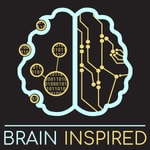
Brain Inspired (Paul Middlebrooks)
Explore every episode of Brain Inspired
Pub. Date | Title | Duration | |
---|---|---|---|
15 May 2020 | BI 070 Bradley Love: How We Learn Concepts | 01:47:07 | |
Brad and I discuss his battle-tested, age-defying cognitive model for how we learn and store concepts by forming and rearranging clusters, how the model maps onto brain areas, and how he's using deep learning models to explore how attention and sensory information interact with concept formation. We also discuss the cognitive modeling approach, Marr's levels of analysis, the term "biological plausibility", emergence and reduction, and plenty more. Notes:
| |||
25 May 2020 | BI 071 J. Patrick Mayo: The Path To Faculty | 01:10:57 | |
Patrick and I mostly discuss his path from a technician in the then nascent Jim DiCarlo lab, through his graduate school and two postdoc experiences, and finally landing a faculty position, plus the culture and issues in academia in general. We also cover plenty of science, like the role of eye movements in the study of vision, the neuroscience (and concept) of attention, what Patrick thinks of the deep learning hype, and more. But, this is a special episode, less about the science and more about the experience of an academic neuroscience trajectory/life. Episodes like this will appear in Patreon supporters' private feeds from now on. Show notes:
| |||
01 Jun 2020 | BI 072 Mazviita Chirimuuta: Understanding, Prediction, and Reality | 01:18:53 | |
Mazviita and I discuss the growing divide between prediction and understanding as neuroscience models and deep learning networks become bigger and more complex. She describes her non-factive account of understanding, which among other things suggests that the best predictive models may deliver less understanding. We also discuss the brain as a computer metaphor, and whether it's really possible to ignore all the traditionally "non-computational" parts of the brain like metabolism and other life processes. Show notes:
| |||
10 Jun 2020 | BI 073 Megan Peters: Consciousness and Metacognition | 01:25:10 | |
Megan and I discuss her work using metacognition as a way to study subjective awareness, or confidence. We talk about using computational and neural network models to probe how decisions are related to our confidence, the current state of the science of consciousness, and her newest project using fMRI decoded neurofeedback to induce particular brain states in subjects so we can learn about conscious and unconscious brain processing. Notes:
| |||
16 Jun 2020 | BI 074 Ginger Campbell: Are You Sure? | 01:22:35 | |
Ginger and I discuss her book Are You Sure? The Unconscious Origins of Certainty, which summarizes Richard Burton's work exploring the experience and phenomenal origin of feeling confident, and how the vast majority of our brain processing occurs outside our conscious awareness. | |||
24 Jun 2020 | BI 075 Jim DiCarlo: Reverse Engineering Vision | 01:16:03 | |
Jim and I discuss his reverse engineering approach to visual intelligence, using deep models optimized to perform object recognition tasks. We talk about the history of his work developing models to match the neural activity in the ventral visual stream, how deep learning connects with those models, and some of his recent work: adding recurrence to the models to account for more difficult object recognition, using unsupervised learning to account for plasticity in the visual stream, and controlling neural activity by creating specific images for subjects to view. Notes:
| |||
04 Jul 2020 | BI 076 Olaf Sporns: Network Neuroscience | 01:45:57 | |
Olaf and I discuss the explosion of network neuroscience, which uses network science tools to map the structure (connectome) and activity of the brain at various spatial and temporal scales. We talk about the possibility of bridging physical and functional connectivity via communication dynamics, and about the relation between network science and artificial neural networks and plenty more. Notes: | |||
14 Jul 2020 | BI 077 David and John Krakauer: Part 1 | 01:33:04 | |
David, John, and I discuss the role of complexity science in the study of intelligence. In this first part, we talk about complexity itself, its role in neuroscience, emergence and levels of explanation, understanding, epistemology and ontology, and really quite a bit more. Notes:
| |||
17 Jul 2020 | BI 078 David and John Krakauer: Part 2 | 01:14:37 | |
In this second part of our conversation David, John, and I continue to discuss the role of complexity science in the study of intelligence, brains, and minds. We also get into functionalism and multiple realizability, dynamical systems explanations, the role of time in thinking, and more. Be sure to listen to the first part, which lays the foundation for what we discuss in this episode. Notes:
| |||
27 Jul 2020 | BI 079 Romain Brette: The Coding Brain Metaphor | 01:19:04 | |
Romain and I discuss his theoretical/philosophical work examining how neuroscientists rampantly misuse the word "code" when making claims about information processing in brains. We talk about the coding metaphor, various notions of information, the different roles and facets of mental representation, perceptual invariance, subjective physics, process versus substance metaphysics, and the experience of writing a Behavior and Brain Sciences article (spoiler: it's a demanding yet rewarding experience).
| |||
06 Aug 2020 | BI 080 Daeyeol Lee: Birth of Intelligence | 01:31:09 | |
Daeyeol and I discuss his book Birth of Intelligence: From RNA to Artificial Intelligence, which argues intelligence is a function of and inseparable from life, bound by self-replication and evolution. The book covers a ton of neuroscience related to decision making and learning, though we focused on a few theoretical frameworks and ideas like division of labor and principal-agent relationships to understand how our brains and minds are related to our genes, how AI is related to humans (for now), metacognition, consciousness, and a ton more. Related:
| |||
16 Aug 2020 | BI 081 Pieter Roelfsema: Brain-propagation | 01:22:05 | |
Pieter and I discuss his ongoing quest to figure out how the brain implements learning that solves the credit assignment problem, like backpropagation does for neural networks. We also talk about his work to understand how we perceive individual objects in a crowded scene, his neurophysiological recordings in support of the global neuronal workspace hypothesis of consciousness, and the visual prosthetic device he’s developing to cure blindness by directly stimulating early visual cortex. Related:
| |||
26 Aug 2020 | BI 082 Steve Grossberg: Adaptive Resonance Theory | 02:15:38 | |
Steve and I discuss his long and productive career as a theoretical neuroscientist. We cover his tried and true method of taking a large body of psychological behavioral findings, determining how they fit together and what’s paradoxical about them, developing design principles, theories, and models from that body of data, and using experimental neuroscience to inform and confirm his model predictions. We talk about his Adaptive Resonance Theory (ART) to describe how our brains are self-organizing, adaptive, and deal with changing environments. We also talk about his complementary computing paradigm to describe how two systems can complement each other to create emergent properties neither system can create on its own , how the resonant states in ART support consciousness, his place in the history of both neuroscience and AI, and quite a bit more. Related:
Topics Time stamps: 0:00 - Intro 5:48 - Skip Intro 9:42 - Beginnings 18:40 - Modeling method 44:05 - Physics vs. neuroscience 54:50 - Historical credit for Hopfield network 1:03:40 - Steve's upcoming book 1:08:24 - Being shy 1:11:21 - Stability plasticity dilemma 1:14:10 - Adaptive resonance theory 1:18:25 - ART matching rule 1:21:35 - Consciousness as resonance 1:29:15 - Complementary computing 1:38:58 - Vigilance to re-orient 1:54:58 - Deep learning vs. ART | |||
05 Sep 2020 | BI 083 Jane Wang: Evolving Altruism in AI | 01:13:16 | |
Jane and I discuss the relationship between AI and neuroscience (cognitive science, etc), from her perspective at Deepmind after a career researching natural intelligence. We also talk about her meta-reinforcement learning work that connects deep reinforcement learning with known brain circuitry and processes, and finally we talk about her recent work using evolutionary strategies to develop altruism and cooperation among the agents in a multi-agent reinforcement learning environment. Related:
Timeline: 0:00 - Intro 3:36 - Skip Intro 4:45 - Transition to Deepmind 19:56 - Changing perspectives on neuroscience 24:49 - Is neuroscience useful for AI? 33:11 - Is deep learning hitting a wall? 35:57 - Meta-reinforcement learning 52:00 - Altruism in multi-agent RL | |||
15 Sep 2020 | BI 084 György Buzsáki and David Poeppel | 01:56:01 | |
David, Gyuri, and I discuss the issues they argue for in their back and forth commentaries about the importance of neuroscience and psychology, or implementation-level and computational-level, to advance our understanding of brains and minds - and the names we give to the things we study. Gyuri believes it’s time we use what we know and discover about brain mechanisms to better describe the psychological concepts we refer to as explanations for minds; David believes the psychological concepts are constantly being refined and are just as valid as objects of study to understand minds. They both agree these are important and enjoyable topics to debate. Also, special guest questions from Paul Cisek and John Krakauer. Related:
Timeline: 0:00 - Intro 5:31 - Skip intro 8:42 - Gyuri and David summaries 25:45 - Guest questions 36:25 - Gyuri new language 49:41 - Language and oscillations 53:52 - Do we know what cognitive functions we're looking for? 58:25 - Psychiatry 1:00:25 - Steve Grossberg approach 1:02:12 - Neuroethology 1:09:08 - AI as tabula rasa 1:17: 40 - What's at stake? 1:36:20 - Will the space between neuroscience and psychology disappear? | |||
30 Sep 2020 | BI 085 Ida Momennejad: Learning Representations | 01:43:41 | |
Ida and I discuss the current landscape of reinforcement learning in both natural and artificial intelligence, and how the old story of two RL systems in brains - model-free and model-based - is giving way to a more nuanced story of these two systems constantly interacting and additional RL strategies between model-free and model-based to drive the vast repertoire of our habits and goal-directed behaviors. We discuss Ida’s work on one of those “in-between” strategies, the successor representation RL strategy, which maps onto brain activity and accounts for behavior. We also discuss her interesting background and how it affects her outlook and research pursuit, and the role philosophy has played and continues to play in her thought processes. Related links:
Time stamps: 0:00 - Intro 4:50 - Skip intro 9:58 - Core way of thinking 19:58 - Disillusionment 27:22 - Role of philosophy 34:51 - Optimal individual learning strategy 39:28 - Microsoft job 44:48 - Field of reinforcement learning 51:18 - Learning vs. innate priors 59:47 - Incorporating other cognition into RL 1:08:24 - Evolution 1:12:46 - Model-free and model-based RL 1:19:02 - Successor representation 1:26:48 - Are we running all algorithms all the time? 1:28:38 - Heuristics and intuition 1:33:48 - Levels of analysis 1:37:28 - Consciousness | |||
12 Oct 2020 | BI 086 Ken Stanley: Open-Endedness | 01:35:43 | |
Ken and I discuss open-endedness, the pursuit of ambitious goals by seeking novelty and interesting products instead of advancing directly toward defined objectives. We talk about evolution as a prime example of an open-ended system that has produced astounding organisms, Ken relates how open-endedness could help advance artificial intelligence and neuroscience, and we discuss a range of topics related to the general concept of open-endedness, and Ken takes a couple questions from Stefan Leijnen and Melanie Mitchell. Related:
Some key take-aways:
0:00 - Intro 3:46 - Skip Intro 4:30 - Evolution as an Open-ended process 8:25 - Why Greatness Cannot Be Planned 20:46 - Open-endedness in AI 29:35 - Constraints vs. objectives 36:26 - The adjacent possible 41:22 - Serendipity 44:33 - Stefan Leijnen question 53:11 - Melanie Mitchell question 1:00:32 - Efficiency 1:02:13 - Gentle Earth 1:05:25 - Learning vs. evolution 1:10:53 - AGI 1:14:06 - Neuroscience, AI, and open-endedness 1:26:06 - Open AI | |||
23 Oct 2020 | BI 087 Dileep George: Cloning for Cognitive Maps | 01:23:00 | |
When a waiter hands me the bill, how do I know whether to pay it myself or let my date pay? On this episode, I get a progress update from Dileep on his company, Vicarious, since Dileep's last episode. We also talk broadly about his experience running Vicarious to develop AGI and robotics. Then we turn to his latest brain-inspired AI efforts using cloned structured probabilistic graph models to develop an account of how the hippocampus makes a model of the world and represents our cognitive maps in different contexts, so we can simulate possible outcomes to choose how to act. Special guest questions from Brad Love (episode 70: How We Learn Concepts) .
Time stamps: 0:00 - Intro 3:00 - Skip Intro 4:00 - Previous Dileep episode 10:22 - Is brain-inspired AI over-hyped? 14:38 - Compteition in robotics field 15:53 - Vicarious robotics 22:12 - Choosing what product to make 28:13 - Running a startup 30:52 - Old brain vs. new brain 37:53 - Learning cognitive maps as structured graphs 41:59 - Graphical models 47:10 - Cloning and merging, hippocampus 53:36 - Brad Love Question 1 1:00:39 - Brad Love Question 2 1:02:41 - Task examples 1:11:56 - What does hippocampus do? 1:14:14 - Intro to thalamic cortical microcircuit 1:15:21 - What AI folks think of brains 1:16:57 - Which levels inform which levels 1:20:02 - Advice for an AI startup | |||
02 Nov 2020 | BI 088 Randy O’Reilly: Simulating the Human Brain | 01:39:08 | |
Randy and I discuss his LEABRA cognitive architecture that aims to simulate the human brain, plus his current theory about how a loop between cortical regions and the thalamus could implement predictive learning and thus solve how we learn with so few examples. We also discuss what Randy thinks is the next big thing neuroscience can contribute to AI (thanks to a guest question from Anna Schapiro), and much more.
