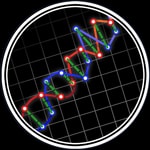
Data & Science with Glen Wright Colopy (Glen Wright Colopy)
Explore every episode of Data & Science with Glen Wright Colopy
Pub. Date | Title | Duration | |
---|---|---|---|
01 Mar 2022 | Jack Fitzsimons | Data Security, Privacy, & Artificial Intelligence | 01:14:22 | |
Dr. Jack Fitzsimons (Oblivious AI) gives a high-level introduction to the technologies that can either exploit or protect your data privacy. If you'd like to survey the landscape of data privacy-preserving technologies (from someone who's building the tech) this is a good place to start! #datascience #privacy #ai
0:00 - Coming up... 3:24 - Introduction 6:20 - Data privacy and privacy enhancing technologies 13:00 - History of privacy enhancing technologies 19:54 - Differential privacy: Hiding the influence of a single data point 22:52 - Trading data utility for data privacy 38:32 - Tracking algorithms and how they decide user preferences 42:04 - Preserving privacy: Anonymizing data & VPNs 50:17 - Exploration vs Exploitation: Combining best of multiple domains to tackle problems 54:13 - Federated learning, input and output privacy of data 58:45 - Balancing data privacy vs data-driven personalization 1:05:50 - What should data scientists/statisticians debate? | |||
02 Aug 2022 | Keith O’Rourke | The Logic of Statistics | 01:13:29 | |
Keith O'Rourke | The Logic of Statistics Dr. Keith O'Rourke talks about the logical reasoning behind statistical modeling. Topics include mathematical vs scientific reasoning, whether science has become too stats focused, and vice versa. Watch it on... Youtube: https://youtu.be/FqE4ROHBKpY Podbean: https://dataandsciencepodcast.podbean.com/e/keith-o-rourke-the-logic-of-statistics/
Topic List: 0:00 - The logic of statistics 0:30 - What is scientific statistics? 5:15 - The logic of statistics and CS Pierce 9:15 - Role of representation in statistics: explicit vs implicit 14:13 - Diagrammatic Reasoning 18:45 - Why is modeling counterfactual? 19:33 - How can statisticians become better scientists? 28:40 - Science is hard 31:24 - Computational approaches to learning 42:00 - Learning through metaphor 46:28 - Diagrammatic representations vs math 48:40 - Is science too statistics-focussed? 59:35 - Is statistics sufficiently science-focussed? 1:08:40 - Scientific Debate
#statistics #datascience #science | |||
16 Mar 2022 | Martin Goodson | Practical Data Science & The UK’s AI Roadmap | 01:16:18 | |
Martin Goodson | Practical Data Science & The UK's AI Roadmap #ai #datascience #startups Martin Goodson (Evolution AI) describes the key aspects of the UK's AI Roadmap & responses to the document by members of the Royal Statistical Society. In particular, Martin describes the disconnect between the priorities of AI startups and industry practitioners on one side, and government and academia on the other. Martin also outlines which skills early career data scientists should focus on while in school versus after entering the workforce. Also available on.... YouTube: https://youtu.be/T9qRl6Hclhg
Topic List 0:00 COMING UP: Scientific culture & AI 1:25 The UK AI Roadmap 8:44 Who is a data science “practitioner”? 12:53 Data science in AI startups 20:36 Is there a disconnect between practitioners & academia? 25:09 Key skills for new data science graduates 32:03 Coding & production level data science 39:30 Learning the right data analysis skills at the course-level. 45:32 AI leadership 58:40 AI from academia & OpenSource initiatives 1:05:37 Large institutions' impact on the AI field 1:08:24 Back to the UK AI roadmap 1:12:16 Building an AI community 1:13:15 AI in our lifetime: Moonshots & realistic goals 1:14:31 Scientific debate | |||
18 Jul 2022 | Scott Cunningham | Causal Inference (The Mixtape) | 01:20:32 | |
Scott Cunningham | Causal Inference (The Mixtape) Scott Cunningham (Baylor University) discusses the ideas of his book "Causal Inference: The Mixtape". Topics include trusting inference in the absence of counterfactuals and the challenges of apply scientific methods to social phenomena. Watch it on... YouTube: https://youtu.be/yNaCudDVTkY Podbean: https://dataandsciencepodcast.podbean.com/e/scott-cunningham-causal-inference-the-mixtape/ 0:00 - COMING UP... 0:35 - What makes it into the mixed tape? 7:10 - Coding to learn 11:15 - More people are expected to work with data & code 12:50 - Design vs program vs estimators 20:40 - Causation with zero correlation 27:00 - Optimization make everything endogenous 28:45 - The hospital example 29:30 - Credible scientific discovery vs motivated discovery 39:55 - Different meanings of causality 43:30 - The impossible counterfactual 47:00 Counterfactual nihilism 49:20 Social experiments / Defund the police 53:35 - Skepticism about the science of social phenomena 1:05:20 - The Italian crime example 1:16:30 - Scientific debate
| |||
22 Feb 2022 | Chris Tosh | The piranha problem in statistics | 01:09:41 | |
The piranha problem (too many large, independent effect sizes influence the same outcome) has received some attention on Andrew Gelman’s blog. But now it’s a paper! Chris Tosh (Memorial Sloan Kettering) talks about multiple views of the piranha problem and detecting the implausible scientific claims that are published. The butterfly effect makes an appearance. If you enjoyed the science-vs-pseudoscience topics, you’ll enjoy this one.
0:00 - Coming up in the episode 2:35 - What is the Piranha Problem? 19:54 - Confusing effect sizes 23:11 - The "words & walking speed" study 26:22 - Declaration of independent variables 30:58 - Piranha theorems for correlations 37:07 - Piranha theorems for linear regression 40:37 - Piranha Theorems for mutual information 44:13 - Bounds on the independence of the covariates 46:12 - Applying the piranha theorem to real data 50:12 - Applying the piranha theorem across studies 54:05 - A Bayesian detour 1:00:12 - The butterfly effect & chaos 1:04:26 - Applying the piranha theorem to cancer research | |||
14 Apr 2022 | Ruda Zhang | Math-Science Duality | 01:22:54 | |
Ruda Zhang | Math-Science Duality Watch it on... Youtube: https://youtu.be/GoDwen-RGZg Podbean: https://dataandsciencepodcast.podbean.com/e/ruda-zhang-math-science-duality/ Statistics is thought to reside at the interface of science and mathematics. Ruda Zhang (Duke University) discusses the friction at this interface and the role that both mathematical formalism & observational/data-driven intuition play in scientific discovery. A great topic for anyone interested in statistics' role in scientific discovery. #datascience #ai #science #mathematics Topic List 00:00 COMING UP... 2:44 Ruda Zhang's compendium of cool ideas + a Gaussian process PSA 7:08 Is intuition undervalued in scientific research? 10:16 Mathematics vs observational science. Rigor vs intuition. 14:07 Intuition & discovery precedes mathematical rigor 21:58 Mathematics vs empirical science & the complexity of induction 30:24 Abstract thinking & the cost/benefit of discovery 37:25 The efficient frontier / Pareto Front of knowledge 42:55 Pragmatism and competence 50:24 Math /science dualism 1:15:52 AI making scientific discoveries 1:19:15 Statistical & scientific debate | |||
06 Apr 2022 | Simon Mak | Integrating Science into Stats Models | 01:19:21 | |
Simon Mak | Integrating Science into Stats Models #statistics #science #ai It’s a common dictum that statisticians need to incorporate domain knowledge into their modeling and the interpretation of their results. But how deeply can scientific principles be embedded into statistical models? Prof. Simon Mak (Duke University) is pushing this idea to the limit by integrating fundamental physics, physiology, and biology into both the models and model inference. This includes Simon’s joint work with Profs. David Dunson and Ruda Zhang (also of Duke University). Scientific reasoning AND stats. What more could we ask for? Enjoy! Watch it on.... YouTube: https://youtu.be/bUbZO7R4z40 Podbean: https://dataandsciencepodcast.podbean.com/e/simon-mak-integrating-science-into-stats-models/
00:00 - COMING UP….Scientists & Statisticians 02:09 - Introduction - Integrating scientific knowledge into AI/ML 06:08 - How much domain knowledge is sufficient? 09:15 - Choosing which prior knowledge to integrate into a model 14:49 - Black box & gray box optimization 19:50 - Non-physics examples of integrating scientific theory into ML models 22:45 - Scientific principles & modeling at different scales 27:20 - Correlation is one just way of modeling linkage 36:37 - Conditional independence & different-fidelity experiments 39:40 - Innovation vs incorporation of known information in the model 42:52 - Aortic stenosis example 52:49 - Which mathematics can be used to represent scientific knowledge 57:09 - How to acquire scientific domain knowledge 1:02:45 - Complementary approaches to integrating science 1:06:48 - Gaussian process & integrating priors over functions 1:12:48 - A topic for statisticians and scientists to debate:science-based vs data-based learning. Simon Mak's Webpage: https://sites.google.com/view/simonmak/home
| |||
10 May 2022 | Ruda Zhang | Gaussian Process Subspace Regression | 01:09:22 | |
Ruda Zhang | Gaussian Process Subspace Regression Ruda Zhang (Duke University) walks us through "Gaussian Process Subspace Regression for Model Reduction" by Zhang, Mak, and Dunson. To keep the topic interesting for both the early career & advanced audience we recap key points at a high level so that no one gets lost.
