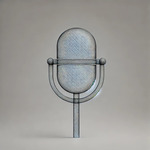
PaperPlayer biorxiv bioinformatics (PaperPlayer)
Explore every episode of PaperPlayer biorxiv bioinformatics
Dive into the complete episode list for PaperPlayer biorxiv bioinformatics. Each episode is cataloged with detailed descriptions, making it easy to find and explore specific topics. Keep track of all episodes from your favorite podcast and never miss a moment of insightful content.
Pub. Date | Title | Duration | |
---|---|---|---|
25 Oct 2022 | meTCRs - Learning a metric for T-cell receptors | ||
Link to bioRxiv paper:
http://biorxiv.org/cgi/content/short/2022.10.24.513533v1?rss=1
Authors: Drost, F. R., Schiefelbein, L., Schubert, B.
Abstract:
T cell receptors (TCRs) bind to pathogen- or self-derived epitopes to elicit a T cell response as part of the adaptive immune system. Determining the specificity of TCRs provides context for immunological studies and can be used to identify candidates for novel immunotherapies. To avoid costly experiments, large-scale TCR-epitope databases are queried for similar sequences via various distance functions. Here, we developed the deep-learning based distance meTCRs. Contrary to most previous approaches, the method avoids computational expansive pairwise string operations by comparing TCRs in a numeric embedding. In contrast to models which are trained specificity-agnostic, we directly utilize epitope information by applying deep metric learning to guide the training. Summarizing, we present meTCRs as a scalable alternative to embed TCR repertoires for clustering, visualisation, and querying against the ever-increasing amount TCR-epitope pairs in publicly available databases.
Copy rights belong to original authors. Visit the link for more info
Podcast created by Paper Player, LLC | |||
20 Mar 2023 | ITNR: Inversion Transformer-based Neural Ranking for Cancer Drug Recommendations | ||
Link to bioRxiv paper:
http://biorxiv.org/cgi/content/short/2023.03.16.533057v1?rss=1
Authors: Sotudian, S., Paschalidis, I. C.
Abstract:
Personalized drug response prediction is an approach for tailoring effective therapeutic strategies for patients based on their tumors' genomic characterization. The current study introduces a new listwise Learning-to-rank (LTR) model called Inversion Transformer-based Neural Ranking (ITNR). ITNR utilizes genomic features and a transformer architecture to decipher functional relationships and construct models that can predict patient-specific drug responses. Our experiments were conducted on three major drug response data sets, showing that ITNR reliably and consistently outperforms state-of-the-art LTR models.
Copy rights belong to original authors. Visit the link for more info
Podcast created by Paper Player, LLC | |||
29 Apr 2023 | scEpiTools: a database to comprehensively interrogate analytic tools for single-cell epigenomic data | ||
Link to bioRxiv paper:
http://biorxiv.org/cgi/content/short/2023.04.27.538652v1?rss=1
Authors: Gao, Z., Chen, X., Li, Z., Cui, X., Chen, S., Jiang, R.
Abstract:
Copy rights belong to original authors. Visit the link for more info
Podcast created by Paper Player, LLC | |||
20 Dec 2022 | EPEK: creation and analysis of an Ectopic Pregnancy Expression Knowledgebase | ||
Link to bioRxiv paper:
http://biorxiv.org/cgi/content/short/2022.12.20.521279v1?rss=1
Authors: Natarajan, A., Chivukula, N., Dhanakoti, G. B., Sahoo, A. K., Ravichandran, J., Samal, A.
Abstract:
Ectopic pregnancy (EP) is one of the leading causes of maternal mortality, where the fertilized embryo grows outside of the uterus. Recent experiments on mice have uncovered the importance of genetic factors in the transport of embryos inside the uterus. In the past, efforts have been made to identify possible gene or protein markers in EP in humans through multiple expression studies. Although there exist comprehensive gene resources for other maternal health disorders, there is no specific resource that compiles the genes associated with EP from such expression studies. Here, we address that knowledge gap by creating a computational resource, Ectopic Pregnancy Expression Knowledgebase (EPEK), that involves manual compilation and curation of expression profiles of EP in humans from published articles. In EPEK, we compiled information on 314 differentially expressed genes, 17 metabolites, and 3 SNPs associated with EP. Computational analyses on the gene set from EPEK showed the implication of cellular signaling processes in EP. We also identified possible exosome markers that could be clinically relevant in the diagnosis of EP. In a nutshell, EPEK is the first and only dedicated resource on the expression profile of EP in humans. EPEK is accessible at https://cb.imsc.res.in/epek.
Copy rights belong to original authors. Visit the link for more info
Podcast created by Paper Player, LLC | |||
18 Jul 2023 | rvTWAS: identifying gene-trait association using sequences by utilizing transcriptome-directed feature selection | ||
Link to bioRxiv paper:
http://biorxiv.org/cgi/content/short/2023.07.16.549227v1?rss=1
Authors: He, J., Li, Q., Zhang, Q.
Abstract:
Towards the identification of genetic basis of complex traits, transcriptome-wide association study (TWAS) is successful in integrating transcriptome data. However, TWAS is only applicable for common variants, excluding rare variants in exome or whole genome sequences. This is partly because of the inherent limitation of TWAS protocols that rely on predicting gene expressions. Briefly, a typical TWAS protocol has two steps: it trains an expression prediction model in a reference dataset containing gene expressions and genotype, and then applies this prediction model to a genotype-phenotype dataset to impute the unobserved expression (that is called GReX) to be associated to the phenotype. In this procedure, rare variants are not used due to its low power in predicting expressions. Our previous research has revealed the insight into TWAS: the two steps are essentially genetic feature selection and aggregations that do not have to involve predictions. Based on this insight disentangling TWAS, the inability of using rare variants to predict expression traits is no longer an obstacle. Herein, we developed rare variant TWAS, or rvTWAS, that first uses a Bayesian model to conduct expression-directed feature selection and then use a kernel machine to carry out feature aggregation, forming a model leveraging expressions for association mapping including rare variants. We demonstrated the performance of rvTWAS by thorough simulations and real data analysis in three psychiatric disorders, namely schizophrenia, bipolar disorder, and autism spectrum disorder. rvTWAS will open a door for sequence-based association mappings integrating gene expressions.
Copy rights belong to original authors. Visit the link for more info
Podcast created by Paper Player, LLC | |||
21 Apr 2023 | Correcting 4sU induced quantification bias in nucleotide conversion RNA-seq data | ||
Link to bioRxiv paper:
http://biorxiv.org/cgi/content/short/2023.04.21.537786v1?rss=1
Authors: Berg, K., Lodha, M., Garcia, Y. C., Hennig, T., Wolf, E., Prusty, B., Erhard, F.
Abstract:
Nucleoside analogues like 4-thiouridine (4sU) are used to metabolically label newly synthesized RNA. Chemical conversion of 4sU before sequencing induces T-to-C mismatches in reads sequenced from labelled RNA, allowing to obtain total and labelled RNA expression profiles from a single sequencing library. Cytotoxicity due to extended periods of labelling or high 4sU concentrations has been described, but the effects of extensive 4sU labelling on expression estimates from nucleotide conversion RNA-seq have not been studied. Here, we performed nucleotide conversion RNA-seq with escalating doses of 4sU with short-term labelling (1h) and over a progressive time course (up to 2h) in different cell lines. With high concentrations or at later time points, expression estimates were biased in an RNA half-life dependent manner. We show that bias arose by a combination of reduced mappability of reads carrying multiple conversions, and a global, unspecific underrepresentation of labelled RNA due to impaired reverse transcription efficiency and potentially global reduction of RNA synthesis. We developed a computational tool to rescue unmappable reads, which performed favourably compared to previous read mappers, and a statistical method, which could fully remove remaining bias. All methods developed here are freely available as part of our GRAND-SLAM pipeline and grandR package.
Copy rights belong to original authors. Visit the link for more info
Podcast created by Paper Player, LLC | |||
18 Jul 2023 | Brightest path tracing: A Python package to trace the brightest path in 2D and 3D images. | ||
Link to bioRxiv paper:
http://biorxiv.org/cgi/content/short/2023.07.16.549233v1?rss=1
Authors: Jha, V., Cudmore, R. H.
Abstract:
Brightest path tracing is a widely used image processing technique in several fields including biology, geography, and geology. However, despite the availability of many image processing libraries in Python, few offer an out-of-the-box implementation of a brightest path tracing algorithm. This paper presents a Python package, brightest-path-lib, that efficiently finds the path with maximum brightness between points in a 2D or 3D image. An example graphical user interface is provided as a Napari plugin. Taken together, the package and plugin provide a powerful and extensible tool for users to efficiently trace structures of interest in 2D or 3D images, regardless of the type of structure being analyzed.
Copy rights belong to original authors. Visit the link for more info
Podcast created by Paper Player, LLC | |||
25 Apr 2023 | SurVIndel2: improving local CNVs calling from next-generation sequencing using novel hidden information | ||
Link to bioRxiv paper:
http://biorxiv.org/cgi/content/short/2023.04.23.538018v1?rss=1
Authors: Rajaby, R., Sung, W.-K.
Abstract:
Deletions and tandem duplications are two major classes of structural variations. They frequently occur in highly repetitive regions, where existing methods fail to detect most of them. We previously introduced SurVIndel, an algorithm that tackled this issue by employing novel statistical methods and had improved sensitivity in repetitive regions. However, its precision was low. Here, we introduce SurVIndel2, an algorithm that uses a novel type of evidence (which we called hidden split reads) and also borrows and adapts the statistical approach of its predecessor. By combining these two approaches, SurVIndel2 is both more sensitive and more precise than any other method we tested.
Copy rights belong to original authors. Visit the link for more info
Podcast created by Paper Player, LLC | |||
15 Jan 2023 | Leveraging public transcriptome data with machine learning to infer pan-body age- and sex-specific molecular phenomena | ||
Link to bioRxiv paper:
http://biorxiv.org/cgi/content/short/2023.01.12.523796v1?rss=1
Authors: Johnson, K. A., Krishnan, A.
Abstract:
Age and sex are historically understudied factors in biomedical studies even though many complex traits and diseases vary by these factors in their incidence and presentation. As a result, there are massive gaps in our understanding of genes and molecular mechanisms that underlie sex- and age-associated physiology and disease. Hundreds of thousands of publicly-available human transcriptomes capturing gene expression profiles of tissues across the body and subject to various biomedical and clinical factors present an invaluable, yet untapped, opportunity for bridging these gaps. Here, we present a computational framework that leverages these data to infer genome-wide molecular signatures specific to sex and age groups. As the vast majority of these profiles lack age and sex labels, the core idea of our framework is to use the measured expression data to predict missing age/sex metadata and derive the signatures from the predictive models. We first curated ~30,000 primary samples associated with age and sex information and profiled using microarray and RNA-seq. Then, we used this dataset to infer sex-biased genes within eleven age groups along the human lifespan and then trained machine learning (ML) models to predict these age groups from gene expression values separately within females and males. Specifically, we trained one-vs-rest logistic regression classifiers with elastic-net regularization to classify transcriptomes into age groups. Dataset-level cross validation shows that these ML classifiers are able to discriminate between age groups in a biologically meaningful way in each sex across technologies. Further, these predictive models capture sex-stratified age-group 'gene signatures', i.e., the strength and the direction of importance of genes across the genome for each age group in each sex. Enrichment analysis of these gene signatures with prior gene annotations helped in identifying age- and sex-associated multi-tissue and pan-body molecular phenomena (e.g., general immune response, inflammation, metabolism, hormone response). Overall, we have presented a path for effectively leveraging massive public omics data collections to investigate the molecular basis of age- and sex-differences in physiology and disease.
Copy rights belong to original authors. Visit the link for more info
Podcast created by Paper Player, LLC | |||
31 Jan 2023 | Single-cell multi-omic topic embedding reveals cell-type-specific and COVID-19 severity-related immune signatures | ||
Link to bioRxiv paper:
http://biorxiv.org/cgi/content/short/2023.01.31.526312v1?rss=1
Authors: Zhou, M., Zhang, H., Bai, Z., Mann-Krzisnik, D., Wang, F., Li, Y.
Abstract:
The advent of single-cell multi-omics sequencing technology makes it possible for researchers to leverage multiple modalities for individual cells and explore cell heterogeneity. However, the high dimensional, discrete, and sparse nature of the data make the downstream analysis particularly challenging. Most of the existing computational methods for single-cell data analysis are either limited to single modality or lack flexibility and interpretability. In this study, we propose an interpretable deep learning method called multi-omic embedded topic model (moETM) to effectively perform integrative analysis of high-dimensional single-cell multimodal data. moETM integrates multiple omics data via a product-of-experts in the encoder for efficient variational inference and then employs multiple linear decoders to learn the multi-omic signatures of the gene regulatory programs. Through comprehensive experiments on public single-cell transcriptome and chromatin accessibility data (i.e., scRNA+scATAC), as well as scRNA and proteomic data (i.e., CITE-seq), moETM demonstrates superior performance compared with six state-of-the-art single-cell data analysis methods on seven publicly available datasets. By applying moETM to the scRNA+scATAC data in human peripheral blood mononuclear cells (PBMCs), we identified sequence motifs corresponding to the transcription factors that regulate immune gene signatures. Applying moETM analysis to CITE-seq data from the COVID-19 patients revealed not only known immune cell-type-specific signatures but also composite multi-omic biomarkers of critical conditions due to COVID-19, thus providing insights from both biological and clinical perspectives.
Copy rights belong to original authors. Visit the link for more info
Podcast created by Paper Player, LLC | |||
02 Apr 2023 | A Bayesian method to infer copy number clones from single-cell RNA and ATAC sequencing | ||
Link to bioRxiv paper:
http://biorxiv.org/cgi/content/short/2023.04.01.535197v1?rss=1
Authors: Patruno, L., Milite, S., Bergamin, R., Calonaci, N., D'Onofrio, A., Anselmi, F., Antoniotti, M., Graudenzi, A., Caravagna, G.
Abstract:
Single-cell RNA and ATAC sequencing technologies allow one to probe expression and chromatin accessibility states as a proxy for cellular phenotypes at the resolution of individual cells. A key challenge of cancer research is to consistently map such states on genetic clones, within an evolutionary framework. To this end we introduce CONGAS+, a Bayesian model to map single-cell RNA and ATAC profiles generated from independent or multimodal assays on the latent space of copy numbers clones. CONGAS+ can detect tumour subclones associated with aneuploidy by clustering cells with the same ploidy profile. The framework is implemented in a probabilistic language that can scale to analyse thousands of cells thanks to GPU deployment. Our tool exhibits robust performance on simulations and real data, highlighting the advantage of detecting aneuploidy from two distinct molecules as opposed to other single-molecule models, and also leveraging real multi-omic data. In the application to prostate cancer, lymphoma and basal cell carcinoma, CONGAS+ did retrieve complex subclonal architectures while providing a coherent mapping among ATAC and RNA, facilitating the study of genotype-phenotype mapping, and their relation to tumour aneuploidy.
Copy rights belong to original authors. Visit the link for more info
Podcast created by Paper Player, LLC | |||
22 Dec 2022 | A method for differential expression analysis and pseudo- temporal locating and ordering of genes in single-cell transcriptomic data | ||
Link to bioRxiv paper:
http://biorxiv.org/cgi/content/short/2022.12.21.521359v1?rss=1
Authors: Zhang, B., Zhang, H.
Abstract:
Identification of differentially expressed genes (DEGs) is a pivotal step in single-cell RNA sequencing (scRNA-seq) data analysis. The sparsity and multi-model distribution of scRNA-seq data decides that the traditional tools designed for bulk RNA-seq have several limitations when applied to single-cell data. On the other hand, tools specifically for DEGs analysis of scRNA-seq data normally does not consider the high dimensionality of the data. To this end, we present DEAPLOG, a method for differential expression analysis and pseudo-temporal locating and ordering of genes in single-cell transcriptomic data. We show that DEAPLOG has higher accurate and efficient in DEGs identification when compared with existing methods in both artificial and real datasets. Additionally, DEAPLOG can infer pseudo-time and embedding coordinates of genes, therefore is useful in identifying regulators in trajectory of cell fate decision.
Copy rights belong to original authors. Visit the link for more info
Podcast created by Paper Player, LLC | |||
04 Apr 2023 | SEQUENCE VS. STRUCTURE: DELVING DEEP INTO DATA DRIVEN PROTEIN FUNCTION PREDICTION | ||
Link to bioRxiv paper:
http://biorxiv.org/cgi/content/short/2023.04.02.534383v1?rss=1
Authors: Tian, X., Wang, Z., Yang, K. K., Su, J., Du, H., Zheng, Q., Guo, G., Yang, M., Yang, F., Yuan, F.
Abstract:
Predicting protein function is a longstanding challenge that has significant scientific implications. The success of amino acid sequence-based learning methods depends on the relationship between sequence, structure, and function. However, recent advances in AlphaFold have led to highly accurate protein structure data becoming more readily available, prompting a fundamental question: given sufficient experimental and predicted structures, should we use structure-based learning methods instead of sequence-based learning methods for predicting protein function, given the intuition that a protein's structure has a closer relationship to its function than its amino acid sequence? To answer this question, we explore several key factors that affect function prediction accuracy. Firstly, we learn protein representations using state-of-the-art graph neural networks (GNNs) and compare graph construction(GC) methods at the residue and atomic levels. Secondly, we investigate whether protein structures generated by AlphaFold are as effective as experimental structures for function prediction when protein graphs are used as input. Finally, we compare the accuracy of sequence-only, structure-only, and sequence-structure fusion-based learning methods for predicting protein function. Additionally, we make several observations, provide useful tips, and share code and datasets to encourage further research and enhance reproducibility.
Copy rights belong to original authors. Visit the link for more info
Podcast created by Paper Player, LLC | |||
01 Mar 2023 | ClockBase: a comprehensive platform for biological age profiling in human and mouse | ||
Link to bioRxiv paper:
http://biorxiv.org/cgi/content/short/2023.02.28.530532v1?rss=1
Authors: Ying, K., Tyshkovskiy, A., Trapp, A., Liu, H., Moqri, M., Kerepesi, C., Gladyshev, V. N.