A few take-home points:
Timestamps: 0:00 - Intro 3:54 - Skip Intro 6:20 - Being in awe 18:57 - How current AI can inform neuro 21:56 - Anna Schapiro question - how current neuro can inform AI. 29:20 - Learned vs. innate cognition 33:43 - LEABRA 38:33 - Developing Leabra 40:30 - Macroscale 42:33 - Thalamus as microscale 43:22 - Thalamocortical circuitry 47:25 - Deep predictive learning 56:18 - Deep predictive learning vs. backrop 1:01:56 - 10 Hz learning cycle 1:04:58 - Better theory vs. more data 1:08:59 - Leabra vs. Spaun 1:13:59 - Biological realism 1:21:54 - Bottom-up inspiration 1:27:26 - Biggest mistake in Leabra 1:32:14 - AI consciousness 1:34:45 - How would Randy begin again? | |||
12 Nov 2020 | BI 089 Matt Smith: Drifting Cognition | 01:26:52 | |
Matt and I discuss how cognition and behavior drifts over the course of minutes and hours, and how global brain activity drifts with it. How does the brain continue to produce steady perception and action in the midst of such drift? We also talk about how to think about variability in neural activity. How much of it is noise and how much of it is hidden important activity? Finally, we discuss the effect of recording more and more neurons simultaneously, collecting bigger and bigger datasets, plus guest questions from Adam Snyder and Patrick Mayo.
Take home points:
Timestamps: 0:00 - Intro 4:35 - Adam Snyder question 15:26 - Multi-electrode recordings 17:48 - What is noise in the brain? 23:55 - How many neurons is enough? 27:43 - Patrick Mayo question 33:17 - Slow drift 54:10 - Impulsivity 57:32 - How does drift happen? 59:49 - Relation to AI 1:06:58 - What AI and neuro can teach each other 1:10:02 - Ecologically valid behavior 1:14:39 - Brain mechanisms vs. mind 1:17:36 - Levels of description 1:21:14 - Hard things to make in AI 1:22:48 - Best scientific moment | |||
23 Nov 2020 | BI 090 Chris Eliasmith: Building the Human Brain | 01:38:57 | |
Chris and I discuss his Spaun large scale model of the human brain (Semantic Pointer Architecture Unified Network), as detailed in his book How to Build a Brain. We talk about his philosophical approach, how Spaun compares to Randy O'Reilly's Leabra networks, the Applied Brain Research Chris co-founded, and I have guest questions from Brad Aimone, Steve Potter, and Randy O'Reilly.
Some takeaways:
Time Points: 0:00 - Intro 2:29 - Sense of awe 6:20 - Large-scale models 9:24 - Descriptive pragmatism 15:43 - Asking better questions 22:48 - Brad Aimone question: Neural engineering framework 29:07 - Engineering to build vs. understand 32:12 - Why is AI world not interested in brains/minds? 37:09 - Steve Potter neuromorphics question 44:51 - Spaun 49:33 - Semantic Pointer Architecture 56:04 - Representations 58:21 - Randy O'Reilly question 1 1:07:33 - Randy O'Reilly question 2 1:10:31 - Spaun vs. Leabra 1:32:43 - How would Chris start over? | |||
04 Dec 2020 | BI 091 Carsen Stringer: Understanding 40,000 Neurons | 01:28:19 | |
Carsen and I discuss how she uses 2-photon calcium imaging data from over 10,000 neurons to understand the information processing of such large neural population activity. We talk about the tools she makes and uses to analyze the data, and the type of high-dimensional neural activity structure they found, which seems to allow efficient and robust information processing. We also talk about how these findings may help build better deep learning networks, and Carsen's thoughts on how to improve the diversity, inclusivity, and equality in neuroscience research labs. Guest question from Matt Smith.
Timestamps: 0:00 - Intro 5:51 - Recording > 10k neurons 8:51 - 2-photon calcium imaging 14:56 - Balancing scientific questions and tools 21:16 - Unsupervised learning tools and rastermap 26:14 - Manifolds 32:13 - Matt Smith question 37:06 - Dimensionality of neural activity 58:51 - Future plans 1:00:30- What can AI learn from this? 1:13:26 - Diversity, inclusivity, equality | |||
15 Dec 2020 | BI 092 Russ Poldrack: Cognitive Ontologies | 01:42:12 | |
Russ and I discuss cognitive ontologies - the "parts" of the mind and their relations - as an ongoing dilemma of how to map onto each other what we know about brains and what we know about minds. We talk about whether we have the right ontology now, how he uses both top-down and data-driven approaches to analyze and refine current ontologies, and how all this has affected his own thinking about minds. We also discuss some of the current meta-science issues and challenges in neuroscience and AI, and Russ answers guest questions from Kendrick Kay and David Poeppel.
Some take-home points:
Time points 0:00 - Introduction 5:59 - Meta-science issues 19:00 - Kendrick Kay question 23:00 - State of the field 30:06 - fMRI for understanding minds 35:13 - Computational mind 42:10 - Cognitive ontology 45:17 - Cognitive Atlas 52:05 - David Poeppel question 57:00 - Does ontology matter? 59:18 - Data-driven ontology 1:12:29 - Dynamical systems approach 1:16:25 - György Buzsáki's inside-out approach 1:22:26 - Ontology for AI 1:27:39 - Deep learning hype | |||
29 Dec 2020 | BI 093 Dileep George: Inference in Brain Microcircuits | 01:06:31 | |
Dileep and I discuss his theoretical account of how the thalamus and cortex work together to implement visual inference. We talked previously about his Recursive Cortical Network (RCN) approach to visual inference, which is a probabilistic graph model that can solve hard problems like CAPTCHAs, and more recently we talked about using his RCNs with cloned units to account for cognitive maps related to the hippocampus. On this episode, we walk through how RCNs can map onto thalamo-cortical circuits so a given cortical column can signal whether it believes some concept or feature is present in the world, based on bottom-up incoming sensory evidence, top-down attention, and lateral related features. We also briefly compare this bio-RCN version with Randy O'Reilly's Deep Predictive Learning account of thalamo-cortical circuitry.
Time Stamps: 0:00 - Intro 5:18 - Levels of abstraction 7:54 - AGI vs. AHI vs. AUI 12:18 - Ideas and failures in startups 16:51 - Thalamic cortical circuitry computation 22:07 - Recursive cortical networks 23:34 - bio-RCN 27:48 - Cortical column as binary random variable 33:37 - Clonal neuron roles 39:23 - Processing cascade 41:10 - Thalamus 47:18 - Attention as explaining away 50:51 - Comparison with O'Reilly's predictive coding framework 55:39 - Subjective contour effect 1:01:20 - Necker cube | |||
08 Jan 2021 | BI 094 Alison Gopnik: Child-Inspired AI | 01:19:13 | |
Alison and I discuss her work to accelerate learning and thus improve AI by studying how children learn, as Alan Turing suggested in his famous 1950 paper. The ways children learn are via imitation, by learning abstract causal models, and active learning by implementing a high exploration/exploitation ratio. We also discuss child consciousness, psychedelics, the concept of life history, the role of grandparents and elders, and lots more.
Take-home points:
Timestamps 0:00 - Intro 4:40 - State of the field 13:30 - Importance of learning 20:12 - Turing's suggestion 22:49 - Patience for one's own ideas 28:53 - Learning via imitation 31:57 - Learning abstract causal models 41:42 - Life history 43:22 - Learning via exploration 56:19 - Explore-exploit dichotomy 58:32 - Synaptic pruning 1:00:19 - Breakthrough research in careers 1:04:31 - Role of elders 1:09:08 - Child consciousness 1:11:41 - Psychedelics as child-like brain 1:16:00 - Build consciousness into AI? | |||
19 Jan 2021 | BI 095 Chris Summerfield and Sam Gershman: Neuro for AI? | 01:25:28 | |
It's generally agreed machine learning and AI provide neuroscience with tools for analysis and theoretical principles to test in brains, but there is less agreement about what neuroscience can provide AI. Should computer scientists and engineers care about how brains compute, or will it just slow them down, for example? Chris, Sam, and I discuss how neuroscience might contribute to AI moving forward, considering the past and present. This discussion also leads into related topics, like the role of prediction versus understanding, AGI, explainable AI, value alignment, the fundamental conundrum that humans specify the ultimate values of the tasks AI will solve, and more. Plus, a question from previous guest Andrew Saxe. Also, check out Sam's previous appearance on the podcast.
0:00 - Intro 5:00 - Good ol' days 13:50 - AI for neuro, neuro for AI 24:25 - Intellectual diversity in AI 28:40 - Role of philosophy 30:20 - Operationalization and benchmarks 36:07 - Prediction vs. understanding 42:48 - Role of humans in the loop 46:20 - Value alignment 51:08 - Andrew Saxe question 53:16 - Explainable AI 58:55 - Generalization 1:01:09 - What has AI revealed about us? 1:09:38 - Neuro for AI 1:20:30 - Concluding remarks | |||
29 Jan 2021 | BI 096 Keisuke Fukuda and Josh Cosman: Forking Paths | 01:34:10 | |
K, Josh, and I were postdocs together in Jeff Schall's and Geoff Woodman's labs. K and Josh had backgrounds in psychology and were getting their first experience with neurophysiology, recording single neuron activity in awake behaving primates. This episode is a discussion surrounding their reflections and perspectives on neuroscience and psychology, given their backgrounds and experience (we reference episode 84 with György Buzsáki and David Poeppel). We also talk about their divergent paths - K stayed in academia and runs an EEG lab studying human decision-making and memory, and Josh left academia and has worked for three different pharmaceutical and tech companies. So this episode doesn't get into gritty science questions, but is a light discussion about the state of neuroscience, psychology, and AI, and reflections on academia and industry, life in lab, and plenty more.
Time stamps 0:00 - Intro 4:30 - K intro 5:30 - Josh Intro 10:16 - Academia vs. industry 16:01 - Concern with legacy 19:57 - Best scientific moment 24:15 - Experiencing neuroscience as a psychologist 27:20 - Neuroscience as a tool 30:38 - Brain/mind divide 33:27 - Shallow vs. deep knowledge in academia and industry 36:05 - Autonomy in industry 42:20 - Is this a turning point in neuroscience? 46:54 - Deep learning revolution 49:34 - Deep nets to understand brains 54:54 - Psychology vs. neuroscience 1:06:42 - Is language sufficient? 1:11:33 - Human-level AI 1:13:53 - How will history view our era of neuroscience? 1:23:28 - What would you have done differently? 1:26:46 - Something you wish you knew | |||
08 Feb 2021 | BI 097 Omri Barak and David Sussillo: Dynamics and Structure | 01:23:57 | |
Omri, David and I discuss using recurrent neural network models (RNNs) to understand brains and brain function. Omri and David both use dynamical systems theory (DST) to describe how RNNs solve tasks, and to compare the dynamical stucture/landscape/skeleton of RNNs with real neural population recordings. We talk about how their thoughts have evolved since their 2103 Opening the Black Box paper, which began these lines of research and thinking. Some of the other topics we discuss:
Timestamps: 0:00 - Intro 5:41 - Best scientific moment 9:37 - Why do you do what you do? 13:21 - Computation via dynamics 19:12 - Evolution of thinking about RNNs and brains 26:22 - RNNs vs. minds 31:43 - Classical computational modeling vs. machine learning modeling approach 35:46 - What are models good for? 43:08 - Ecological task validity with respect to using RNNs as models 46:27 - Optimization vs. learning 49:11 - Universality 1:00:47 - Solutions dictated by tasks 1:04:51 - Multiple solutions to the same task 1:11:43 - Direct fit (Uri Hasson) 1:19:09 - Thinking about the bigger picture | |||
18 Feb 2021 | BI 098 Brian Christian: The Alignment Problem | 01:32:38 | |
Brian and I discuss a range of topics related to his latest book, The Alignment Problem: Machine Learning and Human Values. The alignment problem asks how we can build AI that does what we want it to do, as opposed to building AI that will compromise our own values by accomplishing tasks that may be harmful or dangerous to us. Using some of the stories Brain relates in the book, we talk about:
Links:
Timestamps: 4:22 - Increased work on AI ethics 8:59 - The Alignment Problem overview 12:36 - Stories as important for intelligence 16:50 - What is the alignment problem 17:37 - Who works on the alignment problem? 25:22 - AI ethics degree? 29:03 - Human values 31:33 - AI alignment and evolution 37:10 - Knowing our own values? 46:27 - What have learned about ourselves? 58:51 - Interestingness 1:00:53 - Inverse RL for value alignment 1:04:50 - Current progress 1:10:08 - Developmental psychology 1:17:36 - Models as the danger 1:25:08 - How worried are the experts? | |||
28 Feb 2021 | BI 099 Hakwan Lau and Steve Fleming: Neuro-AI Consciousness | 01:46:35 | |
Hakwan, Steve, and I discuss many issues around the scientific study of consciousness. Steve and Hakwan focus on higher order theories (HOTs) of consciousness, related to metacognition. So we discuss HOTs in particular and their relation to other approaches/theories, the idea of approaching consciousness as a computational problem to be tackled with computational modeling, we talk about the cultural, social, and career aspects of choosing to study something as elusive and controversial as consciousness, we talk about two of the models they're working on now to account for various properties of conscious experience, and, of course, the prospects of consciousness in AI. For more on metacognition and awareness, check out episode 73 with Megan Peters.
