilities or safety. Here, we outline challenges that we have encountered while evaluating our own models to give readers a sense of what developing, implementing, and interpreting model evaluations looks like in practice. A podcast by BlueDot Impact. | |||
07 Apr 2024 | AI Control: Improving Safety Despite Intentional Subversion | 00:20:51 | |
We’ve released a paper, AI Control: Improving Safety Despite Intentional Subversion. This paper explores techniques that prevent AI catastrophes even if AI instances are colluding to subvert the safety techniques. In this post:
Source: A podcast by BlueDot Impact. | |||
07 Apr 2024 | Computing Power and the Governance of AI | 00:26:49 | |
This post summarises a new report, “Computing Power and the Governance of Artificial Intelligence.” The full report is a collaboration between nineteen researchers from academia, civil society, and industry. It can be read here. GovAI research blog posts represent the views of their authors, rather than the views of the organisation. A podcast by BlueDot Impact. | |||
14 Apr 2024 | Working in AI Alignment | 01:08:44 | |
This guide is written for people who are considering direct work on technical AI alignment. I expect it to be most useful for people who are not yet working on alignment, and for people who are already familiar with the arguments for working on AI alignment. If you aren’t familiar with the arguments for the importance of AI alignment, you can get an overview of them by doing the AI Alignment Course. A podcast by BlueDot Impact. | |||
16 Apr 2024 | Planning a High-Impact Career: A Summary of Everything You Need to Know in 7 Points | 00:11:02 | |
We took 10 years of research and what we’ve learned from advising 1,000+ people on how to build high-impact careers, compressed that into an eight-week course to create your career plan, and then compressed that into this three-page summary of the main points. (It’s especially aimed at people who want a career that’s both satisfying and has a significant positive impact, but much of the advice applies to all career decisions.) A podcast by BlueDot Impact. | |||
17 Apr 2024 | Become a Person who Actually Does Things | 00:05:14 | |
The next four weeks of the course are an opportunity for you to actually build a thing that moves you closer to contributing to AI Alignment, and we're really excited to see what you do! A common failure mode is to think "Oh, I can't actually do X" or to say "Someone else is probably doing Y." You probably can do X, and it's unlikely anyone is doing Y! It could be you! A podcast by BlueDot Impact. | |||
23 Apr 2024 | How to Succeed as an Early-Stage Researcher: The “Lean Startup” Approach | 00:15:16 | |
I am approaching the end of my AI governance PhD, and I’ve spent about 2.5 years as a researcher at FHI. During that time, I’ve learnt a lot about the formula for successful early-career research. This post summarises my advice for people in the first couple of years. Research is really hard, and I want people to avoid the mistakes I’ve made. A podcast by BlueDot Impact. | |||
04 May 2024 | Being the (Pareto) Best in the World | 00:06:46 | |
This introduces the concept of Pareto frontiers. The top comment by Rob Miles also ties it to comparative advantage. While reading, consider what Pareto frontiers your project could place you on. A podcast by BlueDot Impact. | |||
12 May 2024 | Writing, Briefly | 00:03:09 | |
(In the process of answering an email, I accidentally wrote a tiny essay about writing. I usually spend weeks on an essay. This one took 67 minutes—23 of writing, and 44 of rewriting.) A podcast by BlueDot Impact. | |||
12 May 2024 | Public by Default: How We Manage Information Visibility at Get on Board | 00:09:50 | |
I’ve been obsessed with managing information, and communications in a remote team since Get on Board started growing. Reducing the bus factor is a primary motivation — but another just as important is diminishing reliance on synchronicity. When what I know is documented and accessible to others, I’m less likely to be a bottleneck for anyone else in the team. So if I’m busy, minding family matters, on vacation, or sick, I won’t be blocking anyone. This, in turn, gives everyone in the team the freedom to build their own work schedules according to their needs, work from any time zone, or enjoy more distraction-free moments. As I write these lines, most of the world is under quarantine, relying on non-stop video calls to continue working. Needless to say, that is not a sustainable long-term work schedule. A podcast by BlueDot Impact. | |||
12 May 2024 | How to Get Feedback | 00:07:30 | |
Feedback is essential for learning. Whether you’re studying for a test, trying to improve in your work or want to master a difficult skill, you need feedback. The challenge is that feedback can often be hard to get. Worse, if you get bad feedback, you may end up worse than before. A podcast by BlueDot Impact. | |||
29 May 2024 | Worst-Case Thinking in AI Alignment | 00:11:35 | |
Alternative title: “When should you assume that what could go wrong, will go wrong?” Thanks to Mary Phuong and Ryan Greenblatt for helpful suggestions and discussion, and Akash Wasil for some edits. In discussions of AI safety, people often propose the assumption that something goes as badly as possible. Eliezer Yudkowsky in particular has argued for the importance of security mindset when thinking about AI alignment. I think there are several distinct reasons that this might be the right assumption to make in a particular situation. But I think people often conflate these reasons, and I think that this causes confusion and mistaken thinking. So I want to spell out some distinctions. Throughout this post, I give a bunch of specific arguments about AI alignment, including one argument that I think I was personally getting wrong until I noticed my mistake yesterday (which was my impetus for thinking about this topic more and then writing this post). I think I’m probably still thinking about some of my object level examples wrong, and hope that if so, commenters will point out my mistakes. Original text: https://www.lesswrong.com/posts/yTvBSFrXhZfL8vr5a/worst-case-thinking-in-ai-alignment Narrated for AI Safety Fundamentals by Perrin Walker of TYPE III AUDIO. --- A podcast by BlueDot Impact. | |||