This episode involves a presentation, so you may prefer to watch the YouTube version here: https://youtu.be/IPtqUUG4XcY
Ruda's website: https://ruda.city/ The paper: https://arxiv.org/abs/2107.04668 | |||
26 Jul 2022 | Jack Fitzsimons | Evil Models: Hiding Malware in Neural Networks | 00:51:30 | |
Jack Fitzsimons | Evil Models: Hiding Malware in Neural Networks Did you know that it's possible to hide malware in neural networks? Actually, you can hide malware in many statistical models. This is the subject of two recently-published papers (aptly titled "EvilModel" & "EvilModel 2.0"). Dr. Jack Fitzsimons makes it easy to understand how this is done, using techniques that began long before computers.
Watch or listen on... Youtube: https://youtu.be/QBnk8ogL8Nk Podbean: https://dataandsciencepodcast.podbean.com/e/jack-fitzsimons-evil-models-hiding-malware-in-neural-networks/ | |||
11 Jul 2022 | Eric Daza | Important Ideas in Causal Inference | 01:23:34 | |
Eric Daza | Important Ideas in Causal Inference YouTube: https://youtu.be/K5nsSMJVIT0 Andrew Gelman and Aki Vehtari wrote a paper titled, "What are the most important statistical ideas of the past 50 years?". The first idea in the list is "counterfactual causal inference". Eric Daza (Evidation Health) walks us through the main ideas of the Gelman & Vehtari paper, drawing examples from several fields, including medical & healthcare statistics. Topics 0:00 - Coming up...Correlation vs Causation 1:20 - Most important statistical ideas over the last 50 years 6:10 - Counterfactual Causal Inference 9:40 - Assumptions Change between Applied Domains 21:10 - Propensity Score Methods 25:15 - Transportability of Scientific Results 26:30 - People don't want generalizable results 32:00 - Generic Computation Algorithms 37:00 - Reweighting 43:57 - Matching Methods 58:20 - Medical Data is Higher Dimensional that we think. 1:00:15 - Is a Trial Population Representative? 1:10:35 - Causal Models in the Future 1:18:45 - Apostates Welcome 1:21:45 - Scientific Debate
| |||
19 May 2021 | Mike Evans | Statistical Reasoning & Evidence | Philosophy of Data Science Series | 01:09:46 | |
Mike Evans | Statistical Reasoning & Evidence | Philosophy of Data Science Series Mike Evans (University of Toronto) describes his approach to statistical reasoning. Mike outlines how to recognize and address problems that are statistical in nature and why these approaches should be grounded in our ability to measure statistical evidence.
Watch it on YouTube at: https://youtu.be/Q7JpGZxHxXU
0:00 Statistical Reasoning 2:30 The Basic Problem: Reasoning on Statistical Problems 13:00 Rules of Statistical Inference 19:30 Bias (The Controversial Bit?!?!) 24:10 Steps of Statistical Reasoning 25:50 Connection to Philosophy of Science 27:35 Measuring Evidence (Frequentist vs Bayesian vs Loss Function) 29:49 Problems with the p-values 32:00 Choosing & Checking Priors 49:25 Idealism, Good Plans, Bad Plans 54:45 Describing Your Reasoning 59:20 Critiques of the Principle of Evidence 1:04:00 Data-Driven Science vs Hypothesis Driven Science | |||
09 Mar 2020 | S01 Ep08 with Dana Al Sulaiman: Engineering Sensing Platforms for Biomarker Detection | 00:38:10 | |
Dana al Sulaimen’s (MIT) work runs the gamut of biomedical engineering areas. She gives a great presentation on the clinical motivation for her work, engineering sensing platforms, and data analysis. Definitely watch the video for this one for some excellent visual material. | |||
08 Jan 2020 | S00 Ep03 with Martin Ho and Greg Maislin Pt 1: MDD Section Activities | 00:20:24 | |
Part 1 of a three part episode with Martin Ho and Greg Maislin, talking about the ASA Section on Medical Devices and Diagnostics (MDD). This part discusses the MDD Section Activities.
The other two parts of this episodes will cover: Part 2: Bayesian Methods and Digital Health Initiatives Part 3: The MDD Idea Exchange and Bayesian p-values | |||
15 Jun 2020 | S01 Episode 17 with Xinyi Li Part 1: Big Data Squared - Combining Brain Imaging and Genomics for Alzheimer’s Studies | 00:25:20 | |
Xinyi continues the conversation on precision medicine research at SAMSI. Xinyi describes the challenges of combining genomic data with imaging data for modelling Alzheimer’s with the goal to supplement subjective diagnosis criteria with the more objective biomarkers.
| |||
08 Feb 2021 | Irina Gaynanova | Replicating Clinical Metrics & Innovating New Methods | 01:12:56 | |
Philosophy of Data Science Series Session 3: Data Science Highlight Reel Episode 2: Irina Gaynanova on Replicating Clinical Metrics & Innovating New Methods
Who makes it into the highlight reel of data science? Irina Gaynanova for her work on replicating clinical metrics for deployment. She then goes into how her grasp of the scientific domain helps her innovate new methods and metrics. Regardless of whether you work in the clinical domain, this is an example of rigorous scientific thinking in data science.
We're always happy to hear your feedback and ideas - just post it in the YouTube comment section to start a conversation.
Thank you for your time and support of the series! | |||
24 Feb 2020 | S01 Ep07 with Shane Burns: Data Platforms to Monitor Animal Health | 00:24:53 | |
The episode of milk and honey. Shane shows us some of the real-time data analytics platforms that track the health of dairy cows and honey bees. | |||
21 Oct 2020 | Philosophy of Data Science | S02 E01 | Round Table on Essential Reasoning Skills for Data Science | 00:53:33 | |
Philosophy of Data Science Series Session 2: Essential Reasoning Skills for Data Science Episode 1: Round Table on Essential Reasoning Skills for Data Science
Session 2 "Essential Reasoning Skills for Data Scientists" is kicking off with a roundtable discussion with Elina Vessonen (Finnish Institute for Health & Welfare), Joseph Wu (Brown University), and Huub Brouwer (Tilburg University & Utrecht University).