Timestamps 0:00 - Intro 7:25 - Steve's upcoming book 8:40 - Challenges to study consciousness 15:50 - Gurus and backscratchers 23:58 - Will the problem of consciousness disappear? 27:52 - Will an explanation feel intuitive? 29:54 - What do you want to be true? 38:35 - Lucid dreaming 40:55 - Higher order theories 50:13 - Reality monitoring model of consciousness 1:00:15 - Higher order state space model of consciousness 1:05:50 - Comparing their models 1:10:47 - Machine consciousness 1:15:30 - Nature of first order representations 1:18:20 - Consciousness prior (Yoshua Bengio) 1:20:20 - Function of consciousness 1:31:57 - Legacy 1:40:55 - Current projects | |||
09 Mar 2021 | BI 100.1 Special: What Has Improved Your Career or Well-being? | 00:42:32 | |
Brain Inspired turns 100 (episodes) today! To celebrate, my patreon supporters helped me create a list of questions to ask my previous guests, many of whom contributed by answering any or all of the questions. I've collected all their responses into separate little episodes, one for each question. Starting with a light-hearted (but quite valuable) one, this episode has responses to the question, "In the last five years, what new belief, behavior, or habit has most improved your career or well being?" See below for links to each previous guest. And away we go... Timestamps: 0:00 - Intro 6:13 - David Krakauer 8:50 - David Poeppel 9:32 - Jay McClelland 11:03 - Patrick Mayo 11:45 - Marcel van Gerven 12:11 - Blake Richards 12:25 - John Krakauer 14:22 - Nicole Rust 15:26 - Megan Peters 17:03 - Andrew Saxe 18:11 - Federico Turkheimer 20:03 - Rodrigo Quian Quiroga 22:03 - Thomas Naselaris 23:09 - Steve Potter 24:37 - Brad Love 27:18 - Steve Grossberg 29:04 - Talia Konkle 29:58 - Paul Cisek 32:28 - Kanaka Rajan 34:33 - Grace Lindsay 35:40 - Konrad Kording 36:30 - Mark Humphries | |||
12 Mar 2021 | BI 100.2 Special: What Are the Biggest Challenges and Disagreements? | 01:25:00 | |
In this 2nd special 100th episode installment, many previous guests answer the question: What is currently the most important disagreement or challenge in neuroscience and/or AI, and what do you think the right answer or direction is? The variety of answers is itself revealing, and highlights how many interesting problems there are to work on. Timestamps: 0:00 - Intro 7:10 - Rodrigo Quian Quiroga 8:33 - Mazviita Chirimuuta 9:15 - Chris Eliasmith 12:50 - Jim DiCarlo 13:23 - Paul Cisek 16:42 - Nathaniel Daw 17:58 - Jessica Hamrick 19:07 - Russ Poldrack 20:47 - Pieter Roelfsema 22:21 - Konrad Kording 25:16 - Matt Smith 27:55 - Rafal Bogacz 29:17 - John Krakauer 30:47 - Marcel van Gerven 31:49 - György Buzsáki 35:38 - Thomas Naselaris 36:55 - Steve Grossberg 48:32 - David Poeppel 49:24 - Patrick Mayo 50:31 - Stefan Leijnen 54:24 - David Krakuer 58:13 - Wolfang Maass 59:13 - Uri Hasson 59:50 - Steve Potter 1:01:50 - Talia Konkle 1:04:30 - Matt Botvinick 1:06:36 - Brad Love 1:09:46 - Jon Brennan 1:19:31 - Grace Lindsay 1:22:28 - Andrew Saxe | |||
17 Mar 2021 | BI 100.3 Special: Can We Scale Up to AGI with Current Tech? | 01:08:43 | |
Part 3 in our 100th episode celebration. Previous guests answered the question: Given the continual surprising progress in AI powered by scaling up parameters and using more compute, while using fairly generic architectures (eg. GPT-3): Do you think the current trend of scaling compute can lead to human level AGI? If not, what's missing? It likely won't surprise you that the vast majority answer "No." It also likely won't surprise you, there is differing opinion on what's missing. Timestamps: 0:00 - Intro 3:56 - Wolgang Maass 5:34 - Paul Humphreys 9:16 - Chris Eliasmith 12:52 - Andrew Saxe 16:25 - Mazviita Chirimuuta 18:11 - Steve Potter 19:21 - Blake Richards 22:33 - Paul Cisek 26:24 - Brad Love 29:12 - Jay McClelland 34:20 - Megan Peters 37:00 - Dean Buonomano 39:48 - Talia Konkle 40:36 - Steve Grossberg 42:40 - Nathaniel Daw 44:02 - Marcel van Gerven 45:28 - Kanaka Rajan 48:25 - John Krakauer 51:05 - Rodrigo Quian Quiroga 53:03 - Grace Lindsay 55:13 - Konrad Kording 57:30 - Jeff Hawkins 102:12 - Uri Hasson 1:04:08 - Jess Hamrick 1:06:20 - Thomas Naselaris | |||
21 Mar 2021 | BI 100.4 Special: What Ideas Are Holding Us Back? | 01:04:26 | |
In the 4th installment of our 100th episode celebration, previous guests responded to the question: What ideas, assumptions, or terms do you think is holding back neuroscience/AI, and why? As usual, the responses are varied and wonderful! Timestamps: 0:00 - Intro 6:41 - Pieter Roelfsema 7:52 - Grace Lindsay 10:23 - Marcel van Gerven 11:38 - Andrew Saxe 14:05 - Jane Wang 16:50 - Thomas Naselaris 18:14 - Steve Potter 19:18 - Kendrick Kay 22:17 - Blake Richards 27:52 - Jay McClelland 30:13 - Jim DiCarlo 31:17 - Talia Konkle 33:27 - Uri Hasson 35:37 - Wolfgang Maass 38:48 - Paul Cisek 40:41 - Patrick Mayo 41:51 - Konrad Kording 43:22 - David Poeppel 44:22 - Brad Love 46:47 - Rodrigo Quian Quiroga 47:36 - Steve Grossberg 48:47 - Mark Humphries 52:35 - John Krakauer 55:13 - György Buzsáki 59:50 - Stefan Leijnan 1:02:18 - Nathaniel Daw | |||
28 Mar 2021 | BI 100.6 Special: Do We Have the Right Vocabulary and Concepts? | 00:50:03 | |
We made it to the last bit of our 100th episode celebration. These have been super fun for me, and I hope you've enjoyed the collections as well. If you're wondering where the missing 5th part is, I reserved it exclusively for Brain Inspired's magnificent Patreon supporters (thanks guys!!!!). The final question I sent to previous guests: Do we already have the right vocabulary and concepts to explain how brains and minds are related? Why or why not? Timestamps: 0:00 - Intro 5:04 - Andrew Saxe 7:04 - Thomas Naselaris 7:46 - John Krakauer 9:03 - Federico Turkheimer 11:57 - Steve Potter 13:31 - David Krakauer 17:22 - Dean Buonomano 20:28 - Konrad Kording 22:00 - Uri Hasson 23:15 - Rodrigo Quian Quiroga 24:41 - Jim DiCarlo 25:26 - Marcel van Gerven 28:02 - Mazviita Chirimuuta 29:27 - Brad Love 31:23 - Patrick Mayo 32:30 - György Buzsáki 37:07 - Pieter Roelfsema 37:26 - David Poeppel 40:22 - Paul Cisek 44:52 - Talia Konkle 47:03 - Steve Grossberg | |||
06 Apr 2021 | BI 101 Steve Potter: Motivating Brains In and Out of Dishes | 01:45:22 | |
Steve and I discuss his book, How to Motivate Your Students to Love Learning, which is both a memoir and a guide for teachers and students to optimize the learning experience for intrinsic motivation. Steve taught neuroscience and engineering courses while running his own lab studying the activity of live cultured neural populations (which we discuss at length in his previous episode). He relentlessly tested and tweaked his teaching methods, including constant feedback from the students, to optimize their learning experiences. He settled on real-world, project-based learning approaches, like writing wikipedia articles and helping groups of students design and carry out their own experiments. We discuss that, plus the science behind learning, principles important for motivating students and maintaining that motivation, and many of the other valuable insights he shares in the book. The first half of the episode we discuss diverse neuroscience and AI topics, like brain organoids, mind-uploading, synaptic plasticity, and more. Then we discuss many of the stories and lessons from his book, which I recommend for teachers, mentors, and life-long students who want to ensure they're optimizing their own learning.
0:00 - Intro 6:38 - Brain organoids 18:48 - Glial cell plasticity 24:50 - Whole brain emulation 35:28 - Industry vs. academia 45:32 - Intro to book: How To Motivate Your Students To Love Learning 48:29 - Steve's childhood influences 57:21 - Developing one's own intrinsic motivation 1:02:30 - Real-world assignments 1:08:00 - Keys to motivation 1:11:50 - Peer pressure 1:21:16 - Autonomy 1:25:38 - Wikipedia real-world assignment 1:33:12 - Relation to running a lab | |||
16 Apr 2021 | BI 102 Mark Humphries: What Is It Like To Be A Spike? | 01:32:20 | |
Mark and I discuss his book, The Spike: An Epic Journey Through the Brain in 2.1 Seconds. It chronicles how a series of action potentials fire through the brain in a couple seconds of someone's life. Starting with light hitting the retina as a person looks at a cookie, Mark describes how that light gets translated into spikes, how those spikes get processed in our visual system and eventually transform into motor commands to grab that cookie. Along the way, he describes some of the big ideas throughout the history of studying brains (like the mechanisms to explain how neurons seem to fire so randomly), the big mysteries we currently face (like why do so many neurons do so little?), and some of the main theories to explain those mysteries (we're prediction machines!). A fun read and discussion. This is Mark's second time on the podcast - he was on episode 4 in the early days, talking more in depth about some of the work we discuss in this episode!
Timestamps: 0:00 - Intro 3:25 - Writing a book 15:37 - Mark's main interest 19:41 - Future explanation of brain/mind 27:00 - Stochasticity and excitation/inhibition balance 36:56 - Dendritic computation for network dynamics 39:10 - Do details matter for AI? 44:06 - Spike failure 51:12 - Dark neurons 1:07:57 - Intrinsic spontaneous activity 1:16:16 - Best scientific moment 1:23:58 - Failure 1:28:45 - Advice | |||
26 Apr 2021 | BI 103 Randal Koene and Ken Hayworth: The Road to Mind Uploading | 01:27:26 | |
Randal, Ken, and I discuss a host of topics around the future goal of uploading our minds into non-brain systems, to continue our mental lives and expand our range of experiences. The basic requirement for such a subtrate-independent mind is to implement whole brain emulation. We discuss two basic approaches to whole brain emulation. The "scan and copy" approach proposes we somehow scan the entire structure of our brains (at whatever scale is necessary) and store that scan until some future date when we have figured out how to us that information to build a substrate that can house your mind. The "gradual replacement" approach proposes we slowly replace parts of the brain with functioning alternative machines, eventually replacing the entire brain with non-biological material and yet retaining a functioning mind. Randal and Ken are neuroscientists who understand the magnitude and challenges of a massive project like mind uploading, who also understand what we can do right now, with current technology, to advance toward that lofty goal, and who are thoughtful about what steps we need to take to enable further advancements.
Timestamps 0:00 - Intro 6:14 - What Ken wants 11:22 - What Randal wants 22:29 - Brain preservation 27:18 - Aldehyde stabilized cryopreservation 31:51 - Scan and copy vs. gradual replacement 38:25 - Building a roadmap 49:45 - Limits of current experimental paradigms 53:51 - Our evolved brains 1:06:58 - Counterarguments 1:10:31 - Animal models for whole brain emulation 1:15:01 - Understanding vs. emulating brains 1:22:37 - Current challenges | |||
07 May 2021 | BI 104 John Kounios and David Rosen: Creativity, Expertise, Insight | 01:50:32 | |
What is creativity? How do we measure it? How do our brains implement it, and how might AI?Those are some of the questions John, David, and I discuss. The neuroscience of creativity is young, in its "wild west" days still. We talk about a few creativity studies they've performed that distinguish different creative processes with respect to different levels of expertise (in this case, in jazz improvisation), and the underlying brain circuits and activity, including using transcranial direct current stimulation to alter the creative process. Related to creativity, we also discuss the phenomenon and neuroscience of insight (the topic of John's book, The Eureka Factor), unconscious automatic type 1 processes versus conscious deliberate type 2 processes, states of flow, creative process versus creative products, and a lot more.