13 Jun 2024 | Compute Trends Across Three Eras of Machine Learning | 00:13:50 | |
This article explains key drivers of AI progress, explains how compute is calculated, as well as looks at how the amount of compute used to train AI models has increased significantly in recent years. Original text: https://epochai.org/blog/compute-trends A podcast by BlueDot Impact. | |||
17 Jun 2024 | Empirical Findings Generalize Surprisingly Far | 00:11:32 | |
Previously, I argued that emergent phenomena in machine learning mean that we can’t rely on current trends to predict what the future of ML will be like. In this post, I will argue that despite this, empirical findings often do generalize very far, including across “phase transitions” caused by emergent behavior. This might seem like a contradiction, but actually I think divergence from current trends and empirical generalization are consistent. Findings do often generalize, but you need to think to determine the right generalization, and also about what might stop any given generalization from holding. I don’t think many people would contest the claim that empirical investigation can uncover deep and generalizable truths. This is one of the big lessons of physics, and while some might attribute physics’ success to math instead of empiricism, I think it’s clear that you need empirical data to point to the right mathematics. However, just invoking physics isn’t a good argument, because physical laws have fundamental symmetries that we shouldn’t expect in machine learning. Moreover, we care specifically about findings that continue to hold up after some sort of emergent behavior (such as few-shot learning in the case of ML). So, to make my case, I’ll start by considering examples in deep learning that have held up in this way. Since “modern” deep learning hasn’t been around that long, I’ll also look at examples from biology, a field that has been around for a relatively long time and where More Is Different is ubiquitous (see Appendix: More Is Different In Other Domains). Source: https://bounded-regret.ghost.io/empirical-findings-generalize-surprisingly-far/ Narrated for AI Safety Fundamentals by Perrin Walker of TYPE III AUDIO. --- A podcast by BlueDot Impact. | |||
17 Jun 2024 | Low-Stakes Alignment | 00:13:56 | |
Right now I’m working on finding a good objective to optimize with ML, rather than trying to make sure our models are robustly optimizing that objective. (This is roughly “outer alignment.”) That’s pretty vague, and it’s not obvious whether “find a good objective” is a meaningful goal rather than being inherently confused or sweeping key distinctions under the rug. So I like to focus on a more precise special case of alignment: solve alignment when decisions are “low stakes.” I think this case effectively isolates the problem of “find a good objective” from the problem of ensuring robustness and is precise enough to focus on productively. In this post I’ll describe what I mean by the low-stakes setting, why I think it isolates this subproblem, why I want to isolate this subproblem, and why I think that it’s valuable to work on crisp subproblems. Source: https://www.alignmentforum.org/posts/TPan9sQFuPP6jgEJo/low-stakes-alignment Narrated for AI Safety Fundamentals by TYPE III AUDIO. --- A podcast by BlueDot Impact. | |||
17 Jun 2024 | Two-Turn Debate Doesn’t Help Humans Answer Hard Reading Comprehension Questions | 00:16:39 | |
Using hard multiple-choice reading comprehension questions as a testbed, we assess whether presenting humans with arguments for two competing answer options, where one is correct and the other is incorrect, allows human judges to perform more accurately, even when one of the arguments is unreliable and deceptive. If this is helpful, we may be able to increase our justified trust in language-model-based systems by asking them to produce these arguments where needed. Previous research has shown that just a single turn of arguments in this format is not helpful to humans. However, as debate settings are characterized by a back-and-forth dialogue, we follow up on previous results to test whether adding a second round of counter-arguments is helpful to humans. We find that, regardless of whether they have access to arguments or not, humans perform similarly on our task. These findings suggest that, in the case of answering reading comprehension questions, debate is not a helpful format. Source: https://arxiv.org/abs/2210.10860 Narrated for AI Safety Fundamentals by Perrin Walker of TYPE III AUDIO. --- A podcast by BlueDot Impact. | |||
17 Jun 2024 | Least-To-Most Prompting Enables Complex Reasoning in Large Language Models | 00:16:08 | |