Abstract:
Aging represents the greatest risk factor for chronic diseases and mortality, but to understand it, we need the ability to measure biological age. In recent years, many machine learning algorithms based on omics data, termed aging clocks, have been developed that can accurately predict the age of biological samples. However, there is currently no resource for systematic profiling of biological age. Here, we describe ClockBase, a platform that features biological age estimates based on multiple aging clock models applied to more than 2,000 DNA methylation datasets and nearly 200,000 samples. We further provide an online interface for statistical analyses and visualization of the data. To show how this resource could facilitate the discovery of biological age-modifying factors, we describe a novel anti-aging drug candidate, zebularine, which reduces the biological age estimates based on all aging clock models tested. We also show that pulmonary fibrosis accelerates epigenetic age. Together, ClockBase provides a resource for the scientific community to quantify and explore biological ages of samples, thus facilitating discovery of new longevity interventions and age-accelerating conditions.
Copy rights belong to original authors. Visit the link for more info
Podcast created by Paper Player, LLC | |||
25 Jul 2023 | ClusterDE: a post-clustering differential expression (DE) method robust to false-positive inflation caused by double dipping | ||
Link to bioRxiv paper:
http://biorxiv.org/cgi/content/short/2023.07.21.550107v1?rss=1
Authors: Song, D., Li, K., Ge, X., Li, J. J.
Abstract:
In typical single-cell RNA-seq (scRNA-seq) data analysis, a clustering algorithm is applied to find putative cell types as clusters, and then a statistical differential expression (DE) test is used to identify the differentially expressed (DE) genes between the cell clusters. However, this common procedure uses the same data twice, an issue known as "double dipping": the same data is used to define both cell clusters and DE genes, leading to false-positive DE genes even when the cell clusters are spurious. To overcome this challenge, we propose ClusterDE, a post-clustering DE test for controlling the false discovery rate (FDR) of identified DE genes regardless of clustering quality. The core idea of ClusterDE is to generate real-data-based synthetic null data with only one cluster, as a counterfactual in contrast to the real data, for evaluating the whole procedure of clustering followed by a DE test. Using comprehensive simulation and real data analysis, we show that ClusterDE has not only solid FDR control but also the ability to find cell-type marker genes that are biologically meaningful. ClusterDE is fast, transparent, and adaptive to a wide range of clustering algorithms and DE tests. Besides scRNA-seq data, ClusterDE is generally applicable to post-clustering DE analysis, including single-cell multi-omics data analysis.
Copy rights belong to original authors. Visit the link for more info
Podcast created by Paper Player, LLC | |||
30 Jun 2023 | PSite: inference of read-specific P-site offsets for ribosomal footprints | ||
Link to bioRxiv paper:
http://biorxiv.org/cgi/content/short/2023.06.27.546788v1?rss=1
Authors: Chang, Y., Lei, T., Zhang, H.
Abstract:
Ribosome profiling is a powerful method for global survey of ribosomal footprints. Inferring the offsets of footprint 5' ends to the ribosomal P-site is essential to pinpoint codons translated by ribosomes. By convention, global or read length-specific P-site offsets are estimated by inspecting the distribution of ribosome footprints around the annotated start or stop codons. However, actual offsets might be different even for footprints of the same length due to the influence of sequence context and the cutting bias of endoribonucleases. To address this issue, we present PSite, a python package for inferring read-specific P-site offsets using a gradient boosting trees model. PSite assigned more reads to the correct reading frame than conventional methods and improved the prediction of translated ORFs by existing software. Besides, PSite is robust to ribosome profiling datasets of varying quality or using endonucleases with cutting bias for digestion.
Copy rights belong to original authors. Visit the link for more info
Podcast created by Paper Player, LLC | |||
24 Jun 2023 | Fine-tuning Protein Embeddings for GeneralizableAnnotation Propagation | ||
Link to bioRxiv paper:
http://biorxiv.org/cgi/content/short/2023.06.22.546084v1?rss=1
Authors: Dickson, A. M., Mofrad, M. R. K.
Abstract:
A central goal of bioinformatics research is to understand proteins on a functional level, typically by extrapolating from experimental results with the protein sequence information. One strategy is to assume that proteins with similar sequences will also share function. This has the benefit of being interpretable; it gives a very clear idea of why a protein might have a particular function by comparing with the most similar reference example. However, direct machine learning classifiers now outperform pure sequence similarity methods in raw prediction ability. A hybrid method is to use pre-trained language models to create protein embeddings, and then indirectly predict protein function using their relative similarity. We find that fine-tuning an auxiliary objective on protein function indirectly improves these hybrid methods, to the point that they are in some cases better than direct classifiers. Our empirical results demonstrate that interpretable protein comparison models can be developed using fine-tuning techniques, without cost, or even with some benefit, to overall performance. K-nearest neighbors (KNN) embedding-based models also offer free generalization to previously unknown classes, while continuing to outperform only pre-trained models, further demonstrating the potential of fine-tuned embeddings outside of direct classification.
Copy rights belong to original authors. Visit the link for more info
Podcast created by Paper Player, LLC | |||
21 Jan 2023 | Prediction Analysis of Preterm Neonates Mortality using Machine Learning Algorithms via Python Programming | ||
Link to bioRxiv paper:
http://biorxiv.org/cgi/content/short/2023.01.20.524905v1?rss=1
Authors: Monfared, V., Hashemi, A.
Abstract:
Prediction analysis of preterm neonate mortality is necessary and significant for benchmarking and evaluating healthcare services in Hospitals and other medical centers. Application of artificial intelligence and machine learning models, which is a hot topic in medicine/healthcare and engineering, may improve physicians skill to predict the preterm neonatal deaths. The main purpose of this research article is to introduce a preterm neonatal mortality risk prediction by means of machine learning/ML predictive models to survive infants using supervised ML models if possible. Moreover, this paper presents some effective parameters and features which affect to survive the infants directly. It means, the obtained model has an accuracy of about 91.5% to predict the status of infant after delivery. After recognizing the critical status for an infant, physicians and other healthcare personnel can help to infant for possible surviving using special medical NICU cares. It has been tried to get some suitable models with high accuracy and comparing the results. In a word, a survival prediction analysis of preterm neonate mortality has been carried out using machine learning methods via Python programming (possible surviving infants after delivery in the hospital).
Copy rights belong to original authors. Visit the link for more info
Podcast created by Paper Player, LLC | |||
18 Nov 2022 | Evaluating the analytical validity of mutation calling pipeline for tumor whole exome sequencing | ||
Link to bioRxiv paper:
http://biorxiv.org/cgi/content/short/2022.11.17.516840v1?rss=1
Authors: Cheng, C., Huang, J.-H., Hsu, J. S.
Abstract:
Detecting somatic mutations from the patients' tumor tissues has the clinical impacts in medical decision making. Library preparation methods, sequencing platforms, read alignment tools and variant calling algorithms are the major factors to influence the data analysis results. Understanding the performance of the tool combinations of the somatic variant calling pipelines has become an important issue in the use of the whole exome sequences (WES) analysis in clinical actions. In this study, we selected four state-of-the-art sequence aligners including BWA, Bowtie2, DRAGMAP, DRAGEN aligner (DragenA) and HISAT2. For the variant callers, we chose GATK Mutect2, Sentieon TNscope, DRAGEN caller (DragenC) and DeepVariant. The benchmarking tumor whole exome sequencing data released from the FDA-led Sequencing and Quality Control Phase 2 (SEQC2) consortium was applied as the true positive variants to evaluate the overall performance. Multiple combinations of the aligners and variant callers were used to assess the variation detection capability. We measured the recall, precision and F1-score for each combination in both single nucleotide variants (SNVs) and short insertions and deletions (InDels) variant detections. We also evaluated their performances in different variant allele frequencies (VAFs) and the base pair length. The results showed that the top recall, precision and F1-score in the SNVs detection were generated by the combinations of BWA+DragenC(0.9629), Bowtie2+TNscope(0.9957) and DRAGMAP+DragenC(0.9646), respectively. In the InDels detection, BWA+DragenC(0.9546), Hisat2+TNscope(0.7519) and DragenA+DragenC(0.8081) outperformed the other combinations in the recall, precision and F1-Score, respectively. In addition, we found that the variant callers could bias the variant calling results. Finally, although some combinations yielded high accuracies of variant detection, but some variants still could not be detected by these outperformed combinations. The results of this study provided the vital information that no single combination could achieve superior results in detecting all the variants of the benchmarking dataset. In conclusion, applying both merged-based and ensemble-based variants detection approaches is encouraged to further detect variants comprehensively.
Copy rights belong to original authors. Visit the link for more info
Podcast created by Paper Player, LLC | |||
02 Jul 2023 | Entropy-based decoy generation methods for accurate FDR estimation in large-scale metabolomics annotations. | ||
Link to bioRxiv paper:
http://biorxiv.org/cgi/content/short/2023.07.02.547371v1?rss=1
Authors: An, S., Lu, M., Wang, R., Wang, J., Xie, C., Tong, J., Jiang, H., Yu, C.
Abstract:
Large-scale metabolomics research faces challenges in accurate metabolite annotation and false discovery rate (FDR) estimation. Recent progress in addressing these challenges has leveraged experience from proteomics and inspiration from other sciences. Although the target-decoy strategy has been applied to metabolomics, generating reliable decoy libraries is difficult due to the complexity of metabolites. Additionally, continuous bioinformatic efforts are necessary to increase the utilization of growing spectra resources while reducing false identifications. Here we introduce the concept of ion entropy and present two entropy-based decoy generation methods. The assessment of public spectral databases using ion entropy validated it as a good metric for ion information content in massive metabolomics data. The decoy generation method developed based on this concept outperformed current representative decoy strategies in metabolomics and achieved the best FDR estimation performance. We analyzed 47 public metabolomics datasets using the constructed workflow to provide instructive suggestions. Finally, we present MetaPhoenix, a tool equipped with a well-constructed FDR estimation workflow that facilitates the development of accurate FDR-controlled analysis in the metabolomics field.
Copy rights belong to original authors. Visit the link for more info
Podcast created by Paper Player, LLC | |||
13 Apr 2023 | PGSbuilder: An end-to-end platform for human genome association analysis and polygenic risk score predictions | ||
Link to bioRxiv paper:
http://biorxiv.org/cgi/content/short/2023.04.12.536584v1?rss=1
Authors: Lee, K.-H., Lee, Y.-L., Hsieh, T.-T., Chang, Y.-C., Wang, S.-S., Fann, G.-Z., Lin, W.-C., Chen, T.-F., Li, P.-H., Kuo, Y.-L., Chen, P.-L., Juan, H.-F., Tsai, H.-K., Chen, C.-Y., Huang, J.-H.
Abstract:
Understanding the genetic basis of human complex diseases is increasingly important in the development of precision medicine. Over the last decade, genome-wide association studies (GWAS) have become a key technique for detecting associations between common diseases and single nucleotide polymorphisms (SNPs) present in a cohort of individuals. Alternatively, the polygenic risk score (PRS), which often applies results from GWAS summary statistics, is calculated for the estimation of genetic propensity to a trait at the individual level. Despite many GWAS and PRS tools being available to analyze a large volume of genotype data, most clinicians and medical researchers are often not familiar with the bioinformatics tools and lack access to a high-performance computing cluster resource. To fill this gap, we provide a publicly available web server, PGSbuilder, for the GWAS and PRS analysis of human genomes with variant annotations. The user-friendly and intuitive PGSbuilder web server is developed to facilitate the discovery of the genetic variants associated with complex traits and diseases for medical professionals with limited computational skills. For GWAS analysis, PGSbuilder provides the most renowned analysis tool PLINK 2.0 package. For PRS, PGSbuilder provides six different PRS methods including Clumping and Thresholding, Lassosum, LDPred2, GenEpi, PRS-CS, and PRSice2. Furthermore, PGSbuilder provides an intuitive user interface to examine the annotated functional effects of variants from known biomedical databases and relevant literature using advanced natural language processing approaches. In conclusion, PGSbuilder offers a reliable platform to aid researchers in advancing the public perception of genomic risk and precision medicine for human disease genetics. PGSbuilder is freely accessible at http://pgsb.tw23.org.
Copy rights belong to original authors. Visit the link for more info
Podcast created by Paper Player, LLC | |||
21 Jan 2023 | Accurate age prediction from blood using of small set of DNA methylation sites and a cohort-based machine learning algorithm | ||
Link to bioRxiv paper:
http://biorxiv.org/cgi/content/short/2023.01.20.524874v1?rss=1
Authors: Varshavsky, M., Harari, G., Glaser, B., Dor, Y., Shemer, R., Kaplan, T.
Abstract:
Chronological age prediction from DNA methylation sheds light on human aging, indicates poor health and predicts lifespan. Current clocks are mostly based on linear models from hundreds of methylation sites, and are not suitable for sequencing-based data.
We present GP-age, an epigenetic clock for blood, that uses a non-linear cohort-based model of 11,910 blood methylomes. Using 30 CpG sites alone, GP-age outperforms state-of-the-art models, with a median accuracy of ~2 years on held-out blood samples, for both array and sequencing-based data. We show that aging-related changes occur at multiple neighboring CpGs, with far-reaching implications on aging research at the cellular level. By training three independent clocks, we show consistent deviations between predicted and actual age, suggesting individual rates of biological aging.
Overall, we provide a compact yet accurate alternative to array-based clocks for blood, with future applications in longitudinal aging research, forensic profiling, and monitoring epigenetic processes in transplantation medicine and cancer.
Graphical abstract
O_FIG O_LINKSMALLFIG WIDTH=158 HEIGHT=200 SRC="FIGDIR/small/524874v1_ufig1.gif" ALT="Figure 1" greater than
View larger version (31K):
org.highwire.dtl.DTLVardef@1109545org.highwire.dtl.DTLVardef@1b82214org.highwire.dtl.DTLVardef@1c5812aorg.highwire.dtl.DTLVardef@1a32dee_HPS_FORMAT_FIGEXP M_FIG C_FIG O_LIMachine learning analysis of a large cohort (~12K) of DNA methylomes from blood
C_LIO_LIA 30-CpG regression model achieves a 2.1-year median error in predicting age
C_LIO_LIImproved accuracy ( greater than or equal to 1.75 years) from sequencing data, using neighboring CpGs
C_LIO_LIPaves the way for easy and accurate age prediction from blood, using NGS data
C_LI
MotivationEpigenetic clocks that predict age from DNA methylation are a valuable tool in the research of human aging, with additional applications in forensic profiling, disease monitoring, and lifespan prediction. Most existing epigenetic clocks are based on linear models and require hundreds of methylation sites. Here, we present a compact epigenetic clock for blood, which outperforms state-of-the-art models using only 30 CpG sites. Finally, we demonstrate the applicability of our clock to sequencing-based data, with far reaching implications for a better understanding of epigenetic aging.
Copy rights belong to original authors. Visit the link for more info
Podcast created by Paper Player, LLC | |||
25 Oct 2022 | Fever temperatures modulate intraprotein dynamics and enhance the binding affinity between monoclonal antibodies and the Spike protein from SARS-CoV-2 | ||
Link to bioRxiv paper:
http://biorxiv.org/cgi/content/short/2022.10.24.513610v1?rss=1
Authors: Kim, D. G., Kim, H. S., Choi, Y., Stan, R. C.
Abstract:
Fever is a typical symptom of most infectious diseases. While prolonged fever may be clinically undesirable, mild reversible fever ( less than 39, 312K) can potentiate the immune responses against pathogens. Here, using molecular dynamics, we investigated the effect of febrile temperatures (38 to 40, 311K to 313K) on the immune complexes formed by the SARS-CoV-2 spike protein with two neutralizing antibodies. We found that, at mild fever temperatures (311-312K), the binding affinities of the two antibodies improve when compared to the physiological body temperature (37, 310K). Furthermore, only at 312K, antibodies exert distinct mechanical effects on the receptor binding domains of the spike protein that may hinder SARS-CoV-2 infectivity. Enhanced antibody binding affinity may thus be obtained using appropriate temperature conditions.
Copy rights belong to original authors. Visit the link for more info
Podcast created by Paper Player, LLC | |||
23 Dec 2022 | mapquik: Efficient low-divergence mapping of long reads in minimizer space | ||
Link to bioRxiv paper:
http://biorxiv.org/cgi/content/short/2022.12.23.521809v1?rss=1
Authors: Ekim, B., Sahlin, K., Medvedev, P., Berger, B., Chikhi, R.
Abstract:
DNA sequencing data continues to progress towards longer reads with increasingly lower sequencing error rates. We focus on the critical problem of mapping, or aligning, low-divergence sequences from long reads (PacBio HiFi) to a reference genome, which poses challenges in terms of accuracy and computational resources when using cutting-edge read mapping approaches that are designed for all types of alignments. A natural idea would be to optimize efficiency with longer seeds to reduce the probability of extraneous matches; however, contiguous exact seeds quickly reach a sensitivity limit. We introduce mapquik, a novel strategy that creates accurate longer seeds by anchoring alignments through matches of k consecutively-sampled minimizers (k-min-mers) and only indexing k-min-mers that occur once in the reference genome, thereby unlocking ultra-fast mapping while retaining high sensitivity. We demonstrate that mapquik significantly accelerates the seeding and chaining steps - fundamental bottlenecks to read mapping - for both the human and maize genomes with greater than 96% sensitivity and near-perfect specificity. On the human genome, mapquik achieves a 30x speed-up over the state-of-the-art tool minimap2, and on the maize genome, a 350x speed-up over minimap2, making mapquik the fastest mapper to date. These accelerations are enabled not only by minimizer-space seeding but also a novel heuristic O(n) pseudo-chaining algorithm, which improves over the long-standing O(n log n) bound. Minimizer-space computation builds the foundation for achieving real-time analysis of long-read sequencing data.
Copy rights belong to original authors. Visit the link for more info
Podcast created by Paper Player, LLC | |||
03 Dec 2022 | Investigating graph neural network for RNA structural embedding | ||
Link to bioRxiv paper:
http://biorxiv.org/cgi/content/short/2022.12.02.515916v1?rss=1
Authors: opuu, v., Bret, H.