Timestamps 0:00 - Intro 16:20 - Where are we broadly in science of creativity? 18:23 - Origins of creativity research 22:14 - Divergent and convergent thought 26:31 - Secret Chord Labs 32:40 - Familiar surprise 38:55 - The Eureka Factor 42:27 - Dual process model 52:54 - Creativity and jazz expertise 55:53 - "Be creative" behavioral study 59:17 - Stimulating the creative brain 1:02:04 - Brain circuits underlying creativity 1:14:36 - What does this tell us about creativity? 1:16:48 - Intelligence vs. creativity 1:18:25 - Switching between creative modes 1:25:57 - Flow states and insight 1:34:29 - Creativity and insight in AI 1:43:26 - Creative products vs. process | |||
17 May 2021 | BI 105 Sanjeev Arora: Off the Convex Path | 01:01:43 | |
Sanjeev and I discuss some of the progress toward understanding how deep learning works, specially under previous assumptions it wouldn't or shouldn't work as well as it does. Deep learning theory poses a challenge for mathematics, because its methods aren't rooted in mathematical theory and therefore are a "black box" for math to open. We discuss how Sanjeev thinks optimization, the common framework for thinking of how deep nets learn, is the wrong approach. Instead, a promising alternative focuses on the learning trajectories that occur as a result of different learning algorithms. We discuss two examples of his research to illustrate this: creating deep nets with infinitely large layers (and the networks still find solutions among the infinite possible solutions!), and massively increasing the learning rate during training (the opposite of accepted wisdom, and yet, again, the network finds solutions!). We also discuss his past focus on computational complexity and how he doesn't share the current neuroscience optimism comparing brains to deep nets.
Timestamps 0:00 - Intro 7:32 - Computational complexity 12:25 - Algorithms 13:45 - Deep learning vs. traditional optimization 17:01 - Evolving view of deep learning 18:33 - Reproducibility crisis in AI? 21:12 - Surprising effectiveness of deep learning 27:50 - "Optimization" isn't the right framework 30:08 - Infinitely wide nets 35:41 - Exponential learning rates 42:39 - Data as the next frontier 44:12 - Neuroscience and AI differences 47:13 - Focus on algorithms, architecture, and objective functions 55:50 - Advice for deep learning theorists 58:05 - Decoding minds | |||
27 May 2021 | BI 106 Jacqueline Gottlieb and Robert Wilson: Deep Curiosity | 01:31:53 | |
Jackie and Bob discuss their research and thinking about curiosity. Jackie's background is studying decision making and attention, recording neurons in nonhuman primates during eye movement tasks, and she's broadly interested in how we adapt our ongoing behavior. Curiosity is crucial for this, so she recently has focused on behavioral strategies to exercise curiosity, developing tasks that test exploration, information sampling, uncertainty reduction, and intrinsic motivation. Bob's background is developing computational models of reinforcement learning (including the exploration-exploitation tradeoff) and decision making, and he behavior and neuroimaging data in humans to test the models. He's broadly interested in how and whether we can understand brains and cognition using mathematical models. Recently he's been working on a model for curiosity known as deep exploration, which suggests we make decisions by deeply simulating a handful of scenarios and choosing based on the simulation outcomes. We also discuss how one should go about their career (qua curiosity), how eye movements compare with other windows into cognition, and whether we can and should create curious AI agents (Bob is an emphatic yes, and Jackie is slightly worried that will be the time to worry about AI).
Timestamps: 0:00 - Intro 4:15 - Central scientific interests 8:32 - Advent of mathematical models 12:15 - Career exploration vs. exploitation 28:03 - Eye movements and active sensing 35:53 - Status of eye movements in neuroscience 44:16 - Why are we curious? 50:26 - Curiosity vs. Exploration vs. Intrinsic motivation 1:02:35 - Directed vs. random exploration 1:06:16 - Deep exploration 1:12:52 - How to know what to pay attention to 1:19:49 - Does AI need curiosity? 1:26:29 - What trait do you wish you had more of? | |||
06 Jun 2021 | BI 107 Steve Fleming: Know Thyself | 01:29:24 | |
Steve and I discuss many topics from his new book Know Thyself: The Science of Self-Awareness. The book covers the full range of what we know about metacognition and self-awareness, including how brains might underlie metacognitive behavior, computational models to explain mechanisms of metacognition, how and why self-awareness evolved, which animals beyond humans harbor metacognition and how to test it, its role and potential origins in theory of mind and social interaction, how our metacognitive skills develop over our lifetimes, what our metacognitive skill tells us about our other psychological traits, and so on. We also discuss what it might look like when we are able to build metacognitive AI, and whether that's even a good idea.
Timestamps 0:00 - Intro 3:25 - Steve's Career 10:43 - Sub-personal vs. personal metacognition 17:55 - Meditation and metacognition 20:51 - Replay tools for mind-wandering 30:56 - Evolutionary cultural origins of self-awareness 45:02 - Animal metacognition 54:25 - Aging and self-awareness 58:32 - Is more always better? 1:00:41 - Political dogmatism and overconfidence 1:08:56 - Reliance on AI 1:15:15 - Building self-aware AI 1:23:20 - Future evolution of metacognition | |||
16 Jun 2021 | BI 108 Grace Lindsay: Models of the Mind | 01:26:12 | |
Grace and I discuss her new book Models of the Mind, about the blossoming and conceptual foundations of the computational approach to study minds and brains. Each chapter of the book focuses on one major topic and provides historical context, the major concepts that connect models to brain functions, and the current landscape of related research endeavors. We cover a handful of those during the episode, including the birth of AI, the difference between math in physics and neuroscience, determining the neural code and how Shannon information theory plays a role, whether it's possible to guess a brain function based on what we know about some brain structure, "grand unified theories" of the brain. We also digress and explore topics beyond the book. Timestamps 0:00 - Intro 4:19 - Cognition beyond vision 12:38 - Models of the Mind - book overview 14:00 - The good and bad of using math 21:33 - I quiz Grace on her own book 25:03 - Birth of AI and computational approach 38:00 - Rediscovering old math for new neuroscience 41:00 - Topology as good math to know now 45:29 - Physics vs. neuroscience math 49:32 - Neural code and information theory 55:03 - Rate code vs. timing code 59:18 - Graph theory - can you deduce function from structure? 1:06:56 - Multiple realizability 1:13:01 - Grand Unified theories of the brain | |||
26 Jun 2021 | BI 109 Mark Bickhard: Interactivism | 02:03:43 | |
Mark and I discuss a wide range of topics surrounding his Interactivism framework for explaining cognition. Interactivism stems from Mark's account of representations and how what we represent in our minds is related to the external world - a challenge that has plagued the mind-body problem since the beginning. Basically, representations are anticipated interactions with the world, that can be true (if enacting one helps an organism maintain its thermodynamic relation with the world) or false (if it doesn't). And representations are functional, in that they function to maintain far from equilibrium thermodynamics for the organism for self-maintenance. Over the years, Mark has filled out Interactivism, starting with a process metaphysics foundation and building from there to account for representations, how our brains might implement representations, and why AI is hindered by our modern "encoding" version of representation. We also compare interactivism to other similar frameworks, like enactivism, predictive processing, and the free energy principle. For related discussions on the foundations (and issues of) representations, check out episode 60 with Michael Rescorla, episode 61 with Jörn Diedrichsen and Niko Kriegeskorte, and especially episode 79 with Romain Brette.
Timestamps 0:00 - Intro 5:06 - Previous and upcoming book 9:17 - Origins of Mark's thinking 14:31 - Process vs. substance metaphysics 27:10 - Kinds of emergence 32:16 - Normative emergence to normative function and representation 36:33 - Representation in Interactivism 46:07 - Situation knowledge 54:02 - Interactivism vs. Enactivism 1:09:37 - Interactivism vs Predictive/Bayesian brain 1:17:39 - Interactivism vs. Free energy principle 1:21:56 - Microgenesis 1:33:11 - Implications for neuroscience 1:38:18 - Learning as variation and selection 1:45:07 - Implications for AI 1:55:06 - Everything is a clock 1:58:14 - Is Mark a philosopher? | |||
06 Jul 2021 | BI 110 Catherine Stinson and Jessica Thompson: Neuro-AI Explanation | 01:25:02 | |
Catherine, Jess, and I use some of the ideas from their recent papers to discuss how different types of explanations in neuroscience and AI could be unified into explanations of intelligence, natural or artificial. Catherine has written about how models are related to the target system they are built to explain. She suggests both the model and the target system should be considered as instantiations of a specific kind of phenomenon, and explanation is a product of relating the model and the target system to that specific aspect they both share. Jess has suggested we shift our focus of explanation from objects - like a brain area or a deep learning model - to the shared class of phenomenon performed by those objects. Doing so may help bridge the gap between the different forms of explanation currently used in neuroscience and AI. We also discuss Henk de Regt's conception of scientific understanding and its relation to explanation (they're different!), and plenty more.
Timestamps: 0:00 - Intro 11:11 - Background and approaches 27:00 - Understanding distinct from explanation 36:00 - Explanations as programs (early explanation) 40:42 - Explaining classes of phenomena 52:05 - Constitutive (neuro) vs. etiological (AI) explanations 1:04:04 - Do nonphysical objects count for explanation? 1:10:51 - Advice for early philosopher/scientists | |||
12 Jul 2021 | BI NMA 01: Machine Learning Panel | 01:27:12 | |
Panelists: This is the first in a series of panel discussions in collaboration with Neuromatch Academy, the online computational neuroscience summer school. In this episode, the panelists discuss their experiences with model fitting, GLMs/machine learning, dimensionality reduction, and deep learning. Other panels:
| |||
15 Jul 2021 | BI NMA 02: Dynamical Systems Panel | 01:15:28 | |
Panelists: This is the second in a series of panel discussions in collaboration with Neuromatch Academy, the online computational neuroscience summer school. In this episode, the panelists discuss their experiences with linear systems, real neurons, and dynamic networks. Other panels:
| |||
22 Jul 2021 | BI NMA 03: Stochastic Processes Panel | 01:00:48 | |
Panelists: This is the third in a series of panel discussions in collaboration with Neuromatch Academy, the online computational neuroscience summer school. In this episode, the panelists discuss their experiences with stochastic processes, including Bayes, decision-making, optimal control, reinforcement learning, and causality. The other panels:
| |||
28 Jul 2021 | BI 111 Kevin Mitchell and Erik Hoel: Agency, Emergence, Consciousness | 01:38:04 | |
Erik, Kevin, and I discuss... well a lot of things. Erik's recent novel The Revelations is a story about a group of neuroscientists trying to develop a good theory of consciousness (with a murder mystery plot). Kevin's book Innate - How the Wiring of Our Brains Shapes Who We Are describes the messy process of getting from DNA, traversing epigenetics and development, to our personalities. We talk about both books, then dive deeper into topics like whether brains evolved for moving our bodies vs. consciousness, how information theory is lending insights to emergent phenomena, and the role of agency with respect to what counts as intelligence.
Timestamps 0:00 - Intro 3:28 - The Revelations - Erik's novel 15:15 - Innate - Kevin's book 22:56 - Cycle of progress 29:05 - Brains for movement or consciousness? 46:46 - Freud's influence 59:18 - Theories of consciousness 1:02:02 - Meaning and emergence 1:05:50 - Reduction in neuroscience 1:23:03 - Micro and macro - emergence 1:29:35 - Agency and intelligence | |||
06 Aug 2021 | BI NMA 04: Deep Learning Basics Panel | 00:59:21 | |
BI NMA 04:Deep Learning Basics PanelThis is the 4th in a series of panel discussions in collaboration with Neuromatch Academy, the online computational neuroscience summer school. This is the first of 3 in the deep learning series. In this episode, the panelists discuss their experiences with some basics in deep learning, including Linear deep learning, Pytorch, multi-layer-perceptrons, optimization, & regularization. GuestsThe other panels:
Timestamps:
| |||
13 Aug 2021 | BI NMA 05: NLP and Generative Models Panel | 01:23:50 | |
BI NMA 05:NLP and Generative Models PanelThis is the 5th in a series of panel discussions in collaboration with Neuromatch Academy, the online computational neuroscience summer school. This is the 2nd of 3 in the deep learning series. In this episode, the panelists discuss their experiences “doing more with fewer parameters: Convnets, RNNs, attention & transformers, generative models (VAEs & GANs). Panelists
The other panels:
| |||
02 Aug 2018 | BI 001 Steven Potter: Brains in Dishes | 00:41:58 | |
Find out more about Steve at his website. I discovered him when I found his book chapter “What Can AI Get from Neuroscience?” in the following: “50 Years of Artificial Intelligence: Essays Dedicated to the 50th Anniversary of Artificial Intelligence,” M. Lungarella, J. Bongard, & R. Pfeifer (eds.) (pp. 174-185). Berlin: Springer-Verlag. Download the chapter. Link to the whole book at Springer. These days Steve is semi-retired, but is an active consultant for high-tech startups, companies, or individuals. Things mentioned in the show (check out his part 2 episode for more links!)