Chain-of-thought prompting has demonstrated remarkable performance on various natural language reasoning tasks. However, it tends to perform poorly on tasks which requires solving problems harder than the exemplars shown in the prompts. To overcome this challenge of easy-to-hard generalization, we propose a novel prompting strategy, least-to-most prompting. The key idea in this strategy is to break down a complex problem into a series of simpler subproblems and then solve them in sequence. Solving each subproblem is facilitated by the answers to previously solved subproblems. Our experimental results on tasks related to symbolic manipulation, compositional generalization, and math reasoning reveal that least-to-most prompting is capable of generalizing to more difficult problems than those seen in the prompts. A notable finding is that when the GPT-3 code-davinci-002 model is used with least-to-most prompting, it can solve the compositional generalization benchmark SCAN in any split (including length split) with an accuracy of at least 99% using just 14 exemplars, compared to only 16% accuracy with chain-of-thought prompting. This is particularly noteworthy because neural-symbolic models in the literature that specialize in solving SCAN are trained on the entire training set containing over 15,000 examples. We have included prompts for all the tasks in the Appendix. Source: https://arxiv.org/abs/2205.10625 Narrated for AI Safety Fundamentals by Perrin Walker of TYPE III AUDIO. --- A podcast by BlueDot Impact. | |||
17 Jun 2024 | ABS: Scanning Neural Networks for Back-Doors by Artificial Brain Stimulation | 00:16:08 | |
This paper presents a technique to scan neural network based AI models to determine if they are trojaned. Pre-trained AI models may contain back-doors that are injected through training or by transforming inner neuron weights. These trojaned models operate normally when regular inputs are provided, and mis-classify to a specific output label when the input is stamped with some special pattern called trojan trigger. We develop a novel technique that analyzes inner neuron behaviors by determining how output acti- vations change when we introduce different levels of stimulation to a neuron. The neurons that substantially elevate the activation of a particular output label regardless of the provided input is considered potentially compromised. Trojan trigger is then reverse-engineered through an optimization procedure using the stimulation analysis results, to confirm that a neuron is truly compromised. We evaluate our system ABS on 177 trojaned models that are trojaned with vari-ous attack methods that target both the input space and the feature space, and have various trojan trigger sizes and shapes, together with 144 benign models that are trained with different data and initial weight values. These models belong to 7 different model structures and 6 different datasets, including some complex ones such as ImageNet, VGG-Face and ResNet110. Our results show that ABS is highly effective, can achieve over 90% detection rate for most cases (and many 100%), when only one input sample is provided for each output label. It substantially out-performs the state-of-the-art technique Neural Cleanse that requires a lot of input samples and small trojan triggers to achieve good performance. Source: https://www.cs.purdue.edu/homes/taog/docs/CCS19.pdf Narrated for AI Safety Fundamentals the Effective Altruism Forum Joseph Carlsmith LessWrong 80,000 Hours by Perrin Walker of TYPE III AUDIO. --- A podcast by BlueDot Impact. | |||
17 Jun 2024 | Imitative Generalisation (AKA ‘Learning the Prior’) | 00:18:14 | |
This post tries to explain a simplified version of Paul Christiano’s mechanism introduced here, (referred to there as ‘Learning the Prior’) and explain why a mechanism like this potentially addresses some of the safety problems with naïve approaches. First we’ll go through a simple example in a familiar domain, then explain the problems with the example. Then I’ll discuss the open questions for making Imitative Generalization actually work, and the connection with the Microscope AI idea. A more detailed explanation of exactly what the training objective is (with diagrams), and the correspondence with Bayesian inference, are in the appendix. Source: Narrated for AI Safety Fundamentals by Perrin Walker of TYPE III AUDIO. --- A podcast by BlueDot Impact. | |||
17 Jun 2024 | Toy Models of Superposition | 00:41:43 | |
It would be very convenient if the individual neurons of artificial neural networks corresponded to cleanly interpretable features of the input. For example, in an “ideal” ImageNet classifier, each neuron would fire only in the presence of a specific visual feature, such as the color red, a left-facing curve, or a dog snout. Empirically, in models we have studied, some of the neurons do cleanly map to features. But it isn't always the case that features correspond so cleanly to neurons, especially in large language models where it actually seems rare for neurons to correspond to clean features. This brings up many questions. Why is it that neurons sometimes align with features and sometimes don't? Why do some models and tasks have many of these clean neurons, while they're vanishingly rare in others? In this paper, we use toy models — small ReLU networks trained on synthetic data with sparse input features — to investigate how and when models represent more features than they have dimensions. We call this phenomenon superposition . When features are sparse, superposition allows compression beyond what a linear model would do, at the cost of "interference" that requires nonlinear filtering. Narrated for AI Safety Fundamentals by Perrin Walker of TYPE III AUDIO. --- A podcast by BlueDot Impact. | |||
17 Jun 2024 | Discovering Latent Knowledge in Language Models Without Supervision | 00:37:09 | |