Abstract:
The biological function of natural non-coding RNAs (ncRNA) is tightly bound to their molecular structure. Sequence analyses such as multiple sequence alignments (MSA) are the bread and butter of bio-molecules functional analysis; however, analyzing sequence and structure simultaneously is a difficult task. In this work, we propose CARNAGE (Clustering/Alignment of RNA with Graph-network Embedding), which leverages a graph neural network encoder to imprint structural information into a sequence-like embedding; therefore, downstream sequence analyses now account implicitly for structural constraints. In contrast to the traditional "supervised" alignment approaches, we trained our network on a masking problem, independent from the alignment or clustering problem. Our method is very versatile and has shown good performances in 1) designing RNAs sequences, 2) clustering sequences, and 3) aligning multiple sequences only using the simplest Needleman and Wunsch's algorithm. Not only can this approach be readily extended to RNA tridimensional structures, but it can also be applied to proteins.
Copy rights belong to original authors. Visit the link for more info
Podcast created by Paper Player, LLC | |||
22 Jul 2023 | Cellular proliferation biases clonal lineage tracing and trajectory inference | ||
Link to bioRxiv paper:
http://biorxiv.org/cgi/content/short/2023.07.20.549801v1?rss=1
Authors: Bonham-Carter, B., Schiebinger, G.
Abstract:
We identify a fundamental statistical phenomenon in single-cell time courses with clone-based lineage tracing. Through simple probabilistic arguments, we show how the relative growth rates of cells influence the probability that they will be sampled in clones observed across multiple time points. We support these arguments with a simple simulation study and a time-course of T-cell development, and we demonstrate that this bias can impact fate probability predictions from trajectory inference methods. Finally, we explore how to develop trajectory inference methods which are robust to this bias. In particular, we show how to extend LineageOT to use data from clones observed across multiple time points.
Copy rights belong to original authors. Visit the link for more info
Podcast created by Paper Player, LLC | |||
02 Apr 2023 | AI-based novel-chemotype GPCRs drugs: introducing ligand type classifiers and systems biology | ||
Link to bioRxiv paper:
http://biorxiv.org/cgi/content/short/2023.03.31.535043v1?rss=1
Authors: Gossen, J., Ribeiro, R. P., Bier, D., Neumaier, B., Carloni, P., Giorgetti, A., Rossetti, G.
Abstract:
Identifying the correct chemotype of ligands targeting receptors (i.e., agonist or antagonist) is a challenge for in silico screening campaigns. Here we present an approach that identifies novel chemotype ligands by combining structural data with a random forest agonist/antagonist classifier and a signal-transduction kinetic model. As a test case, we apply this approach to identify novel antagonists of the human adenosine transmembrane receptor type 2A, an attractive target against Parkinson's disease and cancer. The identified antagonists were tested here in a radioligand binding assay. Among those, we found a promising ligand whose chemotype differs significantly from all so-far reported antagonists, with a binding affinity of 310{+/-}23.4 nM. Thus, our protocol emerges as a powerful approach to identify promising ligand candidates with novel chemotypes while preserving antagonistic potential and affinity in the nanomolar range.
Copy rights belong to original authors. Visit the link for more info
Podcast created by Paper Player, LLC | |||
05 Apr 2023 | asmbPLS: Adaptive Sparse Multi-block Partial Least Square for Survival Prediction using Multi-Omics Data | ||
Link to bioRxiv paper:
http://biorxiv.org/cgi/content/short/2023.04.03.535442v1?rss=1
Authors: Zhang, R., Datta, S.
Abstract:
Background As high-throughput studies advance, more and more high-dimensional multi-omics data are available and collected from the same patient cohort. Using multi-omics data as predictors to predict survival outcomes is challenging due to the complex structure of such data. Results In this article, we introduce an adaptive sparse multi-block partial least square (asmbPLS) regression method by assigning different penalty factors to different blocks in different PLS components for feature selection and prediction. We compared the proposed method with several competitive algorithms in many aspects including prediction performance, feature selection and computation efficiency. The performance and the efficiency of our method were demonstrated using both the simulated and the real data. Conclusions In summary, asmbPLS achieved a competitive performance in prediction, feature selection, and computation efficiency. We anticipate asmbPLS to be a valuable tool for multi-omics research. An R package called asmbPLS implementing this method is made publicly available on GitHub.
Copy rights belong to original authors. Visit the link for more info
Podcast created by Paper Player, LLC | |||
20 Dec 2022 | CircPrime: a web-based platform for design of specific circular RNA primers | ||
Link to bioRxiv paper:
http://biorxiv.org/cgi/content/short/2022.12.20.521155v1?rss=1
Authors: Sharko, F., Rbbani, G., Siriyappagouder, P., Raeymaekers, J. A. M., Galindo-Villegas, J., Nedoluzhko, A., Fernandes, J. M. O.
Abstract:
Background: Circular RNAs (circRNAs) are covalently closed-loop RNAs with critical regulatory roles in cells. The tenth of thousands of circRNAs have been unveiled due to the recent advances in high throughput RNA sequencing technologies and bioinformatic tools development. At the same time, polymerase chain reaction (PCR) cross-validation for circRNAs predicted by bioinformatic tools remains an essential part of any circRNA study before publication. Results: Here, we present the CircPrime web-based platform, providing a user-friendly solution for DNA primer design and thermocycling conditions for circRNA identification with routine PCR methods. Conclusions: User-friendly CircPrime web platform (http://circprime.elgene.net/) works with outputs of the most popular bioinformatic predictors of circRNAs to design specific circular RNA primers. CircPrime works with circRNA coordinates and any reference genome from the National Center for Biotechnology Information database (NCBI).
Copy rights belong to original authors. Visit the link for more info
Podcast created by Paper Player, LLC | |||
10 Feb 2023 | Pharmacodynamic model of PARP1 inhibition and global sensitivity analyses can lead to cancer biomarker discovery | ||
Link to bioRxiv paper:
http://biorxiv.org/cgi/content/short/2023.02.08.527527v1?rss=1
Authors: Mertins, S. D., Isenberg, N. M., Reyes, K.-R., Yoon, B.-J., Urban, N., Jogalekar, M. P., Diolaiti, M. E., Weil, M. R., Stahlberg, E. A.
Abstract:
Pharmacodynamic models provide inroads to understanding key mechanisms of action and may significantly improve patient outcomes in cancer with improved ability to determine therapeutic benefit. Additionally, these models may also lead to insights into potential biomarkers that can be utilized for prediction in prognosis and therapeutic decisions. As an example of this potential, here we present an advanced computational Ordinary Differential Equation (ODE) model of PARP1 signalling and downstream effects due to its inhibition. The model has been validated experimentally and further evaluated through a global sensitivity analysis. The sensitivity analysis uncovered two model parameters related to protein synthesis and degradation rates that were also found to contribute the most variability to the therapeutic prediction. Because this variability may define cancer patient subpopulations, we interrogated genomic, transcriptomic, and clinical databases, to uncover a biomarker that may correspond to patient outcomes in the model. In particular, GSPT2, a GTPase with translation function, was discovered and if mutations serve to alter catalytic activity, its presence may explain the variability in the model's parameters. This work offers an analysis of ODE models, inclusive of model development, sensitivity analysis, and ensuing experimental data analysis, and demonstrates the utility of this methodology in uncovering biomarkers in cancer.
Copy rights belong to original authors. Visit the link for more info
Podcast created by Paper Player, LLC | |||
14 Mar 2023 | Analysis of RNA processing directly from spatial transcriptomics data reveals previously unknown regulation | ||
Link to bioRxiv paper:
http://biorxiv.org/cgi/content/short/2023.03.13.532412v1?rss=1
Authors: Olivieri, J. E., Salzman, J.
Abstract:
Technical advances have led to an explosion in the amount of biological data available in recent years, especially in the field of RNA sequencing. Specifically, spatial transcriptomics (ST) datasets, which allow each RNA molecule to be mapped to the 2D location it originated from within a tissue, have become readily available. Due to computational challenges, ST data has rarely been used to study RNA processing such as splicing or differential UTR usage. We apply the ReadZS and the SpliZ, methods developed to analyze RNA process in scRNA-seq data, to analyze spatial localization of RNA processing directly from ST data for the first time. Using Moran's I metric for spatial autocorrelation, we identify genes with spatially regulated RNA processing in the mouse brain and kidney, re-discovering known spatial regulation in Myl6 and identifying previously-unknown spatial regulation in genes such as Rps24, Gng13, Slc8a1, Gpm6a, Gpx3, ActB, Rps8, and S100A9. The rich set of discoveries made here from commonly used reference datasets provides a small taste of what can be learned by applying this technique more broadly to the large quantity of Visium data currently being created.
Copy rights belong to original authors. Visit the link for more info
Podcast created by Paper Player, LLC | |||
16 Nov 2022 | Revisiting pangenome openness with k-mers | ||
Link to bioRxiv paper:
http://biorxiv.org/cgi/content/short/2022.11.15.516472v1?rss=1
Authors: Parmigiani, L., Wittler, R., Stoye, J.
Abstract:
Pangenomics is the study of related genomes collectively, usually from the same species or closely related taxa. Originally, pangenomes were defined for bacterial species. After the concept was extended to eukaryotic genomes, two definitions of pangenome evolved in parallel: the gene-based approach, which defines the pangenome as the union of all genes, and the sequence-based approach, which defines the pangenome as the set of all nonredundant genomic sequences. Estimating the total size of the pangenome for a given species has been subject of study since the very first mention of pangenomes. Traditionally, this is performed predicting the ratio at which new genes are discovered, referred to as the openness of the species. Here, we abstract each genome as a set of items, which is entirely agnostic of the two approaches (gene-based, sequence-based). Genes are a viable option for items, but also other possibilities are feasible, e.g., genome sequence substrings of fixed length k (k-mers). In the present study, we investigate the use of k-mers to estimate the openness as an alternative to genes, and compare the results. An efficient implementation is also provided.
Copy rights belong to original authors. Visit the link for more info
Podcast created by Paper Player, LLC | |||
24 Nov 2022 | excluderanges: exclusion sets for T2T-CHM13, GRCm39, and other genome assemblies | ||
Link to bioRxiv paper:
http://biorxiv.org/cgi/content/short/2022.11.21.517407v1?rss=1
Authors: Ogata, J. D., Mu, W., Davis, E. S., Xue, B., Harrell, J. C., Sheffield, N. C., Phanstiel, D. H., Love, M. I., Dozmorov, M. G.
Abstract:
Summary: Exclusion regions are sections of reference genomes with abnormal pileups of short sequencing reads. Removing reads overlapping them improves biological signal, and these benefits are most pronounced in differential analysis settings. Several labs created exclusion region sets, available primarily through ENCODE and Github. However, the variety of exclusion sets creates uncertainty which sets to use. Furthermore, gap regions (e.g., centromeres, telomeres, short arms) create additional considerations in generating exclusion sets. We generated exclusion sets for the latest human T2T-CHM13 and mouse GRCm39 genomes and systematically assembled and annotated these and other sets in the excluderanges R/Bioconductor data package, also accessible via the BEDbase.org API. The package provides unified access to 82 GenomicRanges objects covering six organisms, multiple genome assemblies and types of exclusion regions. For human hg38 genome assembly, we recommend hg38.Kundaje.GRCh38_unified_blacklist as the most well-curated and annotated, and sets generated by the Blacklist tool for other organisms. Availability and implementation: https://bioconductor.org/packages/excluderanges/, https://dozmorovlab.github.io/excluderanges/
Copy rights belong to original authors. Visit the link for more info
Podcast created by Paper Player, LLC | |||
23 Mar 2023 | READRetro: Natural Product Biosynthesis Planning with Retrieval-Augmented Dual-View Retrosynthesis | ||
Link to bioRxiv paper:
http://biorxiv.org/cgi/content/short/2023.03.21.533616v1?rss=1
Authors: Lee, S., Kim, T., Choi, M.-S., Kwak, Y., Park, J., Hwang, S. J., Kim, S.-G.
Abstract:
Elucidating the biosynthetic pathways of natural products has been a major focus of biochemistry and pharmacy. However, predicting the whole pathways from target molecules to metabolic building blocks remains a challenge. Here we propose READRetro as a practical bio-retrosynthesis tool for planning the biosynthetic pathways of natural products. READRetro effectively resolves the tradeoff between generalizability and memorability in bio-retrosynthesis by implementing two separate modules; each module is responsible for either generalizability or memorability. Specifically, READRetro utilizes a rule-based retriever for memorability and an ensemble of two dual-representation-based deep learning models for generalizability. Through extensive experiments, READRetro was demonstrated to outperform existing models by a large margin in terms of both generalizability and memorability. READRetro was also capable of predicting the known pathways of complex plant secondary metabolites such as monoterpene indole alkaloids, demonstrating its applicability in the real-world bio-retrosynthesis planning of natural products. A website (https://readretro.net) and open-source code have been provided for READRetro, a practical tool with state-of-the-art performance for natural product biosynthesis research.
Copy rights belong to original authors. Visit the link for more info
Podcast created by Paper Player, LLC | |||
21 Dec 2022 | End-to-end protein-ligand complex structure generation with diffusion-based generative models | ||
Link to bioRxiv paper:
http://biorxiv.org/cgi/content/short/2022.12.20.521309v1?rss=1
Authors: Nakata, S., Mori, Y., Tanaka, S.
Abstract:
Three-dimensional structures of protein-ligand complexes provide valuable insights into their interactions and are crucial for molecular biological studies and drug design. However, their high-dimensional and multimodal nature hinders end-to-end modeling, and earlier approaches depend inherently on existing protein structures. To overcome these limitations and expand the range of complexes that can be accurately modeled, it is necessary to develop efficient end-to-end methods. We introduce an equivariant diffusion-based generative model that learns the joint distribution of ligand and protein conformations conditioned on the molecular graph of a ligand and the sequence representation of a protein extracted from a pre-trained protein language model. Benchmark results show that this protein structure-free model is capable of generating diverse structures of protein-ligand complexes, including those with correct binding poses. Further analyses indicate that the proposed end-to-end approach is particularly effective when the ligand-bound protein structure is not available. The present results demonstrate the effectiveness and generative capability of our end-to-end complex structure modeling framework with diffusion-based generative models. We suppose that this framework will lead to better modeling of protein-ligand complexes, and we expect further improvements and wide applications.
Copy rights belong to original authors. Visit the link for more info
Podcast created by Paper Player, LLC | |||
07 Apr 2023 | euka: Robust detection of eukaryotic taxa from modern and ancient environmental DNA using pangenomic reference graphs. | ||
Link to bioRxiv paper:
http://biorxiv.org/cgi/content/short/2023.04.04.535531v1?rss=1
Authors: Vogel, N. A., Rubin, J. D., Swartz, M., Vlieghe, J., Sackett, P. W., Pedersen, A. G., Pedersen, M. W., Renaud, G.
Abstract:
1. Ancient environmental DNA (eDNA) is a crucial source of information for past environmental reconstruction. However, the computational analysis of ancient eDNA involves not only the inherited challenges of ancient DNA (aDNA) but also the typical difficulties of eDNA samples, such as taxonomic identification and abundance estimation of identified taxonomic groups. Current methods for ancient eDNA fall into those that only perform mapping followed by taxonomic identification and those that purport to do abundance estimation. The former leaves abundance estimates to users, while methods for the latter are not designed for large metagenomic datasets and are often imprecise and challenging to use. 2. Here, we introduce euka, a tool designed for rapid and accurate characterisation of ancient eDNA samples. We use a taxonomy-based pangenome graph of reference genomes for robustly assigning DNA sequences and use a maximum-likelihood framework for abundance estimation. At the present time, our database is restricted to mitochondrial genomes of tetrapods and arthropods but can be expanded in future versions. 3. We find euka to outperform current taxonomic profiling tools as well as their abundance estimates. Crucially, we show that regardless of the filtering threshold set by existing methods, euka demonstrates higher accuracy. Furthermore, our approach is robust to sparse data, which is idiosyncratic of ancient eDNA, detecting a taxon with an average of fifty reads aligning. We also show that euka is consistent with competing tools on empirical samples and about ten times faster than current quantification tools. 4. euka's features are fine-tuned to deal with the challenges of ancient eDNA, making it a simple-to-use, all-in-one tool. It is available on GitHub: https://github.com/grenaud/vgan. euka enables researchers to quickly assess and characterise their sample, thus allowing it to be used as a routine screening tool for ancient eDNA.
Copy rights belong to original authors. Visit the link for more info
Podcast created by Paper Player, LLC | |||
07 Feb 2023 | Heuristics for the De Bruijn Graph Sequence Mapping Problem | ||
Link to bioRxiv paper:
http://biorxiv.org/cgi/content/short/2023.02.05.527069v1?rss=1
Authors: Rocha, L. B., Adi, S. S., Araujo, E.
Abstract:
An important problem in Computational Biology is to map a sequence s into a sequence graph G. One way to do this mapping is to find for a walk (or path) p in G such that p spells a sequence s' most similar to s and this problem is addressed by the Graph Sequence Mapping Problem -- GSMP. In this article we consider the GSMP using De Bruijn graph and we addressed by the De Bruijn Graph Sequence Mapping Problem -- BSMP. Given a sequence s and a De Bruijn graph Gk, with k greater than or equal to 2, BSMP consists of finding a walk p in Gk such that the sequence spelled by p is the most similar to s given a distance, for example, edit distance.
Copy rights belong to original authors. Visit the link for more info
Podcast created by Paper Player, LLC | |||
27 Oct 2022 | Hidden GPCR structural transitions addressed by multiple walker supervised molecular dynamics (mwSuMD) | ||
Link to bioRxiv paper:
http://biorxiv.org/cgi/content/short/2022.10.26.513870v1?rss=1
Authors: Deganutti, G., Pipito, L., Rujan, R. M., Weizmann, T., Griffin, P., Ciancetta, A., Moro, S., Reynolds, C. A.