| |||
02 Aug 2018 | BI 002 Steven Potter Part 2: Brains in Dishes | 01:11:23 | |
Find out more about Steve at his website. Things mentioned during the show:
Extra fun stuff
| |||
02 Aug 2018 | BI 003 Blake Porter: Effortful Rats | 00:42:47 | |
Mentioned during the show:
| |||
02 Aug 2018 | BI 004 Mark Humphries: Learning to Remember | 00:41:39 | |
Mentioned in the show:
| |||
19 Aug 2021 | BI NMA 06: Advancing Neuro Deep Learning Panel | 01:20:32 | |
26 Aug 2021 | BI 112 Ali Mohebi and Ben Engelhard: The Many Faces of Dopamine | 01:13:56 | |
BI 112:Ali Mohebi and Ben EngelhardThe Many Faces of DopamineAnnouncement:Ben has started his new lab and is recruiting grad students. Check out his lab here and apply! Engelhard LabAli and Ben discuss the ever-expanding discoveries about the roles dopamine plays for our cognition. Dopamine is known to play a role in learning – dopamine (DA) neurons fire when our reward expectations aren’t met, and that signal helps adjust our expectation. Roughly, DA corresponds to a reward prediction error. The reward prediction error has helped reinforcement learning in AI develop into a raging success, specially with deep reinforcement learning models trained to out-perform humans in games like chess and Go. But DA likely contributes a lot more to brain function. We discuss many of those possible roles, how to think about computation with respect to neuromodulators like DA, how different time and spatial scales interact, and more. Dopamine: A Simple AND Complex Story GuestsTimestamps: 0:00 – Intro 5:02 – Virtual Dopamine Conference 9:56 – History of dopamine’s roles 16:47 – Dopamine circuits 21:13 – Multiple roles for dopamine 31:43 – Deep learning panel discussion 50:14 – Computation and neuromodulation | |||
02 Sep 2021 | BI ViDA Panel Discussion: Deep RL and Dopamine | 00:57:25 | |
12 Sep 2021 | BI 113 David Barack and John Krakauer: Two Views On Cognition | 01:30:38 | |
Support the show to get full episodes and join the Discord community. David and John discuss some of the concepts from their recent paper Two Views on the Cognitive Brain, in which they argue the recent population-based dynamical systems approach is a promising route to understanding brain activity underpinning higher cognition. We discuss mental representations, the kinds of dynamical objects being used for explanation, and much more, including David's perspectives as a practicing neuroscientist and philosopher.
Timestamps 0:00 - Intro 3:13 - David's philosophy and neuroscience experience 20:01 - Renaissance person 24:36 - John's medical training 31:58 - Two Views on the Cognitive Brain 44:18 - Representation 49:37 - Studying populations of neurons 1:05:17 - What counts as representation 1:18:49 - Does this approach matter for AI? | |||
22 Sep 2021 | BI 114 Mark Sprevak and Mazviita Chirimuuta: Computation and the Mind | 01:38:07 | |
Support the show to get full episodes and join the Discord community. Mark and Mazviita discuss the philosophy and science of mind, and how to think about computations with respect to understanding minds. Current approaches to explaining brain function are dominated by computational models and the computer metaphor for brain and mind. But there are alternative ways to think about the relation between computations and brain function, which we explore in the discussion. We also talk about the role of philosophy broadly and with respect to mind sciences, pluralism and perspectival approaches to truth and understanding, the prospects and desirability of naturalizing representations (accounting for how brain representations relate to the natural world), and much more.
0:00 - Intro 5:26 - Philosophy contributing to mind science 15:45 - Trend toward hyperspecialization 21:38 - Practice-focused philosophy of science 30:42 - Computationalism 33:05 - Philosophy of mind: identity theory, functionalism 38:18 - Computations as descriptions 41:27 - Pluralism and perspectivalism 54:18 - How much of brain function is computation? 1:02:11 - AI as computationalism 1:13:28 - Naturalizing representations 1:30:08 - Are you doing it right? | |||
02 Oct 2021 | BI 115 Steve Grossberg: Conscious Mind, Resonant Brain | 01:23:41 | |
Support the show to get full episodes and join the Discord community. Steve and I discuss his book Conscious Mind, Resonant Brain: How Each Brain Makes a Mind. The book is a huge collection of his models and their predictions and explanations for a wide array of cognitive brain functions. Many of the models spring from his Adaptive Resonance Theory (ART) framework, which explains how networks of neurons deal with changing environments while maintaining self-organization and retaining learned knowledge. ART led Steve to the hypothesis that all conscious states are resonant states, which we discuss. There are also guest questions from György Buzsáki, Jay McClelland, and John Krakauer.
0:00 - Intro 2:38 - Conscious Mind, Resonant Brain 11:49 - Theoretical method 15:54 - ART, learning, and consciousness 22:58 - Conscious vs. unconscious resonance 26:56 - Györy Buzsáki question 30:04 - Remaining mysteries in visual system 35:16 - John Krakauer question 39:12 - Jay McClelland question 51:34 - Any missing principles to explain human cognition? 1:00:16 - Importance of an early good career start 1:06:50 - Has modeling training caught up to experiment training? 1:17:12 - Universal development code | |||
02 Aug 2018 | BI 005 David Sussillo: RNNs are Back! | 00:45:39 | |
Mentioned in the show:
| |||
12 Oct 2021 | BI 116 Michael W. Cole: Empirical Neural Networks | 01:31:20 | |
Support the show to get full episodes and join the Discord community. Mike and I discuss his modeling approach to study cognition. Many people I have on the podcast use deep neural networks to study brains, where the idea is to train or optimize the model to perform a task, then compare the model properties with brain properties. Mike's approach is different in at least two ways. One, he builds the architecture of his models using connectivity data from fMRI recordings. Two, he doesn't train his models; instead, he uses functional connectivity data from the fMRI recordings to assign weights between nodes of the network (in deep learning, the weights are learned through lots of training). Mike calls his networks empirically-estimated neural networks (ENNs), and/or network coding models. We walk through his approach, what we can learn from models like ENNs, discuss some of his earlier work on cognitive control and our ability to flexibly adapt to new task rules through instruction, and he fields questions from Kanaka Rajan, Kendrick Kay, and Patryk Laurent.
0:00 - Intro 4:58 - Cognitive control 7:44 - Rapid Instructed Task Learning and Flexible Hub Theory 15:53 - Patryk Laurent question: free will 26:21 - Kendrick Kay question: fMRI limitations 31:55 - Empirically-estimated neural networks (ENNs) 40:51 - ENNs vs. deep learning 45:30 - Clinical relevance of ENNs 47:32 - Kanaka Rajan question: a proposed collaboration 56:38 - Advantage of modeling multiple regions 1:05:30 - How ENNs work 1:12:48 - How ENNs might benefit artificial intelligence 1:19:04 - The need for causality 1:24:38 - Importance of luck and serendipity | |||
19 Oct 2021 | BI 117 Anil Seth: Being You | 01:32:09 | |
Support the show to get full episodes and join the Discord community. Anil and I discuss a range of topics from his book, BEING YOU A New Science of Consciousness. Anil lays out his framework for explaining consciousness, which is embedded in what he calls the "real problem" of consciousness. You know the "hard problem", which was David Chalmers term for our eternal difficulties to explain why we have subjective awareness at all instead of being unfeeling, unexperiencing machine-like organisms. Anil's "real problem" aims to explain, predict, and control the phenomenal properties of consciousness, and his hope is that, by doing so, the hard problem of consciousness will dissolve much like the mystery of explaining life dissolved with lots of good science. Anil's account of perceptual consciousness, like seeing red, is that it's rooted in predicting our incoming sensory data. His account of our sense of self, is that it's rooted in predicting our bodily states to control them. We talk about that and a lot of other topics from the book, like consciousness as "controlled hallucinations", free will, psychedelics, complexity and emergence, and the relation between life, intelligence, and consciousness. Plus, Anil answers a handful of questions from Megan Peters and Steve Fleming, both previous brain inspired guests.
0:00 - Intro 6:32 - Megan Peters Q: Communicating Consciousness 15:58 - Human vs. animal consciousness 19:12 - BEING YOU A New Science of Consciousness 20:55 - Megan Peters Q: Will the hard problem go away? 30:55 - Steve Fleming Q: Contents of consciousness 41:01 - Megan Peters Q: Phenomenal character vs. content 43:46 - Megan Peters Q: Lempels of complexity 52:00 - Complex systems and emergence 55:53 - Psychedelics 1:06:04 - Free will 1:19:10 - Consciousness vs. life vs. intelligence | |||
01 Nov 2021 | BI 118 Johannes Jäger: Beyond Networks | 01:36:08 | |
Support the show to get full episodes and join the Discord community. Johannes (Yogi) is a freelance philosopher, researcher & educator. We discuss many of the topics in his online course, Beyond Networks: The Evolution of Living Systems. The course is focused on the role of agency in evolution, but it covers a vast range of topics: process vs. substance metaphysics, causality, mechanistic dynamic explanation, teleology, the important role of development mediating genotypes, phenotypes, and evolution, what makes biological organisms unique, the history of evolutionary theory, scientific perspectivism, and a view toward the necessity of including agency in evolutionary theory. I highly recommend taking his course. We also discuss the role of agency in artificial intelligence, how neuroscience and evolutionary theory are undergoing parallel re-evaluations, and Yogi answers a guest question from Kevin Mitchell.
0:00 - Intro 4:10 - Yogi's background 11:00 - Beyond Networks - limits of dynamical systems models 16:53 - Kevin Mitchell question 20:12 - Process metaphysics 26:13 - Agency in evolution 40:37 - Agent-environment interaction, open-endedness 45:30 - AI and agency 55:40 - Life and intelligence 59:08 - Deep learning and neuroscience 1:03:21 - Mental autonomy 1:06:10 - William Wimsatt's biopsychological thicket 1:11:23 - Limtiations of mechanistic dynamic explanation 1:18:53 - Synthesis versus multi-perspectivism 1:30:31 - Specialization versus generalization | |||
25 Aug 2018 | BI 006 Ryan Poplin: Deep Solutions | 00:37:16 | |
[bctt tweet=”Check out episode 6 of the Brain Inspired podcast: Deep learning, eyeballs, and brains” username=”pgmid”]
Mentioned in the show
| |||
11 Nov 2021 | BI 119 Henry Yin: The Crisis in Neuroscience | 01:06:36 | |
Support the show to get full episodes and join the Discord community. Henry and I discuss why he thinks neuroscience is in a crisis (in the Thomas Kuhn sense of scientific paradigms, crises, and revolutions). Henry thinks our current concept of the brain as an input-output device, with cognition in the middle, is mistaken. He points to the failure of neuroscience to successfully explain behavior despite decades of research. Instead, Henry proposes the brain is one big hierarchical set of control loops, trying to control their output with respect to internally generated reference signals. He was inspired by control theory, but points out that most control theory for biology is flawed by not recognizing that the reference signals are internally generated. Instead, most control theory approaches, and neuroscience research in general, assume the reference signals are what gets externally supplied... by the experimenter.
0:00 - Intro 5:40 - Kuhnian crises 9:32 - Control theory and cybernetics 17:23 - How much of brain is control system? 20:33 - Higher order control representation 23:18 - Prediction and control theory 27:36 - The way forward 31:52 - Compatibility with mental representation 38:29 - Teleology 45:53 - The right number of subjects 51:30 - Continuous measurement 57:06 - Artificial intelligence and control theory | |||
21 Nov 2021 | BI 120 James Fitzgerald, Andrew Saxe, Weinan Sun: Optimizing Memories | 01:40:02 | |
Support the show to get full episodes and join the Discord community. James, Andrew, and Weinan discuss their recent theory about how the brain might use complementary learning systems to optimize our memories. The idea is that our hippocampus creates our episodic memories for individual events, full of particular details. And through a complementary process, slowly consolidates those memories within our neocortex through mechanisms like hippocampal replay. The new idea in their work suggests a way for the consolidated cortical memory to become optimized for generalization, something humans are known to be capable of but deep learning has yet to build. We discuss what their theory predicts about how the "correct" process depends on how much noise and variability there is in the learning environment, how their model solves this, and how it relates to our brain and behavior.
0:00 - Intro 3:57 - Guest Intros 15:04 - Organizing memories for generalization 26:48 - Teacher, student, and notebook models 30:51 - Shallow linear networks 33:17 - How to optimize generalization 47:05 - Replay as a generalization regulator 54:57 - Whole greater than sum of its parts 1:05:37 - Unpredictability 1:10:41 - Heuristics 1:13:52 - Theoretical neuroscience for AI 1:29:42 - Current personal thinking | |||
02 Dec 2021 | BI 121 Mac Shine: Systems Neurobiology | 01:43:12 | |
Support the show to get full episodes and join the Discord community. Mac and I discuss his systems level approach to understanding brains, and his theoretical work suggesting important roles for the thalamus, basal ganglia, and cerebellum, shifting the dynamical landscape of brain function within varying behavioral contexts. We also discuss his recent interest in the ascending arousal system and neuromodulators. Mac thinks the neocortex has been the sole focus of too much neuroscience research, and that the subcortical brain regions and circuits have a much larger role underlying our intelligence.