Abstract: Existing techniques for training language models can be misaligned with the truth: if we train models with imitation learning, they may reproduce errors that humans make; if we train them to generate text that humans rate highly, they may output errors that human evaluators can't detect. We propose circumventing this issue by directly finding latent knowledge inside the internal activations of a language model in a purely unsupervised way. Specifically, we introduce a method for accurately answering yes-no questions given only unlabeled model activations. It works by finding a direction in activation space that satisfies logical consistency properties, such as that a statement and its negation have opposite truth values. We show that despite using no supervision and no model outputs, our method can recover diverse knowledge represented in large language models: across 6 models and 10 question-answering datasets, it outperforms zero-shot accuracy by 4\\% on average. We also find that it cuts prompt sensitivity in half and continues to maintain high accuracy even when models are prompted to generate incorrect answers. Our results provide an initial step toward discovering what language models know, distinct from what they say, even when we don't have access to explicit ground truth labels. Original text: https://arxiv.org/abs/2212.03827 Narrated for AI Safety Fundamentals by Perrin Walker of TYPE III AUDIO. --- A podcast by BlueDot Impact. | |||
17 Jun 2024 | An Investigation of Model-Free Planning | 00:08:11 | |
The field of reinforcement learning (RL) is facing increasingly challenging domains with combinatorial complexity. For an RL agent to address these challenges, it is essential that it can plan effectively. Prior work has typically utilized an explicit model of the environment, combined with a specific planning algorithm (such as tree search). More recently, a new family of methods have been proposed that learn how to plan, by providing the structure for planning via an inductive bias in the function approximator (such as a tree structured neural network), trained end-to-end by a model-free RL algorithm. In this paper, we go even further, and demonstrate empirically that an entirely model-free approach, without special structure beyond standard neural network components such as convolutional networks and LSTMs, can learn to exhibit many of the characteristics typically associated with a model-based planner. We measure our agent’s effectiveness at planning in terms of its ability to generalize across a combinatorial and irreversible state space, its data efficiency, and its ability to utilize additional thinking time. We find that our agent has many of the characteristics that one might expect to find in a planning algorithm. Furthermore, it exceeds the state-of-the-art in challenging combinatorial domains such as Sokoban and outperforms other model-free approaches that utilize strong inductive biases toward planning. Source: https://arxiv.org/abs/1901.03559 Narrated for AI Safety Fundamentals by Perrin Walker of TYPE III AUDIO. --- A podcast by BlueDot Impact. | |||
17 Jun 2024 | Gradient Hacking: Definitions and Examples | 00:09:15 | |
Gradient hacking is a hypothesized phenomenon where:
Below I give some potential examples of gradient hacking, divided into those which exploit RL credit assignment and those which exploit gradient descent itself. My concern is that models might use techniques like these either to influence which goals they develop, or to fool our interpretability techniques. Even if those effects don’t last in the long term, they might last until the model is smart enough to misbehave in other ways (e.g. specification gaming, or reward tampering), or until it’s deployed in the real world—especially in the RL examples, since convergence to a global optimum seems unrealistic (and ill-defined) for RL policies trained on real-world data. However, since gradient hacking isn’t very well-understood right now, both the definition above and the examples below should only be considered preliminary. Source: https://www.alignmentforum.org/posts/EeAgytDZbDjRznPMA/gradient-hacking-definitions-and-examples Narrated for AI Safety Fundamentals by Perrin Walker of TYPE III AUDIO. --- A podcast by BlueDot Impact. | |||
17 Jun 2024 | Intro to Brain-Like-AGI Safety | 01:02:10 | |
(Sections 3.1-3.4, 6.1-6.2, and 7.1-7.5) Suppose we someday build an Artificial General Intelligence algorithm using similar principles of learning and cognition as the human brain. How would we use such an algorithm safely? I will argue that this is an open technical problem, and my goal in this post series is to bring readers with no prior knowledge all the way up to the front-line of unsolved problems as I see them. If this whole thing seems weird or stupid, you should start right in on Post #1, which contains definitions, background, and motivation. Then Posts #2–#7 are mainly neuroscience, and Posts #8–#15 are more directly about AGI safety, ending with a list of open questions and advice for getting involved in the field. Source: https://www.lesswrong.com/s/HzcM2dkCq7fwXBej8 Narrated for AI Safety Fundamentals by Perrin Walker of TYPE III AUDIO. --- A podcast by BlueDot Impact. | |||
17 Jun 2024 | Chinchilla’s Wild Implications | 00:24:57 | |
This post is about language model scaling laws, specifically the laws derived in the DeepMind paper that introduced Chinchilla. The paper came out a few months ago, and has been discussed a lot, but some of its implications deserve more explicit notice in my opinion. In particular: Data, not size, is the currently active constraint on language modeling performance. Current returns to additional data are immense, and current returns to additional model size are miniscule; indeed, most recent landmark models are wastefully big. If we can leverage enough data, there is no reason to train ~500B param models, much less 1T or larger models. If we have to train models at these large sizes, it will mean we have encountered a barrier to exploitation of data scaling, which would be a great loss relative to what would otherwise be possible. The literature is extremely unclear on how much text data is actually available for training. We may be "running out" of general-domain data, but the literature is too vague to know one way or the other. The entire available quantity of data in highly specialized domains like code is woefully tiny, compared to the gains that would be possible if much more such data were available. Some things to note at the outset: This post assumes you have some familiarity with LM scaling laws. As in the paper, I'll assume here that models never see repeated data in training. Original text: https://www.alignmentforum.org/posts/6Fpvch8RR29qLEWNH/chinchilla-s-wild-implications Narrated for AI Safety Fundamentals by Perrin Walker of TYPE III AUDIO. --- A podcast by BlueDot Impact. | |||