One of the major challenges in data science is that we use three different modes of critical reasoning (deduction, induction, and abduction) on a daily (or even hourly) basis. It's important to understand the strengths and weaknesses of each mode of reasoning so that we can apply them as appropriate. This round table will begin this conversation on the modes of reasoning and how it applies to & science and data science.
Watch it on... YouTube: https://www.youtube.com/watch?v=5bOuy6VA8Hg Podbean: https://podofasclepius.podbean.com/e/philosophy-of-data-science-s02-e01-round-table-on-essential-reasoning-skills-for-data-science/
You can join our mail list at: https://www.podofasclepius.com/mail-list
We're always happy to hear your feedback and ideas - just post it in the YouTube comment section to start a conversation.
Thank you for your time and support of the series!
0:00 Intro 0:10 Roundtable on Critical Reasoning Skills 2:50 Guest Introductions 5:48 Thesis: Data Science Use All Modes of Reasoning Daily 6:45 Taxonomy of Deduction, Induction, and Abduction 17:21 The Problem of Induction 32:18 The Problem of Induction Creeping into Deduction 36:45 Bayesian Applicability Indices and Signal Quality Indices 40:55 What is "The" Scientific Method? 44:08 What is Pseudo-Science? 47:35 Theory vs Data/Evidence 50:48 Final Remarks | |||
10 Feb 2020 | S01 Ep03 with Nick de Pennington: Ufonia’s Automated Patient Phone Screening | 00:31:29 | |
Neurosurgeon and entrepreneur Nick de Pennington talks about the importance of automating clinical tasks to help doctors focus on the most challenging cases. | |||
21 Jan 2021 | Karel Moons | Validating Medical Predictive Models | Philosophy of Data Science | 01:08:10 | |
Philosophy of Data Science Series
Session 3: Data Science Highlight Reel
Episode 2: Karel Moons on Validating Medical Predictive Models
Watch it on...
YouTube: https://www.youtube.com/watch?v=Y6Qik_5hZog
Podbean:
Who makes it into the highlight reel of data science? Karel Moons and the classic BMJ Series on validating predictive/prognostic models for the clinic. You can start reading the BMJ Series for your self here:
[1] https://www.bmj.com/content/338/bmj.b375
[2] https://www.bmj.com/content/338/bmj.b604
[3] https://www.bmj.com/content/338/bmj.b605
[4] https://www.bmj.com/content/338/bmj.b606
You can join our mail list at: https://www.podofasclepius.com/mail-list
We're always happy to hear your feedback and ideas - just post it in the YouTube comment section to start a conversation.
Thank you for your time and support of the series!
| |||
14 Jun 2021 | Eric Daza | N-of-1 Science & Causal Inference | Philosophy of Data Science | 01:12:59 | |
Interesting in Data Science? Learn Data Science and Statistics from experts as they cover key topics in the field. The Data & Science podcast focusses on teaching data scientists how to think critically in order to solve data analysis problems across various scientific domains.
Eric Daza | N-of-1 Science & Causal Inference | Philosophy of Data Science Much of our scientific inference revolves around the identification and replication of patterns in data. So what can be done when N=1? Eric Daza gives us a statistician's perspective on the ideas behind N-of-1 studies, its best examples, and strongest critiques.
0:00 - The purpose of N-of-1 & generalizability 3:30 - Successes and challenges in N-of-1 9:30 - A lightbulb moment 18:00 – Anomalies, Compliance, & Recurring Patterns 23:00 – Best Critiques of N-of-1, Safety, Efficacy 41:20 - Causal Inference 54:30 – Increasing the number of data scientists 1:03:30 – Biostatistics’ changing place in data science / statistical thinking | |||
16 Sep 2020 | Philosophy of Data Science | S01E00 | Welcome to the Series! | 00:18:49 | |
The Philosophy of Data Science Series Session 1: Scientific Reasoning for Practical Data Science Episode 0: Welcome to the Philosophy of Data Science Series!
This is our very first episode of "The Philosophy of Data Science" series on Pod of Asclepius!
We go over our plans for the series plus some thoughts on why data science is such a rich field for discussions on scientific reasoning. Your time is valuable and you deserve a good explanation of why the topics were chosen and how the series is structured to maximize learning.
Topic List 0:00 New intro jingle for the series! 0:10 Welcome to the Philosophy of Data Science Series! 1:07 Modes of reasoning 5:33 Session 1 Overview: Scientific Reasoning for Practical Data Science 10:15 Session 2 Overview: Essential Reasoning Skills for Data Science 11:32 Keynotes and Session 4 14:15 Future Sessions
Coming up next week: Critical Reasoning in Medical Machine Learning Thank you for your time and support of the series! It only gets better from here! (Seriously, it really does only get better from here. We've got Andrew Gelman coming up, plus Cynthia Rudin, Mihaela van der Schaar...) | |||
28 Oct 2020 | Philosophy of Data Science | S02 E02 | Intro to Deductive Reasoning for Data Scientists | 00:20:17 | |
Philosophy of Data Science Series Session 2: Essential Reasoning Skills for Data Science Episode 2: Intro to Deductive Reasoning for Data Scientists Watch it on... YouTube: https://youtu.be/y93D-55wgX8 Podbean: Deductive reasoning pervades statistics and data science...but how far can it get us to the right conclusion from data? Elina Vessonen (Finnish Institute of Health) gives a great 20-minute presentation reviewing the role of deduction in scientific reasoning. Elina begins with a common statistical example and then covers common deductive fallacies and their role in science. It's a short and gentle introduction to a fundamental concept. Definitely worth your time! You can join our mail list at: https://www.podofasclepius.com/mail-list We're always happy to hear your feedback and ideas - just post it in the YouTube comment section to start a conversation. Thank you for your time and support of the series!
0:00 Intro 0:18 Deduction Example in Statistics 4:05 Deductive Reasoning: Basic Concepts 6:42 Deductive Reasoning in Science 11:00 Falsification 16:05 Deductive Reasoning: A Summary | |||
03 Aug 2021 | Martin Kuldorff | Spatiotemporal Models of Disease Outbreaks | 01:08:26 | |
Note: This conversation was recorded June 25, 2021. Martin Kuldorff | Spatiotemporal Models of Outbreaks Martin Kuldorff (Harvard Medical School) talks about the integration of biological & demographic information (and general reality) in the spatiotemporal models used to detect disease outbreaks. He also discusses how these methods can be applied to non-infectious diseases like cancer. 0:00 - Spatio-temporal modeling of outbreaks 6:02 - Important features of spatio-temporal outbreak models 12:20 - Which diseases wouldn't you track for modeling? 19:02 - Multiple comparison adjustments of alarms 25:15 - Domain knowledge of outbreak features 29:30 Competing hazards & risks 34:30 Comparing hemispheres 37:00 - Bridging the gap for infectious diseases to cancer 45:10 - Retrospective data correction / changing monitoring 57:00 - Competing risks & statistics 1:01:30 - Deducing risks & affects through knowledge of immunological mechanisms 1:09:00 - Future scientific convos #datascience #science | |||
08 Jan 2020 | S00 Ep00 with Glen Wright Colopy: Welcome and hello! | 00:14:30 | |
Welcome and hello!