0:00 - Intro 6:32 - Background 10:41 - Holistic approach 18:19 - Importance of thalamus 35:19 - Thalamus circuitry 40:30 - Cerebellum 46:15 - Predictive processing 49:32 - Brain as dynamical attractor landscape 56:48 - System 1 and system 2 1:02:38 - How to think about the thalamus 1:06:45 - Causality in complex systems 1:11:09 - Clinical applications 1:15:02 - Ascending arousal system and neuromodulators 1:27:48 - Implications for AI 1:33:40 - Career serendipity 1:35:12 - Advice | |||
12 Dec 2021 | BI 122 Kohitij Kar: Visual Intelligence | 01:33:18 | |
Support the show to get full episodes and join the Discord community. Ko and I discuss a range of topics around his work to understand our visual intelligence. Ko was a postdoc in James Dicarlo's lab, where he helped develop the convolutional neural network models that have become the standard for explaining core object recognition. He is starting his own lab at York University, where he will continue to expand and refine the models, adding important biological details and incorporating models for brain areas outside the ventral visual stream. He will also continue recording neural activity, and performing perturbation studies to better understand the networks involved in our visual cognition.
0:00 - Intro 3:49 - Background 13:51 - Where are we in understanding vision? 19:46 - Benchmarks 21:21 - Falsifying models 23:19 - Modeling vs. experiment speed 29:26 - Simple vs complex models 35:34 - Dorsal visual stream and deep learning 44:10 - Modularity and brain area roles 50:58 - Chemogenetic perturbation, DREADDs 57:10 - Future lab vision, clinical applications 1:03:55 - Controlling visual neurons via image synthesis 1:12:14 - Is it enough to study nonhuman animals? 1:18:55 - Neuro/AI intersection 1:26:54 - What is intelligence? | |||
26 Dec 2021 | BI 123 Irina Rish: Continual Learning | 01:18:59 | |
Support the show to get full episodes and join the Discord community. Irina is a faculty member at MILA-Quebec AI Institute and a professor at Université de Montréal. She has worked from both ends of the neuroscience/AI interface, using AI for neuroscience applications, and using neural principles to help improve AI. We discuss her work on biologically-plausible alternatives to back-propagation, using "auxiliary variables" in addition to the normal connection weight updates. We also discuss the world of lifelong learning, which seeks to train networks in an online manner to improve on any tasks as they are introduced. Catastrophic forgetting is an obstacle in modern deep learning, where a network forgets old tasks when it is trained on new tasks. Lifelong learning strategies, like continual learning, transfer learning, and meta-learning seek to overcome catastrophic forgetting, and we talk about some of the inspirations from neuroscience being used to help lifelong learning in networks.
0:00 - Intro 3:26 - AI for Neuro, Neuro for AI 14:59 - Utility of philosophy 20:51 - Artificial general intelligence 24:34 - Back-propagation alternatives 35:10 - Inductive bias vs. scaling generic architectures 45:51 - Continual learning 59:54 - Neuro-inspired continual learning 1:06:57 - Learning trajectories | |||
05 Jan 2022 | BI 124 Peter Robin Hiesinger: The Self-Assembling Brain | 01:39:27 | |
Support the show to get full episodes and join the Discord community. Robin and I discuss many of the ideas in his book The Self-Assembling Brain: How Neural Networks Grow Smarter. The premise is that our DNA encodes an algorithmic growth process that unfolds information via time and energy, resulting in a connected neural network (our brains!) imbued with vast amounts of information from the "start". This contrasts with modern deep learning networks, which start with minimal initial information in their connectivity, and instead rely almost solely on learning to gain their function. Robin suggests we won't be able to create anything with close to human-like intelligence unless we build in an algorithmic growth process and an evolutionary selection process to create artificial networks.
0:00 - Intro 3:01 - The Self-Assembling Brain 21:14 - Including growth in networks 27:52 - Information unfolding and algorithmic growth 31:27 - Cellular automata 40:43 - Learning as a continuum of growth 45:01 - Robustness, autonomous agents 49:11 - Metabolism vs. connectivity 58:00 - Feedback at all levels 1:05:32 - Generality vs. specificity 1:10:36 - Whole brain emulation 1:20:38 - Changing view of intelligence 1:26:34 - Popular and wrong vs. unknown and right | |||
19 Jan 2022 | BI 125 Doris Tsao, Tony Zador, Blake Richards: NAISys | 01:11:05 | |
Support the show to get full episodes and join the Discord community. Doris, Tony, and Blake are the organizers for this year's NAISys conference, From Neuroscience to Artificially Intelligent Systems (NAISys), at Cold Spring Harbor. We discuss the conference itself, some history of the neuroscience and AI interface, their current research interests, and a handful of topics around evolution, innateness, development, learning, and the current and future prospects for using neuroscience to inspire new ideas in artificial intelligence.
0:00 - Intro 4:16 - Tony Zador 5:38 - Doris Tsao 10:44 - Blake Richards 15:46 - Deductive, inductive, abductive inference 16:32 - NAISys 33:09 - Evolution, development, learning 38:23 - Learning: plasticity vs. dynamical structures 54:13 - Different kinds of understanding 1:03:05 - Do we understand evolution well enough? 1:04:03 - Neuro-AI fad? 1:06:26 - Are your problems bigger or smaller now? | |||
31 Jan 2022 | BI 126 Randy Gallistel: Where Is the Engram? | 01:19:57 | |
Support the show to get full episodes and join the Discord community. Randy and I discuss his long-standing interest in how the brain stores information to compute. That is, where is the engram, the physical trace of memory in the brain? Modern neuroscience is dominated by the view that memories are stored among synaptic connections in populations of neurons. Randy believes a more reasonable and reliable way to store abstract symbols, like numbers, is to write them into code within individual neurons. Thus, the spiking code, whatever it is, functions to write and read memories into and out of intracellular substrates, like polynucleotides (DNA, RNA, e.g.). He lays out his case in detail in his book with Adam King, Memory and the Computational Brain: Why Cognitive Science will Transform Neuroscience. We also talk about some research and theoretical work since then that support his views.
0:00 - Intro 6:50 - Cognitive science vs. computational neuroscience 13:23 - Brain as computing device 15:45 - Noam Chomsky's influence 17:58 - Memory must be stored within cells 30:58 - Theoretical support for the idea 34:15 - Cerebellum evidence supporting the idea 40:56 - What is the write mechanism? 51:11 - Thoughts on deep learning 1:00:02 - Multiple memory mechanisms? 1:10:56 - The role of plasticity 1:12:06 - Trying to convince molecular biologists | |||
10 Feb 2022 | BI 127 Tomás Ryan: Memory, Instinct, and Forgetting | 01:42:39 | |
Support the show to get full episodes and join the Discord community. Tomás and I discuss his research and ideas on how memories are encoded (the engram), the role of forgetting, and the overlapping mechanisms of memory and instinct. Tomás uses otpogenetics and other techniques to label and control neurons involved in learning and memory, and has shown that forgotten memories can be restored by stimulating "engram cells" originally associated with the forgotten memory. This line of research has led Tomás to think forgetting might be a learning mechanism itself, a adaption our brains make based on the predictability and affordances of the environment. His work on engrams has also led Tomás to think our instincts (ingrams) may share the same mechanism of our memories (engrams), and that memories may transition to instincts across generations. We begin by addressing Randy Gallistel's engram ideas from the previous episode: BI 126 Randy Gallistel: Where Is the Engram?
0:00 - Intro 4:05 - Response to Randy Gallistel 10:45 - Computation in the brain 14:52 - Instinct and memory 19:37 - Dynamics of memory 21:55 - Wiring vs. connection strength plasticity 24:16 - Changing one's mind 33:09 - Optogenetics and memory experiments 47:24 - Forgetting as learning 1:06:35 - Folk psychological terms 1:08:49 - Memory becoming instinct 1:21:49 - Instinct across the lifetime 1:25:52 - Boundaries of memories 1:28:52 - Subjective experience of memory 1:31:58 - Interdisciplinary research 1:37:32 - Communicating science | |||
20 Feb 2022 | BI 128 Hakwan Lau: In Consciousness We Trust | 01:25:40 | |
Support the show to get full episodes and join the Discord community. Hakwan and I discuss many of the topics in his new book, In Consciousness we Trust: The Cognitive Neuroscience of Subjective Experience. Hakwan describes his perceptual reality monitoring theory of consciousness, which suggests consciousness may act as a systems check between our sensory perceptions and higher cognitive functions. We also discuss his latest thoughts on mental quality space and how it relates to perceptual reality monitoring. Among many other topics, we chat about the many confounds and challenges to empirically studying consciousness, a topic featured heavily in the first half of his book. Hakwan was on a previous episode with Steve Fleming, BI 099 Hakwan Lau and Steve Fleming: Neuro-AI Consciousness.
0:00 - Intro 4:37 - In Consciousness We Trust 12:19 - Too many consciousness theories? 19:26 - Philosophy and neuroscience of consciousness 29:00 - Local vs. global theories 31:20 - Perceptual reality monitoring and GANs 42:43 - Functions of consciousness 47:17 - Mental quality space 56:44 - Cognitive maps 1:06:28 - Performance capacity confounds 1:12:28 - Blindsight 1:19:11 - Philosophy vs. empirical work | |||
02 Mar 2022 | BI 129 Patryk Laurent: Learning from the Real World | 01:21:01 | |
Support the show to get full episodes and join the Discord community. Patryk and I discuss his wide-ranging background working in both the neuroscience and AI worlds, and his resultant perspective on what's needed to move forward in AI, including some principles of brain processes that are more and less important. We also discuss his own work using some of those principles to help deep learning generalize to better capture how humans behave in and perceive the world.
0:00 - Intro 2:22 - Patryk's background 8:37 - Importance of diverse skills 16:14 - What is intelligence? 20:34 - Important brain principles 22:36 - Learning from the real world 35:09 - Language models 42:51 - AI contribution to neuroscience 48:22 - Criteria for "real" AI 53:11 - Neuroscience for AI 1:01:20 - What can we ignore about brains? 1:11:45 - Advice to past self | |||
13 Mar 2022 | BI 130 Eve Marder: Modulation of Networks | 01:00:56 | |
Support the show to get full episodes and join the Discord community. Eve discusses many of the lessons she has learned studying a small nervous system, the crustacean stomatogastric nervous system (STG). The STG has only about 30 neurons and its connections and neurophysiology are well-understood. Yet Eve's work has shown it functions under a remarkable diversity of conditions, and does so is a remarkable variety of ways. We discuss her work on the STG specifically, and what her work implies about trying to study much larger nervous systems, like our human brains.
0:00 - Intro 3:58 - Background 8:00 - Levels of ambiguity 9:47 - Stomatogastric nervous system 17:13 - Structure vs. function 26:08 - Role of theory 34:56 - Technology vs. understanding 38:25 - Higher cognitive function 44:35 - Adaptability, resilience, evolution 50:23 - Climate change 56:11 - Deep learning 57:12 - Dynamical systems | |||
26 Mar 2022 | BI 131 Sri Ramaswamy and Jie Mei: Neuromodulation-aware DNNs | 01:26:52 | |
Support the show to get full episodes and join the Discord community. Sri and Mei join me to discuss how including principles of neuromodulation in deep learning networks may improve network performance. It's an ever-present question how much detail to include in models, and we are in the early stages of learning how neuromodulators and their interactions shape biological brain function. But as we continue to learn more, Sri and Mei are interested in building "neuromodulation-aware DNNs".
0:00 - Intro 3:10 - Background 9:19 - Bottom-up vs. top-down 14:42 - Levels of abstraction 22:46 - Biological neuromodulation 33:18 - Inventing neuromodulators 41:10 - How far along are we? 53:31 - Multiple realizability 1:09:40 -Modeling dendrites 1:15:24 - Across-species neuromodulation | |||
03 Apr 2022 | BI 132 Ila Fiete: A Grid Scaffold for Memory | 01:17:20 | |
Announcement:I'm releasing my Neuro-AI course April 10-13, after which it will be closed for some time. Learn more here. Support the show to get full episodes and join the Discord community. Ila discusses her theoretical neuroscience work suggesting how our memories are formed within the cognitive maps we use to navigate the world and navigate our thoughts. The main idea is that grid cell networks in the entorhinal cortex internally generate a structured scaffold, which gets sent to the hippocampus. Neurons in the hippocampus, like the well-known place cells, receive that scaffolding and also receive external signals from the neocortex- signals about what's happening in the world and in our thoughts. Thus, the place cells act to "pin" what's happening in our neocortex to the scaffold, forming a memory. We also discuss her background as a physicist and her approach as a "neurophysicist", and a review she's publishing all about the many brain areas and cognitive functions being explained as attractor landscapes within a dynamical systems framework.
0:00 - Intro 3:36 - "Neurophysicist" 9:30 - Bottom-up vs. top-down 15:57 - Tool scavenging 18:21 - Cognitive maps and hippocampus 22:40 - Hopfield networks 27:56 - Internal scaffold 38:42 - Place cells 43:44 - Grid cells 54:22 - Grid cells encoding place cells 59:39 - Scaffold model: stacked hopfield networks 1:05:39 - Attractor landscapes 1:09:22 - Landscapes across scales 1:12:27 - Dimensionality of landscapes | |||
15 Apr 2022 | BI 133 Ken Paller: Lucid Dreaming, Memory, and Sleep | 01:29:14 | |
Support the show to get full episodes and join the Discord community. Check out my free video series about what's missing in AI and Neuroscience Ken discusses the recent work in his lab that allows communication with subjects while they experience lucid dreams. This new paradigm opens many avenues to study the neuroscience and psychology of consciousness, sleep, dreams, memory, and learning, and to improve and optimize sleep for cognition. Ken and his team are developing a Lucid Dreaming App which is freely available via his lab. We also discuss much of his work on memory and learning in general and specifically related to sleep, like reactivating specific memories during sleep to improve learning.