Abstract:
G protein-coupled receptors (GPCRs) are the most abundant membrane proteins and the target of about 35% of approved drugs. Despite this, the structural basis of GPCR pharmacology is still a matter of intense study. Molecular dynamics (MD) simulations aim at expanding our knowledge of GPCR dynamics by building upon the recent advances in structural biology. However, the timescale limitations of classic MD hinder its applicability to numerous structural processes happening in time scales longer than microseconds (hidden structural transitions). For this reason, the overall MD impact on the study of GPCRs pharmacology and drug design is still limited. To overcome this, we have developed an unbiased adaptive sampling algorithm, namely multiple walker supervised MD (mwSuMD), and tested it on different hidden transitions involving GPCRs. By increasing the complexity of the simulated process, we report the binding and unbinding of the vasopressin peptide, the inactive-to-active transition of the glucagon-like peptide-1 receptor (GLP-1R), the stimulatory G protein (Gs) and inhibitory Gi binding to the adrenoreceptor {beta}2 ({beta}2 AR) and the adenosine 1 receptor (A1R) respectively, and the heterodimerization between the adenosine receptor A2 (A2AR) and the dopamine receptor D2 (D2R). We demonstrate that mwSuMD is a helpful tool for studying at the atomic level GPCR transitions that are challenging to address with classic MD simulations.
Copy rights belong to original authors. Visit the link for more info
Podcast created by Paper Player, LLC | |||
05 May 2023 | JLOH: Inferring Loss of Heterozygosity Blocks from Sequencing Data | ||
Link to bioRxiv paper:
http://biorxiv.org/cgi/content/short/2023.05.04.539368v1?rss=1
Authors: Schiavinato, M., del Olmo, V., Muya, V. N., Gabaldon, T.
Abstract:
Heterozygosity is a genetic condition in which two or more alleles are found at a genomic locus. Among the organisms that are more prone to heterozygosity are hybrids, i.e. organisms that are the offspring of genetically divergent yet still interfertile individuals. One of the most studied aspects is the loss of heterozygosity (LOH) within genomes, where multi-allelic sites lose one of their two alleles by converting it to the other, or by remaining hemizygous at that site. LOH is deeply interconnected with adaptation, especially in hybrids, but the in silico techniques to infer LOH blocks are hardly standardized, and a general tool to infer and analyse them in most genomic contexts and species is missing. Here, we present JLOH, a computational toolkit for the inference and exploration of LOH blocks which only requires commonly available genomic data as input. Starting from mapped reads, called variants and a reference genome sequence, JLOH infers candidate LOH blocks based on single-nucleotide polymorphism density (SNPs/kbp) and read coverage per position. If working with a hybrid organism of known parentals, JLOH is also able to assign each LOH block to its subgenome of origin.
Copy rights belong to original authors. Visit the link for more info
Podcast created by Paper Player, LLC | |||
03 Jul 2023 | PanTA: An ultra-fast method for constructing large and growing microbial pangenomes | ||
Link to bioRxiv paper:
http://biorxiv.org/cgi/content/short/2023.07.03.547471v1?rss=1
Authors: Le, D. Q., Nguyen, T. A., Nguyen, T. T., Do, V. H., Nguyen, C. H., Phung, H. T., Ho, T. H., Vo, N. S., Nguyen, T., Nguyen, H. A., Cao, M. D.
Abstract:
Pangenome analysis has become indispensable in bacterial genomics due to the high variability of gene content between isolates within a clade. While many computational methods exist for constructing the pangenome from a bacterial genome collection, speed and scalability still remain an issue for the fast-growing genomic collections. Here, we present PanTA, a efficient method to build and analyze pangenomes of bacteria strains. We show that PanTA exhibits an unprecedented 10 times speed up and 2 times more memory efficient over the current state of the art methods. More importantly, PanTA enables the progressive pangenome construction where new samples are added into an existing pangenome without the need of rebuilding the accumulated collection from the scratch. The progressive building of pangenomes can further reduce the memory requirements by half. We demonstrate that PanTA can build the pangenome of the Escherichia coli species from the entire collection of over 28000 high quality genomes collected from the RefSeq database. Crucially, the whole analysis is performed on a modest laptop computer within two days, highlighting the scalability and practicality of PanTA.
Copy rights belong to original authors. Visit the link for more info
Podcast created by Paper Player, LLC | |||
26 Jun 2023 | Fusion Neural Network (FusNet) for predicting protein-mediated loops | ||
Link to bioRxiv paper:
http://biorxiv.org/cgi/content/short/2023.06.24.546360v1?rss=1
Authors: Tang, L., Huang, W., Hill, M. C., Ellinor, P. T., Li, M.
Abstract:
The organization of the three-dimensional (3D) genome is a complex, and requires a plethora of proteins to ensure the proper formation and regulation of chromatin loops as well as higher order structures. Studying protein-mediated loop regulation can help unravel the intricate interplay between these loops and their crucial roles in modulating gene expression across different cellular contexts. However, current targeted chromatin conformation capture experiments face limitations in capturing protein-mediated loops across various cell types, and existing computational methods fail to predict diverse protein-mediated loops. To address these issues, we propose a fusion neural network (FusNet) designed for predicting protein-mediated loops. FusNet leverages genome sequence information, open chromatin, and ChIP-seq data to efficiently represent and analyze the positions of loop anchors. To extract informative features and reduce the complexity of FusNet , we constructed a convolutional neural network, which compresses the dimensionality of the features while also preserving the most significant ones. To enhance the accuracy and generalization capacity of FusNet, we built a fusion layer by stacking the prediction of fundamental models with a meta-model. FusNet demonstrated its effectiveness in predicting protein-mediated loops, exhibiting high consistency with Hi-C data. Moreover, we find that the loops output from FusNet are highly associated with regulatory functions. Through association analysis with genetic risk variants, FusNet further revealed its potential for unraveling disease-related mechanisms. In conclusion, our study offers a novel computational approach for predicting various protein-mediated chromatin loops, which could substantially enhance research on the functional significance of protein-mediated loop structures in diverse cellular contexts.
Copy rights belong to original authors. Visit the link for more info
Podcast created by Paper Player, LLC | |||
14 Nov 2022 | Highly Realistic Whole Transcriptome Synthesis through Generative Adversarial Networks | ||
Link to bioRxiv paper:
http://biorxiv.org/cgi/content/short/2022.11.10.515980v1?rss=1
Authors: Fu, S.
Abstract:
The transcriptome is the most extensive and standardized among all biological data, but its lack of inherent structure impedes the application of deep learning tools. This study resolves the neighborhood relationship of protein-coding genes through uniform manifold approximation and projection (UMAP) of high-quality gene expression data. The resultant transcriptome image is conducive to classification tasks and generative learning. Convolutional neural networks (CNNs) trained with full or partial transcriptome images differentiate normal versus lung squamous cell carcinoma (LUSC) and LUSC versus lung adenocarcinoma (LUAD) with over 96% accuracy, comparable to XGBoost. Meanwhile, the generative adversarial network (GAN) model trained with 93 TcgaTargetGtex transcriptome classes synthesizes highly realistic and diverse tissue/cancer-specific transcriptome images. Comparative analysis of GAN-synthesized LUSC and LUAD transcriptome images show selective retention and enhancement of epithelial identity gene expression in the LUSC transcriptome. Further analyses of synthetic LUSC transcriptomes identify a novel role for mitochondria electron transport complex I expression in LUSC stratification and prognosis. In summary, this study provides an intuitive transcriptome embedding compatible with generative deep learning and realistic transcriptome synthesis.
Copy rights belong to original authors. Visit the link for more info
Podcast created by Paper Player, LLC | |||
30 Apr 2023 | Semantic Representation of Neural Circuit Knowledge in Caenorhabditis elegans | ||
Link to bioRxiv paper:
http://biorxiv.org/cgi/content/short/2023.04.28.538760v1?rss=1
Authors: Prakash, S. J., Van Auken, K., Hill, D. P., Sternberg, P. W.
Abstract:
Copy rights belong to original authors. Visit the link for more info
Podcast created by Paper Player, LLC | |||
31 Jan 2023 | Charge-State-Dependent Collision-Induced Dissociation Behaviors of RNA Oligonucleotides via High-Resolution Mass Spectrometry | ||
Link to bioRxiv paper:
http://biorxiv.org/cgi/content/short/2023.01.29.526146v1?rss=1
Authors: Sun, R., Zuo, M.-Q., Zhang, J.-S., Dong, M.-Q.
Abstract:
Mass spectrometry (MS)-based analysis of RNA oligonucleotides (oligos) plays an increasingly important role in the development of RNA therapeutics and in epitranscriptomic studies. However, MS fragmentation behaviors of RNA oligos are understood insufficiently. In this study, we characterized the negative-ion-mode fragmentation behaviors of 26 synthetic RNA oligos of four to eight nucleotides (nt) in length by collision-induced dissociation (CID) using a high-resolution, accurate-mass instrument. We find that in the CID spectra acquired under the normalized collisional energy of 35%, ~70% of the total peak intensity belonged to sequencing ions (a-B, a, b, c, d, w, x, y, z), ~25% belonged to precursor ions with either complete or partial loss of a nucleobase in the form of a neutral or an anion, and the remainder were internal ions and anionic nucleobases. Of the sequencing ions, the most abundant species were y, c, w, a-B, and a ions. The charge state of the RNA precursor ions strongly affected their fragmentation behaviors. As the precursor charge increased from -1 to -5, the fractional intensity of sequencing ions in the CID spectra decreased, whereas the fractional intensity of precursor ions with neutral and/or charged losses of a nucleobase increased. Moreover, RNA oligos containing U, especially at the 3' terminus, tended to produce precursors that lost HNCO and/or NCO-, which presumably corresponded to isocyanic acid and cyanate anion, respectively. These findings build a strong foundation for mechanistic understanding of RNA fragmentation by MS/MS, contributing to future automated identification of RNA oligos from their CID spectra in a more efficient way.
Copy rights belong to original authors. Visit the link for more info
Podcast created by Paper Player, LLC | |||
21 Jul 2023 | Hidden Markov Models based search in combination with structural bioinformatics pipeline leads to the identification of DAF-12 distant orthologous in Meloidogyne incognita | ||
Link to bioRxiv paper:
http://biorxiv.org/cgi/content/short/2023.07.19.549723v1?rss=1
Authors: Schuster, C. D., Zabala, V. J. S., San Juan, R. B., Sosa, E. J., Rodriguez, C., Kronberg, M. F., Munarriz, E. R., Burton, G., Castro, O. A., Modenutti, C. P.
Abstract:
Root-knot nematode (RKN) Meloidogyne spp. is one of the most damaging parasites due to its wide range of hosts. Here, we report a C. elegans receptor DAF-12 ortholog gene in Meloidogyne incognita (DAF-12Minc), a promising molecular target to modify the RKN life cycle. Using a combination of Hidden Markov Models (HMM) based sequence search and phylogenetic analysis we identified three DAF-12Minc genes. Although the global sequence identity between previously reported DAF-12 genes and DAF-12Minc was acceptable, the correlation between binding site residues was low in the multiple sequence alignment (MSA). Since those residues are critical for DAF-12 interaction with its ligand, the dafachronic acids (DAs), and thus its biological role, we investigated whether even if the sequence conservation is low, the active site structure was conserved and thus able to bind DAs. For this purpose, we built accurate homology models of DAF-12Minc and used them to identify and characterize the ligand binding site (LBS) and its molecular interactions with DAs-like compounds. Finally, we cloned, expressed, and evaluated the biological role of DAF-12Minc in vitro and in vivo using a DAF-12 antagonist. These in vivo results suggest that our strategy was effective to find orthologous genes among species even when sequence similarity is low.
Copy rights belong to original authors. Visit the link for more info
Podcast created by Paper Player, LLC | |||
04 Feb 2023 | Systematic Analysis of KRAS-ligand Interaction Modes and Flexibilities Reveals the Binding Characteristics | ||
Link to bioRxiv paper:
http://biorxiv.org/cgi/content/short/2023.02.01.526731v1?rss=1
Authors: Zhao, Z., Bohidar, N., Bourne, P. E.
Abstract:
KRAS, a common human oncogene, has been recognized as a critical drug target in treating multiple cancers. After four decades of effort, one allosteric KRAS drug (Sotorasib) has been approved, inspiring more KRAS-targeted drug research. Here we provide the features of KRAS binding pockets and ligand-binding characteristics of KRAS complexes using a structural systems pharmacology approach. Three distinct binding sites (conserved nucleotide-binding site, shallow Switch-I/II pocket, and allosteric Switch-II/3 pocket) are characterized. Ligand-binding features are determined based on encoded KRAS-inhibitor interaction fingerprints. Finally, the flexibility of the three distinct binding sites to accommodate different potential ligands, based on MD simulation, is discussed. Collectively, these findings are intended to facilitate rational KRAS drug design.
Copy rights belong to original authors. Visit the link for more info
Podcast created by Paper Player, LLC | |||
24 Oct 2022 | ViReaDB: A user-friendly database for compactly storing viral sequence data and rapidly computing consensus genome sequences | ||
Link to bioRxiv paper:
http://biorxiv.org/cgi/content/short/2022.10.21.513318v1?rss=1
Authors: Moshiri, N.
Abstract:
Motivation: In viral molecular epidemiology, reconstruction of consensus genomes from sequence data is critical for tracking mutations and variants of concern. However, storage of the raw sequence data can become prohibitively large, and computing consensus genome from sequence data can be slow and requires bioinformatics expertise. Results: ViReaDB is a user-friendly database system for compactly storing viral sequence data and rapidly computing consensus genome sequences. From a dataset of 1 million trimmed mapped SARS-CoV-2 reads, it is able to compute the base counts and the consensus genome in 16 minutes, store the reads alongside the base counts and consensus in 50 MB, and optionally store just the base counts and consensus (without the reads) in 300 KB. Availability: ViReaDB is freely available on PyPI (https://pypi.org/project/vireadb) and on GitHub (https://github.com/niemasd/ViReaDB) as an open-source Python software project.
Copy rights belong to original authors. Visit the link for more info
Podcast created by Paper Player, LLC | |||
12 Dec 2022 | Foldcomp: a library and format for compressing and indexing large protein structure sets | ||
Link to bioRxiv paper:
http://biorxiv.org/cgi/content/short/2022.12.09.519715v1?rss=1
Authors: Kim, H., Mirdita, M., Steinegger, M.
Abstract:
Summary: Highly accurate protein structure predictors have generated hundreds of millions of protein structures; these pose a challenge in terms of storage and processing. Here we present Foldcomp, a novel lossy structure compression algorithm and indexing system to address this challenge. By using a combination of internal and cartesian coordinates and a bi-directional NeRF-based strategy, Foldcomp improves the compression ratio by a factor of 3 compared to the next best method. Its reconstruction error of 0.08 angstrom is comparable to the best lossy compressor. It is 5 times faster than the next fastest compressor and competes with the fastest decompressors. With its multi-threading implementation and a Python interface that allows for easy database downloads and efficient querying of protein structures by accession, Foldcomp is a powerful tool for managing and analyzing large collections of protein structures. Availability: Foldcomp is a free open-source library and command-line software available for Linux, macOS and Windows at https://foldcomp.foldseek.com. Foldcomp provides the AlphaFold Swiss-Prot (2.9GB), TrEMBL (1.1TB) and ESMatlas HQ (114GB) database ready-for-download.
Copy rights belong to original authors. Visit the link for more info
Podcast created by Paper Player, LLC | |||
03 May 2023 | Scalable Log-ratio Lasso Regression Enhances Microbiome Feature Selection for Predictive Models | ||
Link to bioRxiv paper:
http://biorxiv.org/cgi/content/short/2023.05.02.538599v1?rss=1
Authors: Fei, T., Funnell, T., Waters, N., Raj, S. S., Devlin, S. M., Dai, A., Miltiadous, O., Shouval, R., Lv, M., Peled, J. U., Ponce, D. M., Perales, M.-A., Gönen, M., van den Brink, M. R. M.
Abstract:
Copy rights belong to original authors. Visit the link for more info
Podcast created by Paper Player, LLC | |||
14 Nov 2022 | VarSCAT: A computational tool for sequence context annotations of genomic variants | ||
Link to bioRxiv paper:
http://biorxiv.org/cgi/content/short/2022.11.11.516085v1?rss=1
Authors: Wang, N., Khan, S., Elo, L.
Abstract:
The sequence contexts of genomic variants play important roles in understanding biological significances of variants and potential sequencing related variant calling issues. However, methods for assessing the diverse sequence contexts of genomic variants such as tandem repeats and unambiguous annotations have been limited. Herein, we describe the Variant Sequence Context Annotation Tool (VarSCAT) for annotating the sequence contexts of genomic variants, including breakpoint ambiguities, flanking sequences, variant nomenclatures, adjacent variants, and tandem repeats with user customizable options. Our analysis demonstrate that VarSCAT is more versatile and customizable than current methods or strategies for annotating variants in short tandem repeat (STR) regions. Variant sequence context annotations of high-confidence human variant sets with VarSCAT revealed that more than 75% of all human individual germline and clinically relevant insertions and deletions (indels) have breakpoint ambiguities. Moreover, we illustrate that more than 80% of human individual germline small variants in STR regions are indels and that the sizes of these indels correlated with STR motif sizes. VarSCAT is available at https://github.com/elolab/VarSCAT.
Copy rights belong to original authors. Visit the link for more info
Podcast created by Paper Player, LLC | |||
21 Nov 2022 | SARS-CoV-2 variant transition dynamics are associated with vaccination rates, number of co-circulating variants, and natural immunity | ||
Link to bioRxiv paper:
http://biorxiv.org/cgi/content/short/2022.11.18.517139v1?rss=1
Authors: Beesley, L. J., Moran, K. R., Wagh, K., Castro, L., Theiler, J., Yoon, H., Fischer, W., Hengartner, N. W., Korber, B., Del Valle, S.