Glen introduces the podcast, himself, and what’s going on! | |||
10 May 2022 | Wenting Cheng & Weidong Zhang | Advances in Biotech/Biopharma | 00:34:44 | |
Wenting and Weidong discuss how the statistical challenges in the biopharm industry have proliferated with the unique demands of biotech and related life science industries. | |||
10 May 2021 | Kristin Morgan | The Data Science of Sports Injury | 01:10:41 | |
Description: In the world of biomechanics, engineers continuously aim to innovate and create new models for better understanding of their research. In this episode, Kristin Morgan (University of Connecticut) returns to the show as she explains how they use gait as a form of diagnostic tool in maximizing human performance. Having experiences on sports herself, Morgan presents how they use gait to measure recovery from physical impairment, specifically for ACL-related injuries. Aside from this, however, she also explains how they use the same tool to measure recovery from cognitive impairment. An insightful episode for all!
Keywords: biomechanics, models, metrics, gait, engineering, statistics, cognitive impairment, physical impairment
0:00 - Intro 03:01 - Creating models for performance optimization 07:23 - Why gait is an effective diagnostic tool 11:38 - Maximizing gait in creating models for post-ACLR 17:35 - Manifestation of different injuries & models 22:01 - Modeling motor control 26:28 - Applying other models in biomechanics 30:50 - Using asymmetric walking for recovery 39:30 - Understanding cognitive impairment recovery 44:19 - Moving forward with gait as diagnostic tool 45:40 - Taking inspiration from other fields / Statistics in Engineering 47:45 - Engineering and statistics hand in hand 52:50 - Limitations of modeling in biomechanics 54:20 - Starting a career in biomechanics 58:20 - Including cognitive impairment 1:00:20 - Tailoring models to specific cases 1:05:33 - Applying the models to injuries other than ACL | |||
08 Jan 2020 | S00 Ep03 Pt03 with Martin Ho and Greg Maislin: The MDD Idea Exchange and Bayesian p-values | 00:32:59 | |
Part 3 of a three part episode with Martin Ho and Greg Maislin, talking about the ASA Section on Medical Devices and Diagnostics (MDD). This part discusses the MDD Idea Exchange and Bayesian p-values. The other two parts of this episodes cover: Part 1: MDD Section Activities Part 2: Bayesian Methods and Digital Health Initiatives | |||
30 Apr 2021 | Andrew Gelman & Megan Higgs | Statistics’ Role in Science and Pseudoscience | 01:11:52 | |
Andrew Gelman & Megan Higgs | Statistics' Role in Science and Pseudoscience
#datascience #statistics #science #pseudoscience
Our science vs pseudoscience discussion continues with Andrew Gelman (Columbia) and Megan Higgs (Critical Inference LLC). Andrew and Megan describe two critical roles that statistics plays in science.... but also how statistics can add the air of scientific rigor to bad research or help statisticians fool themselves. From there the conversation goes on in a way that only a conversation with Andrew and Megan can! A very fun episode.
0:00 - Two roles of statistics in science 4:50 - Many models were intended for designed experiments 10:30 - The biggest scientific error of the past 20 years 15:00 - Feedback loop of over-confidence / Armstrong Principle 21:00 - Science is personal 25:00 - The value of different approaches / Don Rubin Story 34:40 - Statistics is the science of defaults / engineering new methods 45:00 - The value of writing what you did 52:27 - Math vs science backgrounds + a thought experiment 1:01:20 - Fooling ourselves
| |||
22 Jun 2020 | S01 Episode 17 with Xinyi Li Part 2: Big Data Squared - Combining Brain Imaging and Genomics for Alzheimer’s Studies | 00:38:49 | |
Working with brain imaging data, Xinyi has a lot of cool figures to show off in her technical presentation. She walks us through the image-on-scalar regression model and how it is used to infer a personalized “baseline” brain image along with the effects of different cognitive diagnoses. | |||
20 Jul 2020 | Are Challenge Trials Ethical for COVID-19? with Richard Yetter Chappell @Pod of Asclepius | 00:33:32 | |
13 Jul 2020 | How do you forecast the spread of COVID-19? with Lily Wang @Pod of Asclepius | 00:53:40 | |
16 Feb 2021 | Philosophy of Data Science | Step-change and Anomaly Detection | Alex Bolton | 00:59:41 | |
#datascience #ai #earlycareer Philosophy of Data Science Series Session 3: Data Science Highlight Reel Episode 4: Alex Bolton on Step-change and Anomaly Detection
Who makes it into the highlight reel of data science? Alex Bolton for doing the hard work of analyzing data to figure out exactly when things don't look "normal". We discuss the critical reasoning behind step-change detection and anomaly/novelty detection. Alex provides several real-world examples of the data and challenges. Watch it on... YouTube: https://www.youtube.com/watch?v=097FO1JDkhU Podbean: We're always happy to hear your feedback and ideas - just post it in the YouTube comment section to start a conversation. Thank you for your time and support of the series! | |||
20 Sep 2021 | Jingyi Jessica Li | Statistical Hypothesis Testing vs Machine Learning Binary Classification | 00:55:55 | |
Jingyi Jessica Li | Statistical Hypothesis Testing versus Machine Learning Binary Classification Jingyi Jessica Li (UCLA) discusses her paper "Statistical Hypothesis Testing versus Machine Learning Binary Classification". Jingyi noticed several high-impact cancer research papers using multiple hypothesis testing for binary classification problems. Concerned that these papers had no guarantee on their claimed false discovery rates, Jingyi wrote a perspective article about clarifying hypothesis testing and binary classification to scientists. #datascience #science #statistics 0:00 – Intro 1:50 – Motivation for Jingyi's article 3:22 – Jingyi's four concepts under hypothesis testing and binary classification 8:15 – Restatement of concepts 12:25 – Emulating methods from other publications 13:10 – Classification vs hypothesis test: features vs instances 21:55 - Single vs multiple instances 23:55 - Correlations vs causation 24:30 - Jingyi’s Second and Third Guidelines 30:35 - Jingyi’s Fourth Guideline 36:15 - Jingyi’s Fifth Guideline 39:15 – Logistic regression: An inference method & a classification method 42:15 – Utility for students 44:25 – Navigating the multiple comparisons problem (again!) 51:25 – Right side, show bio-arxiv paper | |||
16 Nov 2020 | Philosophy of Data Science | S01 E04 | Values and Subjectivity in Data Science | 01:17:30 | |
Philosophy of Data Science Series Session 1: Scientific Reasoning for Practical Data Science Episode 4: Values and Subjectivity in Data Science
The Value-Free Ideal is a central tenant of objective science. But how do values, value judgements, and subjectivity leak into the practice of data science and statistics. To what extent is it desirable for science to be informed by values? Kevin Zollman (Carnegie Mellon University) covers the range of key ideas, from Heather E. Douglas to W.E.B. du Bois.
Watch it on... YouTube: https://youtu.be/9USkWtX-ydc Podbean: https://podofasclepius.podbean.com/e/philosophy-of-data-science-s01-e04-values-and-subjectivity-in-data-science/
You can join our mail list at: https://www.podofasclepius.com/mail-list
We're always happy to hear your feedback and ideas - just post it in the YouTube comment section to start a conversation.
Thank you for your time and support of the series!