0:00 - Intro 2:48 - Background and types of memory 14:44 -Consciousness and memory 23:32 - Phases and sleep and wakefulness 28:19 - Sleep, memory, and learning 33:50 - Targeted memory reactivation 48:34 - Problem solving during sleep 51:50 - 2-way communication with lucid dreamers 1:01:43 - Confounds to the paradigm 1:04:50 - Limitations and future studies 1:09:35 - Lucid dreaming app 1:13:47 - How sleep can inform AI 1:20:18 - Advice for students | |||
27 Apr 2022 | BI 134 Mandyam Srinivasan: Bee Flight and Cognition | 01:26:17 | |
Support the show to get full episodes and join the Discord community. Check out my free video series about what's missing in AI and Neuroscience Srini is Emeritus Professor at Queensland Brain Institute in Australia. In this episode, he shares his wide range of behavioral experiments elucidating the principles of flight and navigation in insects. We discuss how bees use optic flow signals to determine their speed, distance, proximity to objects, and to gracefully land. These abilities are largely governed via control systems, balancing incoming perceptual signals with internal reference signals. We also talk about a few of the aerial robotics projects his research has inspired, many of the other cognitive skills bees can learn, the possibility of their feeling pain , and the nature of their possible subjective conscious experience.
0:00 - Intro 3:34 - Background 8:20 - Bee experiments 14:30 - Bee flight and navigation 28:05 - Landing 33:06 - Umwelt and perception 37:26 - Bee-inspired aerial robotics 49:10 - Motion camouflage 51:52 - Cognition in bees 1:03:10 - Small vs. big brains 1:06:42 - Pain in bees 1:12:50 - Subjective experience 1:15:25 - Deep learning 1:23:00 - Path forward | |||
06 May 2022 | BI 135 Elena Galea: The Stars of the Brain | 01:17:25 | |
Support the show to get full episodes and join the Discord community. Check out my free video series about what's missing in AI and Neuroscience Brains are often conceived as consisting of neurons and "everything else." As Elena discusses, the "everything else," including glial cells and in particular astrocytes, have largely been ignored in neuroscience. That's partly because the fast action potentials of neurons have been assumed to underlie computations in the brain, and because technology only recently afforded closer scrutiny of astrocyte activity. Now that we can record calcium signaling in astrocytes, it's possible to relate how astrocyte signaling with each other and with neurons may complement the cognitive roles once thought the sole domain of neurons. Although the computational role of astrocytes remains unclear, it is clear that astrocytes interact with neurons and neural circuits in dynamic and interesting ways. We talk about the historical story of astrocytes, the emerging modern story, and Elena shares her views on the path forward to understand astrocyte function in cognition, disease, homeostasis, and - Elena's favorite current hypothesis - their integrative role in negative feedback control.
0:00 - Intro 5:23 - The changing story of astrocytes 14:58 - Astrocyte research lags neuroscience 19:45 - Types of astrocytes 23:06 - Astrocytes vs neurons 26:08 - Computational roles of astrocytes 35:45 - Feedback control 43:37 - Energy efficiency 46:25 - Current technology 52:58 - Computational astroscience 1:10:57 - Do names for things matter | |||
17 May 2022 | BI 136 Michel Bitbol and Alex Gomez-Marin: Phenomenology | 01:34:12 | |
Support the show to get full episodes and join the Discord community. Check out my free video series about what's missing in AI and Neuroscience Michel Bitbol is Director of Research at CNRS (Centre National de la Recherche Scientifique). Alex Gomez-Marin is a neuroscientist running his lab, The Behavior of Organisms Laboratory, at the Instituto de Neurociencias in Alicante. We discuss phenomenology as an alternative perspective on our scientific endeavors. Although we like to believe our science is objective and explains the reality of the world we inhabit, we can't escape the fact that all of our scientific knowledge comes through our perceptions and interpretations as conscious living beings. Michel has used phenomenology to resolve many of the paradoxes that quantum mechanics generates when it is understood as a description of reality, and more recently he has applied phenomenology to the philosophy of mind and consciousness. Alex is currently trying to apply the phenomenological approach to his research on brains and behavior. Much of our conversation revolves around how phenomenology and our "normal" scientific explorations can co-exist, including the study of minds, brains, and intelligence- our own and that of other organisms. We also discuss the "blind spot" of science, the history and practice of phenomenology, various kinds of explanation, the language we use to describe things, and more.
0:00 - Intro 4:32 - The Blind Spot 15:53 - Phenomenology and interpretation 22:51 - Personal stories: appreciating phenomenology 37:42 - Quantum physics example 47:16 - Scientific explanation vs. phenomenological description 59:39 - How can phenomenology and science complement each other? 1:08:22 - Neurophenomenology 1:17:34 - Use of language 1:25:46 - Mutual constraints | |||
27 May 2022 | BI 137 Brian Butterworth: Can Fish Count? | 01:17:49 | |
Check out my free video series about what's missing in AI and Neuroscience Support the show to get full episodes and join the Discord community. Brian Butterworth is Emeritus Professor of Cognitive Neuropsychology at University College London. In his book, Can Fish Count?: What Animals Reveal About Our Uniquely Mathematical Minds, he describes the counting and numerical abilities across many different species, suggesting our ability to count is evolutionarily very old (since many diverse species can count). We discuss many of the examples in his book, the mathematical disability dyscalculia and its relation to dyslexia, how to test counting abilities in various species, how counting may happen in brains, the promise of creating artificial networks that can do math, and many more topics.
0:00 - Intro 3:19 - Why Counting? 5:31 - Dyscalculia 12:06 - Dyslexia 19:12 - Counting 26:37 - Origins of counting vs. language 34:48 - Counting vs. higher math 46:46 - Counting some things and not others 53:33 - How to test counting 1:03:30 - How does the brain count? 1:13:10 - Are numbers real? | |||
06 Jun 2022 | BI 138 Matthew Larkum: The Dendrite Hypothesis | 01:51:42 | |
Check out my free video series about what's missing in AI and Neuroscience Support the show to get full episodes and join the Discord community. Matthew Larkum runs his lab at Humboldt University of Berlin, where his group studies how dendrites contribute to computations within and across layers of the neocortex. Since the late 1990s, Matthew has continued to uncover key properties of the way pyramidal neurons stretch across layers of the cortex, their dendrites receiving inputs from those different layers - and thus different brain areas. For example, layer 5 pyramidal neurons have a set of basal dendrites near the cell body that receives feedforward-like input, and a set of apical dendrites all the way up in layer 1 that receives feedback--like input. Depending on which set of dendrites is receiving input, or neither or both, the neuron's output functions in different modes- silent, regular spiking, or burst spiking. Matthew realized the different sets of dendritic inputs could signal different operations, often pairing feedforward sensory--like signals and feedback context-like signals. His research has shown this kind of coincidence detection is important for cognitive functions like perception, memory, learning, and even wakefulness. We discuss many of his ideas and research findings, why dendrites have long been neglected in favor of neuron cell bodies, the possibility of learning about computations by studying implementation-level phenomena, and much more.
0:00 - Intro 5:31 - Background: Dendrites 23:20 - Cortical neuron bodies vs. branches 25:47 - Theories of cortex 30:49 - Feedforward and feedback hierarchy 37:40 - Dendritic integration hypothesis 44:32 - DIT vs. other consciousness theories 51:30 - Mac Shine Q1 1:04:38 - Are dendrites conceptually useful? 1:09:15 - Insights from implementation level 1:24:44 - How detailed to model? 1:28:15 - Do action potentials cause consciousness? 1:40:33 - Mac Shine Q2 | |||
20 Jun 2022 | BI 139 Marc Howard: Compressed Time and Memory | 01:20:11 | |
Check out my free video series about what's missing in AI and Neuroscience Support the show to get full episodes and join the Discord community. Marc Howard runs his Theoretical Cognitive Neuroscience Lab at Boston University, where he develops mathematical models of cognition, constrained by psychological and neural data. In this episode, we discuss the idea that a Laplace transform and its inverse may serve as a unified framework for memory. In short, our memories are compressed on a continuous log-scale: as memories get older, their representations "spread out" in time. It turns out this kind of representation seems ubiquitous in the brain and across cognitive functions, suggesting it is likely a canonical computation our brains use to represent a wide variety of cognitive functions. We also discuss some of the ways Marc is incorporating this mathematical operation in deep learning nets to improve their ability to handle information at different time scales.
0:00 - Intro 4:57 - Main idea: Laplace transforms 12:00 - Time cells 20:08 - Laplace, compression, and time cells 25:34 - Everywhere in the brain 29:28 - Episodic memory 35:11 - Randy Gallistel's memory idea 40:37 - Adding Laplace to deep nets 48:04 - Reinforcement learning 1:00:52 - Brad Wyble Q: What gets filtered out? 1:05:38 - Replay and complementary learning systems 1:11:52 - Howard Goldowsky Q: Gyorgy Buzsaki 1:15:10 - Obstacles | |||
30 Jun 2022 | BI 140 Jeff Schall: Decisions and Eye Movements | 01:20:22 | |
Check out my free video series about what's missing in AI and Neuroscience Support the show to get full episodes and join the Discord community. Jeff Schall is the director of the Center for Visual Neurophysiology at York University, where he runs the Schall Lab. His research centers around studying the mechanisms of our decisions, choices, movement control, and attention within the saccadic eye movement brain systems and in mathematical psychology models- in other words, how we decide where and when to look. Jeff was my postdoctoral advisor at Vanderbilt University, and I wanted to revisit a few guiding principles he instills in all his students. Linking Propositions by Davida Teller are a series of logical statements to ensure we rigorously connect the brain activity we record to the psychological functions we want to explain. Strong Inference by John Platt is the scientific method on steroids - a way to make our scientific practice most productive and efficient. We discuss both of these topics in the context of Jeff's eye movement and decision-making science. We also discuss how neurophysiology has changed over the past 30 years, we compare the relatively small models he employs with the huge deep learning models, many of his current projects, and plenty more. If you want to learn more about Jeff's work and approach, I recommend reading in order two of his review papers we discuss as well. One was written 20 years ago (On Building a Bridge Between Brain and Behavior), and the other 2-ish years ago (Accumulators, Neurons, and Response Time).
0:00 - Intro 6:51 - Neurophysiology old and new 14:50 - Linking propositions 24:18 - Psychology working with neurophysiology 35:40 - Neuron doctrine, population doctrine 40:28 - Strong Inference and deep learning 46:37 - Model mimicry 51:56 - Scientific fads 57:07 - Current projects 1:06:38 - On leaving academia 1:13:51 - How academia has changed for better and worse | |||
12 Jul 2022 | BI 141 Carina Curto: From Structure to Dynamics | 01:31:40 | |
Check out my free video series about what's missing in AI and Neuroscience Support the show to get full episodes and join the Discord community. Carina Curto is a professor in the Department of Mathematics at The Pennsylvania State University. She uses her background skills in mathematical physics/string theory to study networks of neurons. On this episode, we discuss the world of topology in neuroscience - the study of the geometrical structures mapped out by active populations of neurons. We also discuss her work on "combinatorial linear threshold networks" (CLTNs). Unlike the large deep learning models popular today as models of brain activity, the CLTNs Carina builds are relatively simple, abstracted graphical models. This property is important to Carina, whose goal is to develop mathematically tractable neural network models. Carina has worked out how the structure of many CLTNs allows prediction of the model's allowable dynamics, how motifs of model structure can be embedded in larger models while retaining their dynamical features, and more. The hope is that these elegant models can tell us more about the principles our messy brains employ to generate the robust and beautiful dynamics underlying our cognition.
0:00 - Intro 4:25 - Background: Physics and math to study brains 20:45 - Beautiful and ugly models 35:40 - Topology 43:14 - Topology in hippocampal navigation 56:04 - Topology vs. dynamical systems theory 59:10 - Combinatorial linear threshold networks 1:25:26 - How much more math do we need to invent? | |||
26 Jul 2022 | BI 142 Cameron Buckner: The New DoGMA | 01:43:16 | |
Check out my free video series about what's missing in AI and Neuroscience Support the show to get full episodes and join the Discord community. Cameron Buckner is a philosopher and cognitive scientist at The University of Houston. He is writing a book about the age-old philosophical debate on how much of our knowledge is innate (nature, rationalism) versus how much is learned (nurture, empiricism). In the book and his other works, Cameron argues that modern AI can help settle the debate. In particular, he suggests we focus on what types of psychological "domain-general faculties" underlie our own intelligence, and how different kinds of deep learning models are revealing how those faculties may be implemented in our brains. The hope is that by building systems that possess the right handful of faculties, and putting those systems together in a way they can cooperate in a general and flexible manner, it will result in cognitive architectures we would call intelligent. Thus, what Cameron calls The New DoGMA: Domain-General Modular Architecture. We also discuss his work on mental representation and how representations get their content - how our thoughts connect to the natural external world.