Abstract:
Background: Throughout the COVID-19 pandemic, the SARS-CoV-2 virus has continued to evolve, with new variants outcompeting existing variants and often leading to different dynamics of disease spread. Methods: In this paper, we performed a retrospective analysis using longitudinal sequencing data to characterize differences in the speed, calendar timing, and magnitude of 13 SARS-CoV-2 variant waves/transitions for 215 countries and sub-country regions, between October 2020 and October 2022. We then clustered geographic locations in terms of their variant behavior across all Omicron variants, allowing us to identify groups of locations exhibiting similar variant transitions. Finally, we explored relationships between heterogeneity in these variant waves and time-varying factors, including vaccination status of the population, governmental policy, and the number of variants in simultaneous competition. Findings: This work demonstrates associations between the behavior of an emerging variant and the number of co-circulating variants as well as the demographic context of the population. We also observed an association between high vaccination rates and variant transition dynamics prior to the Mu and Delta variant transitions. Interpretation: These results suggest the behavior of an emergent variant may be sensitive to the immunologic and demographic context of its location. Additionally, this work represents the most comprehensive characterization of variant transitions globally to date. Funding: Laboratory Directed Research and Development (LDRD), Los Alamos National Laboratory
Copy rights belong to original authors. Visit the link for more info
Podcast created by Paper Player, LLC | |||
25 Nov 2022 | Evaluating spatially variable gene detection methods for spatial transcriptomics data | ||
Link to bioRxiv paper:
http://biorxiv.org/cgi/content/short/2022.11.23.517747v1?rss=1
Authors: Chen, C., Kim, J. H., Yang, P.
Abstract:
The identification of genes that vary across spatial domains in tissues and cells is an essential step for spatial transcriptomics data analysis. Given the critical role it serves for downstream data interpretations, various methods for detecting spatially variable genes (SVGs) have been proposed. The availability of multiple methods for detecting SVGs bears questions such as whether different methods select a similar set of SVGs, how reliable is the reported statistics significance from each method, how accurate and robust is each method in terms of SVG detection, and how well the selected SVGs perform in downstream applications such as clustering of spatial domains. Besides these, practical considerations such as computational time and memory usage are also crucial for deciding which method to use. In this study, we address the above questions by systematically evaluating a panel of popular SVG detection methods on a large collection of spatial transcriptomics datasets, covering various tissue types, biotechnologies, and spatial resolutions. Our results shed light on the performance of each method from multiple aspects and highlight the discrepancy among different methods especially on calling statistically significant SVGs across datasets. Taken together, our work provides useful considerations for choosing methods for identifying SVGs and serves as a key reference for the future development of such methods.
Copy rights belong to original authors. Visit the link for more info
Podcast created by Paper Player, LLC | |||
23 Feb 2023 | Ensemble deep learning of embeddings for clustering multimodal single-cell omics data | ||
Link to bioRxiv paper:
http://biorxiv.org/cgi/content/short/2023.02.22.529627v1?rss=1
Authors: Yu, L., Liu, C., Yang, J. Y. H., Yang, P.
Abstract:
Motivation: Recent advances in multimodal single-cell omics technologies enable multiple modalities of molecular attributes, such as gene expression, chromatin accessibility, and protein abundance, to be profiled simultaneously at a global level in individual cells. While the increasing availability of multiple data modalities is expected to provide a more accurate clustering and characterisation of cells, the development of computational methods that are capable of extracting information embedded across data modalities is still in its infancy. Results: We propose SnapCCESS for clustering cells by integrating data modalities in multimodal single-cell omics data using an unsupervised ensemble deep learning framework. By creating snapshots of embeddings of multimodality using variational autoencoders, SnapCCESS can be coupled with various clustering algorithms for generating consensus clustering of cells. We applied SnapCCESS with several clustering algorithms to various datasets generated from popular multimodal single-cell omics technologies. Our results demonstrate that SnapCCESS is effective and more efficient than conventional ensemble deep learning-based clustering methods and outperforms other state-of-the-art multimodal embedding generation methods in integrating data modalities for clustering cells. The improved clustering of cells from SnapCCESS will pave the way for more accurate characterisation of cell identity and types, an essential step for various downstream analyses of multimodal single-cell omics data. Availability and implementation: SnapCCESS is implemented as a Python package and is freely available from https://github.com/yulijia/SnapCCESS.
Copy rights belong to original authors. Visit the link for more info
Podcast created by Paper Player, LLC | |||
08 Mar 2023 | Prediction of protein assemblies by structure sampling followed by interface-focused scoring | ||
Link to bioRxiv paper:
http://biorxiv.org/cgi/content/short/2023.03.07.531468v1?rss=1
Authors: Olechnovic, K., Valancauskas, L., Dapkunas, J., Venclovas, C.
Abstract:
Proteins often function as part of permanent or transient multimeric complexes, and understanding function of these assemblies requires knowledge of their three-dimensional structures. While the ability of AlphaFold to predict structures of individual proteins with unprecedented accuracy has revolutionized structural biology, modeling structures of protein assemblies remains challenging. To address this challenge, we developed a protocol for predicting structures of protein complexes involving model sampling followed by scoring focused on the subunit-subunit interaction interface. In this protocol, we diversified AlphaFold models by varying construction and pairing of multiple sequence alignments as well as increasing the number of recycles. In cases when AlphaFold failed to assemble a full protein complex or produced unreliable results, additional diverse models were constructed by docking of monomers or subcomplexes. All the models were then scored using a newly developed method, VoroIF-jury, which relies only on structural information. Notably, VoroIF-jury is independent of AlphaFold self-assessment scores and therefore can be used to rank models originating from different structure prediction methods. We tested our protocol in CASP15 and obtained top results, significantly outperforming the standard AlphaFold-Multimer pipeline. Analysis of our results showed that the accuracy of our assembly models was capped mainly by structure sampling rather than model scoring. This observation suggests that better sampling, especially for the antibody-antigen complexes, may lead to further improvement. Our protocol is expected to be useful for modeling and/or scoring protein assemblies.
Copy rights belong to original authors. Visit the link for more info
Podcast created by Paper Player, LLC | |||
04 Aug 2023 | Single-linkage molecular clustering of viral pathogens | ||
Link to bioRxiv paper:
http://biorxiv.org/cgi/content/short/2023.08.03.551813v1?rss=1
Authors: Soto Miranda, M., Narvaez Romo, R., Moshiri, N.
Abstract:
Introduction: Public health faces the ongoing mission of safeguarding the population's health against various infectious diseases caused by a great number of pathogens. Epidemiology is an essential discipline in this field. With the rise of more advanced technologies, new tools are emerging to enhance the capability to intervene and control an epidemic. Among these approaches, molecular clustering comes forth as a promising option. However, appropriate genetic distance thresholds for defining clusters are poorly explored in contexts outside of Human Immunodeficiency Virus-1 (HIV-1). Methods: In this work, using the well-used pairwise Tamura-Nei 93 (TN93) distance threshold of 0.015 for HIV-1 as a point of reference for molecular cluster properties of interest, we perform molecular clustering on whole genome sequence datasets from HIV-1, Severe Acute Respiratory Syndrome Coronavirus 2 (SARS-CoV-2), Zaire ebolavirus, and Mpox virus, to explore potential pairwise distances thresholds for these other viruses. Results: We found the following pairwise TN93 distance thresholds as potential candidates for use in molecular clustering: 0.00014 (4 mutations) for SARS-CoV-2, 0.00016 (3 mutations) for Ebola, and 0.0000051 (1 mutation) for Mpox. Conclusion: This study provides valuable information for epidemic control strategies, and public health efforts in managing infectious diseases caused by these viruses. The identified pairwise distance thresholds for molecular clustering can serve as a foundation for future research and intervention to combat epidemics effectively.
Copy rights belong to original authors. Visit the link for more info
Podcast created by Paper Player, LLC | |||
24 Mar 2023 | Estimation of a treatment effect based on a modified covariates method with L0 norm | ||
Link to bioRxiv paper:
http://biorxiv.org/cgi/content/short/2023.03.22.533735v1?rss=1
Authors: Tanioka, K., Okuda, K., Hiwa, S., Hiroyasu, T.
Abstract:
In randomized clinical trials, we assumed the situation that the new treatment is not adequate compared to the control treatment as result. However, it is unknown if the new treatment is ineffective for all patients or if it is effective for only a subgroup of patients with specific characteristics. If such a subgroup exists and can be detected, the patients can receive effective therapy. To detect subgroups, we need to estimate treatment effects. To achieve this, various treatment effect estimation methods have been proposed based on the sparse regression method. However, these methods are affected by noise. Therefore, we propose new treatment effect estimation approaches based on the modified covariate method, one using lasso regression and the other ridge regression, using the L0 norm. The proposed approach was evaluated through numerical simulation and real data examples.
Copy rights belong to original authors. Visit the link for more info
Podcast created by Paper Player, LLC | |||
02 Dec 2022 | Improving Protein Subcellular Localization Prediction with Structural Prediction & Graph Neural Networks | ||
Link to bioRxiv paper:
http://biorxiv.org/cgi/content/short/2022.11.29.518403v1?rss=1
Authors: Dubourg-Felonneau, G., Abbasi, A., Akiva, E., Lee, L.
Abstract:
The majority of biological functions are carried out by proteins. Proteins perform their roles only upon arrival to their target location in the cell, hence elucidating protein subcellular localization is essential for better understanding their function. The exponential growth in genomic information and the high cost of experimental validation of protein localization call for the development of predictive methods. We present a method that improves subcellular localization prediction for proteins based on their sequence by leveraging structure prediction and Graph Neural Networks. We demonstrate how Language Models, trained on protein sequences, and Graph Neural Networks, trained on proteins 3D structures, are both efficient approaches for this task. They both learn meaningful, yet different representations of proteins; hence, ensembling them outperforms the reigning state of the art method. Our architecture improves the localization prediction performance while being lighter and more cost-effective.
Copy rights belong to original authors. Visit the link for more info
Podcast created by Paper Player, LLC | |||
02 Nov 2022 | HISS: Snakemake-based workflows for performing SMRT-RenSeq assembly, AgRenSeq and dRenSeq for the discovery of novel plant disease resistance genes. | ||
Link to bioRxiv paper:
http://biorxiv.org/cgi/content/short/2022.11.01.514708v1?rss=1
Authors: Adams, T. M., Smith, M., Bayer, M. M., Hein, I.
Abstract:
Background In the nine years since the initial publication of the RenSeq protocol, the method has proved to be a powerful tool for studying disease resistance in plants and providing target genes for breeding programmes. Since the initial publication of the methodology, it has continued to be developed as new technologies have become available and the increased availability of computing power has made new bioinformatic approaches possible. Most recently, this has included the development of a k-mer based association genetics approach, the use of PacBio HiFi data and graphical genotyping with diagnostic RenSeq. However, there is not yet a unified workflow available and researchers must instead configure approaches from various sources themselves. This makes reproducibility and version control a challenge and limits the ability to perform these analyses to those with bioinformatics expertise. Results Here we present HISS, consisting of three workflows which take a user from raw RenSeq reads to the identification of candidates for disease resistance genes. These workflows conduct the assembly of enriched HiFi reads from an accession with the resistance phenotype of interest. A panel of accessions both possessing and lacking the resistance are then used in an association genetics approach (AgRenSeq) to identify contigs positively associated with the resistance phenotype. Candidate genes are then identified on these contigs and assessed for their presence or absence in the panel with a graphical genotyping approach that used dRenSeq. These workflows are implemented via Snakemake, a python-based workflow manager. Software dependencies are either shipped with the release or handled with conda. All code is freely available and is distributed under the GNU GPL-3.0 license. Conclusions HISS provide a user-friendly, portable, and easily customised approach for identifying novel disease resistance genes in plants. It is easily installed with all dependencies handled internally or shipped with the release and represents a significant improvement in the ease of use of these bioinformatics analyses.
Copy rights belong to original authors. Visit the link for more info
Podcast created by Paper Player, LLC | |||
30 Dec 2022 | Residue communities reveal evolutionary signatures of γδ T-Cell receptor | ||
Link to bioRxiv paper:
http://biorxiv.org/cgi/content/short/2022.12.29.522230v1?rss=1
Authors: Cheung, N. J., Huang, S.-Y.
Abstract:
Naturally co-occurring amino acids, term coevolution, in a protein family play a significant role in both protein engineering and folding, and it is expanding in recent years from the studies of the effects of single-site mutations to the complete re-design of a protein and its folding, especially in three-dimensional structure prediction. Here, to better characterize such coevolving interactions, we in silico decipher evolutionary couplings from massive homologous sequences using spectral analysis to capture signatures that are important for specific molecular interactions and binding activities. We implement the present approach on the G7 gamma delta T-cell receptor to identify functionally important residues that contribute to its highly distinct binding mode. The analysis indicates the evolutionary signatures (highly ordered networks of coupled amino acids, termed residue communities) of the protein confirm previously identified functional sites that are relevant to dock the receptor underneath the major histocompatibility complex class I-related protein-1 (MR1) antigen presenting groove. Moreover, we analyze the correlation of inter-residue contacts with the activation states of receptors and show that contact patterns closely correlating with activation indeed coincide with these sites. The theoretical results demonstrate our method provides an alternative path towards bridging protein sequence with its function at residue-level without requiring its tertiary structure or highly accurate measurement of its biological activities in vivo/vitro.
Copy rights belong to original authors. Visit the link for more info
Podcast created by Paper Player, LLC | |||
07 Jul 2023 | Pan-cancer analysis reveals embryonic and hematopoietic stem cell signatures to distinguish different cancer subtypes | ||
Link to bioRxiv paper:
http://biorxiv.org/cgi/content/short/2023.07.05.547742v1?rss=1
Authors: Lei, J., Luo, J., Liu, Q., Wang, X.
Abstract:
Purpose: Stem cells-like properties in cancer cells may confer cancer development and therapy resistance. With the advancement of multi-omics technology, the multi-omics-based exploration of cancer stemness has attracted certain interests. However, subtyping of cancer based on the combination of different types of stem cell signatures remains scarce. Methods: In this study, 10,323 cancer specimens from 33 TCGA cancer types were clustered based on the enrichment scores of six stemness gene sets, representing two types of stem cell backgrounds: embryonic stem cells (ESCs) and hematopoietic stem cells (HSCs). Results: We identified four subtypes of pan-cancer, termed StC1, StC2, StC3 and StC4, which displayed distinct molecular and clinical features, including stemness, genome integrity, intratumor heterogeneity, methylation levels, tumor microenvironment, tumor progression, chemotherapy and immunotherapy responses, and survival prognosis. This subtyping method for pan-cancer is reproducible at the protein level. Conclusion: Our findings indicate that the ESC signature is an adverse prognostic factor, while the HSC signature and ratio of HSC/ESC signatures are positive prognostic factors in cancer. The ESC and HSC signatures-based subtyping of cancer may provide insights into cancer biology and clinical implications of cancer.
Copy rights belong to original authors. Visit the link for more info
Podcast created by Paper Player, LLC | |||
11 Apr 2023 | Deep Learning and Transfer Learning for Brain Tumor Detection and Classification | ||
Link to bioRxiv paper:
http://biorxiv.org/cgi/content/short/2023.04.10.536226v1?rss=1
Authors: Rustom, F., Parva, P., Ogmen, H., Yazdanbakhsh, A.
Abstract:
Convolutional neural networks (CNNs) are powerful tools that can be trained on image classification tasks and share many structural and functional similarities with biological visual systems and mechanisms of learning. In addition to serving as a model of biological systems, CNNs possess the convenient feature of transfer learning where a network trained on one task may be repurposed for training on another, potentially unrelated, task. In this retrospective study of public domain MRI data, we investigate the ability of neural networks to be trained on brain cancer imaging data while introducing a unique camouflage animal detection transfer learning step as a means of enhancing the network tumor detection ability. Training on glioma, meningioma, and healthy brain MRI data, both T1- and T2-weighted, we demonstrate the potential success of this training strategy for improving neural network classification accuracy both quantitatively with accuracy metrics and qualitatively with feature space analysis of the internal states of trained networks. In addition to animal transfer learning, similar improvements were noted as a result of transfer learning between MRI sequences, specifically from T1 to T2 data. Image sensitivity functions further this investigation by allowing us to visualize the most salient image regions from a network perspective while learning. Such methods demonstrate that the networks not only look at the tumor itself when deciding, but also at the impact on the surrounding tissue in terms of compressions and midline shifts. These results suggest an approach to brain tumor MRIs that is comparatively similar to that of trained radiologists while also exhibiting a high sensitivity to subtle structural changes resulting from the presence of a tumor.
Copy rights belong to original authors. Visit the link for more info
Podcast created by Paper Player, LLC | |||
25 Jan 2023 | {Theta}-Net: Achieving Enhanced Phase-Modulated Optical Nanoscopy in silico through a computational 'string of beads' architecture | ||
Link to bioRxiv paper:
http://biorxiv.org/cgi/content/short/2023.01.24.525271v1?rss=1
Authors: Kaderuppan, S. S., Wong, E. W. L., Sharma, A., Woo, W. L.
Abstract:
We present herein a triplet string of concatenated O-Net ( bead) architectures (formulated as discussed in our previous study) which we term {Theta}-Net as a means of improving the viability of generated super-resolved (SR) images in silico. In the present study, we assess the quality of the afore-mentioned SR images with that obtained via other popular frameworks (such as ANNA-PALM, BSRGAN and 3D RCAN). Models developed from our proposed framework result in images which more closely approach the gold standard of the SEM-verified test sample as a means of resolution enhancement for optical microscopical imaging, unlike previous DNNs. In addition, cross-domain (transfer) learning was also utilized to enhance the capabilities of models trained on DIC datasets, where phasic variations are not as prominently manifested as amplitude/intensity differences in the individual pixels [unlike phase contrast microscopy (PCM)]. The present study thus demonstrates the viability of our current multi-paradigm architecture in attaining ultra-resolved images under poor signal-to-noise ratios, while eliminating the need for a priori PSF & OTF information. Due to the wide-scale use of optical microscopy for inspection & quality analysis in various industry sectors, the findings of this study would be anticipated to exhibit a far-ranging impact on several engineering fronts.
Copy rights belong to original authors. Visit the link for more info
Podcast created by Paper Player, LLC | |||
06 Apr 2023 | GENI: a web server to identify gene set enrichments in tumor samples | ||
Link to bioRxiv paper:
http://biorxiv.org/cgi/content/short/2023.04.05.535584v1?rss=1
Authors: Hayashi, A., Ruppo, S., Heilbrun, E. E., Mazzoni, C., Adar, S., Yassour, M., Abu Rmaileh, A., Shaul, Y. D.