0:00 Intro 0:03 Welcome Kevin Zollman (Carnegie Mellon University)! 1:44 Is Science Value-Free? 6:08 How might values affect science? 9:00 Choice of Research Problem 10:45 Loss Functions 18:34 Choice of Variables 24:10 Choice of Statistical Model 29:30 Minimizing the Values in Science (W.E.B. du Bois) 35:20 Philosopher in Science 41:20 Statements on Generalizability 47:45 Clarifying Subjective Choices 52:45 Conflicts between Scientific Disciplines 61:18 Scientific Value Judgments & Self Correcting Science 67:50 Choice in Metrics and Research Focus 70:30 Concluding Ideas | |||
05 May 2021 | Michael McRoberts | Football Analytics and Data-Driven Decisions | 01:14:40 | |
Michael McRoberts | Football Analytics and Data-Driven Decisions
Michael McRoberts (Championship Analytics Inc.) uses Monte Carlo simulations to provide strategy analytics to college and NFL football teams. Topics include communicating data-driven recommendations, the need to create counterfactual data, and asymmetric decision rewards.
0:00 The challenge of sports analytics 5:00 Analytics recommendations 16:00 Communicating data-driven recommendations 24:35 Vegas Odds & Ancillary Data 30:00 Football is way behind / Data science projects with a "runway" 41:25 Creating experiments and counterfactuals 49:30 Implementing data science insights 56:15 Asymmetric decision rewards 58:50 How to start in sports analytics 1:10:00 Data science vs analytics vs statistics | |||
18 May 2020 | S01 Episode 14 Part 1 with Mike McArdle: Virtual and Augmented Reality for the Life Sciences | 00:27:15 | |
Mike McArdle, co-founder and Chief Product Officer at Lucid Dream VR, breaks down the key technological factors that have led to the rapid increase in VR and AR solutions for the life sciences. He then walks us through two products helping companies and hospitals to accelerate training and talent development on their staff. | |||
04 Mar 2020 | S01 Ep08 with Mona Kanaan and Ada Keding: What is a Stepped-Wedge Trial? | 00:32:50 | |
10 Jan 2020 | S00 Ep04 Pt 1 with Allison Meisner: Winning the ASA Student Paper Competition and Research Overview | 00:24:46 | |
S00 Ep04 Pt01 with Allison Meisner: Predictive Models in Kidney Injury This is Part 1 of a two-part episode in which Allison talks about her background, research interests and the research that earned her a win in the ASA Student Paper Competition in 2017. In Part 2 she will treat the audience to a technical deep-dive into optimizing bespoke clinical models. | |||
08 Jan 2020 | S00 Ep01 Pt2: A Nifty Statistical Approach to Vital-Sign Artefact Detection | 00:34:33 | |
This episode is Part 2 of a two part episode with yours truly, discussing personalized probabilistic patient monitoring. In this part, Glen describes a nifty statistical approach to vital-sign artefact detection. In the first part of this 2 part episode, Glen talks about using Gaussian Processes for identifying the deteriorating patient. | |||
01 Dec 2020 | Philosophy of Data Science | Deborah Mayo | Philosophy of Science & Statistics | 00:41:11 | |
Philosophy of Data Science | Keynote 1 Presentation | Philosophy of Science & Statistics Philosophy of Data Science Series Keynote with Deborah Mayo Episode 2: The Philosophy of Science & Statistics In the first keynote of the Philosophy of Data Science Series we have a 2-part interview with Deborah Mayo (Virginia Tech). In the second part of our keynote, Deborah Mayo covers the interplay between scientific and statistical philosophy. Deborah highlights some common scientific fallacies, along with suggestions of where statistical thinking can be made more rigorous. Watch it on... YouTube: https://youtu.be/9GGAXZ6htrA Podbean: https://podofasclepius.podbean.com/e/philosophy-of-data-science-keynote-1-presentation-philosophy-of-science-statistics/ You can join our mail list at: https://www.podofasclepius.com/mail-list We're always happy to hear your feedback and ideas - just post it in the YouTube comment section to start a conversation. Thank you for your time and support of the series! | |||
27 Apr 2021 | Irina Gaynanova | Replicability, Reproducibility, Responsibility, and Optimism for the Future of Science | 01:02:49 | |
Irina Gaynanova (Texas A&M) describes why she thinks that replicability is a prerequisite for reproducibility in science and how scientists can (personally) start improving the replicability of research. We also discuss how the concepts of replicability/reproducibility can differ according to the domain-specific context and the methods used. Please forward to any students or colleagues who would find this of interest! | |||
14 Jan 2020 | S01 Ep01 with Jeroen Bergmann and Daniel Mogefors: Needs-led Innovation at Oxford University | 00:23:19 | |
Originally developed in the Stanford biodesign ecosystem, the “needs-led” approach to healthtech innovation has rapidly become a key philosophy for those wanting to develop a viable healthcare solution. Prof. Jeroen Bergmann and Daniel Mogefors from the Oxford Healthtech Labs break down the key aspects of needs-led innovation and how researchers at Oxford University are using it | |||
04 Feb 2022 | Philosophy of Data Science | Deborah Mayo | Revolutions, Reforms, and Severe Testing in Statistical Thinking | 00:53:57 | |
Philosophy of Data Science Series Keynote with Deborah Mayo Episode 1: Revolutions, Reforms, and Severe Testing in Statistical Thinking In the first keynote of the Philosophy of Data Science Series we have a 2-part interview with Deborah Mayo (Virginia Tech). In the first part of our keynote with Deborah Mayo we cover... - The role of scientific revolution and its implications for statistics and data scientist. - The necessity of statistical reforms and why philosophy will play a role. - The value of severe testing of scientific claims. Watch it on... YouTube: https://youtu.be/S4VAEShM3BU Podbean: You can join our mail list at: https://www.podofasclepius.com/mail-list We're always happy to hear your feedback and ideas - just post it in the YouTube comment section to start a conversation. Thank you for your time and support of the series!
Topics: 0:00 - Preface to First Keynote Interview 2:00 - Welcome Deborah Mayo! 5:05 - What is the Philosophy of Statistics? 8:15 - What does philosophy add to data science? 16:10 - Scientific revolution in statistics 20:10 - Statistical reforms 24:25 - Replication & hypothesis pre-specification 31:00 - Failure is severe testing 37:25 - Error statistics 48:00 - Scientific progress and closing remarks | |||
21 Jul 2020 | RelationalAI: Building a Knowledge Graph Database with Julia | Nathan Daly and Molham Aref@POd of Asclepius | 00:41:24 | |
Molham Aref and Nathan Daly describe their experience using Julia to build a next-generation knowledge graph database that combines reasoning and learning to solve problems that have historically been intractable. They explain how Julia's unique features enabled them to build a high-performance database with less time and effort. Both Nathan and Molham with be speaking at JuliaCon 2020 at the end of July. It's free and online, so there's no reason not to attend. You can register for JuliaCon 2020 here: https://juliacon.org/2020/
0:00 Intro 1:25 RelationalAI 3:25 Advantages of Julia as a foundation 4:21 "Full stack" data science 5:38 Advantages of Julia in the tech stack 6:30 Technical requirements of RelationalAI 7:45 Advantages of Julia (cont.) 10:00 Data munging, preprocessing, and transparency 14:30 Advantages of Julia (cont.) 18:35 RelationalAI's Innovation 22:00 Data Analysis and taking computational efficiency for granted 23:38 Who are the users of RelationalAI? 25:45 What are "knowledge graphs"? 28:30 Knowledge graphs for AI and Software 2.0 32:43 Julia as "executable math" 34:10 "Multiple dispatch" in a nutshell 36:20 Julia in the scientific community 38:53 See Nathan and Molham again at JuliaCon 2020 | |||
08 Apr 2021 | Science vs Pseudoscience | Dien Ho | Philosophy of Data Science | 00:58:54 | |
We have a new series that centers on the discussion of science vs. pseudoscience. Guests of different backgrounds share their insights on what really constitutes science and the highly-contested pseudoscience. In today’s episode, we talk to Professor Dien Ho, PhD, a Professor of Philosophy and Healthcare Ethics, of the Massachusetts College of Pharmacy & Health Science University. Discover how philosophical ideas and theories are applied in hopes of understanding what really counts as science and what pseudoscience really is.