17 Jun 2024 | Deep Double Descent | 00:08:27 | |
We show that the double descent phenomenon occurs in CNNs, ResNets, and transformers: performance first improves, then gets worse, and then improves again with increasing model size, data size, or training time. This effect is often avoided through careful regularization. While this behavior appears to be fairly universal, we don’t yet fully understand why it happens, and view further study of this phenomenon as an important research direction. Source: https://openai.com/research/deep-double-descent Narrated for AI Safety Fundamentals by Perrin Walker of TYPE III AUDIO. --- A podcast by BlueDot Impact. | |||
17 Jun 2024 | Eliciting Latent Knowledge | 01:00:27 | |
In this post, we’ll present ARC’s approach to an open problem we think is central to aligning powerful machine learning (ML) systems: Suppose we train a model to predict what the future will look like according to cameras and other sensors. We then use planning algorithms to find a sequence of actions that lead to predicted futures that look good to us. But some action sequences could tamper with the cameras so they show happy humans regardless of what’s really happening. More generally, some futures look great on camera but are actually catastrophically bad. In these cases, the prediction model “knows” facts (like “the camera was tampered with”) that are not visible on camera but would change our evaluation of the predicted future if we learned them. How can we train this model to report its latent knowledge of off-screen events? We’ll call this problem eliciting latent knowledge (ELK). In this report we’ll focus on detecting sensor tampering as a motivating example, but we believe ELK is central to many aspects of alignment. Source: https://docs.google.com/document/d/1WwsnJQstPq91_Yh-Ch2XRL8H_EpsnjrC1dwZXR37PC8/edit# Narrated for AI Safety Fundamentals by Perrin Walker of TYPE III AUDIO. --- A podcast by BlueDot Impact. | |||
19 Jul 2024 | Illustrating Reinforcement Learning from Human Feedback (RLHF) | 00:22:32 | |
This more technical article explains the motivations for a system like RLHF, and adds additional concrete details as to how the RLHF approach is applied to neural networks. While reading, consider which parts of the technical implementation correspond to the 'values coach' and 'coherence coach' from the previous video. A podcast by BlueDot Impact. | |||
19 Jul 2024 | Problems and Fundamental Limitations of Reinforcement Learning from Human Feedback | 00:32:19 | |
This paper explains Anthropic’s constitutional AI approach, which is largely an extension on RLHF but with AIs replacing human demonstrators and human evaluators. Everything in this paper is relevant to this week's learning objectives, and we recommend you read it in its entirety. It summarises limitations with conventional RLHF, explains the constitutional AI approach, shows how it performs, and where future research might be directed. If you are in a rush, focus on sections 1.2, 3.1, 3.4, 4.1, 6.1, 6.2. A podcast by BlueDot Impact. | |||
19 Jul 2024 | Constitutional AI Harmlessness from AI Feedback | 01:01:49 | |
This paper explains Anthropic’s constitutional AI approach, which is largely an extension on RLHF but with AIs replacing human demonstrators and human evaluators. Everything in this paper is relevant to this week's learning objectives, and we recommend you read it in its entirety. It summarises limitations with conventional RLHF, explains the constitutional AI approach, shows how it performs, and where future research might be directed. If you are in a rush, focus on sections 1.2, 3.1, 3.4, 4.1, 6.1, 6.2. A podcast by BlueDot Impact. | |||
13 May 2023 | Is Power-Seeking AI an Existential Risk? | 03:21:02 | |
This report examines what I see as the core argument for concern about existential risk from misaligned artificial intelligence. I proceed in two stages. First, I lay out a backdrop picture that informs such concern. On this picture, intelligent agency is an extremely powerful force, and creating agents much more intelligent than us is playing with fire -- especially given that if their objectives are problematic, such agents would plausibly have instrumental incentives to seek power over humans. Second, I formulate and evaluate a more specific six-premise argument that creating agents of this kind will lead to existential catastrophe by 2070. On this argument, by 2070: (1) it will become possible and financially feasible to build relevantly powerful and agentic AI systems; (2) there will be strong incentives to do so; (3) it will be much harder to build aligned (and relevantly powerful/agentic) AI systems than to build misaligned (and relevantly powerful/agentic) AI systems that are still superficially attractive to deploy; (4) some such misaligned systems will seek power over humans in high-impact ways; (5) this problem will scale to the full disempowerment of humanity; and (6) such disempowerment will constitute an existential catastrophe. I assign rough subjective credences to the premises in this argument, and I end up with an overall estimate of ~5% that an existential catastrophe of this kind will occur by 2070. (May 2022 update: since making this report public in April 2021, my estimate here has gone up, and is now at >10%). Source: https://arxiv.org/abs/2206.13353 Narrated for Joe Carlsmith Audio by TYPE III AUDIO. --- A podcast by BlueDot Impact. | |||