Abstract:
The Cancer Genome Atlas (TCGA) and other projects provide informative tumor-associated genomic data for the broad research community. Hence, several useful web-based tools have been generated to ease non-expert users with the analysis and characterization of a specific gene behavior in selected tumors. However, none of the existing tools offer the user the means to evaluate the expression profile of a given gene in the context of the whole transcriptome. Currently, such analyses require prior bioinformatic knowledge and expertise. Therefore, we developed GENI (Gene ENrichment Identifier) as a fast, user-friendly tool to analyze the TCGA expression data for gene set enrichments. GENI analyzes large-scale tumor-associated gene expression datasets and evaluates biological relevance, thus offering researchers a simplified means to analyze cancer patient-derived data.
Copy rights belong to original authors. Visit the link for more info
Podcast created by Paper Player, LLC | |||
19 Apr 2023 | DeepCORE: An interpretable multi-view deep neural network model to detect co-operative regulatory elements | ||
Link to bioRxiv paper:
http://biorxiv.org/cgi/content/short/2023.04.19.536807v1?rss=1
Authors: Chandrashekar, P. B., Chen, H., Lee, M., Ahmadinajed, N., Liu, L.
Abstract:
Gene transcription is an essential process involved in all aspects of cellular functions with significant impact on biological traits and diseases. This process is tightly regulated by multiple elements that co-operate to jointly modulate the transcription levels of target genes. To decipher the complicated regulatory network, we present a novel multi-view attention-based deep neural network that models the relationship between genetic, epigenetic, and transcriptional patterns and identifies co-operative regulatory elements (COREs). We applied this new method, named DeepCORE, to predict transcriptomes in 25 different cell lines, which outperformed the state-of-the-art algorithms. Furthermore, DeepCORE translates the attention values embedded in the neural network into interpretable information, including locations of putative regulatory elements and their correlations, which collectively implies COREs. These COREs are significantly enriched with known promoters and enhancers. Novel regulatory elements discovered by DeepCORE showed epigenetic signatures consistent with the status of histone modification marks.
Copy rights belong to original authors. Visit the link for more info
Podcast created by Paper Player, LLC | |||
27 Oct 2022 | IntestLine: a Shiny-based application to map the rolled intestinal tissue onto a line | ||
Link to bioRxiv paper:
http://biorxiv.org/cgi/content/short/2022.10.26.513827v1?rss=1
Authors: Yuzeir, A., Bejaran, D., Grein, S., Hasenauer, J., Schlitzer, A., Yu, J.
Abstract:
To allow the comprehensive histological analysis of the whole intestine in one image, the tissue is often rolled to a spiral before imaging. This Swiss-rolling technique facilitates robust experimental procedures, but it limits the possibilities to comprehend changes along the intestine. Here, we present IntestLine, a Shiny-based open-source application to map imaging data of intestinal tissues in spiral shape onto a line. The mapping of intestinal tissues improves the visualization of the whole intestine in both proximal-distal and serosa-luminal axis, and facilitates the observation of location-specific cell types and markers. In summary, IntestLine serves as a tool to visualize and characterize intestine in future imaging studies.
Copy rights belong to original authors. Visit the link for more info
Podcast created by Paper Player, LLC | |||
04 Apr 2023 | MANGEM - a web app for Multimodal Analysis of Neuronal Gene expression, Electrophysiology and Morphology | ||
Link to bioRxiv paper:
http://biorxiv.org/cgi/content/short/2023.04.03.535322v1?rss=1
Authors: Olson, R. H., Kalafut, N. C., Wang, D.
Abstract:
Single-cell techniques have enabled the acquisition of multi-modal data, particularly for neurons, to characterize cellular functions. Patch-seq, for example, combines patch-clamp recording, cell imaging, and single-cell RNA-seq to obtain electrophysiology, morphology, and gene expression data from a single neuron. While these multi-modal data offer potential insights into neuronal functions, they can be heterogeneous and noisy. To address this, machine-learning methods have been used to align cells from different modalities onto a low-dimensional latent space, revealing multi-modal cell clusters. However, the use of those methods can be challenging for biologists and neuroscientists without computational expertise and also requires suitable computing infrastructure for computationally expensive methods. To address these issues, we developed a cloud-based web application, MANGEM (Multimodal Analysis of Neuronal Gene expression, Electrophysiology, and Morphology) at https://ctc.waisman.wisc.edu/mangem. MANGEM provides a step-by-step accessible and user-friendly interface to machine-learning alignment methods of neuronal multi-modal data while enabling real-time visualization of characteristics of raw and aligned cells. It can be run asynchronously for large-scale data alignment, provides users with various downstream analyses of aligned cells and visualizes the analytic results such as identifying multi-modal cell clusters of cells and detecting correlated genes with electrophysiological and morphological features. We demonstrated the usage of MANGEM by aligning Patch-seq multimodal data of neuronal cells in the mouse visual cortex.
Copy rights belong to original authors. Visit the link for more info
Podcast created by Paper Player, LLC | |||
27 Mar 2023 | Artificial Intelligence Boosted Molecular Dynamics | ||
Link to bioRxiv paper:
http://biorxiv.org/cgi/content/short/2023.03.25.534210v1?rss=1
Authors: Do, H. N., Miao, Y.
Abstract:
We have developed a new Artificial Intelligence Boosted Molecular Dynamics (AIBMD) method. Probabilistic Bayesian neural network models were implemented to construct boost potentials that exhibit Gaussian distribution with minimized anharmonicity, thereby allowing for accurate energetic reweighting and enhanced sampling of molecular simulations. AIBMD was demonstrated on model systems of alanine dipeptide and the fast-folding protein and RNA structures. For alanine dipeptide, 30ns AIMBD simulations captured up to 83-125 times more backbone dihedral transitions than 1s conventional molecular dynamics (cMD) simulations and were able to accurately reproduce the original free energy profiles. Moreover, AIBMD sampled multiple folding and unfolding events within 300ns simulations of the chignolin model protein and identified low-energy conformational states comparable to previous simulation findings. Finally, AIBMD captured a general folding pathway of three hairpin RNAs with the GCAA, GAAA, and UUCG tetraloops. Based on Deep Learning neural network, AIBMD provides a powerful and generally applicable approach to boosting biomolecular simulations. AIBMD is available with open source in OpenMM at https://github.com/MiaoLab20/AIBMD/.
Copy rights belong to original authors. Visit the link for more info
Podcast created by Paper Player, LLC | |||
23 Dec 2022 | Neural Networks beyond explainability: Selective inference for sequence motifs | ||
Link to bioRxiv paper:
http://biorxiv.org/cgi/content/short/2022.12.23.521748v1?rss=1
Authors: Villie, A., Veber, P., de Castro, Y., Jacob, L.
Abstract:
Over the past decade, neural networks have been successful at making predictions from biological sequences, especially in the context of regulatory genomics. As in other fields of deep learning, tools have been devised to extract features such as sequence motifs that can explain the predictions made by a trained network. Here we intend to go beyond explainable machine learning and introduce SEISM, a selective inference procedure to test the association between these extracted features and the predicted phenotype. In particular, we discuss how training a one-layer convolutional network is formally equivalent to selecting motifs maximizing some association score. We adapt existing sampling-based selective inference procedures by quantizing this selection over an infinite set to a large but finite grid. Finally, we show that sampling under a specific choice of parameters is sufficient to characterize the composite null hypothesis typically used for selective inference-a result that goes well beyond our particular framework. We illustrate the behavior of our method in terms of calibration, power and speed and discuss its power/speed trade-off with a simpler data-split strategy. SEISM paves the way to an easier analysis of neural networks used in regulatory genomics, and to more powerful methods for genome wide association studies (GWAS).
Copy rights belong to original authors. Visit the link for more info
Podcast created by Paper Player, LLC | |||
03 Mar 2023 | Underlying causes for prevalent false positives and false negatives in STARR-seq data | ||
Link to bioRxiv paper:
http://biorxiv.org/cgi/content/short/2023.03.03.530915v1?rss=1
Authors: Ni, P., Wu, S., Su, Z.
Abstract:
STARR-seq and its variants have been widely used to characterize enhancers. However, it has been reported that up to 87% of STARR peaks are located in repressive chromatins and are not functional in the tested cells. While some of the STARR peaks in repressive chromatins might be active in other cell/tissue types, some others might be false positives. Meanwhile, many active enhancers may not be identified by the current STARR-seq methods. However, the prevalence of and underlying causes for the artifacts are not fully understood. Based on predicted cis-regulatory modules (CRMs) and non-CRMs in the human genome as well as predicted active CRMs and non-active CRMs in a few human cell lines with STARR-seq data available, we reveal prevalent false positives and false negatives in STARR peaks and possible underlying causes. Our results will help design strategies to improve STARR-seq methods and interpret the results.
Copy rights belong to original authors. Visit the link for more info
Podcast created by Paper Player, LLC | |||
10 Nov 2022 | Massive proteogenomic reanalysis of publicly available proteomic datasets of human tissues in search for protein recoding via adenosine-to-inosine RNA editing | ||
Link to bioRxiv paper:
http://biorxiv.org/cgi/content/short/2022.11.10.515815v1?rss=1
Authors: Levitsky, L. I., Ivanov, M. V., Goncharov, A. O., Kliuchnikova, A. A., Bubis, J. A., Lobas, A. A., Solovyeva, E. M., Pyatnitskiy, M. A., Ovchinnikov, R. K., Kukharsky, M. S., Farafonova, T. E., Novikova, S. E., Zgoda, V. G., Tarasova, I. A., Gorshkov, M. V., Moshkovskii, S. A.
Abstract:
The proteogenomic search pipeline developed in this work has been applied for re-analysis of 40 publicly available shotgun proteomic datasets from various human tissues comprising more than 8,000 individual LC-MS/MS runs, of which 5442 .raw data files were processed in total. The scope of this re-analysis was focused on searching for ADAR-mediated RNA editing events, their clustering across samples of different origin, and classification. In total, 33 recoded protein sites were identified in 21 datasets. Of those, 18 sites were detected in at least two datasets representing the core human protein editome. In agreement with prior art works, neural and cancer tissues were found being enriched with recoded proteins. Quantitative analysis indicated that recoding of specific sites did not directly depend on the levels of ADAR enzymes or targeted proteins themselves, rather it was provided by differential and yet undescribed regulation of interaction of enzymes with mRNA. Nine recoding sites conservative between human and rodents were validated by targeted proteomics using stable isotope standards in murine brain cortex and cerebellum, and an additional one was validated in human cerebrospinal fluid. In addition to previous data of the same type from cancer proteomes, we provide a comprehensive catalog of recoding events caused by ADAR RNA editing in the human proteome.
Copy rights belong to original authors. Visit the link for more info
Podcast created by Paper Player, LLC | |||
04 Jul 2023 | Improved protein complex prediction with AlphaFold-multimer by denoising the MSA profile | ||
Link to bioRxiv paper:
http://biorxiv.org/cgi/content/short/2023.07.04.547638v1?rss=1
Authors: Bryant, P., Noe, F.
Abstract:
Structure prediction of protein complexes has improved significantly with AlphaFold2 and AlphaFold-multimer (AFM), but only 60% of dimers are accurately predicted. A way to improve the predictions is to inject noise to generate more diverse predictions. However, thousands of predictions are needed to obtain a few that are accurate in difficult cases. Here, we learn a bias to the MSA representation that improves the predictions by performing gradient descent through the AFM network. We effectively denoise the MSA profile, similar to how a blurry image would be sharpened. We demonstrate the performance on seven difficult targets from CASP15 and increase the average MMscore to 0.76 compared to 0.63 with AFM. We evaluate the procedure on 334 protein complexes where AFM fails and demonstrate an increased success rate (MMscore greater than 0.75) of 8% on these hard targets. Our protocol, AFProfile, provides a way to direct predictions towards a defined target function guided by the MSA. We expect gradient descent over the MSA to be useful for different tasks, such as generating alternative conformations. AFProfile is freely available at: https://github.com/patrickbryant1/AFProfile
Copy rights belong to original authors. Visit the link for more info
Podcast created by Paper Player, LLC | |||
08 Apr 2023 | A Weighted Two-stage Sequence Alignment Framework to Identify DNA Motifs from ChIP-exo Data | ||
Link to bioRxiv paper:
http://biorxiv.org/cgi/content/short/2023.04.06.535915v1?rss=1
Authors: Li, Y., Wang, Y., Wang, C., Fennel, A., Ma, A., Jiang, J., Liu, Z., Ma, Q., Liu, B.
Abstract:
Identifying precise transcription factor binding sites (TFBS) or regulatory DNA motifs plays a fundamental role in researching transcriptional regulatory mechanisms in cells and in helping construct regulatory networks. Current algorithms developed for motif searching focus on the analysis of ChIP-enriched peaks but are not able to integrate the ChIP signal in nucleotide resolution. We present a weighted two-stage alignment tool (TESA). Our framework implements an analysis workflow from experimental datasets to TFBS prediction results. It employs a binomial distribution model and graph searching model with ChIP-exonuclease (ChIP-exo) reads depth and sequence data. TESA can effectively measure the possibility for each position to be an actual TFBS in a given promoter sequence and predict statistically significant TFBS sequence segments. The algorithm substantially improves prediction accuracy and extends the scope of applicability of existing approaches. We apply the framework to a collection of 20 ChIP-exo datasets of E. coli from proChIPdb and evaluate the prediction performance through comparison with three existing programs. The performance evaluation against the compared programs indicates that TESA is more accurate for identifying regulatory motifs in prokaryotic genomes.
Copy rights belong to original authors. Visit the link for more info
Podcast created by Paper Player, LLC | |||
11 Feb 2023 | Female gene networks are expressed in myofibroblast-like smooth muscle cells in vulnerable atherosclerotic plaques. | ||
Link to bioRxiv paper:
http://biorxiv.org/cgi/content/short/2023.02.08.527690v1?rss=1
Authors: Benavente, E. D., Karnewar, S., Buono, M. F., Mili, E., Hartman, R. J. G., Kapteijn, D., Slenders, L., Daniels, M., Aherrahrou, R., Reinberger, T., Mol, B. M., de Borst, G. J., de Kleijn, D., Prange, K. H., Depuydt, M. A., de Winther, M. P., Kuiper, J., Björkegren, J. L., Erdmann, J., Civelek, M., Mokry, M., Owens, G. K., Pasterkamp, G., den Ruijter, H. M.
Abstract:
Objective: Women presenting with coronary artery disease (CAD) more often present with fibrous atherosclerotic plaques, which are currently understudied. Phenotypically modulated smooth muscle cells (SMCs) contribute to atherosclerosis in women. How these phenotypically modulated SMCs shape female versus male plaques is unknown. Approach and Results: Here, we show sex-stratified gene regulatory networks (GRNs) from human carotid atherosclerotic tissue. Prioritization of these networks identified two main SMC GRNs in late-stage atherosclerosis. Single-cell RNA-sequencing mapped these GRNs to two SMC phenotypes: a phenotypically modulated myofibroblast-like SMC network and a contractile SMC network. The myofibroblast-like GRN was mostly expressed in plaques that were vulnerable in females. Finally, mice orthologs of the female myofibroblast-like genes showed retained expression in advanced plaques from female mice but were downregulated in male mice during atherosclerosis progression. Conclusion: Female atherosclerosis is driven by GRNs that promote a fibrous vulnerable plaque rich in myofibroblast-like SMCs.
Copy rights belong to original authors. Visit the link for more info
Podcast created by Paper Player, LLC | |||
06 Apr 2023 | Microbiome Metabolome Integration Platform (MMIP): a web-based platform for microbiome and metabolome data integration and feature identification. | ||
Link to bioRxiv paper:
http://biorxiv.org/cgi/content/short/2023.04.04.535534v1?rss=1
Authors: Gautam, A., Bhowmik, D., Basu, S., Lahiri, A., Zeng, W., Paul, S.
Abstract:
Microbial community maintains its ecological dynamics via metabolites crosstalk. Hence knowledge of the metabolome, alongside its populace, would help us understand the functionality of that community and also predict how it alters in atypical conditions. The metabolic potential of a community from low-cost metagenomic sequencing data signifies the ability to produce or utilize each metabolite and can serve as potential markers of the differentially controlled biochemical pathways among different communities. We developed MMIP (Microbiome Metabolome Integration Platform), a web-based analytical and predictive tool that can describe the taxonomy, diversity variation and the metabolic potential between two sets of microbial communities from targeted amplicon sequencing data. MMIP is capable of highlighting statistically significant taxonomic, enzymatic and metabolic attributes as well as learning based features associated with one group in comparison with another. Further MMIP is capable of predicting the linkages indicating the relationship among species or groups of microbes in the community, a specific enzyme profile related to those organisms, and a specific compound or metabolite. Thus, MMIP can serve as a user-friendly, online web-server for performing most of the analyses of microbiome associated research from targeted amplicon sequencing data, and can provide the probable metabolite signature, along with learning-based linkage associations of any sample set, without the need for initial metabolomic analysis thereby helping in hypothesis generation.
Copy rights belong to original authors. Visit the link for more info
Podcast created by Paper Player, LLC | |||
05 Dec 2022 | Covidscope: An atlas-scale COVID-19 resource for single-cell meta analysis at sample and cell levels | ||
Link to bioRxiv paper:
http://biorxiv.org/cgi/content/short/2022.12.03.518997v1?rss=1
Authors: Yin, D., Cao, Y., Chen, J., Mak, C. L. Y., Yu, K. H. O., Lin, Y., Ho, J. W. K., Yang, J. Y. H.
Abstract:
Recent advancements in the use of single-cell technologies in large cohort studies enable the investigation of cellular response and mechanisms associated with disease outcome, including COVID-19. Several efforts have been made using single-cell RNA-sequencing to better understand the immune response to COVID-19 virus infection. Nonetheless, it is often difficult to compare or integrate data from multiple data sets due to challenges in data normalisation, metadata harmonisation, and having a common interface to quickly query and access this vast amount of data. Here we present Covidscope (http://covidsc.d24h.hk/), a well-curated open web resource that currently contains single-cell gene expression data and associated metadata of almost 5 million blood and immune cells extracted from almost 1,000 COVID-19 patients across 20 studies around the world. Our collection contains the integrated data with harmonised metadata and multi-level cell type annotations. By combining NoSQL and optimised index, our Covidscope achieves rapid subsetting of high-dimensional gene expression data based on both data set level, donor-level (e.g., age and sex of patients) and cell-level (e.g., expression of specific gene markers) metadata, enabling multiple efficient downstream single-cell meta-analysis.