00:03 - Introductions 5:33 - What is pseudoscience? 08:53 - Legitimacy of other sciences 12:11 - What qualifies as science? 19:00 - Inductivism and empirical falsifiability 26:22 - Positivism and the importance of assumptions 31:36 - Assumptions and observations for data scientists 42:34 - The pursuit of science 49:17 - Scientism and revolutionary scientists 54:43 - Pinning down what science is | |||
08 Jun 2020 | S01 Episode 16 with John Nardini Part 2: Machine Learning and Mathematical Modeling of Wound Healing | 00:23:38 | |
John is back to show the how machine learning can vastly speed up the selection of mathematical models. His presentation provides great visual intuition on how machine learning methods can help select mathematical models, even as measurement noise increases. It’s a huge improvement over selecting models by hand! | |||
02 Nov 2020 | Philosophy of Data Science | S02 E03 | Intro to Inductive Reasoning for Data Scientists | 00:14:59 | |
Philosophy of Data Science Series Session 2: Essential Reasoning Skills for Data Science Episode 3: Intro to Inductive Reasoning for Data Scientists Watch it on... YouTube: https://youtu.be/lNOUvOUE_KE Podbean: https://podofasclepius.podbean.com/e/philosophy-of-data-science-s02-e03-intro-to-inductive-reasoning-for-data-scientists/ New episodes of the Philosophy of Data Science Series will now be published on Mondays! Today's episode is a short introduction to a fundamental concept. Definitely worth your time! Inductive reasoning is the fundamental challenge to scientific rigor. Induction is baked into methods like K-fold cross validation or generalizing from a sample to a population. However, many statisticians and data scientists are unfamiliar with the term and its implications. Joseph Wu (Brown University) gets us up-to-speed with a 10-minute presentation on the fundamental role of induction in scientific reasoning. You can join our mail list at: https://www.podofasclepius.com/mail-list We're always happy to hear your feedback and ideas - just post it in the YouTube comment section to start a conversation. Thank you for your time and support of the series! Outline 0:00 Intro 0:18 Inductive vs Deductive Reasoning 2:35 Overview of Induction, Deduction, and Abduction 3:23 Types of Induction: Everyday Life vs Statistical Generalizations 5:25 Sample to Population Induction 6:48 Population to Individual Induction 9:35 The Problem of Induction 11:52 Induction: Fallible but Powerful | |||
06 Apr 2020 | S01 Ep11 with Dave Hunter: Disease Network Modeling, Mixture Models, & Career Opportunities at SDSS 2020 | 00:30:02 | |
Dave Hunter highlight a variety of cool life science collaborations he has worked on, including the network models used to describe AIDS transmissions and mixture modelling to describe pediatric cognitive tests. We then talk about the upcoming SDSS 2020 conference, and its newest additions to benefit early career researchers. | |||
09 Sep 2020 | Innovative Trial Design & Master Protocols: Lisa Lavange | Pod of Asclepius | 00:44:50 | |
Lisa LaVange (Gillings School of Global Public Health at the University of North Carolina at Chapel Hill) was the 2018 American Statistical Association (ASA) president and the director of the Office of Biostatistics in the Center for Drug Evaluation and Research (CDER) at the FDA. She give a high-level overview of issues surrounding Innovative Trial Design and Master Protocols. A great listen for anyone wanting to be introduced to the subject or (for those already familiar) interested in its growing breadth of applications. #datascience #statistics #biopharm #pharma #FDA | |||
20 Apr 2020 | S01 Episode 12 with Paul Elbers: AmsterdamUMCdb, Europe’s first open ICU database | 00:52:48 | |
It’s not everyday that medical researchers give the world access to 13+ years of dense, high-quality critical care data. Intensivist Paul Elbers describes the data set along with the clinical priorities in collecting the data. Paul covers a range of topics including protecting the patients’ interests and anonymity, a clinician’s priorities when selecting clinical performance metrics, and the stages of validating predictive algorithms up to the stage of an RTC. The work done to create AmsterdamUMCdb is an incredible feat and a huge boon to the medical science profession. | |||
12 Feb 2020 | S01 Ep05 with Gajanan Bhat and Xinping Cui: Leveraging Data for Clinical Development & the OC Biostatistics Symposium | 00:30:07 | |
Gajanan Bhat and Xinping Cui discuss the major themes of data science in clinical drug and device development.
| |||
23 Sep 2020 | Philosophy of Data Science | S01 E01 | Critical Reasoning in Medical Machine Learning | 00:56:32 | |
Philosophy of Data Science Series Session 1: Scientific Reasoning for Practical Data Science Episode 1: Critical Reasoning in Medical Machine Learning
Data science in medicine and healthcare requires not only algorithmic and statistical knowledge but also a strong appreciation of the clinical environment in which (i) the data is being collected and (ii) the algorithm will be used. I'll showcase a scenario where a machine learning system failed to perform a "simple" clinical task and how critical reasoning was used to resolve the problem. Guest-host Kristin Morgan (University of Connecticut) joins us to lead the discussion in how this example is applicable to the broader field of biomedical data science. This is... Session 1: Scientific Reasoning for Practical Data Science Episode 1: Critical Reasoning in Medical Machine Learning Watch it on... YouTube: https://youtu.be/o5YmdoCiyug Podbean:
Coming up next week: Applying Scientific Reasoning to Statistical Practice with Andrew Gelman (Columbia University) We're always happy to hear your feedback and ideas - just post it in the YouTube comment section to start a conversation. Thank you for your time and support of the series! | |||
29 May 2020 | S01 Episode 15 with Rita Hendricusdottir: Oxford Global Guidance to Navigate Medical Device Regulations | 00:12:09 | |
Rita Hendricusdottir (Department of Engineering Science, University of Oxford) show cases a new tool to help innovators quickly assess the regulatory buden of their medical devices. From answering the simple question of “Is my invention a medical device?” to the complex considerations for “which classification is my device?” the Oxford Global Guidance tool is designed to facilitate this initial evaluation. | |||
13 May 2021 | Deborah Mayo | Statistics & Severe Testing vs Pseudoscience | 01:35:29 | |
Deborah Mayo | Statistics & Severe Testing vs Pseudoscience Watch it on… YouTube Podbean
In our fourth episode of the “science vs pseudoscience” mini-series, Deborah Mayo (Virginia Tech) specifies several necessary criteria to be scientifically rigorous. She gives several examples of how statistical thinking is essential to scientific thinking and why she believes that the “I’ll know it when I see it” approach to delineating science from pseudoscience is not a good approach.
Looking to catch up with the earlier “Science vs Pseudoscience” episode? You can watch them here: Intro Episode 1 Episode 2 Episode 3
| |||
02 Dec 2021 | Eric Schwitzgebel | Consciousness, Zombies, & First Person Data | Philosophy of Data Science | 01:13:24 | |
The philosophical community continuously aims to reconcile differing views on first person data and the consciousness of the mind. Is it possible to live without consciousness? Can one conceive thoughts without matching images to them? In this episode, Eric Schwitzgebel of the University of California tries to dissect such topics and questions to help us better understand the philosophical world.