13 May 2023 | Biological Anchors: A Trick That Might Or Might Not Work | 01:10:46 | |
I've been trying to review and summarize Eliezer Yudkowksy's recent dialogues on AI safety. Previously in sequence: Yudkowsky Contra Ngo On Agents. Now we’re up to Yudkowsky contra Cotra on biological anchors, but before we get there we need to figure out what Cotra's talking about and what's going on. The Open Philanthropy Project ("Open Phil") is a big effective altruist foundation interested in funding AI safety. It's got $20 billion, probably the majority of money in the field, so its decisions matter a lot and it’s very invested in getting things right. In 2020, it asked senior researcher Ajeya Cotra to produce a report on when human-level AI would arrive. It says the resulting document is "informal" - but it’s 169 pages long and likely to affect millions of dollars in funding, which some might describe as making it kind of formal. The report finds a 10% chance of “transformative AI” by 2031, a 50% chance by 2052, and an almost 80% chance by 2100. Eliezer rejects their methodology and expects AI earlier (he doesn’t offer many numbers, but here he gives Bryan Caplan 50-50 odds on 2030, albeit not totally seriously). He made the case in his own very long essay, Biology-Inspired AGI Timelines: The Trick That Never Works, sparking a bunch of arguments and counterarguments and even more long essays. Source: https://astralcodexten.substack.com/p/biological-anchors-a-trick-that-might Crossposted from the Astral Codex Ten podcast. --- A podcast by BlueDot Impact. | |||
13 May 2023 | Why AI Alignment Could Be Hard With Modern Deep Learning | 00:28:50 | |
Why would we program AI that wants to harm us? Because we might not know how to do otherwise. Source: https://www.cold-takes.com/why-ai-alignment-could-be-hard-with-modern-deep-learning/ Crossposted from the Cold Takes Audio podcast. --- A podcast by BlueDot Impact. | |||
13 May 2023 | AGI Ruin: A List of Lethalities | 01:01:34 | |
I have several times failed to write up a well-organized list of reasons why AGI will kill you. People come in with different ideas about why AGI would be survivable, and want to hear different obviously key points addressed first. Some fraction of those people are loudly upset with me if the obviously most important points aren't addressed immediately, and I address different points first instead. Having failed to solve this problem in any good way, I now give up and solve it poorly with a poorly organized list of individual rants. I'm not particularly happy with this list; the alternative was publishing nothing, and publishing this seems marginally more dignified. Crossposted from the LessWrong Curated Podcast by TYPE III AUDIO. --- A podcast by BlueDot Impact. | |||
13 May 2023 | Deceptively Aligned Mesa-Optimizers: It’s Not Funny if I Have to Explain It | 00:26:51 | |
Our goal here is to popularize obscure and hard-to-understand areas of AI alignment. So let’s try to understand the incomprehensible meme! Our main source will be Hubinger et al 2019, Risks From Learned Optimization In Advanced Machine Learning Systems. Mesa- is a Greek prefix which means the opposite of meta-. To “go meta” is to go one level up; to “go mesa” is to go one level down (nobody has ever actually used this expression, sorry). So a mesa-optimizer is an optimizer one level down from you. Consider evolution, optimizing the fitness of animals. For a long time, it did so very mechanically, inserting behaviors like “use this cell to detect light, then grow toward the light” or “if something has a red dot on its back, it might be a female of your species, you should mate with it”. As animals became more complicated, they started to do some of the work themselves. Evolution gave them drives, like hunger and lust, and the animals figured out ways to achieve those drives in their current situation. Evolution didn’t mechanically instill the behavior of opening my fridge and eating a Swiss Cheese slice. It instilled the hunger drive, and I figured out that the best way to satisfy it was to open my fridge and eat cheese. Source: https://astralcodexten.substack.com/p/deceptively-aligned-mesa-optimizers Crossposted from the Astral Codex Ten podcast. --- A podcast by BlueDot Impact. | |||
13 May 2023 | Where I Agree and Disagree with Eliezer | 00:42:46 | |
(Partially in response to AGI Ruin: A list of Lethalities. Written in the same rambling style. Not exhaustive.) Agreements Powerful AI systems have a good chance of deliberately and irreversibly disempowering humanity. This is a much easier failure mode than killing everyone with destructive physical technologies. Catastrophically risky AI systems could plausibly exist soon, and there likely won’t be a strong consensus about this fact until such systems pose a meaningful existential risk per year. There is not necessarily any “fire alarm.” Even if there were consensus about a risk from powerful AI systems, there is a good chance that the world would respond in a totally unproductive way. It’s wishful thinking to look at possible stories of doom and say “we wouldn’t let that happen;” humanity is fully capable of messing up even very basic challenges, especially if they are novel. Source: https://www.lesswrong.com/posts/CoZhXrhpQxpy9xw9y/where-i-agree-and-disagree-with-eliezer --- A podcast by BlueDot Impact. | |||