Copy rights belong to original authors. Visit the link for more info
Podcast created by Paper Player, LLC | |||
25 Apr 2023 | Model-based dimensionality reduction for single-cell RNA-seq using generalized bilinear models | ||
Link to bioRxiv paper:
http://biorxiv.org/cgi/content/short/2023.04.21.537881v1?rss=1
Authors: Nicol, P. B., Miller, J. W.
Abstract:
Dimensionality reduction is a critical step in the analysis of single-cell RNA-seq data. The standard approach is to apply a transformation to the count matrix, followed by principal components analysis. However, this approach can spuriously indicate heterogeneity where it does not exist and mask true heterogeneity where it does exist. An alternative approach is to directly model the counts, but existing model-based methods tend to be computationally intractable on large datasets and do not quantify uncertainty in the low-dimensional representation. To address these problems, we develop scGBM, a novel method for model-based dimensionality reduction of single-cell RNA-seq data. scGBM employs a scalable algorithm to fit a Poisson bilinear model to datasets with millions of cells and quantifies the uncertainty in each cell's latent position. Furthermore, scGBM leverages these uncertainties to assess the confidence associated with a given cell clustering. On real and simulated single-cell data, we find that scGBM produces low-dimensional embeddings that better capture relevant biological information while removing unwanted variation. scGBM is publicly available as an R package.
Copy rights belong to original authors. Visit the link for more info
Podcast created by Paper Player, LLC | |||
16 Nov 2022 | MOT: a Multi-Omics Transformer for multiclass classification tumour types predictions | ||
Link to bioRxiv paper:
http://biorxiv.org/cgi/content/short/2022.11.14.516459v1?rss=1
Authors: OSSENI, M. A., Tossou, P., Laviolette, F., Corbeil, J.
Abstract:
Motivation Breakthroughs in high-throughput technologies and machine learning methods have enabled the shift towards multi-omics modelling as the preferred means to understand the mechanisms underlying biological processes. Machine learning enables and improves complex disease prognosis in clinical settings. However, most multi-omic studies primarily use transcriptomics and epigenomics due to their over-representation in databases and their early technical maturity compared to others omics. For complex phenotypes and mechanisms, not leveraging all the omics despite their varying degree of availability can lead to a failure to understand the underlying biological mechanisms and leads to less robust classifications and predictions. Results We proposed MOT (Multi-Omic Transformer), a deep learning based model using the transformer architecture, that discriminates complex phenotypes (herein cancer types) based on five omics data types: transcriptomics (mRNA and miRNA), epigenomics (DNA methylation), copy number variations (CNVs), and proteomics. This model achieves an F1-score of $98.37%$ among 33 tumour types on a test set without missing omics views and an F1-score of $96.74%$ on a test set with missing omics views. It also identifies the required omic type for the best prediction for each phenotype and therefore could guide clinical decision-making when acquiring data to confirm a diagnostic. The newly introduced model can integrate and analyze five or more omics data types even with missing omics views and can also identify the essential omics data for the tumour multiclass classification tasks. It confirms the importance of each omic view. Combined, omics views allow a better differentiation rate between most cancer diseases. Our study emphasized the importance of multi-omic data to obtain a better multiclass cancer classification. Availability and implementation: MOT source code is available at url{https://github.com/dizam92/multiomic_predictions}.
Copy rights belong to original authors. Visit the link for more info
Podcast created by Paper Player, LLC | |||
15 Feb 2023 | Single-cell transcriptomic analysis of mouse liver reveals nonparenchymal cells' intricate responses to PCB126 exposure | ||
Link to bioRxiv paper:
http://biorxiv.org/cgi/content/short/2023.02.13.528414v1?rss=1
Authors: Xu, F., Fu, Y., Yang, J., Yu, C., Shen, C.
Abstract:
Polychlorinated biphenyls (PCBs) are ubiquitous and representative pollutants that pose great health risks. While cells responses to dioxin-like PCBs tend to be studied on a bulk scale, few studies have been made from a single-cell level. Here, by using single-cell RNA sequencing, we depicted a detailed landscape of hepatic nonparenchymal cells intricate responses to PCB126 exposure. A total of 13 clusters were identified. Notably, PCB126 exposure resulted in cell-type-specific gene expression profiles and genetic pathways. By analyzing genes related to aryl hydrocarbon receptors, we discovered that PCB126 induced the canonical genomic AhR pathway mainly in endothelial cells. In contrast, other cell types showed little induction. Enrichment pathway analysis indicated that immune cells changed their transcriptional patterns in response to PCB126. ScRNA-seq is a powerful tool to dissect underlying mechanisms of chemical toxicity regarding biological heterogeneity. Taken together, our study not only extends our current understanding of PCB126 toxicity, but also emphasizes the importance of in vivo cell heterogeneity in environmental toxicology.
Copy rights belong to original authors. Visit the link for more info
Podcast created by Paper Player, LLC | |||
14 Apr 2023 | Identification of Mirror Repeats in Viral Genomes using FPCB Analysis | ||
Link to bioRxiv paper:
http://biorxiv.org/cgi/content/short/2023.04.13.536685v1?rss=1
Authors: Yadav, P., Kumari, J., Yadav, P., Yadav, R., Yadav, S., Sharma, D., Singh, A., Sehrawat, B., Yadav, M., Yadav, S.
Abstract:
The majority of living domains consist of DNA as genetic material with the minor exception of viruses. The unique nature of every species determines by its unique pattern of genome or gene products. The genomic features become an evident example of evolutionary study also. Different types of repeat patterns are observed in genomes of living domains including human beings whose two third portion of the genome is repetitive. Among the varied type of repeat sequences Mirror Repeats (MR) play crucial roles at the genetic level in every species. The major focus of our research is on identification & to check the distribution of mirror repeat. For this, we employed a bioinformatics-based approach refer as FASTA PARALLEL COMPLEMENT BLAST (FPCB) to identify unique mirror repeat (MR) sequences in some selected viral genomes from three different categories (Animal, Plant & Human). The identified repeats vary in their length as well as found to be distributed throughout the selected viral genomes. The maximum no of MR were reported in the case of Dengue virus (229) & minimum is in the case of TMV (97). In the remaining selected viruses - HCV, HPV, HTLV-1, PVY, Rabies virus 178, 156, 175, 203 & 204 MR sequences were reported. These sequences can be utilized in many ways like in molecular diagnosis, drug delivery target as well as evolutionary study, etc. The present research also helps in the development of novel tools of bioinformatics to study mirror repeats and their functional perspective in the context of their occurrence in all domains.
Copy rights belong to original authors. Visit the link for more info
Podcast created by Paper Player, LLC | |||
28 Jan 2023 | scGREAT: Graph-based regulatory element analysis tool for single-cell multi-omics data | ||
Link to bioRxiv paper:
http://biorxiv.org/cgi/content/short/2023.01.27.525916v1?rss=1
Authors: Liu, C., Wang, L., Liu, Z.
Abstract:
Motivation: With the development in single-cell multi-omics sequencing technology and data integration algorithms, we have entered the single-cell multi-omics era. Current multi-omics analysis algorithms failed to systematically dissect the heterogeneity within the datasets when inferring cis-regulatory events. Thus, there is a need for cis-regulatory element inferring algorithms that considers the cellular heterogeneity. Results: Here, we propose scGREAT, a single-cell multi-omics regulatory state analysis Python package with a rapid graph-based correlation measurement L. The graph-based correlation method assigns each cell a local L index, pinpointing specific cell groups of certain regulatory states. Such single-cell resolved regulatory state information enables the heterogeneity analysis equipped in the package. Applying scGREAT to the 10X Multiome PBMC dataset, we demonstrated how it could help subcluster cell types, infer regulation-based pseudo-time trajectory, discover feature modules, and find cluster-specific regulatory gene-peak pairs. Besides, we showed that global L index, which is the average of all local L values, is a better replacement for Pearsons r in ruling out confounding regulatory relationships that are not of research interests.
Copy rights belong to original authors. Visit the link for more info
Podcast created by Paper Player, LLC | |||
28 Jul 2023 | AnglesRefine: refinement of 3D protein structures using Transformer based on torsion angles | ||
Link to bioRxiv paper:
http://biorxiv.org/cgi/content/short/2023.07.25.550599v1?rss=1
Authors: Zhang, L., Zhu, J., Wang, S., Hou, J., Si, D., Cao, R.
Abstract:
Motivation: The goal of protein structure refinement is to enhance the precision of predicted protein models, particularly at the residue level of the local structure. Existing refinement approaches primarily rely on physics, whereas molecular simulation methods are resource-intensive and time-consuming. In this study, we employ deep learning methods to extract structural constraints from protein structure residues to assist in protein structure refinement. We introduce a novel method, AnglesRefine, which focuses on a protein's secondary structure and employs a transformer model to refine various protein structure angles (psi, phi, omega, CA_C_N_angle, C_N_CA_angle, N_CA_C_angle), ultimately generating a superior protein model based on the refined angles. Results: We evaluate our approach against other cutting-edge protein structure refinement methods using the CASP11-14 and CASP15 datasets. Experimental outcomes indicate that our method generally surpasses other techniques on the CASP11-14 test dataset, while performing comparably or marginally better on the CASP15 test dataset. Our method consistently demonstrates the least likelihood of model quality degradation, e.g., the degradation percentage of our method is less than 10%, while other methods are about 50%. Furthermore, as our approach eliminates the need for conformational search and sampling, it significantly reduces computational time compared to existing protein structure refinement methods. Availability: https://github.com/Cao-Labs/AnglesRefine.git
Copy rights belong to original authors. Visit the link for more info
Podcast created by Paper Player, LLC | |||
02 May 2023 | memo-eQTL: DNA methylation modulated genetic variant effect on gene transcriptional regulation | ||
Link to bioRxiv paper:
http://biorxiv.org/cgi/content/short/2023.05.02.539122v1?rss=1
Authors: Zeng, Y., Jain, R., Ahmed, M., Guo, H., Zhong, Y., Xu, W., He, H. H.
Abstract:
Copy rights belong to original authors. Visit the link for more info
Podcast created by Paper Player, LLC | |||
28 Jun 2023 | FISHtoFigure: An easy-to-use tool for rapid, multi-target partitioning and analysis of sub-cellular mRNA transcripts in smFISH data | ||
Link to bioRxiv paper:
http://biorxiv.org/cgi/content/short/2023.06.28.546871v1?rss=1
Authors: Bentley-Abbot, C., Heslop, R., Pirillo, C., Sinton, M. C., Chandrasegaran, P. R. G., McConnell, G., Roberts, E., Hutchinson, E., MacLeod, A.
Abstract:
Single molecule fluorescence in situ hybridisation (smFISH) has become a valuable tool to investigate the mRNA expression of single cells. However, it requires a considerable amount of bioinformatic expertise to use currently available open-source analytical software packages to extract and analyse quantitative data about transcript expression. Here, we present FISHtoFigure, a new software tool developed specifically for the analysis of mRNA abundance and co-expression in QuPath-quantified, multi-labelled smFISH data. FISHtoFigure facilitates the automated spatial analysis of transcripts of interest, allowing users to analyse populations of cells positive for specific combinations of mRNA targets without the need for bioinformatics expertise. As a proof of concept and to demonstrate the capabilities of this new research tool, we have validated FISHtoFigure in multiple biological systems. We used FISHtoFigure to identify an upregulation of T-cells in the spleens of mice infected with influenza A virus, before analysing more complex data showing crosstalk between microglia and regulatory B-cells in the brains of mice infected with Trypanosoma brucei brucei. These analyses demonstrate the ease of analysing cell expression profiles using FISHtoFigure and the value of this new tool in the field of smFISH data analysis.
Copy rights belong to original authors. Visit the link for more info
Podcast created by Paper Player, LLC | |||
28 Jan 2023 | HAT: de novo variant calling for highly accurate short-read and long-read sequencing data | ||
Link to bioRxiv paper:
http://biorxiv.org/cgi/content/short/2023.01.27.525940v1?rss=1
Authors: Ng, J. K., Turner, T. N.
Abstract:
Motivation: de novo variant (DNV) calling is challenging from parent-child sequenced trio data. We developed Hare And Tortoise (HAT) to work as an automated workflow to detect DNVs in highly accurate short-read and long-read sequencing data. Reliable detection of DNVs is important for human genetics studies (e.g., autism, epilepsy). Results: HAT is a workflow to detect DNVs from short-read and long read sequencing data. This workflow begins with aligned read data (i.e., CRAM or BAM) from a parent-child sequenced trio and outputs DNVs. HAT detects high-quality DNVs from short-read whole-exome sequencing, short-read whole-genome sequencing, and highly accurate long-read sequencing data. Availability: https://github.com/TNTurnerLab/HAT Contact: tychele@wustl.edu Supplementary information: Supplementary data are available at bioRxiv.
Copy rights belong to original authors. Visit the link for more info
Podcast created by Paper Player, LLC | |||
04 Jul 2023 | Accelerated somatic mutation calling for whole-genome and whole-exome sequencing data from heterogenous tumor samples | ||
Link to bioRxiv paper:
http://biorxiv.org/cgi/content/short/2023.07.04.547569v1?rss=1
Authors: Ji, S., Zhu, T., Sethia, A., Wang, W.
Abstract:
Accurate detection of somatic mutations in DNA sequencing data is a fundamental prerequisite for cancer research. Previous analytical challenge was overcome by consensus mutation calling from four to five popular callers. This, however, increases the already nontrivial computing time from individual callers. Here, we launch MuSE2.0, powered by multi-step parallelization and efficient memory allocation, to resolve the computing time bottleneck. MuSE2.0 speeds up 50 times than MuSE1.0 and 8-80 times than other popular callers. Our benchmark study suggests combining MuSE2.0 and the recently expedited Strelka2 can achieve high efficiency and accuracy in analyzing large cancer genomic datasets.
Copy rights belong to original authors. Visit the link for more info
Podcast created by Paper Player, LLC | |||
19 Jul 2023 | Scalable querying of human cell atlases via a foundational model reveals commonalities across fibrosis-associated macrophages | ||
Link to bioRxiv paper:
http://biorxiv.org/cgi/content/short/2023.07.18.549537v1?rss=1
Authors: Heimberg, G., Kuo, T. C., DePianto, D., Heigl, T., Diamant, N., Salem, O., Scalia, G., Biancalani, T., Rock, J., Turley, S., Bravo, H. C., Kaminker, J., Vander Heiden, J. A., Regev, A.
Abstract:
Single-cell RNA-seq (scRNA-seq) studies have profiled over 100 million human cells across diseases, developmental stages, and perturbations to date. A singular view of this vast and growing expression landscape could help reveal novel associations between cell states and diseases, discover cell states in unexpected tissue contexts, and relate in vivo cells to in vitro models. However, these require a common, scalable representation of cell profiles from across the body, a general measure of their similarity, and an efficient way to query these data. Here, we present SCimilarity, a metric learning framework to learn and search a unified and interpretable representation that annotates cell types and instantaneously queries for a cell state across tens of millions of profiles. We demonstrate SCimilarity on a 22.7 million cell corpus assembled across 399 published scRNA-seq studies, showing accurate integration, annotation and querying. We experimentally validated SCimilarity by querying across tissues for a macrophage subset originally identified in interstitial lung disease, and showing that cells with similar profiles are found in other fibrotic diseases, tissues, and a 3D hydrogel system, which we then repurposed to yield this cell state in vitro. SCimilarity serves as a foundational model for single cell gene expression data and enables researchers to query for similar cellular states across the entire human body, providing a powerful tool for generating novel biological insights from the growing Human Cell Atlas.
Copy rights belong to original authors. Visit the link for more info
Podcast created by Paper Player, LLC | |||
12 Mar 2023 | STdGCN: accurate cell-type deconvolution using graph convolutional networks in spatial transcriptomic data | ||
Link to bioRxiv paper:
http://biorxiv.org/cgi/content/short/2023.03.10.532112v1?rss=1
Authors: Li, Y., Luo, Y.
Abstract:
Spatially resolved transcriptomics performs high-throughput measurement of transcriptomes while preserving spatial information about the cellular organizations. However, many spatially resolved transcriptomic technologies can only distinguish spots consisting of a mixture of cells instead of working at single-cell resolution. Here, we present STdGCN, a graph neural network model designed for cell type deconvolution of spatial transcriptomic (ST) data that can leverage abundant single-cell RNA sequencing (scRNA-seq) data as reference. STdGCN is the first model incorporating the expression profiles from single cell data as well as the spatial localization information from the ST data for cell type deconvolution. Extensive benchmarking experiments on multiple ST datasets showed that STdGCN outperformed 13 published state-of-the-art models. Applied to a human breast cancer Visium dataset, STdGCN discerned spatial distributions between stroma, lymphocytes and cancer cells for tumor microenvironment dissection. In a human heart ST dataset, STdGCN detected the changes of potential endothelial-cardiomyocyte communications during tissue development. Our results demonstrate that STdGCN can serve as a robust and versatile tool for cell type deconvolution across multiple ST platforms and tissues. STdGCN is available as open source Python software at https://github.com/luoyuanlab/stdgcn.
Copy rights belong to original authors. Visit the link for more info
Podcast created by Paper Player, LLC | |||
16 Mar 2023 | Multiple sequence-alignment-based RNA language model and its application to structural inference | ||
Link to bioRxiv paper:
http://biorxiv.org/cgi/content/short/2023.03.15.532863v1?rss=1
Authors: Zhang, Y., Lang, M., Jiang, J., Gao, Z., Xu, F., Litfin, T., Chen, K., Singh, J., Huang, X., Song, G., Tian, Y., Zhan, J., Chen, J., Zhou, Y.