Keywords: philosophy, epistemic data, first person data, stimulus error, imageless thought, consciousness
| |||
27 Jan 2020 | S01 Ep02 with Niven Narain: Berg Health’s Data Platforms and Pharmaceutical Innovation | 00:16:04 | |
Niven Narain, CEO of Berg Health, discusses creating value through data platforms and AI in the pharmaceutical industry. | |||
09 Feb 2022 | Chris Holmes | AI, Digital Health, & The Alan Turing Institute | 01:03:37 | |
Chris Holmes is Professor of Biostatistics at the University of Oxford and Programme Director for Health and Medical Sciences at The Alan Turing Institute. Chris’ research interests include Bayesian nonparametrics (which is the right kind of nonparametrics), statistical machine learning, genomics, and genetic epidemiology. 0:00 - Intro 1:38 - Chris Holmes, Professor of Biostatistics at Oxford University 3:28 - UK Biobank & designing a valuable dataset 8:42 - Healthcare charities in the UK 11:16 - Digital Health: prioritizing research questions 19:55 - Bayes, nonparametrics, and Bayesian nonparametrics 23:30 - Model prediction is at the heart of Bayesian inference 28:00 - Prioritization in model building for biology 33:09 - Model constraints to generate valid inference 37:34 - Hypothesis driven science in statistical learning versus deep learning 43:30 - Developing models in genomics & clinical informatics 48:37 - Building stable, generalizable and robust models 52:41 - Important questions to think about 54:05 - Causal reasoning and clinical risk prediction 57:50 - What topic should the statistical community debate?
| |||
30 Sep 2020 | Philosophy of Data Science | S01 E02 | Scientific Reasoning for Practical Data Science | 00:55:09 | |
Philosophy of Data Science Series Session 1: Scientific Reasoning for Practical Data Science Episode 2: Scientific Reasoning for Practical Data Science Scientific reasoning plays an essential role in data science and statistics, both for developing new methods and applying our methods to real-world problems. In Session 1's titular episode, Andrew Gelman talks through the role of scientific thinking in his approach to data analysis. He also highlights the good ideas that have been generated by the wider statistical community. Watch it on... YouTube: https://youtu.be/R6mq5Esjzfw
Coming up next week: Communicating the Science in Data Science with Kathy Ensor (Rice University & 2022 ASA President)
We're always happy to hear your feedback and ideas - just post it in the YouTube comment section to start a conversation.
Thank you for your time and support of the series! You can join our mail list at: https://www.podofasclepius.com/mail-list
#datascience #statistics #machinelearning #ai #science #stem | |||
23 Mar 2020 | S01 Ep10 with David Madigan and Demissie Alemayehu: Risks and Opportunities of AI in Clinical Drug Development | 00:54:04 | |
There are many places in which ML/AI methods can be of benefit to pharmaceutical research (several have already been covered on the show). David and Demissie explain where AI can fit in to in vivo studies, which carries it’s own benefits, but also with heightened risk to to human test subjects. They go on to cover several other areas of interest including AI for observation studies and real world evidence. It’s a “big tent” conversation as we lead up to the Pfizer/ASA/Columbia University Symposium on Risks and Opportunities of AI in Clinical Drug Development. Mihaela van der Schaar will be following up in a subsequent episode on this subject. | |||
01 Jun 2021 | Edward McFowland III | Anomalous Pattern Detection & Model Building | 01:02:56 | |
#datascience #statistics Edward McFowland III | Anomalous Pattern Detection & Model Building Edward McFowland III (Harvard Business School) describes the differences between "anomalies" and "anomalous patterns". Edward describes how this informs modeling strategies, in particular, when to use an off-the-shelf model versus building a bespoke model from scratch. He then covers how to draw inspiration from different scientific and technical fields. 0:00 Edward: Live in Conference 2:00 Outliers vs Anomalies vs Anomalous Patterns 9:30 Strategy to Identify Anomalous Data Patterns 19:15 Adding Complexity to Models 25:00 Building Blocks vs Comprehensive Models 39:05 New Pieces of Evidence 40:40 Deciding Data Science Strategies 52:30 Connecting the Technical Dots 58:40 Interdisciplinary Interests | |||
17 Aug 2021 | David Dunson | Advancing Statistical Science | Philosophy of Data Science | 01:17:27 | |
David Dunson | Advancing Statistical Science | Philosophy of Data Science Series A fundamental question in the philosophy of science is "what does it mean to make scientific progress?" We will have a series of episodes centered around this question for statistics and data science. In our first episode in the series, David Dunson (Duke University) discusses important advances in Bayesian analysis, big data, uncertainty, and scientific discovery. Topic Timestamps 0:00 Intro to David Dunson 1:54 What does it mean to advance data science and statistics? 6:14 Industry & Optimization, Science & Uncertainty 8:14 Prediction & Discovery / Bayesian Modeling 14:13 What is “complex” data? 22:49 Big Data, Bayes, and Nonparametrics 33:50 Ad hoc approaches vs principled methods 37:08 Should Machine Learning Publications Refocus on Scientific Discovery? 39:50 Mathematically principled data science & statistics 51:40 Do Bayesians just use priors as regularizers? 55:16 Bayesian Priors and Tuning Inference Methods 1:00:00 Prioritize the Most Important Work in Data Science 1:07:07 Good Practices of Star Grad Students 1:13:17 The Science in Statistical *Science* #datascience #science #statistics | |||
01 Feb 2022 | Charlotte Deane | Bioinformatics, Deepmind’s AlphaFold 2, and Llamas | 01:16:45 | |
Charlotte Deane | Bioinformatics, Deepmind's AlphaFold 2, and Llamas #datascience #ai Charlotte Deane (Oxford University) talks about statistical approaches to bioinformatics, the evolution of Google Deepmind's AlphaFold 2 & its place in protein informatics deep learning landscape. She also describes humanizing antibodies, and the increasing role of software engineers in statistical research groups. The topic of llamas, camels, and alpacas (and their unique place in proteomics research) makes a surprise visit. [Note: This episode was originally published in January 2022, but the file contained a buffering error, which prevented the full interview from being played. This version, published Feb 1, 2022 contains the full interview.] Topics 0:00 Intro / An important topic to debate 3:50 What is a protein? Why are proteins foundational? 13:32 Immunotherapies, humanizing antibodies, & creating an scientific databases 16:04 Translating in silico research into immunotherapies 21:03 Nanobodies, camels, alpacas, & llamas. 25:05:00 Databases and data knowledge bases 33:21:00 Targeted therapies 39:45:00 Statistical modeling in proteomics 45:40:00 DeepMind AlphaFold's evolution 55:28:00 Software engineers in academic research groups 1:03:21 The adventure of science 1:07:42 Oxford Blues hockey & scientific debate | |||
10 Jan 2020 | S00 Ep04 Pt 2 with Allison Meisner: Technical Deep-dive Into Optimizing Bespoke Clinical Models | 00:22:53 | |
This is Part 2 of a two-part episode in which Allison treats the audience to a technical deep-dive into optimizing bespoke clinical models. In Part 1 of the episode Allison talked about her background, research interests and the research that earned her a win in the ASA Student Paper Competition in 2017. | |||
11 Feb 2020 | S01 Ep04 with Eric Stephens: Hospital Analytics & CSP 2020 | 00:28:06 | |
Eric Stephens, Chief Analytics Officer at Nashville General Hospital, talks about building analytics capacities in the hospital setting and how hospitals select their priorities for new analytics projects. Then he discusses the cool events coming up at CSP 2020 and how applied data scientists have more options than ever for career advancement. | |||
01 Jun 2020 | S01 Episode 16 with John Nardini Part 1: Machine Learning and Mathematical Modeling of Wound Healing | 00:34:11 | |
John discusses his work in the precision medicine program at the Statistical and Applied Mathematical Sciences Institute (SAMSI) to model wound healing. He describes the physiological mechanisms of wound healing and how to select a applications that are appropriate for mathematical modelling. | |||
19 Feb 2020 | S01 Ep06 with Rob Scott: What are a Clinician’s Priorities for Data-Driven Medicine? | 00:26:50 | |
Rob Scott, Chief Medical Officer at AbbVie, discusses the importance of a clinician’s perspective for keeping clinical trial development focussed on the patients. Rob talks about how the role of a Chief Medical Officer changes between a large pharma company and small biotechs. He then covers the key areas in which new developments in healthcare technology can help us better understand a patient’s response to therapies and interventions. | |||
08 Jan 2020 | S00 Ep02 with Emma Hughes: An Introduction to the IET Innovation Management Technical Network and Innovation in the NHS | 00:25:40 | |
Emma Hughes discusses how to find and cultivate technical entrepreneurial talent. She then talks about the critical challenge of making technical solutions sufficiently robust for clinical implementation. | |||
08 Jan 2020 | S00 Ep03 Pt2 with Martin Ho and Greg Maislin: Bayesian Methods and Digital Health Initiatives | 00:26:19 | |
Part 2 of a three part episode with Martin Ho and Greg Maislin, talking about the ASA Section on Medical Devices and Diagnostics (MDD). This part discusses Bayesian Methods and Digital Health Initiatives. The other two parts of this episodes cover: Part 1: MDD Section Activities Part 3: The MDD Idea Exchange and Bayesian p-values | |||
09 Nov 2021 | Mine Çetinkaya-Rundel | Advancing Open Access Data Science Education | 01:20:55 | |
Mine Çetinkaya-Rundel | Advancing Open Access Data Science Education #datascience #statistics #education Mine Çetinkaya-Rundel (Duke University) describes the current and future states of statistics and data science education. Then she discusses the process of building open access learning material.