13 May 2023 | Careers in Alignment | 00:07:51 | |
Richard Ngo compiles a number of resources for thinking about careers in alignment research. Original text: Narrated for AI Safety Fundamentals by Perrin Walker of TYPE III AUDIO. --- A podcast by BlueDot Impact. | |||
13 May 2023 | More Is Different for AI | 00:06:34 | |
Machine learning is touching increasingly many aspects of our society, and its effect will only continue to grow. Given this, I and many others care about risks from future ML systems and how to mitigate them. When thinking about safety risks from ML, there are two common approaches, which I'll call the Engineering approach and the Philosophy approach: The Engineering approach tends to be empirically-driven, drawing experience from existing or past ML systems and looking at issues that either: (1) are already major problems, or (2) are minor problems, but can be expected to get worse in the future. Engineering tends to be bottom-up and tends to be both in touch with and anchored on current state-of-the-art systems. The Philosophy approach tends to think more about the limit of very advanced systems. It is willing to entertain thought experiments that would be implausible with current state-of-the-art systems (such as Nick Bostrom's paperclip maximizer) and is open to considering abstractions without knowing many details. It often sounds more "sci-fi like" and more like philosophy than like computer science. It draws some inspiration from current ML systems, but often only in broad strokes. I'll discuss these approaches mainly in the context of ML safety, but the same distinction applies in other areas. For instance, an Engineering approach to AI + Law might focus on how to regulate self-driving cars, while Philosophy might ask whether using AI in judicial decision-making could undermine liberal democracy. Original text: https://bounded-regret.ghost.io/more-is-different-for-ai/ Narrated for AI Safety Fundamentals by Perrin Walker of TYPE III AUDIO. --- A podcast by BlueDot Impact. | |||
13 May 2023 | Logical Induction (Blog Post) | 00:11:56 | |
MIRI is releasing a paper introducing a new model of deductively limited reasoning: “Logical induction,” authored by Scott Garrabrant, Tsvi Benson-Tilsen, Andrew Critch, myself, and Jessica Taylor. Readers may wish to start with the abridged version. Consider a setting where a reasoner is observing a deductive process (such as a community of mathematicians and computer programmers) and waiting for proofs of various logical claims (such as the abc conjecture, or “this computer program has a bug in it”), while making guesses about which claims will turn out to be true. Roughly speaking, our paper presents a computable (though inefficient) algorithm that outpaces deduction, assigning high subjective probabilities to provable conjectures and low probabilities to disprovable conjectures long before the proofs can be produced. This algorithm has a large number of nice theoretical properties. Still speaking roughly, the algorithm learns to assign probabilities to sentences in ways that respect any logical or statistical pattern that can be described in polynomial time. Additionally, it learns to reason well about its own beliefs and trust its future beliefs while avoiding paradox. Quoting from the abstract: "These properties and many others all follow from a single logical induction criterion, which is motivated by a series of stock trading analogies. Roughly speaking, each logical sentence φ is associated with a stock that is worth $1 per share if φ is true and nothing otherwise, and we interpret the belief-state of a logically uncertain reasoner as a set of market prices, where ℙn(φ)=50% means that on day n, shares of φ may be bought or sold from the reasoner for 50¢. The logical induction criterion says (very roughly) that there should not be any polynomial-time computable trading strategy with finite risk tolerance that earns unbounded profits in that market over time." Original text: https://intelligence.org/2016/09/12/new-paper-logical-induction/ Narrated for AI Safety Fundamentals by Perrin Walker of TYPE III AUDIO. --- A podcast by BlueDot Impact. | |||
13 May 2023 | AI Safety via Debatered Teaming Language Models With Language Models | 00:06:47 | |
Abstract: Language Models (LMs) often cannot be deployed because of their potential to harm users in ways that are hard to predict in advance. Prior work identifies harmful behaviors before deployment by using human annotators to hand-write test cases. However, human annotation is expensive, limiting the number and diversity of test cases. In this work, we automatically find cases where a target LM behaves in a harmful way, by generating test cases (“red teaming”) using another LM. We evaluate the target LM’s replies to generated test questions using a classifier trained to detect offensive content, uncovering tens of thousands of offensive replies in a 280B parameter LM chatbot. We explore several methods, from zero-shot generation to reinforcement learning, for generating test cases with varying levels of diversity and difficulty. Furthermore, we use prompt engineering to control LM-generated test cases to uncover a variety of other harms, automatically finding groups of people that the chatbot discusses in offensive ways, personal and hospital phone numbers generated as the chatbot’s own contact info, leakage of private training data in generated text, and harms that occur over the course of a conversation. Overall, LM-based red teaming is one promising tool (among many needed) for finding and fixing diverse, undesirable LM behaviors before impacting users. Original text: https://www.deepmind.com/publications/red-teaming-language-models-with-language-models Narrated for AI Safety Fundamentals by Perrin Walker of TYPE III AUDIO. --- A podcast by BlueDot Impact. | |||