Abstract:
Compared to proteins, DNA and RNA are more difficult languages to interpret because 4-letter-coded DNA/RNA sequences have less information content than 20-letter-coded protein sequences. While BERT (Bidirectional Encoder Representations from Transformers)-like language models have been developed for RNA, they are ineffective at capturing the evolutionary information from homologous sequences because unlike proteins, RNA sequences are less conserved. Here, we have developed an unsupervised Multiple sequence-alignment-based RNA language model (RNA-MSM) by utilizing homologous sequences from an automatic pipeline, RNAcmap. The resulting unsupervised, two-dimensional attention maps and one-dimensional embeddings from RNA-MSM can be directly mapped with high accuracy to 2D base pairing probabilities and 1D solvent accessibilities, respectively. Further fine-tuning led to significantly improved performance on these two downstream tasks over existing state-of-the-art techniques. We anticipate that the pre-trained RNA-MSM model can be fine-tuned on many other tasks related to RNA structure and function.
Copy rights belong to original authors. Visit the link for more info
Podcast created by Paper Player, LLC | |||
20 Dec 2022 | HBM-CITEseq: a uniform CITE-seq processing pipeline for the HuBMAP Consortium | ||
Link to bioRxiv paper:
http://biorxiv.org/cgi/content/short/2022.12.19.521058v1?rss=1
Authors: Lu, X., Ruffalo, M.
Abstract:
As part of the HuBMAP Consortium we have been developing methods for uniformly processing and indexing multiple single-cell datasets, which enable efficient integration of data from different platforms. HuBMAP Consortium computational pipelines have so far focused on unimodal data types such as single-cell/nucleus RNA sequencing and single nucleus ATAC-seq. Here we present HBM-CITEseq, a processing pipeline for Cellular Indexing of Transcriptomes and Epitopes by Sequencing (CITE-seq) datasets, the first multimodal sequencing processing pipeline for the HuBMAP Consortium, with transcriptomic outputs from HBM-CITEseq matching those of the HuBMAP RNA-seq pipeline. HBM-CITEseq is a CWL workflow wrapping command-line tools encapsulated in Docker images. It is freely available on GitHub at https://github.com/hubmapconsortium/citeseq-pipeline.
Copy rights belong to original authors. Visit the link for more info
Podcast created by Paper Player, LLC | |||
21 Mar 2023 | S3-CIMA: Supervised spatial single-cell image analysis for the identification of disease-associated cell type compositions in tissue | ||
Link to bioRxiv paper:
http://biorxiv.org/cgi/content/short/2023.03.17.533167v1?rss=1
Authors: Babaei, S., Christ, J. C., Makky, A., Zidane, M., Wistuba- Hamprecht, K., Schuerch, C. M., Claassen, M.
Abstract:
The spatial organization of various cell types within the tissue microenvironment is a key element for the formation of physiological and pathological processes, including cancer and autoimmune diseases. Here, we present S3-CIMA, a weakly supervised convolutional neural network model that enables the detection of disease-specific microenvironment compositions from high-dimensional proteomic imaging data. We demonstrate the utility of this approach by determining cancer outcome- and cellular signaling-specific spatial cell state compositions in highly multiplexed fluorescence microscopy data of the tumor microenvironment in colorectal cancer. Moreover, we use S3-CIMA to identify disease onset-specific changes of the pancreatic tissue microenvironment in type 1 diabetes using imaging mass cytometry data. We evaluated S3-CIMA as a powerful tool to discover novel disease-associated spatial cellular interactions from currently available and future spatial biology datasets.
Copy rights belong to original authors. Visit the link for more info
Podcast created by Paper Player, LLC | |||
26 Apr 2023 | De Novo Design of κ-Opioid Receptor Antagonists Using a Generative Deep Learning Framework | ||
Link to bioRxiv paper:
http://biorxiv.org/cgi/content/short/2023.04.25.537995v1?rss=1
Authors: Salas-Estrada, L., Provasi, D., Qiu, X., Kaniskan, H. U., Huang, X.-P., DiBerto, J., Marcelo Lamim Ribeiro, J., Jin, J., Roth, B. L., Filizola, M.
Abstract:
Likely effective pharmacological interventions for the treatment of opioid addiction include attempts to attenuate brain reward de[fi]cits during periods of abstinence. Pharmacological blockade of the {kappa}-opioid receptor (KOR) has been shown to abolish brain reward de[fi]cits in rodents during withdrawal, as well as to reduce the escalation of opioid use in rats with extended access to opioids. Although KOR antagonists represent promising candidates for the treatment of opioid addiction, very few potent selective KOR antagonists are known to date and most of them exhibit signi[fi]cant safety concerns. Here, we used a generative deep learning framework for the de novo design of chemotypes with putative KOR antagonistic activity. Molecules generated by models trained with this framework were prioritized for chemical synthesis based on their predicted optimal interactions with the receptor. Our models and proposed training protocol were experimentally validated by binding and functional assays.
Copy rights belong to original authors. Visit the link for more info
Podcast created by Paper Player, LLC | |||
01 May 2023 | scGPT: Towards Building a Foundation Model for Single-Cell Multi-omics Using Generative AI | ||
Link to bioRxiv paper:
http://biorxiv.org/cgi/content/short/2023.04.30.538439v1?rss=1
Authors: Cui, H., Wang, C., Maan, H., Wang, B.
Abstract:
Copy rights belong to original authors. Visit the link for more info
Podcast created by Paper Player, LLC | |||
12 Jul 2023 | Hyperparameter optimisation in differential evolution using Summed Local Difference Strings, a rugged but easily calculated landscape for combinatorial search problems | ||
Link to bioRxiv paper:
http://biorxiv.org/cgi/content/short/2023.07.11.548503v1?rss=1
Authors: Pannu, H. S., Kell, D. B.
Abstract:
We analyse the effectiveness of differential evolution hyperparameters in large-scale search problems, i.e. those with very many variables or vector elements, using a novel objective function that is easily calculated from the vector/string itself. The objective function is simply the sum of the differences between adjacent elements. For both binary and real-valued elements whose smallest and largest values are min and max in a vector of length N, the value of the objective function ranges between 0 and (N-1) x (max-min) and can thus easily be normalised if desired. This provides for a conveniently rugged landscape. Using this we assess how effectively search varies with both the values of fixed hyperparameters for Differential Evolution and the string length. String length, population size and generations for computational iterations have been studied. Finally, a neural network is trained by systematically varying three hyper-parameters, viz population (NP), mutation factor (F) and crossover rate (CR), and two output target variables are collected (a) median and (b) maximum cost function values from 10-trial experiments. This neural system is then tested on an extended range of data points generated by varying the three parameters on a finer scale to predict both median and maximum function costs. The results obtained from the machine learning model have been validated with actual runs using Pearsons coefficient to justify the reliability to motivate the use of machine learning techniques over grid search for hyper-parameter search for numerical optimisation algorithms. The performance has also been compared with SMAC3 and OPTUNA in addition to grid search and random search.
Copy rights belong to original authors. Visit the link for more info
Podcast created by Paper Player, LLC | |||
07 Apr 2023 | Binary classification machine-learning Unveils Sex-Dependent mutated gene Signatures in Melanoma | ||
Link to bioRxiv paper:
http://biorxiv.org/cgi/content/short/2023.04.04.535515v1?rss=1
Authors: Levy, C., Elkoshi, N., Parikh, S., Mahameed, S., Meidan, A., Rubin, E.
Abstract:
There are significant differences in the prevalence of cancer type, primary tumor body site, and mutation load between men and women, but the mechanisms underlying these sex-dependent differences is mostly unknown. Here we used binary classification machine-learning methodology to study sex-correlated somatic mutations signatures in cutaneous melanoma. We identified a number of genes that are more frequently mutated in females compared to males. Mutations in two genes, LAMA2 and TPTE, together with a set of specific genes that are not mutated, can predict sex of melanoma patients. Over representation analysis of genes clustered with LAMA2 revealed significant enrichment in androgen and estrogen biosynthesis and metabolism pathways, suggesting that mutation of LAMA2 might be involved in biased sex hormone synthesis in melanoma. Taken together, our analysis shows that gender can be predicted based on mutation status of genes in melanoma and that certain mutations are predictive of survival beyond sex differences. Our results will lead to better diagnosis and more effective personalized treatment of melanoma.
Copy rights belong to original authors. Visit the link for more info
Podcast created by Paper Player, LLC | |||
04 Jul 2023 | Multi-view integration of microbiome data for predicting host disease and identifying disease-associated features | ||
Link to bioRxiv paper:
http://biorxiv.org/cgi/content/short/2023.07.03.547607v1?rss=1
Authors: Muller, E., Shiryan, I., Borenstein, E.
Abstract:
Machine learning (ML) has become a widespread strategy for studying complex microbiome signatures associated with disease. To this end, metagenomics data are often processed into a single "view" of the microbiome, such as its taxonomic (species) or functional (gene) composition, which in turn serves as input to such ML models. When further omics are available, such as metabolomics, these can be analyzed as additional complementary views. Following training and evaluation, the resulting model can be explored to identify informative features, generating hypotheses regarding underlying mechanisms. Importantly, however, using a single view generally offers relatively limited hypotheses, failing to capture simultaneous shifts or dependencies across multiple microbiome layers that likely play a role in microbiome-host interactions. In this work, inspired by the broad domain of multi-view learning, we constructed an integrated ML analysis pipeline using multiple microbiome views. We specifically aimed to investigate the impact of various integration approaches on the ability to predict disease state based on multiple microbiome-related views, and to generate biological insights. Applying this pipeline to data from 25 case-control metagenomics studies, we found that multi-view models typically result in performances that are comparable to the best-performing single view, yet, provide a mixed set of informative features from different views, while accounting for dependencies and links between them. To further enhance such models, we developed a new framework termed MintTea, based on sparse generalized canonical correlation analysis, to identify multi-view modules of features, highlighting shared trends in the data expressed by the different views. We showed that this framework identified multiple modules that were both highly predictive of the disease state, and exhibited strong within-module associations between features from different views. We accordingly advocate for using multi-view models to capture multifaceted microbiome signatures that likely better reflect the complex mechanisms underlying microbiome-disease associations.
Copy rights belong to original authors. Visit the link for more info
Podcast created by Paper Player, LLC | |||
08 Jan 2023 | PASTE2: Partial Alignment of Multi-slice Spatially Resolved Transcriptomics Data | ||
Link to bioRxiv paper:
http://biorxiv.org/cgi/content/short/2023.01.08.523162v1?rss=1
Authors: Liu, X., Zeira, R., Raphael, B.
Abstract:
Spatially resolved transcriptomics (SRT) technologies measure mRNA expression at thousands of locations in a tissue slice. However, nearly all SRT technologies measure expression in two dimensional slices extracted from a three-dimensional tissue, thus losing information that is shared across multiple slices from the same tissue. Integrating SRT data across multiple slices can help recover this information and improve downstream expression analyses, but multi-slice alignment and integration remains a challenging task. Existing methods for integrating SRT data either do not use spatial information or assume that the morphology of the tissue is largely preserved across slices, an assumption that is often violated due to biological or technical reasons. We introduce PASTE2, a method for partial alignment and 3D reconstruction of multi-slice SRT datasets, allowing only partial overlap between aligned slices and/or slice-specific cell types. PASTE2 formulates a novel partial Fused Gromov-Wasserstein Optimal Transport problem, which we solve using a conditional gradient algorithm. PASTE2 includes a model selection procedure to estimate the fraction of overlap between slices, and optionally uses information from histological images that accompany some SRT experiments. We show on both simulated and real data that PASTE2 obtains more accurate alignments than existing methods. We further use PASTE2 to reconstruct a 3D map of gene expression in a Drosophila embryo from a 16 slice Stereo-seq dataset. PASTE2 produces accurate alignments of multi-slice datasets from multiple SRT technologies, enabling detailed studies of spatial gene expression across a wide range of biological applications.
Copy rights belong to original authors. Visit the link for more info
Podcast created by Paper Player, LLC | |||
15 Dec 2022 | NanopoReaTA: a user-friendly tool for nanopore-seq real-time transcriptional analysis | ||
Link to bioRxiv paper:
http://biorxiv.org/cgi/content/short/2022.12.13.520220v1?rss=1
Authors: Wierczeiko, A., Pastore, S., Muendnich, S., Helm, M., Butto, T., Gerber, S.
Abstract:
Oxford Nanopore Technologies' (ONT) sequencing platform offers an excellent opportunity to perform real-time analysis during sequencing. This feature allows for early insights into experimental data and accelerates a potential decision-making process for further analysis, which can be particularly relevant in the clinical context. Although some tools for the real-time analysis of DNA-sequencing data already exist, there is currently no application available for transcriptome data analysis designed for scientists or physicians with limited bioinformatics knowledge. Here we introduce NanopoReaTA, a user-friendly real-time analysis toolbox for RNA sequencing data from ONT. Sequencing results from a running or finished experiment are processed through an R Shiny-based graphical user interface (GUI) with an integrated Nextflow pipeline for whole transcriptome or gene-specific analyses. NanopoReaTA provides visual snapshots of a sequencing run in progress, thus enabling interactive sequencing and rapid decision-making that could also be applied to clinical cases.
Copy rights belong to original authors. Visit the link for more info
Podcast created by Paper Player, LLC | |||
15 Dec 2022 | Disentangling shared and group-specific variations in single-cell transcriptomics data with multiGroupVI | ||
Link to bioRxiv paper:
http://biorxiv.org/cgi/content/short/2022.12.13.520349v1?rss=1
Authors: Weinberger, E., Lopez, R., Hutter, J.-C., Regev, A.
Abstract:
Single-cell RNA sequencing (scRNA-seq) technologies have enabled a greater understanding of previously unexplored biological diversity. Based on the design of such experiments, individual cells from scRNA-seq datasets can often be attributed to non-overlapping "groups". For example, these group labels may denote the cell's tissue or cell line of origin. In this setting, one important problem consists in discerning patterns in the data that are shared across groups versus those that are group-specific. However, existing methods for this type of analysis are mainly limited to (generalized) linear latent variable models. Here we introduce multiGroupVI, a deep generative model for analyzing grouped scRNA-seq datasets that decomposes the data into shared and group-specific factors of variation. We first validate our approach on a simulated dataset, on which we significantly outperform state-of-the-art methods. We then apply it to explore regional differences in an scRNA-seq dataset sampled from multiple regions of the mouse small intestine. We implemented multiGroupVI using the scvi-tools library, and released it as open-source software at https://github.com/Genentech/multiGroupVI.
Copy rights belong to original authors. Visit the link for more info
Podcast created by Paper Player, LLC | |||
02 Jul 2023 | CHAPERONg: A tool for automated GROMACS-based molecular dynamics simulations and trajectory analyses | ||
Link to bioRxiv paper:
http://biorxiv.org/cgi/content/short/2023.07.01.546945v1?rss=1
Authors: Yekeen, A. A., Durojaye, O. A., Idris, M. O., Muritala, H. F., Arise, R. O.
Abstract:
Molecular dynamics (MD) simulation is a powerful computational tool used in biomolecular studies to investigate the dynamics, energetics, and interactions of a wide range of biological systems at the atomic level. GROMACS is a widely used free and open-source biomolecular MD simulation software recognized for its efficiency, accuracy, and extensive range of simulation options. However, the complexity of setting up, running, and analyzing MD simulations for diverse systems often poses a significant challenge, requiring considerable time, effort, and expertise. Here, we introduce CHAPERONg, a tool that automates the GROMACS MD simulation pipelines for protein and protein-ligand systems. CHAPERONg also integrates seamlessly with GROMACS modules and third-party tools to provide comprehensive analyses of MD simulation trajectories, offering up to 20 post-simulation processing and trajectory analyses. It also streamlines and automates established pipelines for conducting and analyzing biased MD simulations via the steered MD-umbrella sampling workflow. Thus, CHAPERONg makes MD simulations more accessible to beginner GROMACS users whilst empowering experts to focus on data interpretation and other less programmable aspects of MD simulation workflows.
Copy rights belong to original authors. Visit the link for more info
Podcast created by Paper Player, LLC | |||
06 Apr 2023 | THAPBI PICT - a fast, cautious, and accurate metabarcoding analysis pipeline | ||
Link to bioRxiv paper:
http://biorxiv.org/cgi/content/short/2023.03.24.534090v1?rss=1
Authors: Cock, P. J. A., Cooke, D. E. L., Thorpe, P., Pritchard, L.
Abstract:
THAPBI PICT is an open source software pipeline for metabarcoding analysis with multiplexed Illumina paired-end reads, including where different amplicons are sequenced together. We demonstrate using worked examples with our own and public data sets how, with appropriate primer settings and a custom database, THAPBI PICT can be applied to other amplicons and organisms, and used for reanalysis of existing datasets. The core dataflow of the implementation is (i) data reduction to unique marker sequences, often called amplicon sequence variants (ASVs), (ii) dynamic thresholds for discarding low abundance sequences to remove noise and artifacts (rather than error correction by default), before (iii) classification using a curated reference database. The default classifier assigns a label to each query sequence based on a database match that is either perfect, or a single base pair edit away (substitution, deletion or insertion). Abundance thresholds for inclusion can be set by the user or automatically using per-batch negative or synthetic control samples. Output is designed for practical interpretation by non-specialists and includes a read report (ASVs with classification and counts per sample), sample report (samples with counts per species classification), and a topological graph of ASVs as nodes with short edit distances as edges. Source code available from https://github.com/peterjc/thapbi-pict/ with documentation including installation instructions.
Copy rights belong to original authors. Visit the link for more info
Podcast created by Paper Player, LLC |
Enhance your understanding of PaperPlayer biorxiv bioinformatics with My Podcast Data
At My Podcast Data, we strive to provide in-depth, data-driven insights into the world of podcasts. Whether you're an avid listener, a podcast creator, or a researcher, the detailed statistics and analyses we offer can help you better understand the performance and trends of PaperPlayer biorxiv bioinformatics. From episode frequency and shared links to RSS feed health, our goal is to empower you with the knowledge you need to stay informed and make the most of your podcasting experience. Explore more shows and discover the data that drives the podcast industry.
© My Podcast Data