0:00 - Introduction 1:40 - Prioritizing topics in curricula 9:07 - Teaching with intent to test 11:22 - Statistics without computing 17:52 - What should be taught? How do we teach it? 19:07 - Computational thinking is valuable (to 31:45) 23:47 - Self reinforcing academics / positive feedback (to 31:45) 31:08 - Data science vs statistics (the computing angle) 37:55 - Statistical collaboration / technical collaboration 39:45 - Common language / imputation under ignorance 41:12 - Are some topics better for hands on or computational learning? 45:32 - Learning computation through visualization 52:40 - Video cut option before she gives an example 52:42 - Let them eat cake first. 56:08 - What is open source education? Open source vs open access. 59:36 - Advancing open source text books 1:03:55 - Economics of open source 1:07:55 - The open education ecosystem 1:12:17 - Modularizing & parallelizing learning topics 1:16:52 - Favorite dataset on OpenIntro.Org? 1:18:14 - What topic should the statistics community debate? | |||
30 Aug 2021 | Gualtiero Piccinini | What Are First-Person Data? | Philosophy of Data Science | 00:51:58 | |
Gualtiero Piccinini | What Are First-Person Data? First-person methods (and its associated data) have been scientifically and philosophically contentious. Are they pseudoscientific? Or simply pushing the bounds of scientific methodology? Obviously, I have no idea… so Prof. Gualtiero Piccinini (University of Missouri – St. Louis) provides a helpful introduction to the topic covering the key points of its history and the philosophical/scientific debate. 0:00 Why cover first-person methods & data? 2:26 First-person methods vs first-person data? 7:10 Are first-person data legitimate at all? 11:50 Phenomenology 13:26 First-person data is extracted from human behavior 18:25 Skepticism & arguments against first-person data 25:40 Psychophysics, introspectionists, behavioralists, cognitivists, and the origins of first-person data 35:20 Using new instruments & methods in science 46:00 Is this where the philosophers roam? #datascience #statistics #science | |||
19 Jul 2021 | Jason Costello | Data Science vs Software, Academia vs Industry | 01:08:40 | |
Interested in Data Science? Learn Data Science and Statistics from experts as they cover key topics in the field. The Data & Science podcast focusses on teaching data scientists how to think critically in order to solve data analysis problems across various scientific domains.
Jason Costello | Data Science vs Software, Academia vs Industry Jason Costello (Hypervector) describes his (non-trivial) transition from academic research into big tech and then the healthcare industry. He outlines a strategy to find the cool research problems that you get in academia while still delivering value to your company. We then talk about the interface of data science / machine learning and software.
0:00 Deploying Data Science into the Real World 8:24 Transitioning from Academic to Industrial Data Science 16:56 First step to delivering value to industry 21:38 Toy example of high value data science 25:28 Deep technical challenges are real and useful too! 29:59 Formalized logic in machine learning solutions 32:54 Data Science & Machine Learning Projects can fail. 38:50 Getting to the cool data science projects 47:21 Putting Machine Learning Models into Software 56:21 Software and Deduction, Machine Learning and Induction 1:06:06 Is Software A Deductive Complex System?
| |||
09 Nov 2020 | Philosophy of Data Science | S02 E04 | Intro to Abductive Reasoning for Data Scientists | 00:20:12 | |
Philosophy of Data Science Series Session 2: Essential Reasoning Skills for Data Science Episode 4: Intro to Abductive Reasoning for Data Scientists Watch it on... YouTube: https://youtu.be/SzQn9SPVhRU Podbean: https://podofasclepius.podbean.com/e/philosophy-of-data-science-s02-e04-intro-to-abductive-reasoning-for-data-scientists/ The third and final of our (planned) short tutorials on key modes of critical reasoning. Abduction is common called "inference to the best explanation"...so it's easy to see why this concept is important for data scientists. Huub Brouwer (Utrecht University) walks us through a brief tutorial on how even a world-famous infer-er can get this wrong and how data scientists can avoid the same mistake. You can join our mail list at: https://www.podofasclepius.com/mail-list We're always happy to hear your feedback and ideas - just post it in the YouTube comment section to start a conversation. Thank you for your time and support of the series! 0:00 Intro 0:18 Example of Abduction in Action 4:55 Definition of Abduction 6:21 Applying Abductive Reasoning 8:35 Why is Abduction Not Deduction? 14:55 Abduction in Data Sciences 17:40 Conclusion | |||
25 May 2020 | S01 Episode 14 Part 2 with Mike McArdle: Virtual and Augmented Reality for Medical Training | 00:22:11 | |
Mike McArdle, co-founder and Chief Product Officer at Lucid Dream VR, is back to walk us through applications of VR that helps clinicians train for rare events and better understand the patient’s experience. | |||
15 Dec 2020 | Philosophy of Data Science | S3 E1 | NeuralNets, GANs, Causality, and Medicine | 01:03:28 | |
Philosophy of Data Science Series Session 3: Data Science Highlight Reel Episode 1: Adler Perotte on NeuralNets, GANs, Causality, and Medicine Watch it on... YouTube: https://www.youtube.com/watch?v=DOf2lVHzZS4 Podbean: https://podofasclepius.podbean.com/e/philosophy-of-data-science-s3-e1-neuralnets-gans-causality-and-medicine/ Who makes it into the highlight reel of data science? Adler Perotte, because he's a clear thinker on why his data needs a specific type of analysis. In this case, it's the need to draw causal inferences from observational data. Go, GANS! Go! You can join our mail list at: https://www.podofasclepius.com/mail-list We're always happy to hear your feedback and ideas - just post it in the YouTube comment section to start a conversation. Thank you for your time and support of the series! |