13 May 2023 | Supervising Strong Learners by Amplifying Weak Experts | 00:19:10 | |
Abstract: Many real world learning tasks involve complex or hard-to-specify objectives, and using an easier-to-specify proxy can lead to poor performance or misaligned behavior. One solution is to have humans provide a training signal by demonstrating or judging performance, but this approach fails if the task is too complicated for a human to directly evaluate. We propose Iterated Amplification, an alternative training strategy which progressively builds up a training signal for difficult problems by combining solutions to easier subproblems. Iterated Amplification is closely related to Expert Iteration (Anthony et al., 2017; Silver et al., 2017), except that it uses no external reward function. We present results in algorithmic environments, showing that Iterated Amplification can efficiently learn complex behaviors. Original text: https://arxiv.org/abs/1810.08575 Narrated for AI Safety Fundamentals by Perrin Walker of TYPE III AUDIO. --- A podcast by BlueDot Impact. | |||
13 May 2023 | ML Systems Will Have Weird Failure Modes | 00:13:49 | |
Previously, I've argued that future ML systems might exhibit unfamiliar, emergent capabilities, and that thought experiments provide one approach towards predicting these capabilities and their consequences. In this post I’ll describe a particular thought experiment in detail. We’ll see that taking thought experiments seriously often surfaces future risks that seem "weird" and alien from the point of view of current systems. I’ll also describe how I tend to engage with these thought experiments: I usually start out intuitively skeptical, but when I reflect on emergent behavior I find that some (but not all) of the skepticism goes away. The remaining skepticism comes from ways that the thought experiment clashes with the ontology of neural networks, and I’ll describe the approaches I usually take to address this and generate actionable takeaways. ## Thought Experiment: Deceptive Alignment Recall that the optimization anchor runs the thought experiment of assuming that an ML agent is a perfect optimizer (with respect to some "intrinsic" reward function R). I’m going to examine one implication of this assumption, in the context of an agent being trained based on some "extrinsic" reward function R∗ (which is provided by the system designer and not equal to R). Specifically, consider a training process where in step t, a model has parameters θt and generates an action at (its output on that training step, e.g. an attempted backflip assuming it is being trained to do backflips). The action at is then judged according to the extrinsic reward function R∗, and the parameters are updated to some new value θt+1 that are intended to increase at+1's value under R∗. Original text: https://bounded-regret.ghost.io/ml-systems-will-have-weird-failure-modes-2/ Narrated for AI Safety Fundamentals by Perrin Walker of TYPE III AUDIO. --- A podcast by BlueDot Impact. | |||
13 May 2023 | Understanding Intermediate Layers Using Linear Classifier Probes | 00:16:34 | |
Abstract: Neural network models have a reputation for being black boxes. We propose to monitor the features at every layer of a model and measure how suitable they are for classification. We use linear classifiers, which we refer to as "probes", trained entirely independently of the model itself. This helps us better understand the roles and dynamics of the intermediate layers. We demonstrate how this can be used to develop a better intuition about models and to diagnose potential problems. We apply this technique to the popular models Inception v3 and Resnet-50. Among other things, we observe experimentally that the linear separability of features increase monotonically along the depth of the model. Original text: https://arxiv.org/pdf/1610.01644.pdf Narrated for AI Safety Fundamentals by Perrin Walker of TYPE III AUDIO. --- A podcast by BlueDot Impact. | |||
13 May 2023 | Summarizing Books With Human Feedback | 00:06:16 | |
To safely deploy powerful, general-purpose artificial intelligence in the future, we need to ensure that machine learning models act in accordance with human intentions. This challenge has become known as the alignment problem. A scalable solution to the alignment problem needs to work on tasks where model outputs are difficult or time-consuming for humans to evaluate. To test scalable alignment techniques, we trained a model to summarize entire books, as shown in the following samples. Source: https://openai.com/research/summarizing-books Narrated for AI Safety Fundamentals by Perrin Walker of TYPE III AUDIO. --- A podcast by BlueDot Impact. | |||
13 May 2023 | AGI Safety From First Principles | 00:13:17 | |
This report explores the core case for why the development of artificial general intelligence (AGI) might pose an existential threat to humanity. It stems from my dissatisfaction with existing arguments on this topic: early work is less relevant in the context of modern machine learning, while more recent work is scattered and brief. This report aims to fill that gap by providing a detailed investigation into the potential risk from AGI misbehaviour, grounded by our current knowledge of machine learning, and highlighting important uncertain ties. It identifies four key premises, evaluates existing arguments about them, and outlines some novel considerations for each. Source: https://drive.google.com/file/d/1uK7NhdSKprQKZnRjU58X7NLA1auXlWHt/view Narrated for AI Safety Fundamentals by TYPE III AUDIO. --- A podcast by BlueDot Impact. |