0:00 - Intro 4:55 - Interpreting old philosophy 8:26 - AI and philosophy 17:00 - Empiricism vs. rationalism 27:09 - Domain-general faculties 33:10 - Faculty psychology 40:28 - New faculties? 46:11 - Human faculties 51:15 - Cognitive architectures 56:26 - Language 1:01:40 - Beyond dichotomous thinking 1:04:08 - Lower-level faculties 1:10:16 - Animal cognition 1:14:31 - A Forward-Looking Theory of Content | |||
05 Aug 2022 | BI 143 Rodolphe Sepulchre: Mixed Feedback Control | 01:24:53 | |
Check out my free video series about what's missing in AI and Neuroscience Support the show to get full episodes and join the Discord community. Rodolphe Sepulchre is a control engineer and theorist at Cambridge University. He focuses on applying feedback control engineering principles to build circuits that model neurons and neuronal circuits. We discuss his work on mixed feedback control - positive and negative - as an underlying principle of the mixed digital and analog brain signals,, the role of neuromodulation as a controller, applying these principles to Eve Marder's lobster/crab neural circuits, building mixed-feedback neuromorphics, some feedback control history, and how "If you wish to contribute original work, be prepared to face loneliness," among other topics.
0:00 - Intro 4:38 - Control engineer 9:52 - Control vs. dynamical systems 13:34 - Building vs. understanding 17:38 - Mixed feedback signals 26:00 - Robustness 28:28 - Eve Marder 32:00 - Loneliness 37:35 - Across levels 44:04 - Neuromorphics and neuromodulation 52:15 - Barrier to adopting neuromorphics 54:40 - Deep learning influence 58:04 - Beyond energy efficiency 1:02:02 - Deep learning for neuro 1:14:15 - Role of philosophy 1:16:43 - Doing it right | |||
17 Aug 2022 | BI 144 Emily M. Bender and Ev Fedorenko: Large Language Models | 01:11:41 | |
Check out my short video series about what's missing in AI and Neuroscience. Support the show to get full episodes and join the Discord community. Large language models, often now called "foundation models", are the model de jour in AI, based on the transformer architecture. In this episode, I bring together Evelina Fedorenko and Emily M. Bender to discuss how language models stack up to our own language processing and generation (models and brains both excel at next-word prediction), whether language evolved in humans for complex thoughts or for communication (communication, says Ev), whether language models grasp the meaning of the text they produce (Emily says no), and much more. Evelina Fedorenko is a cognitive scientist who runs the EvLab at MIT. She studies the neural basis of language. Her lab has amassed a large amount of data suggesting language did not evolve to help us think complex thoughts, as Noam Chomsky has argued, but rather for efficient communication. She has also recently been comparing the activity in language models to activity in our brain's language network, finding commonality in the ability to predict upcoming words. Emily M. Bender is a computational linguist at University of Washington. Recently she has been considering questions about whether language models understand the meaning of the language they produce (no), whether we should be scaling language models as is the current practice (not really), how linguistics can inform language models, and more.
0:00 - Intro 4:35 - Language and cognition 15:38 - Grasping for meaning 21:32 - Are large language models producing language? 23:09 - Next-word prediction in brains and models 32:09 - Interface between language and thought 35:18 - Studying language in nonhuman animals 41:54 - Do we understand language enough? 45:51 - What do language models need? 51:45 - Are LLMs teaching us about language? 54:56 - Is meaning necessary, and does it matter how we learn language? 1:00:04 - Is our biology important for language? 1:04:59 - Future outlook | |||
28 Aug 2022 | BI 145 James Woodward: Causation with a Human Face | 01:25:52 | |
Check out my free video series about what's missing in AI and Neuroscience Support the show to get full episodes and join the Discord community. James Woodward is a recently retired Professor from the Department of History and Philosophy of Science at the University of Pittsburgh. Jim has tremendously influenced the field of causal explanation in the philosophy of science. His account of causation centers around intervention - intervening on a cause should alter its effect. From this minimal notion, Jim has described many facets and varieties of causal structures. In this episode, we discuss topics from his recent book, Causation with a Human Face: Normative Theory and Descriptive Psychology. In the book, Jim advocates that how we should think about causality - the normative - needs to be studied together with how we actually do think about causal relations in the world - the descriptive. We discuss many topics around this central notion, epistemology versus metaphysics, the the nature and varieties of causal structures.
0:00 - Intro 4:14 - Causation with a Human Face & Functionalist approach 6:16 - Interventionist causality; Epistemology and metaphysics 9:35 - Normative and descriptive 14:02 - Rationalist approach 20:24 - Normative vs. descriptive 28:00 - Varying notions of causation 33:18 - Invariance 41:05 - Causality in complex systems 47:09 - Downward causation 51:14 - Natural laws 56:38 - Proportionality 1:01:12 - Intuitions 1:10:59 - Normative and descriptive relation 1:17:33 - Causality across disciplines 1:21:26 - What would help our understanding of causation | |||
07 Sep 2022 | BI 146 Lauren Ross: Causal and Non-Causal Explanation | 01:22:51 | |
Check out my free video series about what's missing in AI and Neuroscience Support the show to get full episodes and join the Discord community. Lauren Ross is an Associate Professor at the University of California, Irvine. She studies and writes about causal and non-causal explanations in philosophy of science, including distinctions among causal structures. Throughout her work, Lauren employs Jame's Woodward's interventionist approach to causation, which Jim and I discussed in episode 145. In this episode, we discuss Jim's lasting impact on the philosophy of causation, the current dominance of mechanistic explanation and its relation to causation, and various causal structures of explanation, including pathways, cascades, topology, and constraints.
0:00 - Intro 2:46 - Lauren's background 10:14 - Jim Woodward legacy 15:37 - Golden era of causality 18:56 - Mechanistic explanation 28:51 - Pathways 31:41 - Cascades 36:25 - Topology 41:17 - Constraint 50:44 - Hierarchy of explanations 53:18 - Structure and function 57:49 - Brain and mind 1:01:28 - Reductionism 1:07:58 - Constraint again 1:14:38 - Multiple realizability | |||
13 Sep 2022 | BI 147 Noah Hutton: In Silico | 01:37:08 | |
Check out my free video series about what's missing in AI and Neuroscience Support the show to get full episodes and join the Discord community. Noah Hutton writes, directs, and scores documentary and narrative films. On this episode, we discuss his documentary In Silico. In 2009, Noah watched a TED talk by Henry Markram, in which Henry claimed it would take 10 years to fully simulate a human brain. This claim inspired Noah to chronicle the project, visiting Henry and his team periodically throughout. The result was In Silico, which tells the science, human, and social story of Henry's massively funded projects - the Blue Brain Project and the Human Brain Project. 0:00 - Intro 3:36 - Release and premier 7:37 - Noah's background 9:52 - Origins of In Silico 19:39 - Recurring visits 22:13 - Including the critics 25:22 - Markram's shifting outlook and salesmanship 35:43 - Promises and delivery 41:28 - Computer and brain terms interchange 49:22 - Progress vs. illusion of progress 52:19 - Close to quitting 58:01 - Salesmanship vs bad at estimating timelines 1:02:12 - Brain simulation science 1:11:19 - AGI 1:14:48 - Brain simulation vs. neuro-AI 1:21:03 - Opinion on TED talks 1:25:16 - Hero worship 1:29:03 - Feedback on In Silico | |||
25 Sep 2022 | BI 148 Gaute Einevoll: Brain Simulations | 01:28:48 | |
Check out my free video series about what's missing in AI and Neuroscience Support the show to get full episodes and join the Discord community. Gaute Einevoll is a professor at the University of Oslo and Norwegian University of Life Sciences. Use develops detailed models of brain networks to use as simulations, so neuroscientists can test their various theories and hypotheses about how networks implement various functions. Thus, the models are tools. The goal is to create models that are multi-level, to test questions at various levels of biological detail; and multi-modal, to predict that handful of signals neuroscientists measure from real brains (something Gaute calls "measurement physics"). We also discuss Gaute's thoughts on Carina Curto's "beautiful vs ugly models", and his reaction to Noah Hutton's In Silico documentary about the Blue Brain and Human Brain projects (Gaute has been funded by the Human Brain Project since its inception).
0:00 - Intro 3:25 - Beautiful and messy models 6:34 - In Silico 9:47 - Goals of human brain project 15:50 - Brain simulation approach 21:35 - Degeneracy in parameters 26:24 - Abstract principles from simulations 32:58 - Models as tools 35:34 - Predicting brain signals 41:45 - LFPs closer to average 53:57 - Plasticity in simulations 56:53 - How detailed should we model neurons? 59:09 - Lessons from predicting signals 1:06:07 - Scaling up 1:10:54 - Simulation as a tool 1:12:35 - Oscillations 1:16:24 - Manifolds and simulations 1:20:22 - Modeling cortex like Hodgkin and Huxley | |||
05 Oct 2022 | BI 149 William B. Miller: Cell Intelligence | 01:33:54 | |
Check out my free video series about what's missing in AI and Neuroscience Support the show to get full episodes and join the Discord community. William B. Miller is an ex-physician turned evolutionary biologist. In this episode, we discuss topics related to his new book, Bioverse: How the Cellular World Contains the Secrets to Life's Biggest Questions. The premise of the book is that all individual cells are intelligent in their own right, and possess a sense of self. From this, Bill makes the case that cells cooperate with other cells to engineer whole organisms that in turn serve as wonderful hosts for the myriad cell types. Further, our bodies are collections of our own cells (with our DNA), and an enormous amount and diversity of foreign cells - our microbiome - that communicate and cooperate with each other and with our own cells. We also discuss how cell intelligence compares to human intelligence, what Bill calls the "era of the cell" in science, how the future of medicine will harness the intelligence of cells and their cooperative nature, and much more.
0:00 - Intro 3:43 - Bioverse 7:29 - Bill's cell appreciation origins 17:03 - Microbiomes 27:01 - Complexity of microbiomes and the "Era of the cell" 46:00 - Robustness 55:05 - Cell vs. human intelligence 1:10:08 - Artificial intelligence 1:21:01 - Neuro-AI 1:25:53 - Hard problem of consciousness | |||
15 Oct 2022 | BI 150 Dan Nicholson: Machines, Organisms, Processes | 01:38:29 | |
Support the show to get full episodes and join the Discord community. Check out my free video series about what's missing in AI and Neuroscience Dan Nicholson is a philosopher at George Mason University. He incorporates the history of science and philosophy into modern analyses of our conceptions of processes related to life and organisms. He is also interested in re-orienting our conception of the universe as made fundamentally of things/substances, and replacing it with the idea the universe is made fundamentally of processes (process philosophy). In this episode, we both of those subjects, the why the "machine conception of the organism" is incorrect, how to apply these ideas to topics like neuroscience and artificial intelligence, and much more.
0:00 - Intro 2:49 - Philosophy and science 16:37 - Role of history 23:28 - What Is Life? And interaction with James Watson 38:37 - Arguments against the machine conception of organisms 49:08 - Organisms as streams (processes) 57:52 - Process philosophy 1:08:59 - Alfred North Whitehead 1:12:45 - Process and consciousness 1:22:16 - Artificial intelligence and process 1:31:47 - Language and symbols and processes | |||
30 Oct 2022 | BI 151 Steve Byrnes: Brain-like AGI Safety | 01:31:17 | |
Support the show to get full episodes and join the Discord community. Steve Byrnes is a physicist turned AGI safety researcher. He's concerned that when we create AGI, whenever and however that might happen, we run the risk of creating it in a less than perfectly safe way. AGI safety (AGI not doing something bad) is a wide net that encompasses AGI alignment (AGI doing what we want it to do). We discuss a host of ideas Steve writes about in his Intro to Brain-Like-AGI Safety blog series, which uses what he has learned about brains to address how we might safely make AGI. | |||
08 Nov 2022 | BI 152 Michael L. Anderson: After Phrenology: Neural Reuse | 01:45:11 | |
Check out my free video series about what's missing in AI and Neuroscience Support the show to get full episodes and join the Discord community. Michael L. Anderson is a professor at the Rotman Institute of Philosophy, at Western University. His book, After Phrenology: Neural Reuse and the Interactive Brain, calls for a re-conceptualization of how we understand and study brains and minds. Neural reuse is the phenomenon that any given brain area is active for multiple cognitive functions, and partners with different sets of brain areas to carry out different cognitive functions. We discuss the implications for this, and other topics in Michael's research and the book, like evolution, embodied cognition, and Gibsonian perception. Michael also fields guest questions from John Krakauer and Alex Gomez-Marin, about representations and metaphysics, respectively.
0:00 - Intro 3:02 - After Phrenology 13:18 - Typical neuroscience experiment 16:29 - Neural reuse 18:37 - 4E cognition and representations 22:48 - John Krakauer question 27:38 - Gibsonian perception 36:17 - Autoencoders without representations 49:22 - Pluralism 52:42 - Alex Gomez-Marin question - metaphysics 1:01:26 - Stimulus-response historical neuroscience 1:10:59 - After Phrenology influence 1:19:24 - Origins of neural reuse 1:35:25 - The way forward |